Exploring the Future of Math & AI with Terence Tao and OpenAI
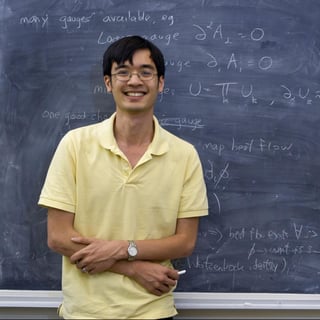
Terence Tao is a professor of Mathematics at UCLA; his areas of research include harmonic analysis, PDE, combinatorics, and number theory. He has received a number of awards, including the Fields Medal in 2006. Since 2021, Tao also serves on the President's Council of Advisors on Science and Technology.
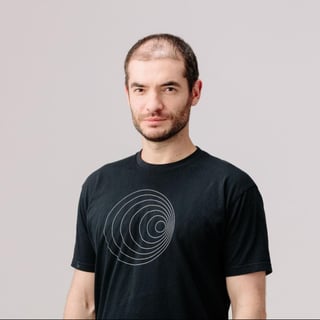
Ilya Sutskever is Co-founder and Chief Scientist of OpenAI, which aims to build artificial general intelligence that benefits all of humanity. He leads research at OpenAI and is one of the architects behind the GPT models. Prior to OpenAI, Ilya was a co-inventor of AlexNet and of Sequence to Sequence Learning. He earned his Ph.D in Computer Science from the University of Toronto.
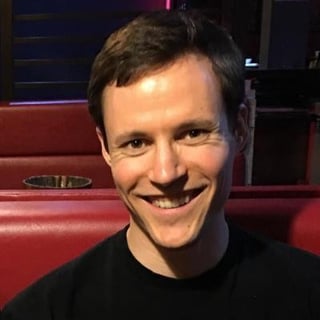
Daniel Selsam is a researcher at OpenAI. He completed his PhD at Stanford University in Computer Science in 2019, and has worked on many paradigms of AI reasoning including interactive theorem proving, probabilistic programming, neural SAT solving, formal mathematics, synthetic data generation, and now large language models.
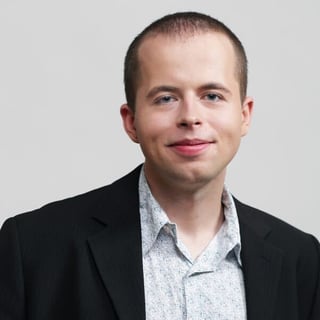
Jakub is the Principal of Research at OpenAI, where he led training of GPT-4 and OpenAI Five. He finished a PhD studying optimization methods.
I'd like to start our forum events by reminding us all of OpenAI's mission.
OpenAI's mission is to ensure that artificial general intelligence, AGI, by which we mean highly autonomous systems that outperform humans at most economically valuable work benefits all of humanity.
Tonight, we will be exploring topics related to the future of math and AI. Our guest of honor this evening is Terry Tao, a professor of mathematics at UCLA. His areas of research include harmonic analysis, PDE, combinatorics, and number theory. He has received a number of awards, including the Fields Medal in 2006. Since 2021, Tao also serves on the President's Council of Advisors on Science and Technology.
Ilya Sutskever is co-founder and chief scientist of OpenAI. He leads research at OpenAI and is one of the architects behind the GPT models. Prior to OpenAI, Ilya was co-inventor of AlexNet and of Sequence-to-Sequence Learning. He earned his PhD in computer science from the University of Toronto.
Daniel Selsom is a researcher at OpenAI. He completed his PhD at Stanford University in computer science in 2019 and has worked on many paradigms of AI reasoning, including interactive theorem proving, probabilistic programming, neural SAT solving, formal mathematics, synthetic data generation, and now large language models.
Yaku Pachoki is the principal of research at OpenAI, where he led training of GPT-4 and OpenAI-5. He has a PhD in optimization methods from Carnegie Mellon.
And last but not least, facilitating our conversation this evening is Mark Chen. Mark is the head of frontiers research at OpenAI, where he focuses on multimodal and reasoning research. He led the team that created DALE-2 and then the team that incorporated visual perception into GPT-4. As a research scientist, Mark led the development of Codex, ImageGPT, and contributed to GPT-3.
So without further delay, Mark, I will let you take it from here.
Cool. Thanks a lot, Natalie. So I'm really honored to be facilitating the discussion today. Before we begin, I just want to really quickly thank Natalie and Avital for putting this event together, to Terry, Jakob, Ilya, Dan for generously donating your time today, and to everyone in the audience for joining us. I actually see quite a few familiar faces from even before OpenAI, so from Olympiad winners, other math professors, I'm really glad AI can bring all of us together.
So for the first part of the panel discussion, I'll be running through a series of questions. And I'd like to preferentially give Terrence, Terry, sorry, the first stab at answering, but I hope that the panel will feel pretty conversational. So other panelists should feel free to jump in, you know, ask follow-ups and so forth. I'll do my best to keep time and cover ground.
So I think one fact that wasn't mentioned about Terry was that he's played around with GPT quite a bit in the past, and he's also written about some of his experiences doing so. So my first question is, how do you use ChatGPT in your day-to-day life, and are there any kind of particularly notable interactions that you've had with the model?
Okay. So I think like many mathematicians, once GPT came out, the first thing we did was we sort of vetted research questions from our own area of expertise. And the results are very, very mixed when you directly try to ask GPT to do what you are already expert at, because it does feel like asking like an undergraduate who has some superficial knowledge, and it's kind of extemporizing doing some stream of consciousness type answer. And sometimes it's correct, sometimes it's almost correct, sometimes it's nonsense.
I do find it's still useful for doing some mathematics, as well, in idea generation. So when I'm at the phase of tossing out possible things to try, if I'm out of ideas, I'm asking it, like it may give me a list of maybe eight suggestions, and four or five are kind of obvious things that I know are not going to work, two are rubbish, but like one or two are things that I didn't think about, and those are useful. You have to have the prior expertise to select those.
What I'm finding GPT is most useful for is actually my secondary tasks, like coding, organizing my bibliography, doing some really unusual latex type things, things that I would normally have to Google a lot and do a lot of trial and error. My own competency is just quite low, but still high enough to evaluate the output of the LLM. And that I'm finding really useful, and it's changing the way I do math. So I'm coding a lot more now, because the barrier to entry is so much lower. So yeah, it's changing my, it's really upskilling my secondary tasks the most, I would say.
Cool. I would love to get answers from the rest of the panel, too. Let's maybe go with Jakob first.
I think where I find GPT most useful is for, yeah, for secondary tasks, for analyzing data. I think in particular for plotting, I found it to be a phenomenal tool. I have not had very much success using it for idea generation yet.
Cool. How about you, Dan?
I use it heavily to find snippets of Python code, using various libraries and APIs. For me, that's a lifesaver. I don't use it that much for hard research problems or idea generation. But I use it for poems, short stories, jokes, and all sorts of leisure things.
Yeah, go ahead, Javier.
In my case, it would be primarily asking questions about historical events, or is such-and-such, like recently I was curious if a particular, like the causes of a particular disease, or the efficacy of a particular medication. Questions around broad, not like generally stuff which I don't know, where I don't have a particularly deep question, I just want to know the answer.
