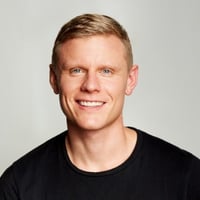
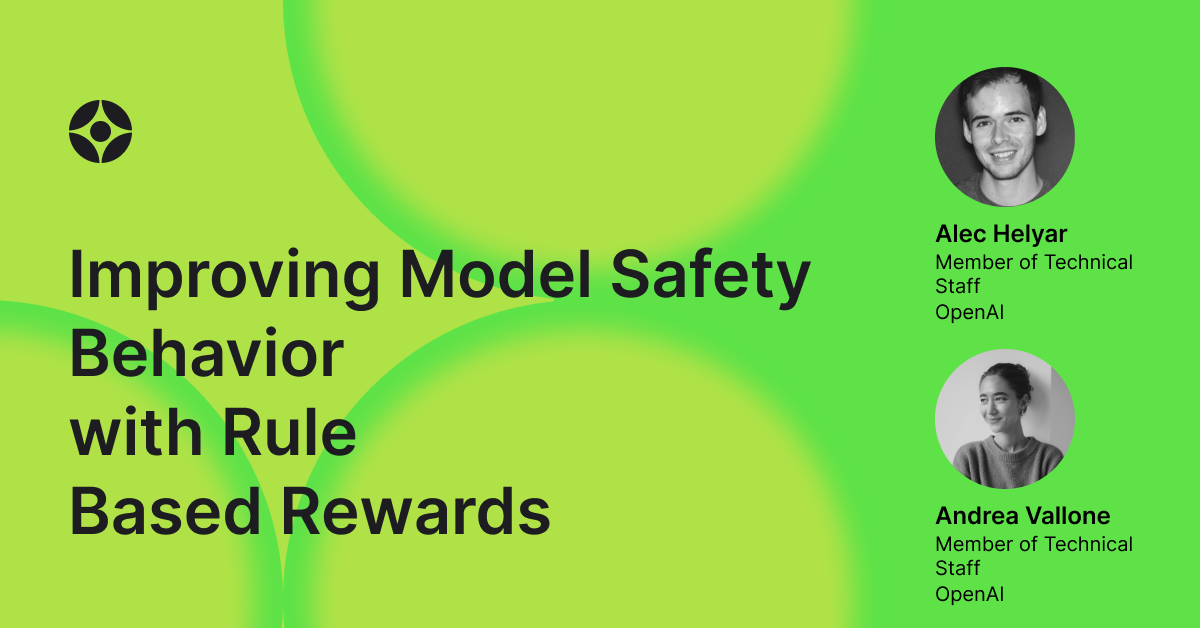
Virtual Event: (New Date Coming Soon) Improving Model Safety Behavior with Rule Based Rewards
Event Update:
We wanted to inform you that the Virtual Event: Improving Model Safety Behavior with Rule-Based Rewards scheduled for Thursday, September 12th at 12:00 PM PT will be rescheduled to a later date in a few weeks. The exact date and time are still to be determined. We are excited about the insights this event will offer, and we look forward to sharing them with you soon.
For now, we will keep the event card available, but registration will be temporarily closed until we finalize the new schedule. We appreciate your understanding and will send out a new invitation as soon as the details are confirmed.
Please note that we will be unregistering all current registrants, which might remove the event from your calendars. This will allow you to re-register once the new date is set, ensuring you have the most up-to-date details.
Thank you for your interest and patience. We look forward to your participation once the new date is set.
About the Talk:
Alec Helyar and Andrea Vallone present their research, Improving Model Safety Behavior with Rule-Based Rewards.
Traditionally, language models have been fine-tuned for safety using reinforcement learning from human feedback (RLHF), where humans define desired behaviors and provide feedback to guide AI systems. However, this approach often involves inefficient data collection, especially when safety policies evolve. To address these challenges, the researchers introduce Rule-Based Rewards (RBRs), a method that leverages explicit rules rather than extensive human feedback to align AI models with safe behavior.
RBRs use clear, step-by-step rules to evaluate whether a model’s outputs meet safety standards, allowing them to be integrated into the RLHF pipeline. This integration maintains a balance between helpfulness and harm prevention, ensuring models behave safely and effectively without the inefficiencies of recurrent human inputs. Since the launch of GPT-4 and GPT-4o mini, OpenAI has employed RBRs as a core component of its safety stack, making AI systems more reliable for everyday use by people and developers.
The researchers demonstrate that RBRs not only enhance model safety but also offer a cost- and time-efficient alternative to traditional methods. RBRs can be easily updated to reflect new safety requirements, and their application extends beyond safety training. They can be adapted to other tasks where explicit rules define desired behaviors, such as customizing the personality or format of model responses for specific applications. Future work includes more extensive studies on RBR components, the use of synthetic data for rule development, and human evaluations to validate the effectiveness of RBRs across diverse domains.
This research marks a significant step toward advancing safe and aligned AI, showing that RBRs can effectively reduce reliance on human feedback while maintaining robust safety standards. The authors invite researchers and practitioners to explore the potential of RBRs in their own work, contributing to the collective effort of creating safer AI systems.
Speakers
Agenda
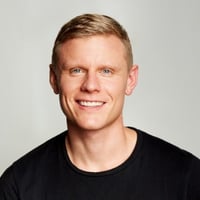
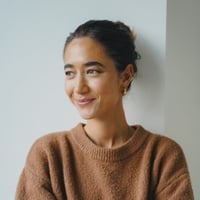
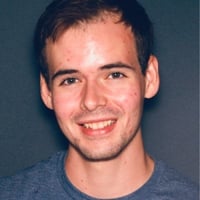
Attendees













