Teaching Data Science with AI at Harvard Business School
speakers
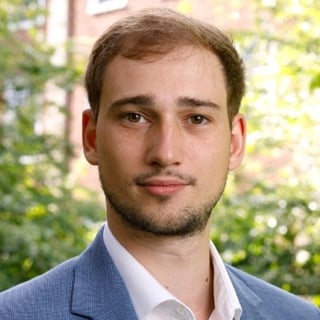
Iavor Bojinov is an Assistant Professor of Business Administration and the Richard Hodgson Fellow at Harvard Business School. He is the co-PI of the AI and Data Science Operations Lab and a faculty affiliate in the Department of Statistics at Harvard University and the Harvard Data Science Initiative. His research focuses on developing novel statistical methodologies to make business experimentation more rigorous, safer, and efficient, specifically homing in on the application of experimentation to the operationalization of artificial intelligence (AI), the process by which AI products are developed and integrated into real-world applications. His work has been published in top academic journals such as Annals of Applied Statistics, Biometrika, The Journal of the American Statistical Association, The Journal of Econometrics, Quantitative Economics, Management Science, and Science, and has been cited in Forbes, The New York Times, The Washington Post, and Reuters, among other outlets. More broadly, as one of the few scholars who work at the intersection of data science and business, he was the first author to have spotlight featured articles in both the Harvard Business Review and the Harvard Data Science Review.
Professor Bojinov is also the co-creator of the first-year required MBA course “Data Science and AI for Leaders” and has previously taught the “Competing in the Age of AI” and “Technology and Operations Management” courses. Before joining Harvard Business School, Professor Bojinov worked as a data scientist leading the causal inference effort within the Applied Research Group at LinkedIn. He holds a Ph.D. and an MA in Statistics from Harvard and an MSci in Mathematics from King’s College London.
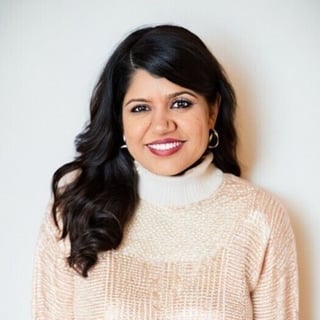
Siya Raj Purohit is an education leader, author, and investor. She works on Education at OpenAI and is a General Partner at Pathway Ventures, an early-stage fund investing in the future of learning and work. Siya was previously an early employee at Udacity and Springboard, an investor at GSV Ventures, and the founding EdTech/Workforce category lead for AWS Marketplace. She is the author of Engineering America, a book on the country's jobs-skills gap
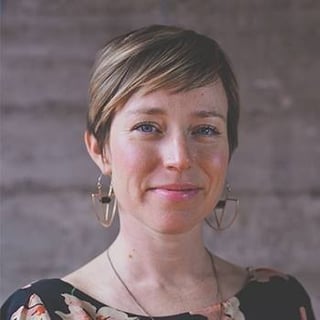
Natalie Cone launched and now manages OpenAI’s interdisciplinary community, the Forum. The OpenAI Forum is a community designed to unite thoughtful contributors from a diverse array of backgrounds, skill sets, and domain expertise to enable discourse related to the intersection of AI and an array of academic, professional, and societal domains. Before joining OpenAI, Natalie managed and stewarded Scale’s ML/AI community of practice, the AI Exchange. She has a background in the Arts, with a degree in History of Art from UC, Berkeley, and has served as Director of Operations and Programs, as well as on the board of directors for the radical performing arts center, CounterPulse, and led visitor experience at Yerba Buena Center for the Arts.
SUMMARY
The OpenAI Forum's first event of 2025 brought together Assistant Professor Iavor Bojinov from Harvard Business School (HBS) and Siya Raj Purohit from OpenAI's Education Team for a compelling discussion on the integration of artificial intelligence into education and business learning. Hosted by Natalie Cone, the forum highlighted groundbreaking efforts to establish AI-native universities and innovative strategies for incorporating AI into teaching and learning.
Siya Raj Purohit introduced the audience to OpenAI for Education's initiatives, particularly the launch of ChatGPTEDU in 2024. This enterprise-grade platform offers secure and collaborative tools for educators, students, and institutions to deepen engagement with knowledge. ChatGPTEDU fosters network effects on campuses by enabling professors to create custom solutions for their classes and allowing students to engage with content in an interactive, conversational manner. Siya also shared OpenAI's broader vision for AI-native universities, where students experience AI touchpoints throughout their academic journey—from orientation to career services and beyond. These innovations are designed to empower students to achieve their goals with greater efficiency and creativity.
Professor Iavor Bojinov shared the journey of integrating AI into the MBA curriculum at HBS. He detailed the development of the school's first AI-native course, "Data Science and AI for Leaders," which emphasizes foundational data science, AI, machine learning, and managerial decision-making. This course reflects a significant shift in business education, incorporating tools like ChatGPT, custom tutor bots, and advanced analytics bots to support interactive and practical learning. By redesigning the course as an AI-native experience, HBS has replaced traditional coding lessons with a focus on prompting, enabling students to engage deeply with data analysis while still addressing managerial challenges related to AI integration.
