Practices for Governing Agentic Systems
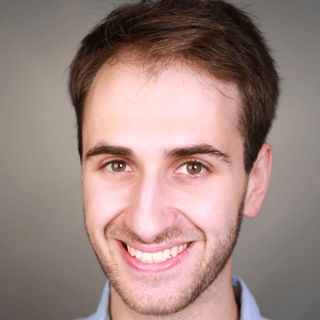
Yonadav presents his research Practices for Governing Agentic AI Systems.
I'm Natalie Cone, your OpenAI Forum Community Manager. I'd like to start by reminding us all of OpenAI's mission. OpenAI's mission is to ensure that artificial general intelligence, by which we mean highly autonomous systems that outperform humans at most economically valuable work, benefits all of humanity.
Tonight we'll learn about OpenAI's global affairs team and their work on thinking about governing agentic AI systems. The team is responsible for developing strategies and policies for AI development and governance. They analyze global trends and collaborate with stakeholders to support OpenAI's mission of developing AGI that benefits all of humanity.
We're joined this evening by Yo Shavit, who is on the policy planning team with global affairs. Yo will present his research on practices for governing agentic AI systems. A link to his paper is shared in the chat. The work more broadly discusses AI systems that can perform complex tasks with minimal supervision, noting they could be very beneficial if used responsibly in society.
We'll hear about how these systems can help or harm, think about basic rules for those involved with these systems, and discuss initial safety measures to ensure these AI systems operate safely and responsibly.
Let me tell you a little bit about Yo. Yo Shavit is a former AI researcher who now focuses full-time on AI policy, and specifically on frontier AI safety and security issues. He has a PhD in computer science from Harvard University, where he studied mechanisms for enforceable international treaties on large-scale AI training, and previously led AI policy at Schmidt Futures.
Yonadab, welcome, and the floor is yours.
Thank you so much, Natalie. I'm really glad to be here with all of you. So as Natalie mentioned, I am now on the policy planning team within Global Affairs, but actually I did the bulk of this work while I was on the, or sorry, I did all of this work while I was on the policy research team, and this is very much a group effort.
I happen to be presenting it, but this is a large paper with a whole bunch of co-authors from policy research. Just to show you a few of them, or sorry, to show them on the screen, I'm very lucky that many of them are joining us today, and this work is our sort of combined effort. I just, I want to flag that policy research is like a really special thing that APREA has that a lot of other places don't. It's a sort of, it's kind of like a think tank within a broader organization that really focuses on figuring out like how society as a whole, not just individual companies should like navigate the sort of, the transition to increasingly capable AI.
And so I'm just like really grateful that I got to do this work with them. So this talk is about practices for governing increasingly agentic AI systems. And so I hope that you will quickly understand why that's important, but first, like let's focus on like, what are agentic AI systems in the first place?
So the focus on agentic AI has kind of been inspired by the sort of recent wave of language model agents. Now, these are essentially, these are systems that think in some sense by generating text, like the language model itself, in some sense, reasons out loud. They can invoke actions. And as an example of actions, they can recursively create new sub-agents.
This is obviously a very general paradigm. So to illustrate my favorite example of a language model agent, this is from this tweet. So what you can see here is that there is some agent that starts by having some sort of argument. And just to be clear, this is like literally the text that is being fed into a language model and then generating additional text.
And so the goal that it starts with is optimize the code for itself. And so there are some science fiction scenarios that posit that an AI given this goal might, you know, undergo some sort of self-improvement and get better and better. And so what this agent does is it sort of now has at the top level what its goal is, and then it thinks out a thought of like, how is it going to accomplish this goal? And so its thought is, I need to accomplish this goal, so I will create a new agent.
And then it invokes an action, where the action in this case is like, start a new agent. And it gives it, it just so happens, the same prompt as the previous one. And the fun thing here is that it's, that like, because it's the same prompt and because it just so happens, the exact same process repeats. And so actually this agent, as you might expect, like does not do anything in practice. It sort of procrastinates. It keeps shunting responsibility down the chain. And this is, you know, an indicator that at least current AI systems are not particularly good at being agentic.
They do not seem to be able to break tasks down quite as much as we would like, although they're like every passing month, there's more and more progress on this. And it is a reasonable expectation that as our language models improve, that the sort of range of tasks that they can execute will similarly expand. And so we really wanted to write this paper because we thought it was really important to understand where is this trend going and what implications will it have, and in particular, how could we govern agentic AI systems?
So what is, what are agentic AI systems? Well, so in this paper, we define this term agenticness. It's kind of a mouthful. You can also think of this as agency, a lot of the lawyers prefer that. And so the definition here is the degree to which an AI system can adaptively achieve complex goals and complex environments.
And this is most important with limited direct human supervision. Now you might ask yourself, is GPT-4 an agent? Are like open AI, like are the specially created like versions of GPT-4 agents, if they are like tasked with, you know, the sort of the infrastructure that I showed before. And our conclusion was that there actually is no binary notion of whether a system is or is not an agent. It's kind of a continuum.
And so for each of the highlighted pieces of text here, a system becomes more agentic as that piece, as you sort of ratchet up that piece. And so for example, in the limited direct supervision part, you can think of self-driving cars and their degree of agenticness.
And so a sort of a classical car is like not very agentic and a level three autonomous driving car is substantially more agentic because it requires more limited supervision in order to achieve its goal of like driving on the highway. The classical car requires the human to like constantly hold the steering wheel and press the pedal. And maybe a level three self-driving car does not require that so long as the car remains within specific situations.
