The Future of Math with o1 Reasoning
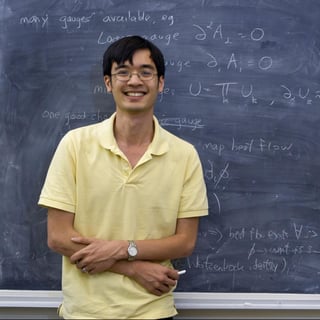
Terence Tao is a professor of Mathematics at UCLA; his areas of research include harmonic analysis, PDE, combinatorics, and number theory. He has received a number of awards, including the Fields Medal in 2006. Since 2021, Tao also serves on the President's Council of Advisors on Science and Technology.
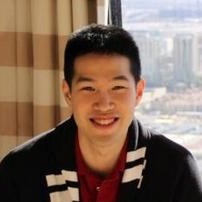
Mark Chen is the Senior Vice President of Research at OpenAI, where he oversees advanced AI initiatives, driving innovation in language models, reinforcement learning, multimodal models, and AI alignment. Since joining in 2018, he has played a key role in shaping the organization's most ambitious projects. Mark is dedicated to ensuring AI developments benefit society while maintaining a focus on responsible research.
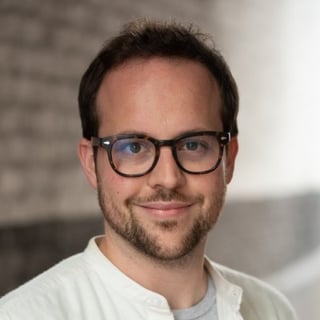
James Donovan leads Science Policy and Partnerships in GA, focusing on how our models can best be used to accelerate scientific research & commercialization. He came to OpenAI having been a founder, VC investor and Partner at Convergent Research; where he helped launch multiple moonshot science organizations including the LEAN FRO (an automated theorem prover for complex mathematics).
During the virtual event on December 3rd, Prof. Terence Tao and OpenAI's Mark Chen and James Donovan engaged in a deep discussion on the intersection of AI and mathematics. They explored how AI models, particularly new reasoning models, could enhance traditional mathematical problem-solving and potentially transform mathematical research. The speakers discussed the integration of AI into various scientific fields, emphasizing AI's role in accelerating discovery and innovation. Key topics included the challenges of AI in understanding and contributing to complex mathematical proofs, the evolving nature of mathematical research with AI integration, and the future of collaboration between AI and human mathematicians. The conversation highlighted both the potential and the current limitations of AI in advancing mathematical sciences.
I'm Natalie Cone, your OpenAI Forum Community Architect. I like to begin all of our talks by reminding us of OpenAI's mission, which is to ensure that artificial general intelligence benefits all of humanity. To conclude our speaker series for the year, we're hosting one of our favorite all-time guests, Professor Terence Tao, and two of my very inspiring colleagues at OpenAI, Mark Chen and James Donovan.
Terence Tao is a professor of mathematics at UCLA. His areas of research include harmonic analysis, PDE, combinatorics, and number theory. He's received a number of awards, including the Fields Medal in 2006. Since 2021, Tao also serves on the President's Council of Advisors on Science and Technology.
Mark Chen is the Senior Vice President of Research at OpenAI, where he oversees advanced AI initiatives, driving innovation in language models, reinforcement learning, multimodal models, and AI alignment. Since joining in 2018, he has played a key role in shaping the organization's most ambitious projects. Mark is dedicated to ensuring AI developments benefit society while maintaining a focus on responsible research.
Finally, James Donovan leads science policy and partnerships in global affairs, focusing on how our models can be best used to accelerate scientific research and commercialization. He came to OpenAI having been a founder, VC investor, and partner at Convergent Research, where he helped launch multiple moonshot science organizations, including LeanFro, an automated theorem prover for complex mathematics.
Please help me welcome our special guest to the OpenAI Forum.
Hey, fantastic. Thank you so much, Nassi. I really appreciate the introduction. The mic is yours, James. Thank you so much. What an honor to be here with such great minds tonight. Before we get going, I just want to give a big thank you to Nassi and team for organizing all of this. It's no easy thing to get so many people together and run it as smoothly as she always does. It's a great honor for me specifically to be here to talk to you both. So thank you for finding the time. And just as a sort of general note, though this is the conclusion to one year's forum events, it is the beginning as always of the next year running, where we'll have a theme focusing on science and how our models intersect and accelerate science, hopefully safely and equitably for the wider world.
So to get going both, I wanted to start just by getting a sense of maybe first Tara and then you, Mark. What are the most interesting questions that you're focused on in your write-to-fills today? And why is it important that we try and solve those questions?