During his presentation, Professor Bojinov highlighted key lessons learned from his pioneering efforts. Training, he emphasized, is essential for students and faculty to adopt AI tools effectively. He also noted the importance of aligning AI applications with the curriculum to prevent confusion and ensure meaningful learning outcomes. Exposing students to a variety of AI platforms prepares them for real-world scenarios where multiple tools are used in tandem, and understanding the strengths and limitations of these tools is vital for success.
One of the standout features of the event was Professor Bojinov's hands-on teaching exercise, the "AI-First Snack Company." This activity enables students to explore AI's capabilities in market research, product development, and marketing strategies, providing an interactive way to learn prompting techniques and understand the creative potential of generative AI. The exercise also underscores AI's limitations, offering a balanced perspective on its applications in problem-solving and innovation.
The session concluded with announcements of upcoming events, including a panel discussion with OpenAI’s new VP of Education, Leah Belsky, and other academic leaders, as well as a session featuring the integration of AI in research led by Dr. Rojansky from Stanford University. The OpenAI Forum continues to prioritize meaningful collaboration and invites members to engage actively through its global chapters and referral network.
This event set the tone for 2025, providing valuable insights into the transformative potential of AI in education and fostering collaboration among academics and professionals. The discussions and initiatives presented underscored the pivotal role AI is poised to play in shaping the future of learning and professional development.
TRANSCRIPT
I'm Natalie Cone, your OpenAI Forum Community Architect, and it's always my pleasure to host all of you. I like to start all of our events by reminding us of OpenAI's mission, which is to ensure that artificial general intelligence, AGI, benefits all of humanity.
For our first forum event of 2025, Iavor, this is our very first event, thank you for opening us up. We're going to be welcoming Iavor, Bojinov and my colleague on the education team at OpenAI, Siya Raj Purohit.
Iavor, Bojinov is an assistant professor of business administration and the Richard Hodgson Fellow at Harvard Business School. He's the co-principal investigator of the AI and Data Science Operations Lab and the faculty affiliate in the Department of Statistics at Harvard University and the Harvard Data Science Initiative. His research focuses on developing novel statistical methodologies to make business experimentation more rigorous, safer, and efficient, specifically homing in on the application of experimentation to the operationalization of artificial intelligence, the process by which AI products are developed and integrated into real-world applications.
His work has been published in top academic journals, such as Annals of Applied Statistics, the Journal of American Statistical Association, the Journal of Econometrics, Quantitative Economics, Management Science, and Science, among many more. Iavor, you're definitely giving me a good tongue twister there.
The New York Times, the Washington Post, and Rutgers, among many other outlets. More broadly, as one of the few scholars who work at the intersection of data science and business, he was the first author to have spotlight featured articles in both the Harvard Business Review and the Harvard Data Science Review.
Professor Bojinov is also the co-creator of the first-year required MBA course, Data Science and AI for Leaders, and has previously taught the Competing in the Age of AI and Technology and Operations Management courses. Before joining Harvard Business School, Professor Bojinov worked as a data scientist leading the causal inference effort within the Applied Research Group at LinkedIn. He holds a PhD and an MA in statistics from Harvard and an MSI in mathematics from King's College London.
My colleague, Siya Raj Purohit is an education leader, author, and investor. She works on education at OpenAI and is a general partner at Pathway Ventures, an early-stage fund investing in the future of learning and work. Sia was previously an early employee at Udacity and Springboard, an investor at GSV Ventures, and the founding EdTech workforce category lead for AWS Marketplace. She is the author of Engineering America, a book on the country's job skills gap.
Please help me, community, in welcoming my awesome colleague, Siya.
Amazing. Thank you so much, Natalie. And thank you so much, everyone, for joining tonight. It's great to see so many educators in the forum joining live today. So I'm going to provide a little bit of context about what OpenAI for Education is working on and how we're thinking about AI-native universities. And then I'll pass it over for the main session.
High level, the goal of OpenAI for Education is to get ChatGPT in the hands of all educators, knowledge workers, and students around the world. One of the products we have launched under OpenAI for Education is ChatGPTEDU. So as many of you might have heard, we launched ChatGPTEDU last summer. It provides enterprise-grade security and a lot of workspace features for universities and school districts to adopt. One of the most exciting things about ChatGPTEDU is the type of network effects that it enables. Professors are able to create solutions for their classes and across their campus, something we'll talk a lot more about today. And students are able to engage with the knowledge of their university in a much deeper, more conversational way.
Our aspiration for AI-native universities is that campuses will have multiple AI touchpoints across. So when a student comes from orientation through their classes and career services and clubs, they're going to have a lot of different AI touchpoints that help them understand the knowledge of the university more deeply and be able to solve for their own goals and work towards their own ambitions more effectively. So that's our aspiration. We're working with a lot of great universities, very excited about our partnership with Harvard Business School, and very excited to be able to feature some of these great use cases.