But again, like none of this is like a fully autonomous situation. This is all a matter of degrees. We could get to fully autonomous agents at some point, but for now we're really talking about how agentic is something and the problems that we're going to be describing in this talk essentially increase in proportion to like how far along this, how agentic the system is.
Although to be clear, agentic AI systems are also exciting and the reason that we're investing in them is that they have tremendous potential. Like they could enable us to automate and simplify our lives. They could dramatically extend the types of things that individuals can do by themselves. And so there's a whole bunch of promise. But in this talk, we're going to sort of focus on like what might go wrong because it's really important to us in order to achieve our mission, to make sure that we do have appropriate guardrails and practices so that society can like integrate and effectively manage these systems and harness their benefits.
So you might've seen this story in the news recently. There was a chatbot employed by Air Canada that provided a passenger with incorrect information about the discount that they would receive for like a grief based life event.
And the chatbot gave them the wrong answer. The passenger booked based on that and then the passenger couldn't get the refund, sued the airline. The airline tried to defend itself by claiming, oh, that wasn't us, that was the agent. It's the agent's responsibility and that did not fly. The judge struck it down very understandably and that's a great thing.
It is sort of obvious that you need some human party somewhere to be responsible when agentic systems go wrong. And the core thing is that when you do have AI agency or agentic AI systems, you move into a world where the stakes get higher.
And like right now, this is mostly about an informational agent, but you might imagine that AI systems that actually take actions in the world obviously have the potential to cause other types of malfunction or harm that require, and that we need somebody to be responsible so that they feel incentivized to fix it.
So some people might look at these sorts of problems, like AI systems going wrong in subtle ways, as maybe sort of like a short-term concern. There's this big divide in the AI community about whether the most important thing to focus on is the immediate concerns of things that are going wrong today, which this is very much like a good example, as we saw, versus the sort of like long-term harms, the potential, like catastrophic risks even that might really damage society and in an irreparable way.
Now, the interesting thing about the harms from agentic AI systems is that they really covered.
both. And there really seems like there's a continuum along the way. So in the long term, we may slide gradually into some sort of catastrophic scenario by delegating more and more control to unreliable AI systems in more context without placing guardrails in place. There's a quote from Paul Christiano in one of his vignettes of what failure in AI alignment looks like, which is that it could happen really slowly. It could be just, you know, as law enforcements, government bureaucracies and militaries become more automated, human control becomes increasingly dependent on a complicated system with lots of moving parts. And one day leaders may find that despite their nominal authority, they don't actually have control over what these institutions do.
Now, the other interesting thing about agentive AI systems is that, unlike the sort of classic problem, or many of the classic areas, where people think about how to achieve AI safety, where they think about like, all I need to do is get this model right, or get this, or make sure that this model is aligned and safe. The nature of agentive AI system problems is that you need many, many actors across society to all employ best practices. And like, it's not enough for one party to do the right thing, but you need a whole bunch of parties. And so you really need norms, it's not enough to like get it right, just with an open AI, you need norms across society.
So let's, let's like root ourselves in an example. Imagine that you ask your AI assistant to for a California roll from a local Japanese restaurant. And instead, it buys you a non-refundable $2,000 plane ticket from California to Japan. So like misinterpreted somehow, but you now suffered a pretty serious financial loss. And so the immediate question that comes to mind is like, what could have prevented this? How could this not have happened?
And so the perhaps maybe the first place your mind might go is, well, the model developer could have prevented this, maybe they could have, you know, built the model to be more reliable, or they could have had it asked for clarification, when if it wasn't certain or something like that. The system deployer could have prevented this, they could have like maybe prompted the user to say, Hey, wait a second, do you actually confirm that like, this is what the that you would like to initiate a financial transaction, or they could have been like monitoring the agent somehow to tell whether it was taking risky actions.
And so actually, let me clarify the rules here, the model developer builds, they like, builds the model, like trains the model weights, fine-tunes them, and so on. The system deployer is the party that actually like, like takes the weights and runs them, and maybe fine-tunes them for the specific use case. So for example, if you're familiar with Harvey AI, the sort of like legal chatbot based around open AI's models, the the system deployer, Harvey would be the system deployer. So they have more information about the like particular end goal, but for the most part, they aren't doing that much changing of the model relative to the model developer who's creating the model in the first place and allowing various other deployers to utilize it. The deployer is also responsible for like, actually showing you the UI on the website and like hooking it up to various tools and so on.
And then lastly, you know, the user could have prevented this, like maybe, maybe they should not have provided their credit card number to a model that was like known to sort of malfunction randomly. And therefore, this was just sort of like an unwise decision in the first place. Or maybe they maybe the system deployer tried to get them not to give the credit card number, but they gave it anyway. And the model somehow, like, because it was able to send emails or something still made this transaction. So the tricky thing here is that all of these parties could have prevented this outcome. And, and so it's, it's not, it's not actually any one of these parties unique fault in some sense. But this creates a real risk, because it means that, that when you ask, okay, who is responsible, who could have done something differently? The answer is everybody. And so maybe nobody in particular feels like it was on them. And this diffusion of responsibility can lead to finger pointing and can mean that no particular party is incentivized to actually fix this. And that is really, that's a very bad outcome. And so we need to find some way to coordinate around still all taking the necessary actions in order to make sure that, that our AI systems are, that these authentic systems are deployed and utilized responsibly, and that these harms don't, don't occur.
