The Importance of Public Input in Designing AI Systems: In Conversation with The Collective Intelligence Project
speakers
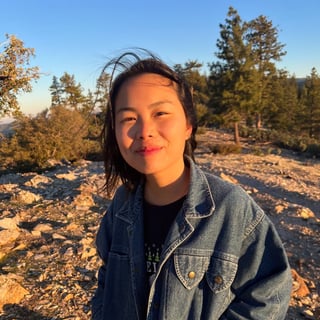
Saffron Huang is the co-director & co-founder of the Collective Intelligence Project and a technologist, researcher and writer. She was previously a research engineer at DeepMind working on topics such as multi-agent RL and language models, and has worked on technology governance research with organizations including the Center for the Governance of AI, the Ethereum Foundation, Reboot, the Harvard Berkman Klein Center and the British Foreign Office. She co-founded Kernel Magazine.
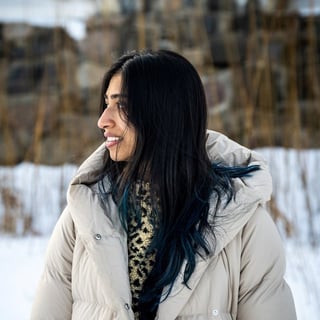
Divya Siddarth is the Co-Founder and Co-Director of the Collective Intelligence Project. She is a political economist and social technologist at Microsoft’s Office of the CTO, a research director at Metagov and the RadicalXChange Foundation, a research associate at the Ethics in AI Institute at Oxford, and a visiting fellow at the Ostrom Workshop. Her work has been featured in Stanford HAI, Oxford, Mozilla, the Harvard Safra Center, WIRED, Noema Magazine, the World Economic Forum, and Frontiers in Blockchain.
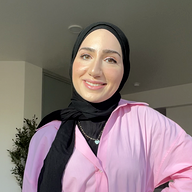
Lama Ahmad is on the Policy Research team at OpenAI, where she leads efforts on external assessments of the impacts of AI systems on society. Her work includes leading the Researcher Access Program, OpenAI's red teaming efforts, third party assessment and auditing, as well as public input projects.
SUMMARY
About the Talk: AI will have significant, far-reaching economic and societal impacts. Technology shapes the lives of individuals, how we interact with one another, and how society as a whole evolves. We believe that decisions about how AI systems behave should be shaped by diverse perspectives reflecting the public interest. Join Lama Ahmad (Policy Researcher at OpenAI) and Saffron Huang and Divya Siddarth (Co-Directors of the Collective Intelligence Project) in conversation to reflect on why public input matters for designing AI systems, and how these methods might be operationalized in practice.
The Collective Intelligence Project White Paper: The Collective Intelligence Project (CIP) is an incubator for new governance models for transformative technology. CIP will focus on the research and development of collective intelligence capabilities: decision-making technologies, processes, and institutions that expand a group’s capacity to construct and cooperate towards shared goals. We will apply these capabilities to transformative technology: technological advances with a high likelihood of significantly altering our society.
Read More About the OpenAI Grant, Democratic Inputs to AI
TRANSCRIPT
The importance of public input in designing AI systems is the perfect introduction to the tenor of content that we plan to produce in the forum.
Today we are joined by three incredible speakers. Lema Ahmad is a member of the policy research team at OpenAI, where she leads efforts on external assessments of the impacts of AI systems on society. Her work includes leading the research access program, OpenAI's red teaming efforts, third party assessment and auditing, as well as the public input projects.
Welcome. Thank you for being here, Lema.
Saffron Wang is the co-director and co-founder of the Collective Intelligence Project, and a technologist, researcher, and writer. She was previously a research engineer at DeepMind, working on topics such as multi-agent RL and language models, and has worked on technology governance research with organizations including the Center for Governance of AI, the Ethereum Foundation, Reboot, the Harvard Berkman Klein Center, and the British Foreign Office. She also co-founded Kernel Magazine.
Welcome Saffron. So nice to have you.
Last but not least, Divya Siddharth is also the co-founder and co-director of the Collective Intelligence Project. She is a political economist and social technologist at Microsoft's Office of the CTO. She's a research director at MetaGov and the Radical Change Foundation, a research associate at the Ethics in AI Institute at Oxford, and a visiting fellow at the Ostrom Workshop. Her work has been featured in Stanford HAI, Oxford, Mozilla, the Harvard Saffron Center, Wired, the Nima Magazine, the World Economic Forum, and Frontiers in Blockchain.
Now I will pass the mic to our three researchers to discuss the importance of public input in designing AI systems.