Cool. How do you find the accuracy of the answers then? I mean, if it's not your own area of knowledge, do you have to double-check them?
So, it is true that there is an element of risk involved. And from time to time, I do notice that in an area where I do know something will give me a confidently wrong answer. Like also translation between languages, or let's say, telling me the shared root of some words. So, like, I would maybe cross-examine it. But there is an element of risk, and I feel comfortable with it.
That's true. It feels like kind of there's a discovery process where people kind of become calibrated and kind of understand the level of risk and kind of what it's capable of.
Yeah. I find that certain people seem to be better at managing that. So, like people who teach small kids for a living, or teach undergraduates, or parents of small kids, seem to actually do well. I mean, I think they're familiar with the idea of an intelligent entity that makes a lot of mistakes.
I'm curious, Terry, could you say more about some example maybe of how you found GenGPT useful for idea generation?
Yeah, so just usually the very start of a problem. So I mean, a very specific problem I remember was that there was this identity I wanted to prove. And I had a couple of ideas. I wanted to try some asymptotic analysis and some other things. But just as an experiment, I asked, you know, pretend that you're a colleague and I'm asking you for advice, and just to suggest some ideas.
And so it's, why don't you try induction, some examples. So many of these suggestions were kind of generic, like any problem-solving advice in the method would try. But there was just one suggestion to use generating functions that I did. In retrospect, it was an obvious choice. It just had not occurred to me.
So I mean, just for covering more systematic ground of what to try. Sometimes you can think yourself into a rut as a human, you know, you reach for the first tool that you think of and you forget it's a rather obvious tool. So it's a sanity check.
Interesting. And it does feel like there's parallels there with kind of using it as a writing tool. You know, a lot of people will kind of hit a rut when they're writing and you want some way to break through that barrier, even if it's something that's kind of obvious in retrospect.
Sometimes if it's wrong, sometimes there'll be times when I've asked it how to approach a problem and it gives an answer that's obviously wrong. And that triggers something in my brain that says, no, you don't do that. You do this. Oh, I should do that. You know, like somehow, like there's a law on the internet called Cunningham's law that the fastest way to get a correct answer on the internet is to post an incorrect answer and someone will very helpfully correct you. So you can, sometimes you can leverage the inaccuracy of GPT to your advantage actually.
Yeah, that makes a lot of sense. I guess kind of zoning into the mathematical abilities of GPT, I'm curious how you've kind of found it along dimensions such as creativity. So I think you've alluded to a little bit of that in your, in your last answer, but also kind of insightfulness or accuracy, or even the ability to kind of calculate long chains of, you know, let's say inferences or implications.
So, accuracy is a mixed bag, long chain of calculations, possibly with the right prompting. I mean, it does really feel like, you know, guiding an undergraduate during office hours to work through a complex problem. If you break it down into individual steps and you correct the student at every step, you can guide the student to a complex problem, but you just let the student just run, you know, the student make a mistake and it will compound and they'll get hopelessly confused.
It does have uncanny pattern matching sometimes. So like you'll ask it a problem and it will somehow know that some other mathematical concept is relevant, but the way it brings it up is, is wrong. And, but, so, but then like if you then consult a more reliable source or what that, what the term being alluded to, you can, you can sometimes work out what, why the language world thought it was a connection. But you need some expertise of your own to, to do that.
So I feel like what these tools do is that they allow you to sort of stretch your skills by sort of one level beyond your, your, your normal range. So if you're a, if you're a complete novice, you can become like an advanced beginner. If you're a beginner, you can come intermediate, you can become an expert. Then most of what the LM does is not so useful, but every so often it plugs some gaps that in your own knowledge.
Makes sense. I think you returned to this point of kind of comparing it to an undergrad in some ways. I'm curious how you would compare it to, to an undergrad, let's say at UCLA, like, you know, in what ways is it better or worse or, yeah.
So it's certainly a lot broader, more broadly educated. It, yeah, at least the way that sort of default way that you interact, it's, it does feel like an undergraduate who is like at the board, a bit nervous, running a stream of consciousness, not really stepping back to think. I haven't really, yeah, I mean, maybe there's a mode that makes it much more reflective. And, but it's, I think, you know, when an undergraduate has a, has a shaky grasp on the subject, he or she will, will sort of revert to sort of an autopilot pattern matching type of approach, like make guesses based on if they vaguely remember in class and so forth. And so, so like the elements of a very polished advanced version of that mode of thinking, but that's only one mode of thinking that we use.
Yeah, it makes sense. I guess to the other panels, do you guys have any kind of different perspectives on the capability profile of GPT for mathematics? Cool. I guess, yeah, kind of returning to, you know, like the undergrad point. So I'm curious about kind of the role GPT models will have in education going forward. So, you know, like, can undergrads benefit from using this as a tool? Or, or is that already kind of at the, at the kind of advanced nearing expert category that you're, you're mentioning?
Yeah, so, okay, so, so most obviously, like, like many standard math undergraduate homework exercises cannot be solved more than just directly by entering it into GPT. They will make mistakes. So you still need some baseline of, of knowledge to be able to detect and fix them. But yeah, so, but as I said, it's, it's, it's, it's an upskiller in that way. I think the more encyclopedic students are using it as a teaching assistant, so not just asking for the answer directly, but asking to explain. I know there's experiments by the Khan Academy and some other places to actually explicitly set up a very well prompted models to, to serve as tutors, not to give the answer directly to a problem, but, but to make the student go step by step. Once you have much better integration with, with other mathematical tools, I think that'll become much more useful.
There are also efforts underway to, to use these AR tools to generate much more interactive textbooks that, or some presently, you know, so this, this is what it also combines with tools like, like, like formal proof verification. So if you maybe, if you have a textbook and the proofs have somehow all been placed in some very formal language, the AR could maybe convert that into a human readable text, which is where, like, if there's a step, which is a reasonable explanation, a student can click on it and it will expand it. And, and there'll be a chat bot that you can ask questions to sort of a virtual author of the text and so forth. So I think we're going to see much more intelligent teaching materials than we have now.
Yeah. Do you, do you know of any stories of kind of students using this already?
Well, everyone uses, I mean, they will all try it for their homework. I mean, this is, you know, at this point it's a lost cause to try to prohibit. You know, and I think we have to adapt and create, you know, so I think the type of homework questions we need to assign now are things like, here's a question and here's GPT's response to this question. It is wrong. Critique it, fix it. And, you know, we need to, to, to train our kids how to use this or, you know, or design a question that, design like an integral that GPT can't answer, but you can. You know, I think we need to sort of embrace the technology, but also really train how to deal with its limitations.
Makes a lot of sense. I think for the next question, I'd love...
To turn to our other panelists first for a perspective and then to go to Terry. So I'm curious for perspectives on when we think a novel theorem will be proved with LLM, like Chachupiti, both, you know, with a contribution alongside a human and just purely by itself.
And I'm curious for perspectives also on what kind of theorem this is likely to be. Yeah, maybe I'll call on Dan first.