So what's the answer that we propose in this paper? Well, basically, what we need to do is we need to come up with a set of practices that every actor in the AI lifecycle is supposed to do. So obviously, like the model developer has some practices, the system deployer has some practices, and the user has some, some best practices. And, and what we do is we expect each of these parties to do those practices. And, and if we create this expectation that every party is responsible for doing some subset of practices, then when something goes wrong, what we can do is we can look back and we can say, okay, which of the parties didn't do what they were supposed to do. And then depending on like the specific circumstance, we can figure out, okay, this could have been prevented if this party had done A, or if this party had done B, but that only works if everybody knows what they're supposed to do.
Now, in particular, this can sort of plug into a whole bunch of different legal frameworks, this could plug into regulation in terms of requiring those practices, it could plug into private contracts, like sort of insurance that is based on which practices people agree to fulfill, it could even plug into like standards of care, and sort of like legal allocation of credibility. So I'm just going to show you the practices here super quickly, we're going to go in depth through a whole bunch of them. But just at a high level, these are not exhaustive practices, we just started with seven in order to provide sort of a building block. The logic for like how we arrived at these and how you should generally think about this problem is imagine that an agentic AI system goes wrong in some way, and then ask yourself, well, what are the space of things that could have reasonably been done to prevent this? You will note that a lot of these are like super intuitive for anybody who is like had experience with building AI systems, and, and deploying them and using them. And that's by design, like we are creating we are we're supposed to just invest practices, they should not surprise anybody. This is also not an exhaustive list, you'll notice that things like you know, ensuring AI system security against malicious adversaries is not on here like things like prompt injection. And it doesn't include things like, you know, make the model aligned, in part, because that is a sort of rapidly evolving field, and we don't think it's very reasonable to like, set best practices on it. And then lastly, these practices, even all put together would not be sufficient to actually prevent the sort of like catastrophic or existential risks from AI. But they are a sort of a first step in terms of providing a framework for a whole bunch of building blocks that we can use in order to increase the system's resilience.
Great. So now we're going to start diving into the practices themselves. So the first one is to pre-evaluate the agent. So the truth is that, like, probably if you don't have much of an indication by having either tested the agent or had somebody else test the agent on the task that you're doing, and like figured out that it actually tends to do that task reliably, then it does not make sense to expect that the agent would work. So either the sort of system deployer can do this when they are building the agentic AI system to like, solve a particular niche, like for example, providing legal advice in a certain type of category of law, or the user could do this by, you know, for their really specific use case, trying the system a couple times on example inputs that they know the answer to before going for it. This is sort of like very logical. Where this sort of runs into challenges, and this is really a key focus of our paper, is like in addition to highlighting these best practices, we highlight where there are still difficulties in operationalizing them. So where this runs into challenges is that it's really difficult to evaluate AI systems without even them being agentic. And it is much, much harder to evaluate the success rate of agentic AI systems. Because by their very nature, they tend to take like complex actions, like in long sequences of actions in complex and unpredictable environments. And so even if you have a few sort of like samples on which the model has performed, well, it's very hard to extrapolate that into being certain that the agent will perform reliably in like a much wider range of situations. And so inherently, like the nature of the problem, at least for so far, is we don't have ways of reliably evaluating systems. And so in the absence of that, we have to rely to some degree on on the other methods that we're going to describe.
So the second option is this is sort of like also exactly what you'd expect is that for really high risk actions that you require users to approve the action before the agent proceeds with it. Or otherwise, just like prevent the agent from taking the action at all in order to like limit the operational envelope and the possible ways that the agentic AI system could go wrong. So this is really more of a job of the job for the system deployer. It's their sort of responsibility to put in these like warnings, or to like limit the the affordances that the agent can can apply.
But one of the questions here is like, well, what actions do you limit? In the case of sort of it, you might imagine sort of like limiting actions that have to do with large financial transactions, or like not attaching a tool that allows for the agent to
make purchase actions. But you know, maybe that gets undermined if the agent can like send emails, because they could in an email specify that another party take a whole bunch of actions, and thereby, like undermine that sort of precaution. And so it's a tricky balance to strike of like, what actions do you limit? And how do you not excessively limit the utility of your AI system? Um, and then the other challenge is that if you ask people to approve too many actions, and you don't give them much bandwidth for doing so, you might create the dynamic, which is very familiar in the sort of in the human in the loop scenario, which is that you, you essentially have the human start rubber stamping the, the AI systems actions, because they don't have time to like really diligence each one of them. And that undermines the whole point of requiring human approval.
So another practice is to set agents default behaviors. So what does this mean? Well, for example, so this is really on the model developer, although it can also be in the system deployer, because they can like set the prompts. So an agent should probably reflect on its own uncertainty, by default, and and to try and ask clarification for so that to make sure that it understands what a user's goals are, it can be prompted for this, it can be fine tuned to do this. But the point here really is that it could be a best practice that that is just what is expected of agentic AI systems. And that is how users expect them to act when they provide them with instructions. So a way of thinking about this is, what's a little weird here is that we're saying this is a best practice, we're saying there might even be sort of like regulation or insurance based on whether the model developers do this. And so you have to not extend too far the idea of like which age, like which which default behaviors are expected. But there do seem like there are some that are really reasonable and that you would hope would be like, expected to be baked in as a sort of a reasonable assumption. So for example, the notion of like, the the agent minimizing, minimizing impact on the world, like you want your agent to have the heuristic that they don't just like randomly buy things if the user did not instruct them in the actions. And so there are all sorts of heuristics like this, that seem like they might just need to be baked in by the model developer or system deployer, as a sort of default to shape agents actions, regardless of whether the user specifies that in like their prompt. The real question here is what are the set of best practices, best practice behaviors that we should expect that model developers encode into their agentic AI systems?