Thank you so much for the kind introduction, Natalie. And I'm so excited to share the wonderful work that I've had the opportunity to collaborate on with Divya and Saffron over the past few months, and to get to really reflect on some of the key questions underpinning this work that we're undertaking together.
In October of 2022, the White House released the blueprint for an AI Bill of Rights. One of the action items called for in the document is the right for the public to give input on how AI systems are developed and deployed. There's a great interest in understanding how to do this, and the path ahead of us is a long and tricky one, but I'm excited about the work we have in front of us.
My own motivation for this work comes from a general theme of the things that I get to do at OpenAI, which is bring in external perspectives to help assess the impacts of and shape the trajectory of the development of our systems, whether that's through research or access to our models, or conducting red teaming and risk assessments that feed back into our policies and safety mitigations. I care especially about bringing a wide array of perspectives into these processes and decisions, and there's so much more that we can and should be doing on that front.
That's why I'm particularly excited about the Democratic Inputs into AI grant process that is put on in collaboration with several teams at OpenAI, and the work of organizations like the Collective Intelligence Project.
So I'd first like to start with Divya, and then go over to Saffron. I want to hear a little bit more about your background and how you started working on public input for AI systems.
Yeah, absolutely, and it's really wonderful to be here. I know we're one of the first panels on the forum, which is a huge honor. Really excited to share the space with all of you.
I think for me, I've been working on kind of questions of technology and democracy in different ways for a long time, and I started out working on cybersecurity and activism and thinking about the organization of political institutions, whether technology makes those kind of more flat, whether we can build new kinds of political institutions with technology. Did that work in India for a few years, worked on data cooperatives and collective data governance in different forms, switched, actually did COVID policy and kind of public input as related to COVID policy a bit, as well as other kinds of things around contact tracing. And all of these working on different kind of questions of transformative technology, of transformations in society generally led me to thinking about collective intelligence as a good frame for approaching those problems, because, you know, all of them come down to different ways that we prioritize and make decisions collectively over incredibly important, often fast-moving things that we have to deal with collectively that aren't individual problems that can't be broken down into individual problems.
So I think that frame, I've taken into different kinds of work in AI. It's obviously, you know, one of the most important transformative technologies of our time and have been working on AI governance since I was at the office of the CTO at Microsoft, which I left when we started at AIP, kind of taking that frame and saying, well, now we have a major collective kind of question to deal with. There are incredible risks, there are incredible opportunities. How do we take that sense of collective power and public and expert input and balancing collective intelligence institutions to this new space?
Thanks so much, Divya, I really appreciate the wealth of experience that you bring to this problem space, and I'm excited to be working with you on this problem.
Saffron, what about you?
Yeah, so I have been working on AI safety and ethics for a while. I worked at DeepMind before this, as mentioned in the intro, but kind of thought a lot about the various intersections of economic society and AI, whether it's from the multi-agent perspective or, you know, we did we did work on actually, you know, how do you align AI to particular human values and how do you choose those values? And I published a paper recently in PNAS on using the Rawlsian idea of the veil of ignorance to have people choose principles for AI from behind a veil. So thinking really hard about, you know, if we are going to, quote unquote, align AI to the values of society or humanity, who is it going to be? How are you going to do it? What does that actually mean in practice? And how can we make sure that the incentives of the organizations that are developing AI are actually pointing towards genuinely trying to do that?
And so I think in kind of working on sort of how do we make AI go better, both from a technical and from a governance perspective? I think starting CFU was really about thinking, OK, you know, this collective decision making thing is incredibly important for a lot of facets of AI. One part of it is the alignment, which I talked about, like how do we actually sort of funnel this collective input into the technology itself, but also, you know, the incentive structures around it. I know OpenAI has tried to innovate on a bunch of this in terms of, you know, the Windfall Clause, various aspects of the charter, but I think we need a lot more types and forms of innovation on that front in order to really guide this, like, very fast moving transformative technology.
And I think one way of thinking about it is, you know, technology is really accelerating much faster than our democratic structures can handle. And it's affecting people really quickly and really deeply. Child GPT is the fastest growing consumer product in history. We need to get better at making decisions in the collective interest, you know, better institutional designs, more effective democratic processes so that we can actually point our AI capabilities in the directions that we want. And so I think it was it's kind of been leading up to this, but really near the beginning of this year and also in collaboration with the Center for the Governance and Development of AI, started really sort of actively working on pilots around public input into AI systems. And we can talk a bit more about that later. But yeah.
Yeah, thanks so much, Saffron, for sharing some of how you came to this work and leading into a little bit of the next question that I had, which is, why did you both think it was, of course, this this issue is something that you both have been thinking about in various ways and forms throughout the pandemic.