There's quite a spectrum of how much it's contributing. It seems like there is a clear trend towards more people integrating it more deeply in their workflows. And so it seems very clear that it will play some, has a good chance of playing some role in most future discoveries of all kinds. As for a really autonomous role where it's posing the ideas and making the main leaps, I think it's not this generation and it's quite hard to predict.
Cool. Ilya or Jakob, any thoughts on this, like timelines or guesses?
It's hard to be... Go ahead.
I'll just say, I think it's hard to be sure, but wouldn't bet against deep learning progress.
Yeah, I think maybe the first place I would expect LLMs to contribute meaningfully to proof is problems where you need to check a lot of cases, but the cases are not very easily definable. So you cannot solve them with existing approaches, which I think resembles AlphaGo where it is similar to chess in some ways, but you cannot quite do the same brute force approach. I think that's where we could see these models excel first.
Cool. Yeah. I'm curious if this aligns with your intuition. I think there also feels like a lot of math these days becomes more about connecting two fields where there may not exist a very clear connection. I'm wondering if maybe GPT can help with some of that pattern matching or...
I think it can. I think we haven't discovered the right way to use it necessarily. I think it will be complimentary more than a direct replacement of human intelligence in many ways. So as I said, making connections. So I could see that some mathematician in one field said, let's say analysis proves a result and GPT says, you know, this result seems connected to this result in topology, in quantum field theory, other areas that you wouldn't think of. This is currently sort of what conferences are often about. You give a talk and some of the audience says, oh, what you're saying is connected to this. We're making these unexpected connections. I think there's a lot of future promising, say, automatic conjecture generation, that once you have big data sets of mathematical objects, you just get the AI to fall through them and discover all kinds of empirical laws or conjectures that then maybe some combination of humans and computers could then resolve.
It is already very good at mimicking existing arguments. So I was recently writing a blog post actually about mathematics and using a VS code, which has a GitHub co-pilot enabled. And so I had this integral and I was trying to compute this integral and estimate it. And I broke it into three pieces and I said, okay, piece one is I can estimate by this method. And I wrote, I tapped the method. And then co-pilot surprised me by supplying how to estimate the other two parts of the integral completely correctly, actually. And so it was like, you know, an extra two paragraphs of text. So I could see very, in the near future, a mathematician will do one, will write down in full detail one case of, or like solve one problem of a certain type. And then the AI can then solve the other 999 problems in the same class, almost automatically. And that's just a type of mathematics that we just can't do right now. You know, mathematics is still kind of a very bespoke, handcrafted, artisanal type of craft where each theorem is sort of lovingly handmade.
Yeah. It does seem like a common theme is, you know, like case work heavy kind of mathematics. So maybe, you know, like characterizing like all groups of a certain type or like, yeah, something like that.
Cool. I guess one question I have is, let's say we do make some more progress on the AI front, right? How does mathematics look when we get to a world where, let's say an automatic theorem prover could prove statements, you know, at a rate faster than humans? So maybe like an expert mathematician kind of working on a problem would take a month to a year and this kind of system could give us a proof within a day. Like how does that change mathematics and the landscape of mathematics?
Yeah, I think it changes the speed and scale of what we can do. I mean, it's happened in other fields, you know, sequencing a genome of a single animal used to be an entire PhD thesis, you know, and now you can do that in minutes. It doesn't mean that PhD students in genomics are out of work, you know, they do other types of science, maybe much more large scale things that they couldn't do before. You know, I mean, what mathematicians do has changed so much over the centuries, you know.
In the Middle Ages, you know, mathematicians were hired mostly to make calendars, you know, to predict, you know, when is Easter and when do we plant our crops? And then, you know, it was how do we navigate the globe? And then, you know, or, you know, in the early 20th century, how do you compute integrals? And how do you model a nuclear weapon or something? But, you know, all these tools, all these things we can now do more or less by automated tools. So mathematicians do other things, we do some more higher order tasks. So yeah, we won't be doing, when that happens, we won't, the math we do will not look like what we're doing now, but we will still call it math. I don't actually know what it will be like. I'm curious if the other panelists have some kind of vision of, you know, like a post-AGI scientific world or what that would look like.
Ilya, it looks like you unmuted.
I mean, I can go very, like we all, like very briefly. One thing that we've seen was what happened to chess. Chess used to be, you had those great chess players. Now the computer is the great chess player and it turned into a spectator sport. Maybe that's something that could happen. More kind of a, well, something like that.
I have a question for Terry, a follow-up question. How might radically accelerated progress in mathematics affect the world rather than how might it affect mathematics?
That's a good question. I could see it, I could see improved models and discover like new laws of nature. Like discovering new laws of nature is really hard right now. You know, you have to be like, you know, an Einstein level sort of, you know, to actually, you know, but because, you know, you can make a hypothesis and then you'd have to do a large scale experiment or a lot of theoretical calculation to predict sort of the consequences of your hypothesis. And then see if it's the data and then you have to go back and try again.
this type of process, you just hypothesis generation and testing. It's a combination of both sides of math and science that, if that gets automated, we could really accelerate all kinds of science and technology.
I think, you know, there are all these materials that we would love to have in the room, such as superconductors, or, or, or improved solar cells or whatever. And, you know, while we have some of the basic laws of chemistry and physics, in principle, to sort of understand when, what the material properties of various things are, you know, our modeling capability, we don't have the mathematics and all the supercompute to actually model that. But with AI, either through by some combination of theory, like actually proving things mathematically, and also just, you know, direct machine learning, trying to predict the properties without actually going through those principles, that I think will be quite transformative.
Cool. And just to piggyback on kind of Ilya's answer for a little bit, I'm curious what your intrinsic motivations to do math are, like, is it something about discovering new knowledge? Is it kind of more the joy of kind of going through the process? I'm curious what kind of makes you so, yeah.
Yeah, it's, it's both. I mean, I'm a firm believer in Ricardo's law of comparative advantage, you know, that you should, you know, you should try to contribute where you can do more good relative to other people. And, and so, you know, I mean, yeah, I can, you know, I can do math, I can do a few other things, I can program, for instance, but I am not as good a programmer as, as, as, as a professional programmer. So, and that, that I can, yeah, so, you know, I think I can, I can do comparatively. That's my strength. I certainly enjoy making, making connections. And I enjoy actually communicating mathematics to others. And, you know, I've, you know, I've trained many students and seeing them sort of develop over, over time, it has been a great pleasure.
Cool. Maybe also to follow up on Dan's question, I'm curious, if you if we only automated the fear improving part, so let's say we had an AI system that could prove in a minute, what you could reasonably prove in a year, a formal statement. How do you think that alone would would change the world?