Across the board, the most impactful use cases we're seeing with ChatGPTEDU are around lecture recall. Being able to engage deeply with a professor's content, being able to ask questions about it, like, which CEO handled layoffs well, and get exact case studies and examples that help indicate that. We're seeing this used a lot for exam preparations. Students are like, these are the questions my stats professor's been asking. Can you create additional quizzes or tests for me? And just be able to practice that way. And then, of course, as a super-powered assistant, all of us are using it to improve our emails and our executive communication. And it's been really great to be able to see that kind of impact in campus.
If you're interested in learning more about OpenAI for Education, we just launched a LinkedIn page. Caitlin will share that in chat here. Basically, follow our LinkedIn page, learn more about what we're doing there. And also a sub-stack we recently launched to profile some professors who are doing incredible work. And if you're interested in learning more about ChatGPTEDU, feel free to send me a message on LinkedIn or over the OpenAI forum. And now I'll pass it over to Iavor to talk about how he's using this at Harvard Business School. Thank you so much.
So exciting to see all of the amazing work you guys are doing on trying to bring in ChatGPT and other generative AI tools into the classroom. First of all, thank you so much for inviting me to share some of this. Let me just quickly share some slides that I've put together.
Now I want to begin by prefacing this that this isn't just my work. This is many other people have been involved in the creation of the courses and the materials that I'm talking about. And so huge shout out to all of my colleagues that have worked with me on this. So none of this is just my work, just to be very clear. It's very much joint work.
Now I'm going to basically my plan is I'm going to talk to you a little bit about this new course we've developed on data science. And I'm going to talk to you about how we've integrated and really tried to rebuild it from the ground up as an AI-native course. And that's basically all I'm going to talk to you about. But before we get started, I noticed there were many people from other universities. And so I wanted to spend a moment just telling you a little bit about HBS and sort of the Harvard Business School experience. I'm assuming no one has complained, I'm assuming all the slides are working and everything. So I'm going to just keep going unless someone tells me to stop.
Essentially, we have a two-year MBA program. It's in person, it's full-time. And essentially, all of our classes are basically this coliseum style where all of the students sit around. And there's a faculty in the middle, lots of boards, they ask questions, and you deeply debate and discuss a case. And that's basically what they do for every one of not, I wouldn't say every one of the classes, but the vast majority of our classes. The first year is basically all required. So all of the students, we've got about 950 of them, just under a thousand, they all take the same set of courses, pretty much at the same time. And so we take these students, we break them up into 10 sections, and they all have this very sort of similar experience.
To give you a sense of some of the classes that they're taking, you know, it's what you would expect. So things like finance, or financial reporting control, which is our accounting scores, leadership, marketing, technology and operations management, strategy, business, government, international economy, etc. You know, these are like, really what we think of as the core foundational things that every manager and every business leader needs to understand.
Now what you're probably noticing, and this is sort of pre-2023, is that there's no course on data science or AI. That's a new course that we added. So that's what I'm going to be talking to you about.
Okay, so what does the student experience look like? What is it preparing for class? Actually, one thing just to mention. The first year is all required, everyone takes the same classes. The second year is all elective, so the students pick to study whatever they're interested in.
So hopefully that gives you a good sense of what the MBA curriculum looks like. What does a typical class look like? Well, there are three things our students do in the first year. The first thing is they read the case. And this is basically a scenario or a situation that explains a business use case and presents the students with some questions. So here, this is an example of a case I wrote on the Warriors, the NBA basketball team. So I'm assuming a lot of you are in California, so I thought I should talk about my California case. And this case really follows Ray Yockey, who at the time was the senior director of business analytics and strategy, and he was tasked with building a whole data science group at the Warriors for sort of business data science.
So the students will get given this case. Typically, they'll have two or three classes, so there will be two or three cases to read before each class. In addition to the case, they're given an assignment, which is a set of questions for students to think about. So for example, here, it could be something like, why did the Warriors invest in data science? And then more detailed things like, in addition to the data that's listed in the case, what other data might they use to really build models around customer types, etc. So these are the types of things that the students should really think about when they're preparing for class.
Once they've read the case, they thought about it. They have a discussion with their discussion group. These are basically other peers in different classes, so they come together and they talk about all the cases that will be discussed that day, and that's essentially the preparation. Then they come to class, we have a discussion, and we sort of inductively try to come up with lessons. So this type of case teaching is very common in many business schools. Law schools tend to teach like this. The HBS model very much is inspired by the law school, which sort of predates us a little bit. And so this is essentially how we do all of our teaching.
Now, what did we do? Well, we started in January 2021, and the story is quite interesting. Essentially COVID happened, and we had to cancel one of our field courses where we would send our MBA students to go and work with a company, and because no one was traveling, we canceled that course. And so there was space in the curriculum to add a new data science course, and they asked us to build this sort of the first version of this data science for managers course. And so we started working on it in January 2021. We iterated on them many times, and in September 2023, it was added to the required curriculum. And so along with finance, accounting, etc., our students were starting to learn about data science for managers.
So what did we teach them? We basically teach them... We tried to teach them the foundations of statistics.
Give you some examples, more explicit examples of the types of topics we covered. We also, at the time, this is, remember, we started this course in January 2021. Of course, ChatGPT was out, but not really in the UI. It wasn't really, frankly, it's still 3.5 wasn't out. It wasn't very good at the time.