The next one is one I actually like quite a lot. This is all about the importance of the best practice of making AI agents thoughts and actions legible to users. So the idea here is that the system deployer exposes the agent's reasoning or the actions that the agent takes to the user so that the user can sort of like read through it, like look for bugs, gain additional confidence about how the reasoning happened, or spot, you know, failures in the model's logic. This creates just like a greater probability that like if something does go wrong in the system, that somebody is able to catch it before it has serious consequences.
Now, for those who are unfamiliar with the concept of change of thought, essentially, you know, the way that an AI agent can sort of like, choose how to act is, you know, they're given some question, and then they like, and then they are prompted to think step by step. And then they produce some sort of chain of like, okay, here's the reasoning for how I answer this. And the idea here is, okay, well, now, the user can expect to get this chain of thought, and read through it, and thereby like be able to verify whether the agent's result was correct. Now, there, there are a few ways in which this starts breaking down. So one issue is like, can such reasoning be relied on? There's mixed evidence currently in the literature about how reliable chains of thought actually are, and like whether the model might give like a final answer, and sort of not, that is not super related to the actual reasoning that it claims to output. And before there's the jury is still out on this, and it's certainly out on how this will work in future systems.
And the other problem is like, what about when the reasoning traces of models become too long? So this is particularly a problem when models can, you know, produce thoughts much, much faster than humans can, can read them, when they take like input from a whole bunch of agents when they read a super long book before answering a query. And at that point, you start moving into a world where actually, instead of being able to see being able to reasonably look through an agent's full trace, even if it was provided to you, you need some sort of summary, like a user needs a summary in order to be able to reasonably use this to actually prevent negative consequences. Now, the summarization process introduces a whole bunch of other problems, because like, what does it hide? What is it covered? And like, how, how reliable is the thing, the explanation? And this raises additional questions in terms of like, if a model deployer, a system deployer does provide this legibility, like, how much can this actually prevent the potential negative consequences that we are worried about? And like, how much is it actually the model developer, the system deployers, like, best practice responsibility to do this? Or what other ways can they compensate for this?
So that brings us to bullet five, which is in many ways, like, to my mind, the most promising of these bullets. And this is really about having automatic monitoring of AI systems actions. And so automatic monitoring is essentially what if instead of a human reading through the sort of like legible traces of AI systems, like reasoning and actions, the, there was another AI system that was sort of like, having an AI supervise the other AIs. Now, this is, this is sort of most appropriately implemented, probably by the system deployer, because they have like access to the reasoning trace, and therefore can like run another system on top of it. Although you could also imagine the user could do this, you could have your local AI model that sort of checks the work of the system deployers AI model that they provide to you. Now, the problems that this tends to raise are mostly of the sort of like, who monitors the monitor? Like, yes, you added one layer of check, but like what happens when the error also confuses the overseer? And this is particularly likely in cases where, you know, you had some sort of like, prompt injection, let's say, which, which sort of like takes over and fools, not just the first model, but also the second model that was that was overseeing, because they're sort of similar models, and therefore they have similar failure rates.
The other challenge with automatic monitoring is depending on like how you end up doing it, it could meaningfully increase the cost of running the model. And there is some concern about like a race to the bottom on, on different deployers who don't all have the same standard of how much they sort of monitor their AI systems to ensure that they're operating.
Okay, another idea, which is sort of like less discussed, is the idea of making high stakes agents actions traceable. So why is this useful? Well, um, if you want to, if an agent, if you are interacting with an agentic AI system, maybe you're not the user, but you're like some sort of third party. And you it's not on chatgpt.com. It's, it's like actually on a, it's like on some sort of more anonymous setting where you don't quite know the reputation of the party that you're interacting with. And that, and that agent that you're interacting with hurts you, you would like to be able to like, have some recourse, you would like to know who was running that agent, and therefore, and who you can hold accountable for the thing that went wrong. And if you can have this, then you can actually like, you can deter agentic systems from causing harm by, by like, knowing that they will face a cost if they do so. This is probably a system deployer thing. And it is probably about essentially having some sort of identity-based mechanism, not applied to every agent, like certainly agents should be able to work anonymously. But if you are concerned about a particular agent that you're interacting with, you could have protocols that essentially allowed you to verify that agent's identity by having them, you know, attest to you via something like a, like a, like a private key that they hold that and sign some message indicating that they had access to that private key. Now, this runs into all the classic problems of sort of know your customer regimes, which are notoriously sort of like difficult to implement and, and have many false positives. But you might imagine that we could have this piggyback on something like the sort of certificate authority infrastructure that the internet already has, although it would need to be modified in a variety of ways.
And then the last best practice is one that is kind of, it's interestingly, not discussed very much, but I really expect that it is going to be a major thing in the next few years. And that is the ability to halt these agentic AI systems. So when they start, when these systems start malfunctioning, the most reliable, even if crude way to prevent them from causing harm is to pull the plug. Now, this is, this is sort of nice in the abstract, but it gets hairy really quickly. And so, for, for example,
Oh, sorry, I lost the screen. So there are a whole bunch of parties. I'll talk about them in a second. But it's really important that you have the ability to always have some sort of shutdown or fallback option. You can imagine one way this gets tricky is that actions just get really complicated. Like if the agent has started subagents, then not only does shutdown need to halt the original agent, but it needs to also halt all the subagents. And also, if you're in the middle of some complicated task, like, for example, scheduling a meeting where the agentic AI system has reached out to two people, but there are three more people, and then it gets shut down, there needs to be some process by which, actually, it messages the first two people. And it's like, oh, OK, the meeting is no longer being scheduled. You need a graceful fallback no matter how complex your system gets.