See, okay, so yeah, the the influence on mathematics on the rest of science is a little indirect. So yeah, so sometimes other scientists, you know, like physicists and so forth, they sometimes sort of tease mathematicians that, you know, you're trying to prove something that you that we already know empirically to be true, like 99% confidence. Why do you care about making it 100% certain with proof? So sometimes there are surprises that sometimes mathematically, there's a, you discover that a certain thing, you know, there's a symmetry that breaks, there's some phenomena that everyone thought would never happen, and it does happen. And that can sometimes lead to an experiment of verification. I mean, it's also just a different way to probe. As I said, like, you know, there are ways to, to just use pure thought, use mathematics to, to understand the universe without experiment. A very classic example is Galileo. So, you know, Aristotle used to think that that heavy bodies fall faster than light bodies. And everyone just accepted this fact. Now, the story goes, you know, Galileo, the way we teach it, or in popular belief, you know, Galileo dropped like two, like a cannonball and a small ball off of a tile piece or something. But actually, he actually disproved this with a thought experiment. He said that supposedly, you have a small object, a light object is connected by a very light string. So it's a single object, so it'll fall at a single rate, and then you just disconnect the string. But if the string is negligible, it shouldn't actually have any effect on the motion. And just by pure thought, you can actually easily see that Aristotle's theory is inconsistent. So, you know, with possibly with really advanced, you know, maybe people will make theological fallacies. I mean, I think people often, you know, they make financial mistakes, because they don't understand probability or, or compound interest or, or very basic things. Yeah, I mean, maybe it's a little bit naive. I mean, there's so much rationality in the world. But in principle, you know, having a very rational, mathematically informed AI is an assistant to everybody could really help everyone's reasoning abilities.
Mm hmm. Yeah, I guess, to kind of expand on the formal theme, I'm curious what your current views in terms of, you know, how important are formal systems to to mathematics these days? And I think one follow up question that's, I think, a couple of us on the panel are curious about is, you know, like, does formal mathematics generalize to all of human reasoning? So you have a system that's just amazing at formal mathematics? How much, you know, does, does that kind of cover all of human reasoning? Or do you need something, you know, else, that's a little softer, or qualitative?
It is one mode of thinking, I think, it will help. Okay, so, like, you know, so the lifecycle was already captured, but somehow, they captured the intuitive pattern recognition type of reasoning, which is one mode of thinking. Formal rigorous thinking is another mode we have. And then there's like visual thinking, and then there's sort of emotional based thinking. I mean, I think there are other ways to, to make conclusions. So I haven't thought so much about, yeah, I mean, I think it's, formal reasoning is limited to mostly formal situations. But I think it has great promise in really complementing the biggest weakness of the current language models, which is the inaccuracy. But that if you force the LLM to generate output that has to pass through a proof verifier, and so you can only say that you're asking to do mathematics, you know, if you without the proof verifier, you can make mistakes. But if what, what the output generates has to be verified, and if it doesn't, it gets fed back to the LLM, and it doesn't reinforce the learning or whatever to, to, to train it out, then it becomes a much more useful system. And I think, conversely, like these formal proof verification languages, they're quite hard to use. I've been learning, I started picking up one of these languages just a few weeks ago, I just started learning it. And I just proved my first basic theorem, which was like, like, one equals one kind of level of prevent. It took me like an hour to get to the point where I can actually get off a syntax rack to do that. You know, there are experts, you know, people who have spent, you know, years using the languages, they can, they can verify things quite quickly. But like the average mathematician who can prove theorems in natural language, they cannot prove theorems in this formal proof assistance. But with the, with LLMs, there's really this big opportunity that a mathematician could explain a proof to an LLM in natural, in natural mathematical English. And the LLM will try its best to convert it line by line into a formal proof and come back to the human whenever there's a step that they need more clarification. And so I think you can see much more widespread adoption of formal proof methods. But conversely, as I said, these formal proof verifiers will also augment the LLMs quite a bit. And possibly, once you figure out how to do that, this may help more broadly solve the hallucination problem. I mean, you can't use a formal proof verifier to verify all types of
reasoning, but possibly some of the lessons are transferable.
Cool. Yeah. That's a very interesting perspective. Like I could be using the verification process and maybe there's some interplay between, you know, models and humans where models will go back to humans and ask for kind of expansions on key parts of the proof.
Yeah. Interactivity is the key.
Yeah.
Yeah. I guess you touched on a couple of things, you know, like modes of thinking, like there's kind of informal thinking and kind of a formal mode and maybe like a visual mode. And I was curious just to get some more insight onto kind of your thought process when attacking a complex math problem. Are you, do you like flip between these modes? Do you focus on one of these modes? Kind of when, can you kind of characterize like when you come up with insights?
This is a good question. It's actually, I don't really know. Part of the problem is that I'm not an external observer to my own thinking process. It's actually easier to see me see how a student thinks when I'm watching them on the board than to observe my own thinking. I am not a particular visual person myself, actually. I reason a lot by analogy, like I do a lot of translation. So I may be working on a problem which is written in algebraic form. And then at some point I realized it has a kind of geometric structure. And then so I should maybe translate everything into some geometric form. And so I do a lot of calculation. And then I have a geometric, I now have the problem expressed as a geometric problem. And then I just notice something else. Maybe there are many points in this configuration, but one of them is always in the center or near the center. And then that's somehow an important fact. So often like you just haven't noticed, just from experience and pattern matching, you notice that sort of one piece of a puzzle, one piece of a possible approach, and then you have to work to fill in the rest.
The process was a little bit like sometimes if you watch a lot of movies and TV shows, and then you watch a TV show that you haven't seen before, sometimes you can guess the plot or guess what's going to happen the next five minutes. Because you see someone come in and say, oh, probably this person is going to do something dramatic. Because you recognize some tropes. And then you can kind of fill in the next steps by yourself. So there is a lot of pattern matching, at least the way I do mathematics. Once something looks like a paradigm that I think I'm familiar with, then there's a lot of computation. I can almost never solve a problem in my head. I get an idea, then I have to work it out. Often I make a mistake and then I have to correct it. More recently, I sometimes test things on a computer. I have tried chatting with GPT during this process. I find, as I said, at the very initial stages of suggesting an idea, it's useful. After a while, the signal to noise ratio is not great. Once I start knowing how to do things myself, it's best to just do it.
Cool. Yeah, thanks a lot for that answer. I think one question we had also was in kind of defining AGI or ASI. And we're curious, what concrete milestones would make you think that we're close to achieving human-level reasoning? Is it something like we want to solve all the IMO problems or something like that?
Well, I mean, I don't need to tell you what the AI effect is, right? Any target you use, whether it's solving chess or image recognition or whatever, it's a good goal until it's solved. And then you realize it wasn't actually the goal. I think we don't understand what intelligence is. And I think one of the byproducts of AI research that is really quite exciting is that we actually learn a lot about what human intelligence is. And we don't have a definition. We sort of can recognize symptoms of intelligence, signals, but we don't recognize intelligence itself. So every benchmark, it's a good short-term goal to advance the subject forward. But I think it's definitely premature to say that we have the ultimate definition of intelligence and the ultimate benchmark.
Could you expand on that a little bit, just kind of like what kind of insights maybe into human intelligence have you had as a result of seeing the AI progress?
Well, so the fact that these language models can carry on a very human-sounding conversation and sound very natural, I think what it has told me is that that's something that humans can do in autopilot. And actually, as someone who's raised a small child, I think they already realize this, that a child can babble and actually be reasonably coherent without really having a deep understanding of what they're saying. And I think intelligence is to some extent a cognitive illusion. I mean, there are times when we really are thinking deeply and really using all of our mental capacity, but that's actually quite rare. I think for most of our daily tasks, we act on instinct and pattern recognition, and our brain fills in the gaps. And sometimes it provides retroactively rationales for why you did something that you did. And so I think we give ourselves the illusion that we're more intelligent than we are. I think it helps us keep us sane. But yeah, I mean, it's been surprising to me just how much of what we do can be done on autopilot. And just by using this pattern matching level of intelligence.