And so we made, oh, Paul, a new slide's being shown. They should be. Are you still seeing the, Natalie, are you having this issue? I see data science for managers, September, 2023. Perfect, so that is the right slide. Just keeping track of the chat.
Okay, so in chat, in January, 2021, we strongly believe that to teach data science, you really need to teach people how to code. And so that's when we made the decision that we're going to teach students the basics of coding. And we decided to do it in R instead of Python because it's a little bit easier to get started.
And of course, in addition to the statistics and the coding, we focused a lot on sort of the managerial questions related to building, managing, and working with data science organizations.
And the course, keeping in the HBS tradition, was very much designed to still use cases. So we built the course, we were really excited. We iterated, we taught it multiple times in the second year elective curriculum. We taught it in the first year as an optional course so people could sign up in addition to their required courses. They could sign up to learn about data science.
And then in September, actually it was August because we sort of began on August 29th or something like that, whatever the Monday or Tuesday is, we were ready to launch this course.
Now, for some of you who are academics, you probably remember that this was the summer when most universities were really freaking out. And this is, you know, most essay-based classes were really worried around how do you still evaluate students if you give them an assignment? Are they going to cheat and use Chad GPT to just write the essay?
And so there was a lot of panic and people were really starting to get very, very nervous. Now, because I was teaching this data science course, I felt great because at the time, Chad GPT was really bad at doing, solving statistical problems or analytics problems.
And so I felt really good. And I remember on July 16th, 2023, I was teaching this executive education course on the promise of AI. And the whole class was basically showing, teaching them sort of the basics of machine learning and AI, so supervised, unsupervised reinforcement. And then we were, I was going to do a live demo of how you can use generative AI. And I think at the time, most people still had not really used Chad GPT. I remember asking, you know, how many people have tried this? In a room of 90 people, maybe about 10 had tried it before, 20 had heard of it. So it was still, you know, even though this is only a year and a half ago, not that many people were using it.
I remember literally the day before, or maybe it was two days before, I remember going to Chad GPT and suddenly being able to upload data sets and it was able to spin up a virtual machine. It was able to start writing Python code and it was able to execute.
Now, of course, the video I'm sharing is not from exactly that moment. This is a much more recent video, but this was, to me, a big step change in how we teach data science and how we teach machine learning because suddenly we were able to have an additional layer of abstraction if you think about all the languages like Fortran and C, Python provided a layer of abstraction and it made it much easier to learn coding for people who were getting started.
And here, we suddenly had this amazing tool that was able to write code, do data analysis. And so my initial reaction was to freak out because this is July, mid-July. In six weeks, for the first time ever, we're starting to teach this data science for managers course to a thousand MBA students.
And I kept laughing at all these other professors that were freaking out about people being able to write essays, but certainly my whole teaching plan goes out the window because you can now do all of the data analysis that I had planned and prepared. You could just go and do it in ChatGPT.
And so I just remember spending a whole afternoon trying to figure out, well, what is the solution? And as Nalini told you, a lot of my research is on experimentation and I thought, hey, why not just give ChatGPT to everyone? Right, like let's just give it to everyone and see what happens.
And I have to give a huge amount of credit to my colleague, Jan Hammond, who at the time was the course head. She believed in me and believed that this crazy idea could be pulled off. And so in the space of six weeks, she was able to petition the school and convince them to get everyone access with ChatGPT.
Now for context, at the time, there was no ChatGPT enterprise. I actually think it launched the day that we sort of launched the course. But of course, IT groups take forever, so there was no way we would be able to pull this off.
And so what we did was we basically said, okay, all of the students can buy their own ChatGPT accounts, premium accounts, for three months, and we're going to reimburse them. Now we found out maybe a week before we started teaching the course that we were able to do this and we're able to give all the permissions and all the support.
Again, you cannot imagine the lift that happened both on the administration side and the number of faculty and sort of our leaders on the education side who sort of made this happen.
But we didn't really have any time to come up with any type of training. We literally received the permission days before the whole thing started. There was no real integration into the course.
We added, for a few exercises, we basically said, you know, go on ChatGPT and try to replicate this analysis using, I think at the time it was advanced data analysis and then change the code interpreter. I went through several iterations of names.
Every time I would try to make a video to showcase how to use it, the UI would change and something would change. And so the shelf life of every, all the material we're creating was basically zero.
And one thing we did say, of course, was that you couldn't use this tool during our exams. And I'll tell you one fun anecdote.
So the plan was all of the faculty in the very first class. So the very first class we taught was on the data science at the Warriors. So the case I showed you, that was our very first case. And in class, we had every student basically put up their laptop. And if you know anything about HBS, we don't allow students to use laptops in class.
So this was also already a big deal was to let them use laptops in class. So they pulled up their laptops, they did their analysis. And then with about 10 minutes to go, I said, okay, who's heard of ChatGPT? And maybe 20 hands go up.
And I'm like, who's used it? Maybe 10 hands go up. And then I was like, okay, let me give you a demo. So I pull up my laptop and I go to ChatGPT. And of course, ChatGPT is down. And so I can't, like the whole website was down. I couldn't load it. So now it's much more stable, but I think we've forgotten that this is a crazy scale that has been, you know, has grown like crazy.