And I'm curious, why did you think it was important to start a collective intelligence project specifically? What's your vision for the organization? And also taking a step back even further, is how do you even define this concept of collective intelligence? I think we talk about public input, collective intelligence and throw these words around. But maybe people have different definitions of what this means.
So tying those two things together of what you think collective intelligence is, what collective intelligence is and how it'll manifest in your vision for the organization.
Yeah, I can.
Start because I think Seth touched on a bit of this already, but collective intelligence is a really capacious term. This is a great thing about it and a difficult thing about it. And so, you know, the field of collective intelligence has covered everything from team collaboration methods, right, to understanding bacterial colonies and how they proliferate to swarm intelligence, to, you know, influencing parts of machine learning.
And so the reason we took the frame of collective intelligence, or at least from my perspective, is it describes kind of a sense of decision making technologies, processes and institutions that expand capacity to address shared concerns. That is broader than technology or sorry, democracy and public input, because we can include markets, for example, as a highly effective information sharing collective intelligence mechanism. You can think of different kinds of institutions like corporations or collective intelligence mechanism. Right.
Nation states take different approaches to collective intelligence. And so when we think about how to direct transformative technology, public input is a crucial part of that. But how you structure and imagine that public input kind of feeds into the broader frame of collective intelligence. And we also think on a theoretical level, there's a lot you can learn from these different forms that CI research has taken over the years to understand what that could reasonably look like, especially with a fast moving technology, because our starting premise is like our existing collective intelligence systems aren't up to the task. Right.
And I think part of that is understanding, you know, when you look at transformative tech, if you think about our typical collective intelligence mechanisms, say democracy, democracy, as it's currently practiced, doesn't really touch transformative technology that well. People don't really have visibility into it. There aren't clear ways to make your voice heard. Markets don't touch transformative technology as much either, though, because of the opacity and the fast moving nature and the way that they get funded. It's not the case that, you know, price setting is a big reason why certain choices get picked over other choices. And so our existing collective intelligence methods aren't very good at making collecting collective input and understanding people's collective preferences at that level.
And we wanted to take a bunch of what has been learned and apply it to that space. And, you know, before this, I worked a bit on standard setting, for example, like understanding how Internet standard setting bodies came about and thinking about these different ways for open source governance or Wikipedia governance. Like, how do we take this idea of collective input and structure it in different ways based on the problem we want to solve? And I think CIP, the Collective Intelligence Project, although I love that you refer to it as a collective intelligence project, which is really what it is, but the Collective Intelligence Project, which is our organization, you know, we wanted to specifically focus on this question. There's a lot of work being done on democracy. There's a lot of work being done on tech governance. But in terms of applying collective intelligence kind of framing and methods to transformative technology in particular, we just thought that there wasn't enough of an overlap between people who are working on CI and people who are working on kind of transformative technology governance. So we're really excited about building those kinds of bridges.
Yeah, and to add a little bit to that, I think the way that I think about CI, collective intelligence, and how it's defined is really kind of influenced by working in AI because, you know, I've been in a lot of debates with researchers like, what is it? What does intelligence mean? And I think a lot of people converge more on the ability to achieve goals and tasks rather than, say, like having a lot of information or knowledge. So thinking more about like being able to achieve outcomes or intelligent outcomes and not necessarily just having information, although the right information is a key part of achieving the outcomes that you want.
And so when I think about collective intelligence, you know, in this world, I think about like, you know, there is people who are working on crowd science or kind of crowdsourcing, like that kind of thing of it's like pool information. But I think another important component when it comes to governance is how do we it's not just about information, it's about what you do with it and the outcomes and like achieving intelligent behavior and being able to achieve the goals that we want to. So, you know, coordination problems are a failure of collective intelligence, things like climate change. So those kind of classic sort of social dilemmas.
And I think, you know, part of failures to coordinate properly, you know, can probably be information problems. So with technologies that are moving really quickly, Vivian and I talk all the time about how like we don't actually know what's going on with AI. You know, we don't really have good evaluations. I mean, nobody's really monitoring anything. We've been working on a proposal around an IPCC for AI where, you know, we we literally need like the metrics and the like studies and that kind of infrastructure in place. And that's like an information problem. But that's like a means to the end of being able to once you know what's going on, do something about it.
You both touched a little bit on the limitations of current CI systems. I'm curious if you want to address some more limits of the CI systems, but then also think about how we start to address some of these limitations. I know that the grant process is starting to do some of that in your own work as well. So I'm curious how we start to think about tackling some of the limitations such as the technology moving at a breakneck pace and many forms of gathering input moving much more slowly and how we start to reconcile some of those tradeoffs.