Yeah, I've noticed Ilya was pretty expressive during that answer. I'm curious if you have anything to add, Ilya.
Very little. The only thing which I think I may have had an area, maybe a nod, is I think it's very unclear where pattern matching ends and reasoning and deep intelligence begins. And I think often when thinking about where deep learning is going and how do we recognize if language models are becoming smarter and what it means, we do look to math and math competitions as kind of a standard for formalized reasoning that currently seems like a clear weak point for language models. So I'm very curious, let's say we had a language model like GPT-4 that was capable of carrying on the conversation, but also it could solve any IMO problem, including these more novel combinatorics problems consistently. What do you think that system could still be missing compared to humans in terms of ability to do research or progress technology forward?
Well, certainly I know that humans who are very good at IMO olympiads, some of them go on to be research mathematicians and many don't. It's a different skill. It's more of a sport, it's a controlled environment where the problems are sort of known and they're balancedly solvable. And the techniques are, there's always a standardized set of techniques. You know, you're not expected to invent really deep new mathematics to solve a problem. So this, I mean, some skills are, I mean, like I found, you know, I participated in olympiads as a child. And sometimes there are certain little steps in a long argument in my research that are having a little bit of an impact on the way I solve the problem.
You add fuel to them. They're sort of self-contained mini-game, if you wish, where there's a certain small number of rules. And there I find the Unloopy training to be useful. So it will definitely help.
I think how the actual gets to solve these problems may be even more useful. Is it a new architecture? Or is it just 10 times more training?
There are other aspects of doing mathematics, forming conjectures, making connections, articulating general principles. Mathematics is not a single, unified, one-dimensional activity. But I think it's a good benchmark because it's definitely beyond our current capability. So any progress for that goal will be useful.
Yeah, I guess a lot of our focus is on taking known problems and solving them. I'm curious how much of mathematics in your eyes is the problem of coming up with the right new problems and forming the right kind of aesthetic conjectures.
Yeah, there are so many types of ways to do mathematics. I mean, sometimes taking something that's already been, I think, understood, but explaining it in a much better way. Unifying two concepts into a single more abstract concept without actually changing much the arguments, but just changing the perspective or the emphasis even. Sometimes even giving things the right name can be extremely influential. So the concept of what a number is has been generalized over and over again in mathematics. It's almost unrecognizable to say what the Greeks would think of as a number. But these are really important conceptual developments.
There's a social aspect to mathematics. I mean, it's not just... Nowadays, if you want to interest people in your work, you have to actually do a good job communicating it to other humans. And the effort of doing that has actually transformed sort of maybe the directions in math we go to. And I mean, some areas are more popular than others, but also we try to arrange, we provide motivation for what we do a lot more than we used to in the past. Partly because we also have funding agencies that we need to make money from and so forth too. But there is also, I mean, it's not just individual mathematicians solving problems. There is kind of a super intelligence of the whole community trying to move in certain directions.
Yeah. That part of... So there's a social aspect, which maybe the AI the automation won't touch until the AI has become fully-fledged members of the community. That's socializing with us. That's way in the future.
Yeah. I mean, it's so multidimensional. I mean, I think you can't reduce it to one activity or another.
Natalie, just a very quick check on time.
I know you've been working on this for a long time, cool.
Natalie, just a very quick check on time. We have three minutes, so maybe one more question.
Fantastic. Awesome. Yeah. I'll end with maybe one last question. So one of the primary goals at OpenAI is not just to build AGI, but to build safe AGI. And we imagine AGI eventually becoming very powerful with the ability to do its own research, recursively improve itself, think about really hard problems and maybe interact or act in the world. And I'm curious how much thought you've given to the AI alignment problem, making sure the AI doesn't go rogue or have any kind of large negative societal impact.
Yeah. I mean, I think a lot of it is... I mean, it is an interesting question, but the other technologies that we have, the existing automation we have, we almost never shoot for 100% automation because precisely because of all of these risks.
So, I mean, we can partially automate driving and aviation and financial trading and so forth. But we don't completely run things on autopilot and we shouldn't, I think. I mean, there's a diminishing returns to automation up to a certain point. I mean, so I think 100% automation is not necessarily the end goal. I mean, maybe just automating the humans, but not replacing a human.
I think there'll be so many visible examples of 100% automated AI going amok. I mean, not in the sense of killing all humanity, but just sort of failing in hilariously bad ways that I don't see... You don't see people experiment with completely automated cars. Well, I mean, we're trying a little bit now, but I mean, I think at least in the near term, in the next few decades, we can already leave a substantial portion of this AI just by partial automation and just using AI to empower humans. And theoretically, you could turn everything to a pure AI, but it's not clear that outside of some very specialized subtasks that this is necessarily a thing to do in general. In part because we can't solve the alignment problem, but maybe we don't have to, to have a much more useful technological tool.
Yeah. Thank you.
Thank you, Greg. Anybody else want to jump in? Oh, Yufi, I see your hand. Welcome. So happy to have you, Yufi. I think you know some of the panelists.
Thank you very much.
Hi Yifei, good to see you again. So as, you know, I'm a research mathematician myself and one of the often frustrating routine challenges is reading and checking whether some written proof is correct, right? So this includes my own writing draft as well as other people's writing on the archive. I think of both refereeing, but also just trying to understand what's going on in these proofs.
And I wonder, you know, in practical terms, how soon can we expect an AI tool that could dramatically assist us in this part of the research process?
It's already beginning to happen. I mean, you can already upload a PDF, maybe not directly to GPT, but there are other APIs and things that do this. And, you know, so I use something called ChatPDF. I'm not quite sure what it's powered by. And it can give kind of a high level description of the thing that they can tell you like what the main theorems are. It can summarize in its own words what the main ideas are. It's not completely accurate, but it's a start.
And then at the low level, we have these formal proof assistants that can take individual steps and turn them into rigorous formalized proofs. And there's beginning to be software that they could turn those formal proofs into a very interactive document that you can use to explain. And at some point, like maybe both the high levels of AI interpretation of a paper and the low level formalization will start merging. Once formal proof verification becomes user friendly enough, hopefully the AI, the language models provide enough kind of a pleasant interface that the average mathematician can write their papers no longer by typing LaTeX, which is normally just like by explaining, you know, in plain English to an LLM, which will then write in LaTeX and in a formal language. And the end product could be something that is already in a format suitable for explaining at whatever level you like.
I mean, GPT is already great at taking a topic that is well covered. I don't know, say nuclear fusion. And if they explain it at the level of a 12 year old, as an undergraduate and so forth, it's already good at doing that. You can't do that yet with a math paper, but that's well within the possibility in a few years.
Have you tried feeding one of your own proof lemma drafts into GPT for the check for mistakes?
Not to check for mistakes. I don't think checking for correctness is the strength of a language model. I haven't really tried.