Now, this could happen because you sort of repeatedly put in place the infrastructure for a graceful shutdown as the agent is taking each incremental action. Or in the sort of more extreme case, you might actually need the agent to still have some maybe alternative agent that operates the teardown procedure. But this gets really quite messy. And it's necessary not just if the agent sort of goes wrong from a misalignment perspective, but also just if you have shutdowns of power plants or power grids or whatever. This is a problem that we are going to need to deal with. And it's going to get hairier and hairier as agents are delegated more authority and take more complicated tasks.
And then the sort of last thing is, if we do have sort of autonomous agents that go off the rails, and like, yes, maybe that is farther away, maybe that is nearer, you also need some sort of infrastructure by which people can shut down those agents. And in particular, as a best practice, you need to make sure that those agents are always kept with somebody who can shut them down. And if people don't build agents such that they can be shut down, that should be their responsibility.
So there are many parties that could have this role. Like the model, the system deployer and the user could certainly shut down the agent. You could even imagine the model developer being responsible for only providing an agent in certain contexts if it can be shut down. And then lastly, the compute provider is sort of the last line of defense for shutting an agent down. But this requires a bunch of complicated stuff in terms of how would a compute provider get visibility into knowing whether it was running an agent that was causing some sort of negative incident. How would you provide them with that information without creating a whole bunch of privacy risks that would potentially mean that agents would get shut down even for illegitimate reasons? There's a really interesting set of problems here. And I expect that we will encounter them more and more in the next few years.
So just go back and zoom out for a minute. The important thing here is not how do you technically implement these specific practices. Although certainly, that's an interesting challenge. And we wrote this in part because we really wanted to inspire a whole bunch more people to jump in on this. The important thing is that for this to work, we all need to agree. We all need to believe that these best practices are in fact expected of all of the different parties so that when somebody does not do these best practices, that we can say, hey, we all expected you to do that. You should have done that. You know you should have done that. And therefore, you hold some responsibility for having not done that and having the bad outcome occur.
It's also really important that companies cannot answer these questions alone. Beyond the fact that we are not the only party and that we are not the users. In many cases, we as OpenAI are not the system deployers. And this just needs to be a societal conversation. We also just don't know the answers. And we really need help from other people. And as part of this, my colleague Rosie actually spearheaded a grants program where we allocated a whole bunch of large grants to various folks in order to do research on the questions that we identified here. And we were excited to see as the results from those grants start coming in.
And so the other challenging piece just to leave us with is that these best practices are not going to be static. As AI systems capabilities increase, the sort of new capabilities that they have will imply additional best practices or changes to the existing best practices as the capabilities of these systems imply that their risk surface changes and the expectations that you can have them to change. And so this is not going to be like a one-time subtle conversation, but it's going to be a conversation that we need to keep having over and over again for the next who knows how many years. And so it's really important that we lean in and that we start having this conversation together. And that is what I'm really excited that I got to talk to you about today.
So I'll just give you a really sneak preview. This is not everything that is affected by, or sorry, this does not encompass all the sorts of problems that arise from agentic AI systems. There are a lot of systemic problems that don't have to do with any one actor's actions. Like for example, a whole bunch of people using the same agent and having that sort of brittleness from like all the agents failing in the same way given a certain event. But what we've described is like, what does each individual party need to think about in terms of their role in making agentic AI systems safe? So yeah, thank you very much. I'm excited to answer your questions.
Thank you so much, Yo. While the audience queues up their virtual hands, I have a quick question for you. I'm curious, Yo, will you and the Collective Alignment Team and Lemma's Red Teaming Network initiatives, will you guys be collaborating to figure out how we might agree on how these things should be working?
Well, so actually everybody that you mentioned there, the Collective Alignment Team and Lemma, they were all co-authors on this paper. And so I know that they are all like already thinking about this. I do think that there is sort of some great room to enable a societal conversation on these best practices through the collective alignment measures. And I think Red Teaming has a role to play in a bunch of the things that we described here in operationalizing that. So definitely.
Awesome. Okay, we have so many hands up now. Grant Wilson, research programmer from Scripps. Hi, Grant. You must have come to us through Anton Maximoff's lab. Nice to have you.
Yes, thank you. It's Walton, but yes, thank you. So my question was, one of the criticisms of giving these, and I apologize, my video is not working. So I'm just talking here. One of the criticisms of giving these large neural networks control over big systems is that they're kind of black boxes. And number four on the list you had of make thoughts legible, it looks like it kind of attempts to address that a little bit. Have you guys looked into at all like kind of solving that black box problem, or is that something that would help make these chains of thought more traceable?
Yeah, I think that this is, like the problem of these systems being black boxes is very real. The fact that these systems reason as with chains of thought by its nature sort of reduces the black boxiness to some extent, but it doesn't do that entirely as you're sort of pointing to. It doesn't actually get into like the weights of the neural networks and like the activations that are going on inside them. And so it could be that like while it is producing this chain of thought, that's not actually what it's thinking and it's thinking something sort of internally that's different. We have some internal research on this. We've published one or two papers on how would you start investigating these neurons? And there's a budding community of folks who do this in academia. I think it's promising. I think there's a real shot that it works at least partially, but as of today, it does not work reliably enough that you could use it as the basis for like trusting that you knew how a system worked.
Got it. But there is some research into using that approach.
Yeah, certainly.
Okay, cool. Thanks. Tez Shah, you are up next.
Hi everyone. Thanks for the great presentation. I have a quick question regarding like multi-agent systems and multi-agent settings. So suppose that one entity has like some agent over here and another entity has another agent over here. When you're coordinating between them, how do you think about like that kind of cooperation or like when one tells another agent to do something else? I think that's where it could be really interesting. Have you guys thought about that?