I think a big part of it is making sure that when you're I mean, this is getting a bit more tactical than the theory we've been talking about, but like when you run a collective intelligence process, do you have a sense of the decision you want to affect? Do you have a sense of the kinds of people that would be best to involve to affect that decision? Do you have a sense of how you're going to ask those people a question that will end up affecting the decision? Do you have a sense of how you're going to reach them and the tools you're going to use to do that?
And I think a lot of what's necessary and part of the reason we set CIP up is doing a bunch of pilots in this space. There aren't preconceived notions of the exact right institutions to set up for AI governance or for governance of other kinds of transformative tech. There weren't when we got hit with a pandemic. There was a lot of great work done, for example, on the beginnings of how to coordinate globally over vaccines. But a lot of that work had to be done ad hoc because it hadn't been experimented with sufficiently before. And I think that's even more true here.
And so we want to have concrete pilots, which I think the grant program is excellent for soliciting and trying to enable. We've done a bunch of pilots on what we're calling alignment assemblies that look at risk assessments, like let's affect evaluations. Let's think about how to do that in a collective way because it's an information asymmetry problem in a lot of senses. We're putting out a technology into the world. You need something like pollution sensors or air quality sensors or whatever. And in this case, that comes from people being able to tell us the risks that they're most concerned about, the impact of having all of the different kinds of data we're used to aggregating on problems that we care about. We need to aggregate here. This is like a collective intelligence issue and let's deal with it in that way.
But there are also questions of principles, like what principles should AI be built on, like Saffron mentioned. And I think that's also a collective intelligence problem in a slightly different sense. And you need a different kind of stack to deal with that problem than you do with something like risk assessments than you might with something like what is the best investment here? How do we involve people in investment decisions? And so trying to develop different stacks for each of those and piloting them is, I think, one of the ways to plug that gap because we don't already know. There are so many new things to try. And this is a space where we're also excited about the reasons that this technology can help. Now, things like language models allow us to have ways to aggregate qualitative information that we couldn't before, ways to understand large amounts of qualitative information that we couldn't before. People can express preferences in natural language and have that be more understood. All of these kinds of capabilities can allow for collective intelligence in different ways than we've already seen.
One classic issue in kind of the space of collective intelligence generally is the tradeoff between scale and nuance, essentially. It's really difficult to have people meaningfully participate in complicated decisions in a nation state, in a neighborhood. It's difficult, right? It's really hard to express your opinions in a nuanced way and then aggregate them and end up with something that works at scale. And maybe language models can support that. Maybe they can do it with facilitation. Maybe they can do it different modes of aggregation, representation. AI in general can allow for different kinds of algorithms or like batching processes to support this. And part of CIP's work is around this question of innovating on AI for institutions, as well as innovating on institutions for AI, that kind of feedback loop of AI for CI, for AI, et cetera. And I think that's part of the way we can plug some of these gaps. Although a lot of it also
I'm curious how potentially we've had some conversations around what makes collective intelligence for AI different, and I'm curious how you think about that.
So we both talked about using AI for the collective intelligence problem and thinking about how to use AI itself to benefit seeking more input, but I'm also curious when we're seeking input about AI systems, how does that look different from other forms of input that people have tried to seek before?
Yeah. I mean, I think when it comes, we've talked about how fast moving things are, and I think when it comes to trying to seek input at a pace that is commensurate with the pace at which decisions are being made about it, it's kind of difficult to know where people are at and to kind of gauge what the right, like who the right people should be, like what context do they need, kind of trying to anticipate what that input is going to look like, or like what the, what direction the conversation is going in and how you can incorporate that into your decision.
So, you know, with LLMs, it's like, okay, maybe lots of people have used chat GPT, but are they the same people who have thought about chat GPT's impact? Not necessarily. And, you know, should we actually be polling, should we be going and talking to a representative sample of, say, if we're limiting to Americans for kind of just because of constraints, you know, should you be talking to a representative sample of Americans, or should you be talking to people who might be disproportionately affected, but it's kind of difficult to figure out what that's going to be, because it's a new technology. If you just talk to the users, you might just find the most enthusiastic people in the room.
So I think there's, and I think there's some real sense in which like people don't necessarily, like people are busy, like, I personally don't want to have input onto on every kind of tool I use. But I do want to have the opportunity to have a say in the things that I do care about and the things that do affect me. And so for something like, you know, chat GPT, it's hard to find the heuristics for that, because it's like unevenly distributed throughout the population.