But even in August, it hadn't even been a year since it was launched, it was still reasonably unstable at times. And so luckily I had a second tab. So this is a lesson I learned from one of my colleagues, Mitch Wise, which is never have, whenever you're doing a live demo, make sure you have a backup version.
And so I had a backup version that had preloaded all the analysis. So I showed them and I was able to walk through them. So here is the big aha. So what did we learn from this crazy experiment of giving a thousand students ChatGPT before ChatGPT Enterprise?
I'm pretty sure we were the first business school to do this at that scale. I wouldn't be surprised if we were the first school to try something like this. And so what did we learn?
The first thing we learned was you need training. As much as we think this is a very intuitive tool, there are things that you learn and these learnings can be shared. And it's super important that you prepare proper training, especially for sort of that bottom 10, 20% of people who are really terrified of this technology.
The second thing we learned was that ChatGPT did not follow our syllabus. And what was really interesting was that we had, of course, we had prepared students and we tried to teach them how to code and all of the assignments, we had many assignments on basics of coding and how to use it, et cetera.
And a lot of students, they would try to do the same analysis on ChatGPT and then they would go beyond the things that they had learned. Because of course, we weren't using any sort of rag architecture, it was just the generic vanilla version.
And so it would do all these analysis and the students just didn't know what those were, they could try to figure it out. And so that was a really big aha for us, which was, if you want to use this and you want to make sure the students are progressing in the stages that you want them to progress, as an educator, you have to think very, very carefully around how do you ensure that the tools you're using stay close to your syllabus.
The third thing that we learned was when things go wrong, they do really, really go wrong. And I think this is just sort of building on this, didn't follow their syllabus.
We had a number of students that tried it, it didn't work for them, it didn't give them the right answers. And they were frankly quite annoyed.
And full-on just were like, I'm not using this tool again. And this was completely our fault. Because as I told you, we didn't provide any educational material. We didn't provide them any guidance. It was very much, well, what happens if we throw this in the wild? Can they do stuff? The answer is, they sort of could, but things could go wrong. And it was really sort of very, very unstructured.
OK, what else did we learn? One thing that we were really excited about was that when you remove the coding element, students could go much further. And remember, this was a data science for managers course. We weren't really teaching them coding. The reason we were teaching them coding was really to think algorithmically, so they could interact with data scientists. We weren't training them to be data scientists. But because we were teaching them how to code, we were really limited on how much statistics and how much data analysis and data intuition and teaching them how to really use their intuition and judgment for data and how that data can inform their intuition.
And so this allowed us, we saw there was a glimmer of hope that this could be used to really go beyond and go further into that data science curriculum that we cared about. And the fifth thing we learned, and this is probably the most important thing, is that you could actually teach data science using Gen AI tools. And so what did we do? Well, we realized that, obviously, our experiment, we learned something from it. I wouldn't say it was the biggest success. Some people loved the tool. Some people, we probably got a lot of people quite annoyed and negative about it. But I think more people came out being really positive.
And so what we decided to do was to redesign the course and build what we think of as the first AI-native course, at least at HBS. And what I mean by that is, and I'll get into the details of the technology of what we're doing is, but we basically wanted to ensure that we had a course bot that was fully integrated into the pedagogy. And I'll get into all the details of all of this.
And we updated, of course, the curriculum. So instead of just teaching them data science, we're teaching them data science and AI. Instead of just teaching them statistics, we're leaning a lot more into machine learning and AI. And we're not teaching them coding anymore. We're really teaching them the foundations of prompting for data analysis and everyday work. And of course, we're still teaching them all of the managerial questions related to building, managing, and working with data science and AI organizations.
So yeah, one thing to say, we haven't actually taught the course. We're starting on February 5th. So wish me luck, and wish the other six faculty that are doing this with me. Wish us luck. This is, again, going to be a huge experiment. But let me dive into and just tell you a little bit more about what we're teaching.
So on the technical side of it, sort of the statistics side, we're teaching things like exploratory data analysis, some hypothesis testing, regression for interpretation, but also for prediction and forecasting, decision trees, and k-means. So these are our technical topics. Of course, if you're a statistician, this is very, very basic stuff. But you have to remember, we have a very wide range of expertise in MBA students. Some are humanities majors who maybe haven't even taken statistics since they were in high school. And so we have to make sure they have those foundations.
How are we teaching this? We're using lots of cases. Just to give you a little sense of the breadth of cases, and a lot of these are brand new, we have cases on Moderna. OpenAI has a big partnership with Moderna that they've talked about publicly. And so we have a new case that's coming out on their use of GPT Enterprise and how they have over 1,000 custom GPTs. And so that's our very first case that sort of shows them what AI is doing today.
We've got Yelp case, a case on LinkedIn, K Health, Pernod Ricard, one of the biggest alcohol producers in the world, P&G, Procter & Gamble, Coursera, JP Morgan. So we're looking at both very, very large companies and also small tech startups that are being covered in the course. And so our vision, and I sort of mentioned this, is really to ensure that the technology is fully integrated into the pedagogy and that the students interact with the course bot daily.