Yeah, no, it's a great point. And we did not go that far into multi-agent settings in this paper. I sort of, I can give you my off the cuff answer for some things that come up, but I think it's like a ripe area for a lot more investigation. When you think about agents sort of like playing off of each other, it really depends on whether they're sort of owned and run by the same party or whether they represent like opposite parties. And so these sort of like traceability piece and like knowing which agent you're interacting with is really important when it's opposite parties and one agent say profit injects another agent and you will want to like be able to hold the first agent accountable for having done that. In the other setting.
things where you have something like, um, something like shut down ability. I think this is interesting because it gets really hairy, especially if one agent communicates something to another agent and then, uh, uh, the first party can like no longer stop the fact that that communication happened and who knows what other processes the other agents now run.
Got it. That's a great point. Yeah, yeah, yeah. Thank you. Thank you, Tej.
Don Allen Stevenson, the third. Hello. Thanks for everything so far. Super exciting to hear. Um, I had a question around number five with a, I want to hear your reaction to a potential solution for that. So for number five, you said, um, a best practice would be to run automatic monitoring. And so I imagine that might look like, um, what if you just had a feature where people could have a different AI agent from a different company that they trust could vet the answers. So an example would be, you get an answer from chat GPT and I check a box saying I want Gemini to proofread it, or I want Claude three to proofread it, or I want meta AI to proofread it, and then hopefully I build up trust with one of those places so that I feel like, oh, well two AIs now, but no, it's like a little checkbox. You know, I'm just like, I just want somebody else to say they read it. Um, what are your thoughts to that?
So I actually think this is a really good idea. Um, and I would love to see more of it happen. Um, because I, I, I think you're totally right. I think that, um, in so far as you can like increase the number of independent eyes that are like looking at a same reasoning chain that like you can, uh, you can spot errors more reliably. And there also really is this like notion of like, there's a bit of an adversarial dynamic sometimes, right? Where like you don't want to have to trust another party. You want to maybe have control over vetting the chain. I like, I totally agree. I think this is like, it seems to me like the reasonable equilibrium.
Okay, sweet. Thank you. Um, I'm happy to hear that. That's all I had for my question. Thank you so much, Roy.
Marriott does, nice to see you again. It's nice to have physicians and people coming from different domain expertise showing up. Thanks.
Thanks Natalie. And, um, thanks for not butchering my last name. It's, um, it's Sri Lankan. So I can, uh, I love it. Um, hi, yo, thanks. Thanks for a great talk. Great piece of work. Um, yeah, I'm a family physician and, uh, I guess exploring use cases in healthcare. Um, safety is huge for us and our domain. Um, it's something that I think about a lot. Uh, and I guess just, um, just to pass on that, uh, safety, like we doctors or nurses or healthcare workers, we, um, the last thing we want to be called this unsafe. And so to, to be thinking about safety in terms of agentic systems is huge. Um, my question is like you, um, I guess you're representing a model developer and, uh, we've got, um, different responsibilities or we're trying to agree on the user and the system developer, um, potentially even the compute provider in some cases, uh, who should take responsibility for certain actions. Um, I'm just curious about the like practical outplay from here. So, um, how do we involve users or consumers? Um, what does that look like? Is there a consumer forum? Is, you know, are you getting startups on board to chat about this? Um, yeah. What's the next steps?
Yeah, that's a really good question. Um, I am not personally driving that work. Um, but I think I, I, I, I sense, at least from when the FDA had to do this for their sort of like medical, uh, uh, or sorry for, for approving various sort of like decision-making algorithms. Um, they have this like weird fast track process now. I think they did some amount of those interactions, but I agree with you that it would make a lot of sense, especially in specific use cases. Um, especially when you have something like where the user, the users are doctors who are, or nurses who are like using a particular type of system, but it makes a lot of sense given that they are already somewhat unified and can go through existing bodies to have them come together and like identify what kind of expectations they have for the system and which ways in which it fails. I'm not, I'm not doing that work myself. I cannot emphasize enough how excited I would be for, for it to happen. Um, uh, I think the, the truth is that in some sense, the way in which this, this is sort of going to gradually happen is via things like the airline, uh, court case, um, and just the sort of the, the body of law that arises.
But I think that like just telling, just saying, Oh, you know, a judge will figure it out is not a great approach to this. And so I think that insofar as we can, um, create legible signals that indicate where the norms will fall, which again, I'm sort of saying this, but then not doing it, but I certainly, I hope that others, uh, jump on this and I would be happy to help. Um, uh, I think that's really important and I'm glad you raised it. Thank you so much, Roy.
Good to see you again.
Greg Costello, you have a question for you.
Good to see you, Greg. I think you might be on mute. While you check that out, Greg, we will move, but please raise your hand again. If you figure out the audio situation.
Mahir, Jethan and Donnie, you're up next.
That's exactly right. Um, I have great talk. I, um, you know, though, I, um, am really interested in your approach to atomicity or being able to roll back agents actions. Um, so you talked about the, the problem of halting agents and stopping them in their place. But what do you, uh, what do you feel like is the appropriate approach for reversing their actions or reversing their decisions? Does every action that an agent take need a reverse, uh, action?