Yeah. Some very bite-sized statements, I've tried it. And then, yeah. And then to get the high level picture, it's somewhat accurate. It's not really ready for prime time yet, but it has potential.
Daniel. I just wanted to add a little bit to the possible promise of formal math for this exact problem. So Peter Schultze was genuinely in doubt of one of his results. And with the lean community's help, they formalized it and gave a lot more confidence in the veracity. My understanding is that most of the work was after the formal statement, and something that there's no philosophical barrier to automating with a more advanced GPT. And so really, there's maybe just a little bit of a connection there of also getting the language model to write the formal statement in a way that the human can audit, who is maybe not an expert. There's a clear path to automatically verifying much more sophisticated. It could be that the only bottleneck is data. Like if we have enough, if we have more data sets of people formalizing proofs, maybe the language model can just take it from there. Thank you, Daniel.
Anybody else wanna throw their question in the ring?
I've got one.
Nikhil, what's on your mind?
Hi, Nikhil. I had a lot of people... Hi, Terry. Hi, Akub. So it's more a question for the AI researchers. So there are a lot of questions of the type, when do you think these models will reach graduate level or postdoc level or so on? But how do you begin to answer these questions at a scientific level? Is it based on looking at some scaling laws or like how do you actually begin to make these predictions?
Yeah, this is a question we've thought a lot about. One thing we can predict with a good degree of confidence and what scaling laws are about is that there is room for these models to continue improving and we can predict how they will improve at the core task we are training them on, which is predicting the next word in arbitrary text. And I think it's a good question. And so for that, we have concrete predictions. We can kind of say, if we expand this much compute in this way, we'll get a model that is this accurate. And the big question is, how does that correspond to actually being able to do things and actually being useful? And on that, our understanding is much more limited. And I think for GPT-2 and GPT-3 and GPT-3.5, every time you see these models, you see like, well, they are clearly a big step change from the previous thing, but probably nearing the limit and probably the next thing will not be quite as good. And probably we are missing something fundamental to really push it forward. And I think so far we've been surprised every time with the new capabilities that these models show. So I think maybe this time expectations are maybe a bit higher for the future and we are less confident there is some clear plateau that we're approaching.
I still think we can be more, we can still develop methodology to actually be able to predict when we will be able to solve particular problems. So one thing that we have studied for GPT-4 is trying to predict when we'll be able to solve problems of a certain difficulty. Consistently, and we've observed that there is also a certain scaling law that if you can solve a problem very infrequently at some scale, at some level of being able to predict the next word, then as that improves, you can predict you will solve problems much more consistently later. And there are ideas on how you could extend that methodology to problems that current models are just completely unable to solve, but that's an active research problem.
Now there, I want to add another very quick comment here.
To the already, to the comments that were said earlier. Some of the remarks describing the capabilities of the models were like, well, this is an undergrad and then you have a grad student. And one thing that's worth keeping in mind that these models are extremely non-human in their capability profile. It's like a human being some without frontal lobes or it's something different because it's obviously very superhuman in some ways and it's very not human level at all in other ways. So it's radically better than GPT-4 is radically better than an undergrad in terms of its breadth of knowledge and the way it sees connections and the way it has this obviously superhuman intuition. But on the other hand, if you botch simple calculations and make simple errors and fail to find obvious flaws in a proof, be unreliable. As the reliability crosses a threshold, that's where things will stop.
start to get really different from what they are right now.
Thank you, Ilya. Thank you, Nikhil. Wonderful question.
Ryan Kinnear: Hey, so thanks for taking the question. So there's a lot of discussion about how you might use language models to do math, but not so much discussion about the math of the language models. So maybe is there, so for an example, there's a Millennium Prize problem inspired by fluid dynamics. There's certainly lots of other interesting pure mathematical ideas that have come out of other areas, engineering or so forth. Is there anything related to language modeling or machine learning that you think has inspired a truly interesting purely mathematical problem? I've asked some experts in this. So far, I don't, there's nothing on the level of say, P goes NP, which has really motivated a lot of very good mathematics. I think machine learning is still quite an empirical science in many ways. And it's a bit opaque, actually quite a bit opaque how these things work, even though a basic theory is there. So I think at the current stage of knowledge, not really, but people are still trying. There are some non-trivial math questions coming out of, inspired by neural networks, but nothing as sort of as central as say, P equals NP. What I found talking to applied mathematicians, a little bit of math can go a long way. I think the way one of my colleagues described it, it's like chapter two of a math textbook is very useful for applied mathematicians. But then after that, it's decreased exponentially how much value they get out of the remaining chapters.
Scott Aronson: Do you have a question for the panel?
Hi Scott. Yeah, hi Terry. So when we think about the greatest open problems of mathematics, like P versus NP or Navier-Stokes that were just mentioned, I can imagine someone saying, well, maybe we shouldn't kill ourselves at this point to solve these things because in 10 years or 20 years, it's as likely as not that AI will be able to help us. Maybe that's faster than humans will be able to do these things. But I could imagine someone else saying, well, for the sake of human dignity, I want humans to have solved these things. And if we only have a 10 year deadline for that, then we better get cracking now. I mean, do either of those ways of thinking have purchase on you?
Well, the great thing about math is that the problems are not fixed. I mean, we select our own problems. And this is something that sort of already happens. Like mathematicians get scooped by other mathematicians all the time. And it can be emotionally annoying when it happens. But it's also, but actually it's a good thing. I mean, so once you get past the initial psychological shock of someone proving what you wanted to prove, well, first of all, it's validation that what you're working on is an interesting problem. But also often the solution to problems opens up many more problems that you can now solve. And it's a net positive. So I mean, it's not, mathematical problems are not really a finite resource. So it's certainly not the constraining, limiting risk. The bottlenecks to mathematics is a lack of problems. So I would not worry about AI causing a math problem shortage. That's really, out of all the AI risks in the world, that's like number 110, really way on the bottom.
Well, until it can solve all the problems, right?
Yeah, but then the fact that it can solve as a subset of all the math problems is not, that will be a very niche concern, I would say.
Daniel: Did you want to hop in? You're muted, though.
Yes, hi, sorry. To the point that mathematics does not have a finite number of problems, but has a potentially infinite number, one of the challenges to scaling language models further is the limited data from the past. And the idea of there being this infinite set of, this infinite playground, where, especially in a formal regime where you can automatically tell whether something is logically sound, it's very tantalizing from a research perspective. Do you have thoughts on how you might formulate an objective to say whether a formal theorem is interesting? Or do you think there's any way to turn this into a big open-ended game that language models can play?