Okay, well, there's lots of technical math questions that I would love to solve. I think more relevantly for this meeting, I mean, I'm really interested in how we can just rework mathematics from the ground up and how we can use all these new tools to really collaborate in ways that we couldn't do before, to do mathematics at a scale we couldn't do before. I think it could be a new age of discovery. Right now, mathematicians, we work on individual problems at a time. We spend months working on one problem and then move on to the next. With these tools, we could potentially just scan hundreds or thousands of problems at once and do really different types of mathematics. So I'm really excited about that possibility. And you, Mark?
Cool, yeah. I mean, one of our big focuses over the last year has been reasoning. So since GPT-4, we've kind of shifted our focus slightly. I think GPT-4, for all intents and purposes, is a very smart model. It contains a lot of world knowledge, but it's also stupid in many ways, too. It gets tripped up by simple puzzles and oftentimes very reliant on the prior, like if it has some kind of prior knowledge of how a puzzle should shape out, it often kind of makes that same kind of pattern-matching mistake. I think these pointed to us really to deficiency in the model's ability to deeply reason. And so we've been focused on developing what we now see as the O series of models. So these are models that are more like system two thinkers than system one thinkers. I think they less often kind of give the intuitive fast response and spend some time kind of reflecting on the problem before producing a response. I think just to highlight two other problems that are key to our research agenda, data efficiency is certainly one of them. I think we care about how to ingest all of the data in the world, including non-text data. And third is a very practical problem, just how do we create intuitive, delightful experiences for our users?
Yes, it's true. I mean, that last problem is maybe a little beyond the world of maths specifically, but is a critical one, that kind of human computer interface question there. I do, Terry, want to ask you specifically about the O1 models as Mark has outlined them. But before I do, you just mentioned a potentially new type of maths. At various times, you've spoken about maths at industrial scale. You've also talked about different ways of cooperating in maths. Would you mind unpacking that for us a little bit?
Sure. So maths has always been perceived as a really difficult activity. And it currently is for many reasons. But one of which is that it's relying on one human or maybe a small number of humans to do a lot of different tasks to achieve a complex goal. If you want to make progress in mathematics, you have to first come up with a good question, and then you have to find the tools to solve it. And then you have to learn the literature. You have to try some arguments. You have to do the computations. You have to check the arguments, make sure it's correct. And then you have to write it up in a way that can be explained. And then you have to give talks, and you have to apply for grants. And there's lots of other different things you have to do. And these are all kind of different skills. But in other industries, we have division of labor. You don't rely on one, like if you're making a movie, you don't have one person produce the movie, edit the movie, act in the movie, and get financing for the movie, and so forth. You have different roles. But we've not found a way to decouple all these tasks in mathematics until recently. I think now that we have these tools, in principle, you could have a collaboration where one person has the vision, one person, or maybe an AI does the computations, and then another tool writes the paper, and so forth. And so you don't need one person to be expert in all aspects. So I think a lot of people are discouraged from doing mathematics because they look at all the different things, a checklist of things they have to do to be a good mathematician, and it's really daunting. But maybe there are people who are good at looking at data and inspecting patterns, and then
asking an AI to check, you know, can you confirm if this pattern exists? You know, or maybe they're not very good at finding the right questions to ask, but they can work on some very narrow, specific piece of a larger project. So I think these tools are, the job of doing mathematics to be decoupled in many, to be made more modular. And then so some tasks be done by AI, some tasks by humans, some tasks by maybe like formal proof assistants, some tasks by the general public. We have, you know, we have big science, we have citizen science in other disciplines. You know, we have, you know, the amateur astronomers who discover comets or amateur biologists who collect butterflies. And we don't really have a way of utilizing amateur mathematicians outside of some very small sort of fringe projects. So there's a lot of potential and I think we have to throw a lot of things on the wall and see what sticks. Terry, I have a quick follow-up question for you. I'm curious, like AI aside, like what the maximum number of humans up to date, like that have been kind of able to collaboratively work on a single math, you know, project or yeah. Do you think there's like an upper limit here?
You know what? Right. So in practice, the limit is around five or six. It's really hard past that point. You have to check each other's work and also just that getting everyone in the same room and so forth. There are a small number of projects which have many authors, for example, proof formalization projects where a big group gets formalized. That's a task which already that's one of the few tasks in mathematics that we already do know how to crowdsource and split because you know, you run it all on GitHub or something and all the contributions are verified because they're in this formal language such as Lean. And so these can have 20, 30 authors. Lean has this thing called Mathlib, it's a library of all undergraduate mathematics. It's never been officially a research project, but I think technically it has like thousands or at least hundreds. So yeah, but it's only really in the formal mathematics that we're really getting to see the large collaboration so far.
Fantastic. And I do want to echo that shout out to Lean. They've done some really incredible work and I think we might have a few members of the Lean team on the call today. As you were unpacking that, Terry, it sounded like your default assumption was that humans will still divvy up tasks. They'll still understand enough about the process to decide who's doing what where. My first question for you would be, do you think, therefore, there'll be different roles that emerge for mathematicians, different specialties that they adapt? And I'd flip it over to you, Mark, to say whether you think that's likely to always be humans or you see a world in which O1 itself is breaking down problems or the O class.