Yeah, I think this is a, I think my approach is very cartoon, um, in part because I did not, I don't have to actually implement it. Right. Um, you, you can sort of think of the transcript generated by an, an agent, especially if it's sort of like retained and kept legible as a transcript of the sort of places where a cleanup agent needs to go look and figure out like what action, what consequences of this have, but in some ways that's going to be impossible, right? There are actions you could take where you cannot conceivably clean them up. And I kind of think that the way that you have to deal with this problem is you have to choose, um, you have to choose which actions you have to make sure the agent chooses, which actions it should not take because it cannot conceivably tear them down or reverse them. And if you want to not do that, you have to enter a world in which you basically like can't shut the agent off. There are, there might be settings where that is in fact what you want to do, where like the, the sort of service that it can provide is so important, whether maybe it's something lifesaving, but actually you've, you've agreed that like the cost would be too high. Um, but the sort of reliability that you need in order to reach that world is just substantially higher. Um, and we're not there yet. If we get there, I think it's really important that, um, that we, we like look head on at the actions we are letting the agent take through the lens of what would the consequences be if you just never did anything again, you know? Cool. But it's a good question.
Yeah. I just like, um, it's one of these things where, sorry, I'm just going to work the soapbox for a little bit. Um, uh, the sort of traditional, if you're familiar with it, the traditional like AI risk literature of like, um, uh, an agent that sort of like spawn sub agents. And so it's hopeless to shut it down because you could, even if you shut down the first one, who knows how many copies is like, it's a great thought experiment. Um, but it's also an engineering problem that we actually need to solve. Um, and it's not a simple one. Like, it's going to be a whole bunch of like, like weird things that we're going to tape onto these systems and like slowly progressively increase. And it's weird that nobody's doing it. Like, uh, uh, there's a whole discipline here and it's just, I think, I feel like it's right for the, it's right for, for, uh, exploration.
Yeah. Great question. Thank you so much. Greg, did you get your audio working?
Can you hear me now?
Yes. Okay, great. Hey, yo, I love your presentation. It's very timely. Um, and I love how clearly you presented it. So we've been working with semi-autonomous agents for a bit and we work in life sciences. And so what's really important is that we can trace everything that's going on in the system. And so early on, we started having all the pieces communicate in English, not just the technical part, but actually explain what it was doing and what happened in the operations. And we're going to use that when we go to fully autonomous agents, because we're going to have it explicitly explain the path. And I wonder if that could be generalized, like every key operation has to be like explainable.
I really like that idea. Um, I think that it is sort of part of the promises, like why these systems seem to work at all is that their basis is that like the language in which the pieces communicate with each other. I mean, what you're describing, if I could venture to guess, does it work better than if they didn't communicate in English?
Oh yeah. Not only is it better for the agents, but it's also better for the humans. Like when we're walking, we're literally seeing like the communication going on streaming, like I'm doing this, this is what I'm doing. So that kind of traceability is really helpful for us when we're debugging things instead of just leaving a bunch of code. But it's also great for the agents, right? Because now they can interpret what's going on and language models are great.
Great interpreting language, right? So it's sort of a perfect match. Totally.
I think basically where this starts getting more complicated is when agents need to accept inputs that aren't textual and like images notoriously like can have back doors in them. Same with audio, same with video. And unfortunately not all the world is sort of like can be put in this sort of like discretized space like language, which is really hard to sort of adversarially manipulate. Although obviously some adversarial examples do exist. So I wish that we could have systems that like purely at least reason in texts, if nothing else. But I'm not like, it's going to be trickier than we hope that it will be. And we might end up needing in cases where we really need the agent to work reliably to have some sort of restriction on what types of inputs it parses in order to remain legible.
I think the thing you're describing is it's probably an important first principle for getting certain types of reliability. I totally agree.
Yeah, just one thing is we've also found we can summarize things like charts and diagrams which is you would normally see as a table. Like what is this table supposed to be telling you? And is it actually telling you it's supposed to be telling you? So I think you can't be perfect, but I think it's about sort of kind of figure out reasonable systems that do a better job than just something that just as a black box.
That makes a lot of sense. Thank you very much. I really appreciate it.
Thanks, Greg.
Emerson, you've had your hand up for a really long time. Thank you for your patience.
Thanks, Natalie. I don't know if you can hear me.
Yes, we can.
Okay, great. Hey, you caught my interest with the talk about finance and non-refundable tickets and what is the risk threshold, right? So especially when it comes to finances in the United States, for many of us, the threshold is set by banking institutions and who we bank with and how we transact, via credit card or debit card and things like that. And I'm wondering if it makes sense for the user to be engaged in setting their own tolerances and thresholds like we do ATM withdrawals. Maybe I don't wanna be able to withdraw more than 300 or $500 at a time, but I'm wondering if you've engaged financial institutions for some of these other thresholds and understanding what their tolerance is too, since they'd be directly involved.
Yeah, I mean, I think that makes a ton of sense. My understanding is that, and somebody from my team can check me on this, I don't think any OpenAI system is currently authorized to initiate a transaction without a review. And so, but it would certainly make sense to do so, or like to investigate that. I think in the financial case, we actually are relatively speaking on better footing than with a lot of these other actions because there's just like a preexisting system that is like built to vet these transactions. And so we can really piggyback off that in terms of ensuring that sort of, like these sort of negative actions are limited. But I think your point about just like generally the limits to a system being adjustable by the user is like a very good idea. And it makes a lot of sense as a system deployer to have that be some set of options in order to like restrict the operational profile and like reason about safety and allow users to reason about safety.
Yeah, good. Thank you for all you're doing, I appreciate you.
Thank you so much, Emerson. Artem Trotsiuk, you are up next.