And so what are the tools that we have? The focus point, I'll get into this in a moment, is our homegrown RAG solution for all of sort of what we call the tutor bot. We're also using Julius AI for all of the data analysis. And we're also using Chad GPT as our generalist.
So if we zoom in, so our tutor bot, this is basically a solution that our digital transformation team has built for us. They've actually built this for several other courses at HBS, and it's been used and very effective. And this is basically RAG architecture. It's built on OpenAI's APIs. And so even though we're not using the interface, we're still, of course, leveraging the underlying large language models.
This is trained on all of our technical nodes. So we have a number of technical nodes on basics of statistics, basics on machine learning, hypothesis testing, visualization, etc. That's all put into the model. We've got a number of books on there, including Karim Lakhani, who's the current course head, the marker in Citi, their book on competing in the age of AI, and several other open source books. We've got a bunch of other courses, which are sort of online courses that we built at HBS for HBS Online that have transcripts of videos, etc. That's all fed in.
We also actually have every single case in the bot. And so as part of the student preparation, they can just go and have a conversation with the bot. And they can ask questions about the case. One of the features that I'm really excited about and I really like is that basically we have these. I don't know if you can see my mouse. I think you can, actually. We have sort of suggested prompts, which we update weekly based on what we're currently covering. And so this is sort of the go-to point. So our students basically go to dcell.ai. It takes them to the bot. And this has links to everything else that they need, including Canvas. So we're still using Canvas. We're still using our analytics bot.
Let me tell you our analytics bot. So this is a wonderful company, Julius AI, that I've gotten to know over the past few years. Maybe Raul, the CEO, might be here. So they're basically a custom solution for doing data analysis, heavily leveraging, of course, OpenAI. The way we built it is they hit our Azure OpenAI points. And we have our own sort of SSO login. And just to be clear, we still have OpenAI EDU.
But there's a couple of reasons why we opted to use this for the data analysis. One of them is, and I'll speak about the tutorials in a moment, but it allows us to define sort of specific workflows so that instead of having a student just have a conversation, there is a predefined set of steps that are done and force the student to follow those steps. And it has some nice features around code editing and explanations, etc. Oh, I think I might even have a couple of screenshots here.
It does a really good job of editing the code and, of course, interactive visualizations, etc. So that's our analysis. And then ChatGPT is our generalist. This is our go-to for doing case updates. So for example, in some of our assignments, and at the very end, I'll give you a little bonus of how I've taught a case without using a case and just using ChatGPT.
So in some of our assignments, we actually ask students to go to ChatGPT and get updates on the company and ask sort of very specific questions. I've done a case where we actually had the students use ChatGPT to analyze the latest earning calls of a company and use that as preparation for the case discussion. Of course, it's an alternative for the tutoring, the data analysis.
One of the key things we're also doing is we're teaching about agents. And so as one of the assignments, we actually have students build custom GPTs. And then we have them do a course project where they build automations of a current job or a past job that they've had or something for their summer internships. And so that's where they're sort of heavily leveraging ChatGPT.
And just sort of a final word on this. One of the reasons we wanted to use multiple tools is because in the real world, they are going to be exposed to multiple tools. They're not just going to be exposed to a single tool. Maybe they're going to have Microsoft's copilots. Maybe you're going to have OpenAI. You might have Anthropic. There's so many tools out there, and we had the point of view that it's important to expose students to multiple tools because they all have their strengths and weaknesses. And I think teaching them the strengths and weaknesses and limitations is a really vital part of the pedagogy.
OK, so let me just get into a little bit more details on how we really deliver on our vision. So I told you about the technology. The way we deliver on the data science portion of it, this is not the sort of the case discussion. This is specifically on the data science bit of it, is we basically have three touch points. We have tutorials, we have assignments, and then we have in-class discussions.
So the tutorials are basically where we teach the basic statistical concepts. These usually involve some sort of video, which we're using, for example, Haygen to generate those videos. And then they have step-by-step guides. So here on the right, I'm just giving you an example of the task. So it explains what you're trying to do. And then it gives you a specific prompt to achieve this. Now, one of the key things about the tutorials is that we have designed them to be as rigid as possible because we don't want variation. We want our 1,000 students to experience the prompt output in as little variation as possible so that we can ensure that all of these students have the same sort of foundational understanding. And this was probably one of the hardest things for us to build, was to ensure that our prompts really lead to consistent answers that are replicatable across either 1,000 people. Now, we haven't tested it with 1,000 people. But when we test it with 20, 30 people, they're really, really stable.
So that's the tutorials. That's sort of the first time you see how a prompt can be used for data analysis. And this is really how we get you started on statistical concepts. Typically.
most of our classes have some sort of tutorial. Now, in addition to that, we also have assignments. So this is in addition to reading the case, a lot of our cases have integrated with data. And so in order for you to understand the key question in the case, you have to do some data analysis.
So this is the piece where we pull back the reins and we allow the students to really have a free-flowing conversation. And we give them some guidance. We give them questions around like, explore this thing, do this, do this. But we don't give them the prompts. The idea is, if you take the prompts from the tutorials, you should be able to tweak them a little bit to achieve everything in the assignment.