So I see software engineering as kind of a template for where math might go. So in the past, maybe there was one heroic programmer who did everything the same way that mathematicians sort of do everything now. But now you have project managers and programmers and quality assurance teams and so forth. And so one can imagine doing that right now. So I'm involved right now in several projects which are collaborative and they involve both a theoretical math component and a formal proof of component. And people also are running various code algorithms and so forth. And it's already specializing the way that I was expecting. So there's some people who don't know the math, but they're very good at formalizing theorems. It's almost like solving puzzles to them. And then the ones that are good at running GitHub and doing all the project management and just making sure that all the back end runs smoothly. And then there's people who do data visualization and so forth. And we know we all coordinate. So far, it's been mostly humans and sort of more old-fashioned AI-type theorem improvers and often just running Python code or something. But I think it's a paradigm in which AI will slot very, very nicely in once it gets good enough.
Yeah, no, that makes a lot of sense to me, too. I feel like, you know, today I almost kind of treat AI as a co-worker in many respects. There are things that I don't do very well that I can farm off to an AI. I'm only kind of conjecturing here because I'm not a mathematician. But in terms of where AIs might be strong in in helping to solve mathematical problems could be first just like recognizing patterns, right? Machines are fairly good at this, especially if there's a lot of kind of data or just a lot of stuff to sift through. And then I think from identifying patterns, you can start to form conjectures. And I think they might have a unique strength in doing that. Again, coming up with proof strategies, I think this is something we talked about last time. I think humans today still probably have a better intuition for what the right steps forward are. But maybe you have a blind spot when it comes to one particular step. And I think last time we mentioned there's some generating function approach that a model suggested in one of the kind of problems you're trying to solve. And that actually turned out to be not a terrible idea in that situation. Also, maybe like just like verification, models might be able to kind of verify certain steps that you're pretty sure are right, but you just want to get another another pair of eyes on. And maybe also kind of like generating counterexamples, too. Like, I think if there's something where you just want to think of a lot of potential ways that a theorem could be false or something, a model may be able to exhaust that a lot more efficiently.
That makes a great deal of sense. You both mentioned in your answers the role of theorem provers and formalization more broadly. Is it fair to say that you both think that that is a necessary intermediary layer between doing the maths and using LLMs or equivalent technologies? Largely, yes. I mean, the proof has to be correct. And I think what math proves is that if you have 100 steps in the proof and one of them is wrong, then the whole group can fall apart. And AI, of course, it makes all these mistakes. There are types of mathematics where a positive failure rate is acceptable. Like Mark said, like finding patterns, finding conjectures, it's OK to have an AI that is only correct 99% of the time, if you have some other way to check it. And in particular, if it tries to output an argument, it's a very natural synergy to force the AI to output in something like Lean. And then if it compiles, great. If not, it sends back an error message and it updates its answer. People have already implemented this and they can prove kind of maybe short proofs on the level of an undergraduate homework assignment can be sort of done by this iterative technique. Definitely not at the point where you can just ask it a high-level math question and it will output a huge proof. I mean, alpha proof can do it with three days of compute, but it doesn't scale. Yeah, for some soft things where a positive error rate is acceptable, you won't need the formal proof assistance. But anything really complex where one mistake can propagate, it's basically indispensable. I think at OpenAI, you know, in various times in our history, we've focused more or less on formalized mathematics. And I think today we do a little bit less, I think, primarily just because we want to explore reasoning in full generality. And we do hope that kind of reasoning that you learn in fields like computer science are fairly similar to reasoning that you learn in fields like math. So, yeah, definitely understand the advantages, though, of doing formal mathematics.
I'd quite like to come back to that architecture of the theorem proof in math's AI and see whether or not that's true for other domains of science as well. But one question I have before that is that even in the training process, there's probably a lot of incorrect ways of solving things that don't get into the training models, because mathematicians on the whole don't publish incorrect things. And that's true for science more broadly. Do you both think that would make a big difference as to whether or not it's true for other domains of science?
Is that a sort of cultural norm that we should be trying to push, that people do publish failed answers? Separately from AI, I think that's a good idea. It is hard to encourage that. I mean, people don't like to admit their mistakes. But yeah, this could really be precious training data for AIs.
When I teach my classes, sometimes the classes I give that are most effective, accidentally, when I prepared a proof and I give it in class and I screw up, the proof does not work. And I have to fix it in real time. And the class sees me try various things. Oh, okay, what if I change this hypothesis, might try to work out this example. And I've gotten feedback later that those were the most valuable classes that I ever taught. And it was because I made mistakes. And this is data that largely, I think, people like you, you just don't have access to. I mean, this is, I think, in fact, many experts in a domain have the expertise built on decades of mistakes that taught them what not to do, the negative space.