It's hard to be like, oh, yeah, let's let's take sort of existing kind of profiles of people and trust that that approximately aligns with like people who are most likely to be impacted, if that makes sense. And also, I mean, this is maybe obvious, but folks who are most likely to be impacted are not necessarily the same people who are using the thing. So basically, that discoverability problem is challenging. And we have so far done some mixture of like self-selected input and representative input where we try to get demographic representativeness in the U.S. along age, gender, income and ethnicity lines. And that requires more education.
And, you know, you're going to have more people who have less context or maybe are less interested in the topic to begin with. So anyway, there's a lot of tradeoffs. But I think it's really interesting because it's really different from asking about, say, the economy, which has been the political and social talking point for, you know, forever. Like this is this is a new thing. And it's not clear that like actually every single person should have the same weigh in or can be assumed to have the same context.
I think that's a great point to kind of like why is to the to the title of the session, like why is public input important in designing AI systems? We don't think that like when Google Sheets gets an upgrade, we should ask the people what they want through like a deliberative democracy process. Right. And yet, like in this context, we've been discussing it very seriously.
And what what is it about AI or like what are we concerned about that makes this like that makes this even a conversation worth having? And I think the way we structure public input is based on our answer to that question. Like maybe we think it's going to be really transformative in certain kinds of ways. Then we should ask people about what this transformation should look like. Maybe we're worried about a bunch of risks that don't come up with other technologies. Well, then we should ask people about those kinds of risks.
Like maybe we think that there's a ton of information latent in the population that's necessary for the design of these technologies. And we've talked a bunch about like our HF versus like our LCIS, right, like reinforcement learning from collective intelligence, collectively intelligent feedback. They are just collective feedback.
Like maybe there's something in the process that actually requires input in a way that the design of other technologies don't. Or maybe we should do this for Google Sheets. Like that's a valid opinion. It's not mine. And so I think understanding why we think it's important influences the design of the process really significantly. And, you know, to the point about like people being busy, I've I've said this stuff on a bunch, but I started my work on like democratic input into technology, doing data co-ops.
And when I started working on my collective data governance, I was really excited about directly democratic control over data. I was like, this is a really powerful resource. You know, my background is in some sense in political economy, was thinking about like, OK, if you have collective governance over this crucial input into a bunch of different systems, then like that collective governance propagates. We should build new institutions for data governance, which I still think.
But realizing quite quickly how little directly democratic control kind of makes sense for something like data. If you went up to someone and said, what do you want to happen with your data? If you went up to me, a person who worked on this and said, what exactly do you want to happen with your data? I wouldn't know. I wouldn't want to think about it a huge amount every day.
I don't want to constantly be thinking about my privacy concerns versus like monetization concerns versus, you know, it's just an exhausting and difficult problem. And so this is why we have more complex procedures than directly asking people. This is why we have representation. This is why we have collective bargaining and why we can have different forms of new collective intelligence structures that understand that direct democracy isn't exactly what's always needed, but also that the current system for something like data, say, or for something like AI is very, power is very concentrated.
You know, understandings of what's happening with the technology are very opaque. It's also not great. If you go to people and you say, would you rather directly democratically control your data or just keep the status quo, which I have done in my field research, they're quite conflicted because they're like, I don't like either of those options, but I certainly don't like what's happening now.
And so how do we give, you know, have that sense of collective input and empowerment and ability to provide feedback without defaulting to like a huge amount of onerous decision making over technologies that don't require that or a lot of other gates that aren't always necessary.
I think that's a big part of like designing something collectively intelligent in addition to caring about public.
Yeah, yeah, challenge is, oh, go ahead, Saffron.
Oh, yeah, I just wanted to jump in on that quickly, so I think this is why things like collective intelligence mechanisms that involve like delegation such as liquid democracy are really interesting to me, because it's true that like representatives and delegates have their place.
Another thing is just on the, you know, how is AI different? Like, I think that LLMs in particular and, you know, generative AI, they directly they're like an automation of human expression and it like directly affects language and opinion and what's in people's brains. It's a tool that can talk in a way that previous technologies haven't been able to talk.
And so I think it makes it much more explicitly values laden than other kinds of technology. Just thinking about the Google Sheets distinction, you know, it's much more explicitly values laden, like it will give you an opinion on abortion if you ask it to. And like what, you know, open AI does with that kind of moral responsibility is important.
And so I think it's it is just very exciting to be part of that kind of time.
and part of these kinds of processes. Yeah, I think the coolest thing is like, what can be done? It's not, there's so much possibility. And for me, I also, you know, true democracy in some sense has never been tried, right? Like we've had these ideas about people having self-determination or ways to express themselves, but it's only improved over time. We certainly have not like achieved those ideals in any meaningful way.