That is actually a great question. Yeah, so Go was mentioned, AlphaGo was mentioned previously. One thing that language models are potentially very good at is making qualitative assessments of whether, for example, whether a mathematical statement looks easy or difficult, but also whether it looks interesting or hard. And maybe once we have good AI tools of doing that, that can help us guide mathematics in very productive ways. So it can actually help us sort of generate the vision for future direction. But there's no data on this. There's no data set of what is interesting mathematics and what is not interesting mathematics. So correctness is a much more promising near-term goal, because we at least have a lot of data on what states are correct or not. But yeah, in the far future, I can see AI actually making really good value judgments, not just in mathematics, but elsewhere. But I think we do need some non-data-based AI tools. So language models are great. But yeah, they need data, huge amounts of data to function. And so a large part of doing science and mathematics is actually moving into regimes where we don't have good data sets. But I mean, I don't think that's going to happen. But I mean, maybe still a way of reasoning is so similar enough to previous years' reasoning that you can use reasoning as the data. I don't know. I mean, humans don't reason by massive amounts of data exclusively. I mean, they definitely do to some extent. It's not the only way we think.
Quick follow-up. You mentioned before about using GPT for a somewhat routine idea generation. But have you explored its sense of taste in conjectural definitions?
I mean, the time that I haven't tried very hard. I mean, the thing is, it captures the form of these things much better than the substance. So I've asked it to generate a potential talk, like someone's playing with the Riemann hypothesis. So what would the title and abstract look like? And it will produce a convincing looking sort of talk that I would actually go to. But then you ask it to elaborate, like what if you said the talk, and then you realize it's all nonsense. So I mean...
I mean, it's more like the question of, is this theorem statement, is this conjecture interesting? Which is a little bit more of an intuition. I haven't tried, but I suspect that it's just too far from the data sets that it's trained on to. I would expect that it would not give great results, but I haven't tried.
On the comparison of math to Go, I think one important feature of Go and other games that deep learning has found success in is that the space is not only kind of has a closed infinite number of problems, but also it's kind of smooth where you can improve a bit on easy games or easy positions and go from that and improve beyond human ability. And in comparison, naively at least, the space of math problems feels much sparser where you have to make big leaps. Okay. It depends on how you sample. So if you randomly generate a math problem out of random symbols, then neither humans nor AI will ever...
Inverting a cryptographic hash function is basically impossible. That's why we use cryptographic hashes. AI is not going to break crypto. Crypto is done correctly. It can be refined, the loopholes and so forth. But yeah, but the, you know, it's like the drunk person looking for the keys under the flashlight because that's where they can actually have a chance of solving the problem. You know, the problems mathematicians solve, the areas that we work in by nature are the ones where the solution space does have structure. And I mean, the structure often took us decades to realize, you know, that there are certain directions in which you can move. And, you know, it takes years for humans to understand how to work in advanced geometry or whatever. But AI can navigate the space in principle quite well.
Yeah, so I mean, yeah, I mean, if you focus on the areas of math where mathematicians actually like to work in, I think the situation is a lot better than in just random generic math problems. But I'm curious, even within those spaces, right? It's not clear that you can find a smooth gradation of problem difficulty, let's say, as that of, you know, ELOs in Go opponents.
Yeah, maybe not for mathematics in general, but for very localized tasks, you know, like you want to prove a specific theorem, you know, using a certain set of tools. So the type of thing that you do all the time in the form of proof verification. I think AIs could have a reasonable chance of assessing what intermediate steps might be sort of halfway between your hypotheses and your goal, and how to split up a problem into two problems of half the difficulty. And then have sort of tree branching sort of AlphaGo style, maybe way of solving sort of specific lemmas and things like that. I think that has a game-like feature to it that might actually be feasible.
Thank you. Shrenik, Shah, I'd love to hear your question. You'll just have to unmute yourself. The icon is at the bottom left center of the screen, the microphone icon, Shrenik. The very bottom of the screen below Daniel, Ilya, and your face. You can also type it and I'll ask it for you. I think earlier Shrenik had typed something he asked, perhaps I'd ask whether AI might be in the future more effective in fields that are theory building, like the Lang Lang's program versus technique development, like combinatorics, some kinds of analytic number theory.
It's not really quite, it's not a really well posed question because that's a fake dichotomy. Yeah, so I mean, that's much more in the purpose of me as a mathematician myself than the theory building. It's theoretically possible. I think, again, there may be a lack of data. So we have a lot more data on, there are millions of math problems out there, ranging from elementary level to advanced graduate. There are not millions of math theories out there. So it's not clear to me, that is maybe I mean, again, you could maybe generate plausible pseudo math theories, things that look kind of like math theories, but then they don't have the detailed inspection makes them fall apart.
So this actually is something you were just addressing in a way, but suppose we get to a point in the future where AI has the ability to solve some kinds of math problems and not others. Do you think it's more likely to be successful at addressing the types of mathematics where you kind of have to build up a large amount of structure, create new mathematical notions and objects and so on to build up to proving some major conjectures? Like for instance, like the proofs of the various conjectures about pure and mixed motives or the resolution of the Langlands program, their balance and conjectures versus areas which feel like there's a lot of different techniques that are developed. And then when you combine them in extremely complex ways, you can prove stronger theorems like mixing sieve methods, like sub-convexity bounds and things in analytic number theories, like the example I have in mind.
I think, I mean, it may not necessarily be language models, but I think there will be progress in both these directions made by different AI tools. So, you know, different fields have different bottlenecks. So like some, as you say, we know what the tools are, but the question is how do you combine them to optimum effect? There are some where we don't have the right definitions or the right conjectures to make. And yes, some we don't even know what the questions are. Like the questions that you start, like the initiative that you would make end up eventually becoming less relevant as time goes by. So in all these areas, there is a chance that AI tools could help. I mean, it's a question of what architecture you're using, how they interact with humans, what data sets you have. Yeah, I don't know. But as I said before, also, I think the way we will do mathematics, it's, these questions may be moot in 20 years because just our entire practice of mathematics is just, it's unrecognizable from the way we do today. And so arguing how, you know, as I said, like in the early 20th century, like a lot of mathematicians were computing integrals, exact integrals, and doing a very basic numerical analysis. And we just don't do that stuff anymore. And so arguing which automated tool solves these problems, any automated tool sort of solves those problems, but that's not what we do. So I think it's premature to ask the question.
Thank you, Srenik. Well, it's our last 10 minutes, and I fear we might not have Terry back for a very long time. So does anybody else want to throw their question in the ring? We are happy to give everybody their time back, but again, this has been such a special moment. And if anybody's holding back just because you're shy, please don't. I think there's someone in chat.
Yeah, I see. Oh, Ilya. And after Ilya, Ryan.
Ilya, you can go ahead and unmute yourself.
Oh, I apologize, I don't have a question.
Okay, okay, how about Annie Kwan? We haven't heard from Annie yet.
Hi, nice to meet you. Thank you so much for this session. My question is related to just if AI is going to take over a lot of these, you know, basic math. I'm just curious how we can educate the next generation of students, like what we should focus on given that we have AI now, which we've never had before back when we were students.