And one of the great things about working with Julius is that we can actually track their progress on how far they've gone on the analysis. So we can actually see if they've done question one, if they've done question two, did they get stuck, where did they get stuck, et cetera.
So we can use that information to help them or to really have them to basically help them and ensure that we have all of the necessary preparations in the tutorial. And of course, we also encourage them to use the course board as an RA. This is where they go for all of their technical questions. And actually, our technical notes that we've written have been updated. So they have sample prompts of how to achieve the type of analysis that you're hoping to achieve, what type of prompt to use.
And we even have examples where we'll have them do some additional analysis in chatGPT. Of course, as I said, we want to be using multiple platforms to achieve this. So that's the assignments. And then, of course, in class, we actively encourage them to screen share, walk through the analysis, talk about the prompts. We actually will go through and say, what type of prompt did you use to get this analysis? Share that prompt with the rest of the class.
Why was it so good? Or what prompt did you use that didn't work? So that we can actually get them into this repetitive behavior where they share their learnings. And of course, in class, we can now perform additional analysis.
For all of our business school friends that use HBS cases, we are writing a bunch of teaching notes that explain how we teach this. We have some of these already written. For example, for my data science at the Warriors case, if you look at the teaching note, it actually has a teaching plan step by step how you would teach this, in addition to the prompts you would use to get the data analysis done.
OK. So that's the technical piece of it. The final piece I want to spend a few moments on is the getting started. If you remember at the start, I said, you need some sort of training.
And over the past year, I've spent a lot of time developing this type of training. And I figured out the way to get started is really with snacks. And what do I mean by snacks? Well, I built a hands-on interactive session, which is really around creating an AI-first snack company. This has been taught to several thousand. Maybe we're hitting about 10,000 executives.
And it's, in my opinion, and several of my colleagues' opinion, probably the best way of getting started with ChatGPT. And so why is that? So what do you do? Let me walk you through the exercise really quickly. And by the way, this is our second class in the course.
So we begin with the Moderna case that showcases how that company is integrating generative AI into everything. And then we go into this hands-on experience so they can really get familiar with ChatGPT and the various tools that we have. And so why snacks?
Well, the snack food industry is very large, but it's very traditional. It takes about two to four years to produce a product, and about six to 12 months to actually launch a product. And of course, it's a very fast-growing industry. And so what we've done is I've developed this exercise where in 45 minutes, students will analyze current market trends. They will identify opportunities. They'll create a company. They'll generate a recipe. They'll figure out how to scale that recipe. They'll identify. They'll create a whole marketing strategy. They'll figure out how to market it. And then they'll summarize it. And they will actually create a PowerPoint pitch.
And just to give you, this is a quick snippet of the exercise. So it's very much task and then example prompts. So it's really ready to prepare people. And it doesn't tell you the ideas that it's using, but it's using the best prompting techniques that we have. And so in class, you can then talk about what are the various prompting techniques. You can talk about the various capabilities.
And just to grab your attention to a couple of things, it begins with just asking something like, hey, I'm looking to create a snack company. Give me some questions that help me figure out what type of snack I should go after. And then another example is we get them to go in. Of course, we get them to do data analysis. We get them to use the internet search features. We get them to get feedback and criticize the output, et cetera.
So we try to expose them to the full breadth of how you would do prompting. And what does this look like? I just want to give you a little sense of some of the example companies that have come out of this. So you always get these amazing visuals around what the company is doing. You get some really nice mood boards. And then some people really push it, and they go and use other tools. So they actually create adverts.
Fueled up with Bites, a club-based AI-powered snacks crafted just for you. So whether you're running to a meeting or taking a quick break, Bites keeps your diet gluten-free, high-protein, and AI-friendly. I'll just pause this. This keeps going. This was before people started getting access to SORA. So they were using other tools.
But even in this exercise, for example, we encouraged them to make a jingle, a song to go with this. This is one of my favorite ones. Come, my whispers, and call me cold dreams. It feels like sunshine. It's so peaceful. Such a monotheme of nature's treasures, the pure.
All right, so that can keep going. So why? You might be wondering, why are you doing this? Well, the first point in this, we're actually doing research on this. I actually have a field experiment right now happening with this hands-on generative AI training at a large financial institution in the UK, where we've got a few hundred people getting access to this type of hands-on training and other people not.
So we're trying to actually validate that this works. But the point is, what I've learned is you really need to get people excited about this technology in a very low-risk environment. And that, to me, has been a big aha moment. It lets us really teach them the basics of prompting. And it also allows you to, in one of the, and I haven't finished writing the teaching note on this.
But if you email me, I'll give you my email at the end. I can give you the teaching plan. One of the things that we do, because it exposes you to a huge range of capabilities, one of the things you can do in class is to use this to understand sort of the jagged frontier.
So this is sort of research that has come out of HBS and BCG around how generative AI is really good for some things and kind of bad at other things. And so in class, you get the students to present this. They show how this huge variation, even though everyone had the same prompts, they still come up with completely different products because it allows you, it sort of expands the human creativity that they have.
But then it also allows you to deeply talk about, what are the things that generative AI is good for? And then what are the things that generative AI is not very good at? And so that's one of the key learnings. I already mentioned this, the variation, and it really highlights sort of new ways of working in this.