And a lot of the reasons for that are like concentration of power issues and both, like a lot of things that LLMs aren't going to fix, but there are also questions around like, how do you express yourself? How do you express yourself collectively? Like these kinds of things are really exciting to just think about and work on. I don't know if we're allowed to do this, but Lama, I'd love to like ask you this question too, because you work a ton on public input into AI. Like, why do you think that that's important or like the things that are missing?
Yeah, I mean, I think the explicitness, it's interesting Safran, what you're saying about like the explicitness or like very in your face nature of the values being expressed by technologies like ChatGPT, because we, at some point we're talking about like, what would have happened if, you know, pre 2006, when certain social media platforms were still coming to bear, what would happen if we had designed like a massive collective input project and tried to understand like how people's preferences were based on social media news feeds? What would have changed? What would people have answered? And I think there was almost like a hidden, value laden question behind there, behind the algorithm in a way that's like much more explicitly defined in outputs of generative AI systems. And so I think that's just like an interesting thing to think about in terms of what questions actually matter to people, and how do we also inform people about why they matter? And I think that, you know, when we're thinking about public input at OpenAI, we're not just thinking about actually like, how does the model express or not express certain values or ideas? It's also about everything adjacent to that. So how do these systems affect people's day-to-day lives? When we're thinking about the use of language models in medical contexts or legal contexts, what kinds of impacts might occur? And then how do we actually walk people through that process of how these technologies will be embedded into their lives in ways that you can see and maybe not see, and start to gather input on those questions as well. And one of the things that I'd love for you to be able to touch on in the last couple minutes too is the work of the alignment assemblies. I'm curious how you came to the question that we're first piloting and exploring together, which is around evaluations of AI systems, which may not be the first like obvious question to ask the public. So walk me through how you're thinking about that and what you're excited about with the alignment assemblies.
Okay, I can start. So we actually did a long process of trying to figure out for alignment assemblies what the right question was to start on. And I guess the concept of alignment assemblies was how do we put into practice collective intelligence over AI? Very simple, right? How do we take these things that we've been talking about and really try them out? What does that look like? Kind of answering those four questions that I mentioned in terms of like, what is the decision we want to affect? Who are the people we think are appropriate to affect that decision? Thinking about representativeness versus like who's most affected versus who's most knowledgeable, right? How do we ask them the question? How do we exactly frame the question and the technologies we want to use to do that? And I think we kind of looked at the development process, everything from like the data that goes into the model to the RLHF process, to evaluations, terms of service, right? All of these different things that go into that end to end, various different kinds of policies, content moderation, thought about like what is the most appropriate place to start? And I think the reason we landed on evaluations is actually something Safran mentioned early on, which is evaluating is kind of upstream of many other decisions that are important. You have to understand the technology before you can make a bunch of those other calls in a way that is legitimate and is good basically. And so it seemed like evaluations are really core thing that we want people to weigh in on and we want people to understand what's going on because they can't make their own individual decisions even over questions like values and principles, et cetera, if they don't understand the capabilities of what they're looking at, right? And we collectively don't quite understand the capabilities of what we're looking at and that's only going to be more true over time. So it seems really crucial that this question of evaluations, which I think a bunch of folks have also done really great work on like societal impact evaluations, understanding why it is very difficult to design good evaluations of models, which it is. It's not easy. You can't just say like, I think we should evaluate for fairness. Like, great. Everyone, I think most folks on this call are intimately familiar with this problem. And so how do we get people involved in that process? And it's quite a complicated design question of public and expert input because you can't, again, go to folks and say like, what kinds of evaluations would you design for models? So we were thinking a lot about like, how do you phrase a question around risks? What are the kinds of things that we could eventually evaluate on building a mapping framework between the answers that we were likely to get and the kinds of evaluations that could be built out of them? And yeah, I don't want to take up too much time so I can hand it over to Saf because I know we have questions soon.