Yeah, well, you know, we've had to adapt in the past to, you know, calculators and the internet and the question and answer side that can already, like you can crowdsource a lot of homework questions to humans already, and now you can crowdsource a lot to AI. I think, you know, AI tutors will become very widespread. So, I mean, that, you know, that basically you have 24-7 office hours where you can talk to a teaching assistant, that AI assistant that will, you know, it's often in a math problem, if you're not supervised, you can get stuck at a step and there's nobody to nudge you. You can just, it can be very frustrating. I think we will have to be more creative about how we design assessments and how we have to somehow weave in the AI tools into our homework that we assign. As I said, I gave some examples of trying to get students to critique an AI-driven answer or to, I don't know, maybe use the AI to generate a new question and try to challenge each other to solve it. I don't know, it's very, very early days of how people are going to use it. As I said, textbooks, I think, are going to change quite a bit. Textbook technology has not advanced that much in hundreds of years. I mean, the graphics have become slightly better in typesetting, but I think we could really see really interactive textbooks. And, yeah, and one way you can calibrate, you know, if there's a passage that's too hard, just say, can you explain this part of the text at the level of a five-year-old, or can you make it into an animated comedy sketch or something? And, you know, everyone's got their own different way of learning, and like really personalized, mass personalized learning could really be a potential thing that AI can deliver.
Annie, thanks for your question, it's good to see you.
Thank you.
Stephen, did you want to unmute yourself and ask your question? You've been writing a lot in the chat.
Sure. Hi, Terrence, yeah, thank you for being here. I wonder how often you've been pitched on working on alignment directly, and in particular, you know, is it off-putting, what has been interesting? There's obviously a lot of discussion of getting very smart talent to people like yourself interested in working on it.
I did get a phone call from Sam at one point. Yeah, no, it's an interesting question. I think it's not my comparative advantage. I mean, it's not a question that is really mathematically well-defined to the point where sort of the skills that I have are really the right ones. It may be that you really need people from the humanities, actually, to address this problem currently. Yeah, no, it's a great question, but there's plenty of other people working on it, so I'm happy to let them think of these hard problems.
Daniel, did you want to hop in? You've had your hand up for a while. But you're still on mute.
Hi, sorry about that. I was going to ask, Terry, why are humans so good at math in the first place? It seems like phenomenally interesting that these abilities are so strong, despite it not being that obviously significant in the end.
So, right. I mean, we don't have a specialized math portion of the brain, but I think we have general intelligence, HGI, I mean, evolution has given us various modules of thinking, right? So there's a vision processing module, and there's an amygdala, right? And there are certain problem-solving modules that I think, as primates and so forth, we had to develop, and how to run away from predators and whatever. But we also have, there's something in the human brain that lets us repurpose some of our modules for other tasks. So, I talked to different mathematicians, and some are very visual mathematicians. They have somehow repurposed the visual center to do mathematics. And some of that have repurposed their verbal center, and some have repurposed some of their fight or flight. So I don't know. It's somehow, we have enough raw material in our brain, like raw capability, and some way to learn and refine skills. That lets us do complicated things. I mean, not just math, but art and so many things that evolution doesn't directly give us the ability to do. But we just have a lot of raw material in our brain that doesn't directly give us the ability to do. But we just have a lot of raw primitives somehow, the cognitive primitives that can be harnessed in emergent ways. Somehow, we, our intuitions about logical correctness are extremely, extremely accurate. When the formal system, the formal representation of these arguments is unbelievably complicated. I'll say that this requires training, by the way. As I said, you talk to an undergraduate, their intuition of formal correctness is always insanely accurate. I think there's also a social intelligence component. I mean, we are good at math because our society has a collective institutional knowledge, and we teach our younger generation, and we have books and pedagogy and so forth. If you just sort of clone humans in a vat and set them on an alien planet, it's not clear that they would have anywhere near the type of math skills that took us 3,000 years to develop. It's not because our genetics is that much better, but there is a super intelligence beyond the individual human unit, and that's a large part of where the skills are coming from. Plus, even things like common language. Language is a technology, just like AI. The fact that we all speak a mutually intelligible language is hugely important. We cannot do math without it.
Awesome, thanks, Daniel. Okay, last but not least, another member of our technical staff, Shimon Sidor.
Hello. Yeah, so my question is, could you give an example of a math problem such that you assign around 5% probability that it will be solved by LLMs next year? Like the type of problem, it doesn't have to be a specific problem. There are sort of degenerate cases. If you want to modify two 1,000-digit numbers.
This is a problem that humans can't do, barely, okay, but even just plain old-fashioned calculators can do. So, I mean, you can cook up artificial problems that LLM could do.
I mean, I think we may have to start inventing new categories of problems to work on because they are particularly amenable to an AI-assisted paradigm, and we don't really know what types of problems these are yet. I mean, one parallel I can give is that about 10 years ago, I started getting interested in crowdsourced mathematics, so where you post a problem on the internet and you collect contributions from many different people at various levels of mathematics.
And initially, these projects are called polymath projects. So initially, there was sort of this dream that this would be a new paradigm and it could solve huge unsolved problems in mathematics. What we found was that there were certain specific types of problems that were amenable to a crowdsourced approach, but a very small fraction. Problems that you could break up into lots of modular pieces, where each piece did not require too much expertise, so different communities could work on different pieces, and ones for which there was some sort of overall good metrical progress, like sort of score function that kept going down. There's a couple of other features like that. So there was a certain narrow sort of criteria. And there were a few successes, you know. So there was one annals paper that was a polymath project, the very first one. It did actually come up with a new type of proof of an important theorem. But it didn't scale very well. One thing we also realized was that the human moderation needed to run these projects was quite intensive.
Maybe one day there'll be crowdsourced AI-assisted projects that maybe, you know, also AI-motivated to manage the chaos. But these are things that we could only work out after we tried quite a few of these projects, and then we drew conclusions. So I think we're only going to ask these questions empirically. I think we can speculate, but pretty much all speculations would be wrong.
Thank you, Simone. Okay, I think that was perfect timing. I want to say sincerely, Terry, thank you so much for joining us. You really worked hard tonight. You've been talking for an hour and a half, answering all of our questions. There were more than 100 OpenAI staff members that showed up tonight. And I really think that's testament to the way that you said you appreciate being a teacher of math. I think there are a lot of people here, whether informally or formally, have been motivated by your teaching. So thank you so much for spending your time here with us. And Mark, thank you for being an amazing facilitator of the conversation. Ilya, Daniel, Jakob, thank you so much, guys, for joining us.
And before we leave, I just want to tell everybody a little bit about what's coming up. We only have two more events in the OpenAI Forum to take us through to the end of 2023. Next week, we'll be switching gears a bit, and we're going to have Professor Dr. Ahmed Elgamal from Rutgers. He's going to take us on a journey of the history of AIR from the uncanny valley to prompting gains and losses. I hope you guys can join us for that.
And then the final event of 2023, we'll close out with another take on the future of work with Chief Economist from LinkedIn, Karen Kimbrough. And she'll present her findings reflected in the report, Preparing for the Workforce for Generative AI Insights and Implications. So all of us, we're going to be able to re-watch this on demand and share it with anybody in our community. If any of you have referrals that you'd like to add to the community so that they can watch this video on replay, and same to you, Terry, if there's ever anybody, any of your students that are interested in having a seat at the table with OpenAI and contributing to our conversations, I'm happy to invite any of your students or your peers. And I hope to see you guys all really soon. Again, Terry, thank you so much for joining us tonight. I hope everybody has a beautiful evening and I will see you all again soon, I hope. Okay. It's a pleasure. Good night, everybody. Good night.
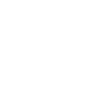