And so throughout the course and this exercise, we basically are trying to do a free step. What we are thinking of as our free steps to success, which is when it comes to generative AI, we really want people in the beginning, we want to get them comfortable so they understand the capabilities and they're not scared of using generative AI.
So that's what the snack food exercise is really designed for. The rest of the course then uses the various other tools and they're integrated, as I said, in pretty much every one of our classes. So the hope is we get people to adopt this and they start to use this regularly to help them with what they call AI problems.
But that is not the end goal. I think adoption is sort of not the right thing. What we're really aiming for at the end of this course is for people to be proficient to the point where they don't think about using generative AI, they just use it.
In the same way you don't say, I need to write a document so I'm going to use Word or Google Docs. You just know if you need to write a document, go use Word and Google Docs. It's not a task, it's not a problem. And so we want people to get to that level when it comes to AI where it isn't, ooh, should I use AI for this? It's I use AI when it's the right time to use it, which is most of the time.
So that's kind of what we're hoping to achieve. And just that's kind of a quick summary. So what do we do? We've tried to build the first AI native course. Really trying, it's a first year required course. We're beginning in a couple of weeks. We're using the analytics part will be allowed in the exam, but we're not going to use the course part or chat GPT. That's one big decision. I think that's where we landed on, but we're still debating.
And let me, I'll give you just one more fun aside bonus, and then I'll finish and we can have a little discussion and take some Q&A. So for all my business school professors that have taught cases, I did an experiment a couple of weeks ago in this course that I was doing with Marco and sitting on competing in the age of AI.
And we did a case, caseless case. So in the past, we would teach ethical AI using this case on Facebook, and there was a slightly older case. And so instead of using the case, we didn't give them the case, but we just gave them a set of prompts to use on chat GPT. So this is the week after they had done the snack food exercise.
So they're all really excited about chat GPT. And so it was amazing because we were able to have an amazing case conversation even without having a case. And what was beautiful was that, so from the feedback, some people were really interested in the Cambridge Analytica scandal.
So we had one person and she was like, I just spent ages going really deep on Cambridge Analytica. And so she was our Cambridge Analytica go-to person because she knew everything about it. And then we had another person who was really busy that week and they just needed like a five minute summary of all of this stuff.
So they were able to get that using chat GPT. And so it was amazing because we were able to cover the topics we wanted to cover, but also be flexible in sort of people's availability. And so I think this is a really interesting direction. I'm working on basically building a case where it's basically a custom GPT that has the transcripts of case interviews of protection.
and so people can go and have conversations with the protagonist. So we're just trying to reinvent everything, basically, around pedagogy. But I'll stop here. If you want to email me, you can message me, DM me on the forum, or just email me. I have the easiest email. It's ib.hbs.edu. Thank you so much. I hope this was useful for you all.
Iavor, Siya, thank you so much for being here this evening. And Iavor, we're going to come back and tap you to present one more time in the wake of teaching your first course and see how it goes. And hopefully you can invite some of your students as well.
Perfect. Thank you so much, Natalie. And thank you, Siya and Caitlin, for helping run the session. Thank you all.
Totally our pleasure. You are such a pleasure to listen to, Iavor. That was really fun. Thank you. Bye-bye.
So, folks, thank you for joining us this evening. I know it was kind of an early event for the Pacific time zone, but we wanted to make sure that we were also able to include the middle of the United States and the East Coast tonight. So thanks for bearing with us as we explore new times.
We have some really awesome events on the horizon. Please join us next week to meet OpenAI's new VP of Education, Leah Belsky. She's going to host a panel discussion with leaders from top academic institutions to discuss how AI is shaping the future of higher education. And then shortly after that, we'll be hosting Dr. Rozanski, who's an assistant professor and co-director for pathology informatics at Stanford University. She's going to be showing us how she's integrated AI into her research lab, and that should be really fun.
And then we have our first in-person event of the year happening at the end of February. We haven't published it yet. The details are forthcoming. But space for those is always very limited. We're going to live stream it as always, but the in-person space is only 80 people capacity. So please do pay attention when we send you a newsletter or an email.
Some of you are new, so we want to let you know that once you're a member of the forum, the forum is a closed community. It's invite or application only, and that's so that we can continue to have these really meaningful conversations. Some of you I was chatting in the message asking you to please update your profile with a picture. That's because we really aim to keep this community feeling personal and for the conversations to be meaningful and for you to walk away with real collaborators in the world.
But now that you're in the community, you have the agency to invite your peers and your network of experts, and so we're going to drop the referral applications in the chat, and we prioritize membership to all of your referrals.
As I mentioned earlier, we just launched global chapters. So if you're joining us from somewhere else in the world besides the United States, please take a look and see if there's a chapter that's been opened in your region.
And then we have a few. No, that's it, guys. That's it for now. So I hope you had a wonderful evening. It was lovely to see you all. It's really fun to see all the new faces, and we will see you next week for another event focused on AI and higher education.
Good night, everybody. Thank you, Iavor,. Thank you, Siya. Thank you for all your hard work, Caitlin. We'll see you guys next week.