Yeah, I don't think I have that much more to add to this. Like I think, yeah, I think evaluations are, important to because, I think the question of combining expert and public input is really interesting. And I keep going on about it, but I think like experts have a lot of context and they know what's plausible to evaluate on and they have all this experience and that the public are like worried about specific things. And I think that should be accounted for. I don't think anybody can say exactly how much, but like getting an expert committee to basically make sure that like all the risk areas and the things we could evaluate on look complete and plausible and sort of doable within the next X years. And by the way, these are not just technical evaluations. They're also kind of like, oh, maybe we're gonna have to send a psychologist out to do an RCT or some kind of study on how like LLMs impact mental health or something or like cognitive abilities or something, like it's not just evaluation evals in the kind of like ML sense, but evaluations as a general idea. And so thinking, okay, actually there's so many dimensions here and how do we prioritize among them? We don't have evaluations for like any of these things basically. So if we can say, hey, here's like a list that has both expert and public input that's prioritized, how do you kind of, sorry for the interruption, but I'm in a hotel. But yeah, if we kind of have this prioritized list, how do we, okay, how do we like direct resources basically towards the things that are most important, like sort of in order? What I really liked about the evaluations question, I think both of you know that like in the beginning, I was like, is this actually the right question to ask? But I think that over time, what I really liked about the question is that it's also something that's actionable and important for AI companies, how we understand the capabilities and the limitations of the models comes from evaluations. So finding an accessible way to bridge what we do to actually understand the models at an AI company and bridging that with public understanding is a really, I think, critical piece in bringing the public along into decision-making. So I'm really excited about the alignment assemblies and this initial question and really looking forward to when you share the results. I know OpenAI and others will be committed audiences to the alignment assemblies and think about how to actually start actioning on the results, which is something that is really, really critical.
I wanna make sure we open it up to questions from the audience. And while folks are raising their hands, preparing to ask a question, I'll ask one last question to you both, which is, what are you most excited about right now or where do you think people aren't paying attention? And we can start queuing up the questions from the audience, hopefully.
Honestly, I think I'm most excited in a scoped sense for the outcomes of our initial pilot processes. It's been actually incredibly heartwarming and exciting to see the initial responses rolling in. Because when we started putting these out, we didn't know what we were going to get. Like it's a really new technology. People don't always have time for public input. I've done different kinds of public input processes. They're not always super engaging and it's often for very good reasons. Also, we could have designed it badly, right? And just seeing the initial responses, so many.
Folks around the US, we've done some processes in Taiwan, saying, I really want to be a part of this. I'm thinking a lot about this topic. Here are the pros I see. Here are the cons I see. Folks being like, I have grandchildren, I've talked to them about this, and I'm worried about it from their perspective, or this is going to be really great for my work. Just nuanced, engaged, thoughtful responses that have been really wonderful. It is still a question of figuring out how to aggregate that collective intelligence piece, what to do about it. How to make sure actual outcomes change, which is what we care about a lot, but just seeing that and being able to join that conversation has been super exciting. I think in terms of what people aren't paying attention to, I mean, there's a lot of great work being done in a lot of these different spaces, so I wouldn't claim that it's only us thinking about any of these topics, but one thing is, I think this question of what are the kinds of trade-offs that we currently face with collective decision-making that AI can address is something just we're really excited about in the long-term.
Awesome. Thank you so much, ladies. Well, we do have our first question from the audience, Teddy Lee, a product manager here at OpenAI. Teddy, go ahead and unmute yourself. Hi. Can you hear me? Yes. Hey, Divya. It's Aaron. Thanks for joining us and sharing your insights. One of my first questions is a democratic deliberation system, I think, is only as good as people's trust in it, and we're even seeing that in the democratic system in the U.S., for example. If people are not trusting the results, then it doesn't really matter how well thought out it was. How do you think about ensuring legitimacy of a collective intelligence decision-making system, and how should we think about building that legitimacy versus just building a really cool software solution that may not have a legitimacy? Okay, I can take a stab at this. I think legitimacy is kind of fuzzy, but I think one approach to this is transparency. There are some points at which we are designing alignment symbols where I'm like, oh, this stage might be kind of... People might not trust us on this. For example, how we actually translate public input to policy recommendations, that's an interpretation that we make that people might just be like, whoa, I didn't like that. But I think trying to create a framework up front and being really clear about how we did this, and then publishing everything as much as possible, just like we're planning on publishing basically everything except personally sensitive data, and that's part of it. I think also part of it is this idea that I learned from Divya actually of subsidiarity, of having the decision-making, having the decisions be made as local to the locale, like the people that it's affected as much as possible. If you can have communities running their own alignment assemblies and fine-tuning models themselves, or with minimal support, that naturally does not require placing trust in a faraway, foreign institution that they are not familiar with, and so I think that just makes things easier. But yeah, I don't know. I think trust is just a slow, long thing to build, and there's not really that many hacks around it. I was going to say the challenge for OpenAI right now, as we think through the Democratic Inputs