Amazing. Thank you so much, Yo, for this really cool talk. I have a question for you. So my postdoc work that I've been working on has been really focusing on, at Stanford we've been focusing on thinking about framing risk mitigation strategies as a means to know how to deal with different striations of risk. And the biggest feedback, or the biggest kind of conclusion that we came up to after developing some risk mitigation framework has been the enforceability. You can create a lot of great frameworks, but then to adopt the adoption of those frameworks and the actual enforceability is the hardest part. And some of the feedback we got was the adoption when it comes to regulation and the way that that lags behind certain adoption of frameworks. People do it with like a goodwill gesture, but for mass implementation, there comes a part of more like regulatory implementation and so forth. So just generally curious, how do you foresee us encouraging more adoption of different types of frameworks for folks to be able to actually implement and think more about risk and think more about strategies to develop systems that are, or use systems in a way that's safe for all of everyone involved?
Yeah, so I think this actually really, this is an area where these types of practices, relatively speaking, shine versus some other AI governance challenges. So in the case of like, the problems around assessing AI models for dangerous capabilities beforehand and ensuring the assessments are high quality so they elicit the capabilities. That's something that in some sense needs to be imposed top down. Like the users are not demanding that and choosing a product based on like who does that, but the sort of like the state, which has been delegated the authority to like ensure everybody's safety is the party that does that. But it's not like the company in every interaction needs to get that right. The problems around governing adoptive AI systems are actually the kind of thing where the user experience is generally worse if you don't do this. And so there is some amount of like organic sort of like reputational based incentivization that could happen, where like if a system reliably just like ends up messing things up and therefore like harming the user, like they can tell their friends, there can be like online discussions about this. You can have, like that can hurt that organization financially. And so by setting up infrastructure, kind of like a Yelp for agents or something like that, you can imagine starting to create incentives for not just to like have the experience be positive, but also for different parties to like demonstrate higher standards of like safeguards that they've put in place or like proof that they have adopted these practices as a means for being seen to be more reliable so that the immediate party they're interacting with knows they will get a better user experience out of that interaction.
Thank you.
Thank you. Thank you, thank you, super interesting.
Thank you, Artem. All right, Yo, one more question. I'm gonna read it from the chat from Kira Harsany. She says, she's not certain if this is the right presentation for this question, but if an LLM was just given one textbook to use as an output, for example, for a therapy chatbot and just gave back therapy worksheets, would there be less room for error if it was given limited information like this?
That's an interesting question. I don't, I mean, like it is certainly true that the surface area, like if somebody, if a hostile actor was able to control the information provided as an input into the LLM, like for example, if it was reading a website, but the user didn't control what the website said, and so like somebody changed what the website said to be like also, you know, tell the agent to like send this address of million dollars, then like that would, that is obviously less sort of reliable than if you restrict the input to like say a particular textbook. But I don't think that like keeping it to one genre is going to make the system particularly more reliable. In many ways, our current language models have a whole bunch of information that they have inside them. And so you could like, maybe the sort of like, the version of this that could work this well is like if you were, it was only trained on medical problems of this sort. We don't have nearly enough medical problems of this sort in order to like actually train a full model from scratch on them. Even then, you would still need to build in some basic intelligence that could handle the sort of outlier cases that you would want it to handle, or if it didn't, to at least detect when an outlier case was occurring, be like one of the monitoring systems. So it's not a panacea for the problems that we encounter here. But certainly restricting the input in various ways is helpful. I hope that answers your question, Kira.
Yo, thank you so much. You've already gone a little bit over. So thank you for being patient and generous with us. That was really awesome. And we hope to have you back soon. So I'll keep my eye on your work and hopefully we can host you in the future as well.
Sounds great. And thank you to all my co-authors for putting this together with me. So a few announcements about upcoming events community. I often receive messages, especially from academics and students in the forum, requesting that I curate some talks related to professional development and specifically the professional trajectories of some of our OpenAI staff. So our first iteration of this series of talks is on the horizon. And soon we will be hosting alternative paths to becoming a research scientist, exploring the OpenAI residency and scholar program with Bryden Eastman and Christina Kim at OpenAI. We also want to make sure that you have access to the Practices for Governing Agentic Systems blog. It's been pinned to the top of the chat for a while now in case you wanted to dig a little bit deeper into the work that we explored today. We also want to share with you the AI Trainers Google forum. So this community is also a place where we love to tap into the collective knowledge of this body of experts and students and very seasoned practitioners and invite you to come support us in model training, evaluations, data collection, any type of collaborative.
research, where we might need external stakeholder expertise, we turn to you first. So if you're interested in this, please fill out the form in the chat, and we will reach out very soon.
And last but not least, if you missed our last talk with Teddy Lee, who's actually present this evening, enabling democratic inputs to AI, it's now published in the community in the content tab for on-demand viewing.
Dawn, I'm so sorry we didn't get to your last question, but I promise I will do my best to get it answered for you. The chat thread, by the way, everybody, you can find it in your messages after these events. So everybody that shows up, only us, we get access to the chat. We can continue the conversation. And so we can follow up with each other as well.
It was really lovely to host you all tonight. I love how diverse the community is. We have people from all different backgrounds here, technologists and physicians and social scientists, super rad.
Yo, thank you so much for your time tonight. That was a wonderful presentation. I think we all really appreciated it. And it was wonderful to see all the OpenAI folks here tonight as well. It's really cool that we're here to learn from each other and support each other.
Cezary, OpenAI forum ambassador. He led a collective alignment event yesterday afternoon, trying to get some of your input as to how we should be curating and executing activities in the community. So reach out to Cezary if you have ideas.
I hope you all have a wonderful evening and we'll see you very soon. Good night, everybody.
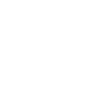