grants and participating in the alignment assemblies, is we do want to be intentional about the ways that we build input into our systems, because certainly, especially at the training level, that could have unintended consequences for many, many people who are exposed to these technologies every day. And so as we experiment with what are the right methods to do this, how do you weigh different types of input, expert versus public, or different geographies? How do we actually action on them? And so slowly actioning and intentionally actioning may also trade off a little bit with this legitimacy question in some sense, because legitimacy a little bit is kind of tied to taking an action on the results. But thinking about the intermediary steps between like, hey, we got all this data, we're just going to train our models on it, what can we actually do in between that, including thinking about deliberating these decisions publicly, talking about why we don't do something or why we do do something? Yeah, so I just say like, it's a slow and long process and thinking about levers of legitimacy over time is going to be really critical for us. Thank you, Teddy, that's an awesome question. Next up, we have Victoria Green. Victoria, please feel free to unmute yourself. Hi, thank you so much for the talk. It was really excellent. I very much enjoyed it. My question is, is there a way to incorporate conflicting values in a way that they coexist with one another, or is the goal to try to find shared values? Because my one concern of trying to find shared values is that then that just becomes the dominant value system and pushes out values from minority groups or users or people who are impacted. And so if there's ways for conflicting values to coexist, what does that look like or how can that be incorporated? Yeah, I think we very much in terms of thinking about tradeoffs like scale and nuance, for example, this question of collective versus individual values, I think is very much one of those core tradeoffs. And in particular, is it like, OK, on one side of the spectrum, the ideal would be if you take something like chat GPT, everyone has a personal assistant that's like perfectly tailored to their own personal values. And then maybe on the way other end, it's like there's one single model that has the consensus values of the world. And neither of those really make a lot of sense. But then if neither of those make a lot of sense, like where on the spectrum do you land is, I think, quite an open question. You know, actually, this is one of the reasons we started working on evals first, because there's a sense in which they're additive, like it is possible to have different kinds of values like explored in evaluations in terms of the things you're evaluating for that don't require like arriving at consensus before you put them into practice. Right. And that's actually one of the major things we were excited about when we went through this process of figuring out, like, where are we going to start? Oh, maybe evals make sense. But I do think we're also thinking a lot about like community based value setting for language models, because it's at least my opinion that there aren't truly that many purely individual values. And so it doesn't necessarily make sense to do that at an individual level. But there are certainly community values. And even when we've done work in Taiwan, a lot of those questions around like language communities, right, like how do we deal with the fact that language communities of different kinds have different needs? And in particular, like language models are much better at certain languages than others. Audrey Tong, who's the digital minister of Taiwan that we work with closely, has this issue where much of the kind of Chinese available that the model is trained on is from the CCP. And that contains a lot of values that like she doesn't want to incorporate into her work and a bunch of folks in Taiwan don't want to incorporate in. Like, how do you deal with those questions? Well, I think that's a community sized kind of question. And so thinking a lot about like what are the kinds of overlapping communities that we can try to incorporate values from as opposed to either one of the two. I'll just add a little bit onto that. So I think one thing that is in the context of like literally what is a language model saying about a specific like contested topic, right? One thing that I find interesting is Wikipedia's editorial guideline of mutual point of view, where it's not kind of averaging all the points of view or taking the majority one. It's trying to present all of the different sides of the argument in a sort of unbiased way as possible. And I think that's like an interesting, probably a good approach. And it seems like that's what it seems like. That's the kind of way that chat GPT speaks already. But, you know, that seems like a good way of doing that. And maybe also we've been saying, like, we can put in debates and discussions and sort of polis based policies that Wikiservice will use a lot, you know, put those conversations into language models.
the things we've seen before. That presentation will be followed by the creator of ChatGPT, Emily Bender, who's going to dive into the implementation of ethical, responsible AI systems. We also have a series of webinars in the pipeline where our research team will be sharing more technical details on ChatGPT and taking questions from the forum members. So please stay tuned for those. And finally, we're planning to launch regular office hours where OpenAI team members can provide updates and answer questions from the community. So keep an eye out for those as well. Thank you all so much for being here. It's been a pleasure hosting this conversation. Have a great rest of your day.
anything we've experienced and what could potentially be holding us back from really utilizing it in our every day. So don't hesitate to register for that and you can also reach out to Connor and ask any questions about that event because he's present in this community as well.
And finally, we're hosting an in-person welcome reception for all of our new community here in the forum on August 24th. If you're able to join us in SF for what will surely be a memorable evening. I hope you register for that event as the capacity is limited.
And last but not least, I just want to say happy Thursday, everybody. It was really lovely seeing all of your faces here. Thank you to Saffron and Divya who joined us from a completely different time zone. And they're basically here with us in the middle of the night. Thank you, ladies. Thank you, Lemma, for taking the time to be here and share your work and your thoughts on democratic inputs. And we'll see you guys all really soon. Happy Thursday, everybody.
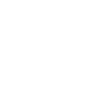