There's beginning to be, I think, as we move to a more formal environment, like, so right now we are formalizing sort of the proofs after they're done. We'll eventually get to the point where we will formalize as we go. We will maybe converse with an AI while we think about math, and it will try to formalize the steps as we go. And then maybe it doesn't work, and you get a backtrack and so forth. And that will sort of naturally create some of this data that we don't have right now.
Out of interest, a lot of mathematicians talk to the beauty of a theorem and the kind of eureka moment when everything fits together and can be expressed elegantly. Is there a chance we lose that kind of cognitive process by using tools like these?
I think, well, a similar situation came up when calculators became ubiquitous, right? People said, you know, now that you don't have to do everything by hand, you lose your number sense. And you know, to some extent, this is true. You know, I would imagine that a mathematician from 50, 100 years ago is much better with getting number sense from direct calculations. But you also get a different type of number sense from just playing the calculator. And so I think there'll be a different type of beauty standard. I think there will be some computer-generated proofs that are also really, really elegant and amazing in a different way. But I think, well, I don't think the AI paradigm will take over completely for many decades. I think mathematicians are somewhat slow to, you know, we still use chalk and blackboard, you can see on them, I know. So there'll be people who will still craft, you know, really wonderful proofs, you know. I think there'll be a class of mathematicians who will take AI-generated mathematics and convert it into something much more human. I think that will be a common thing to do in the future.
Out of interest, Mark, when you hear an answer like that from Terry, do you put a lot of thought into thinking not just how do you make reasoning high quality, so accurate, but also how a human can work with the kind of outputs and that side of the equation too?
Yeah, yeah. So I mean, I think when you think about RL, right, it's like also just kind of incentivizing the model and having the model learn from its mistakes. So that like highly resonated with me. Yeah, and I do think that's how you develop, you know, robust and strong reasoning skills, right? You can't really just kind of be shown a lot of examples of accurate reasoning because there's so much negative space in mathematical reasoning.
I think, I do think, you know, models will become helpful, much more useful. Like I'm quite an optimist on this. And in terms of kind of like the impacts, right? Yeah, it's really kind of interesting to hear about, you know, not so much that like people will lose a sense of like aesthetics or intuition, but maybe like kind of develop new abstraction layers and kind of new abstractions and intuitions will form out of that. And yeah, that seems interesting and quite likely as well. So yeah, that'll be cool to see, and especially if it happens fairly soon.
Yeah, it's a really interesting line to follow, just in the sense of, I think in my own world, biology, the assumption tends to be these models will find patterns across things that are otherwise seen as unrelated, and you'll find all these underlying unity across things. But that's kind of on the idea, there's lots of low hanging fruit, we just haven't noticed. Whereas I think for things like maths and parts of physics, the refinement is almost in the way that that activity is done. And that we feel like that might be fundamentally different.
I wonder, Terry and Mark too, whether you think it will have an implication how we educate people in maths, and in particular, support people who are going to do frontier maths research?
Well, yeah, no, I mean, it's, of course, students are already using, you know, large language models to, you know, most obviously, help them do homework. But you know, also get a second perspective on the topic. And so actually, you know, we're also figuring out how to integrate large language models into our teaching. So like, one thing that's become increasingly common is to present some math problem or some other field, give, you know, GPT's answer to it and say, this answer is wrong, please critique it, or, you know, or teach or have a conversation with the AI and I can teach it to actually fix the answer. There's actually one class where they made a group project, where the teacher handed out a practice final for the class. And they said, okay, try to train AI with prompt engineering and data analysis to actually figure out and generating synthetic versions of the final exam, how to most efficiently, you know, teach an AI to solve the final and they did, you know, so they had one group to do prompts and one group to do, you know, to do benchmarking and so forth. Sorry, I lost my light. But no, but it also forced them, you know, for example, to generate all the data for like, to generate synthetic exams, they really had to understand the class material to do it. So it was actually kind of an excuse to really delve deep and learn both the class material and how to use these AI tools. So we'll find innovative ways to combine these two.
Yeah, I guess, kind of some people, they point to, you know, fears, right? It's like, if you have too much of a dependence on AI systems, right, like, do your skills erode or kind of, do you have like, less insight? I'm actually very curious for Terry's take on this. But yeah, I think while he's figuring out his light, maybe I can, okay. It did give a very dramatic answer, Terry. So I quite enjoyed the lone genius in the dark, propping it out of the cave.
Yeah, well, sorry, what was your question again? Yeah, just like, do you think there's any truth to like, reliance of, on AI tools kind of leading to maybe like, less, less kind of skill in general in mathematics, or maybe loss of insight or something like that?
Well, it will be a transfer, I think we will use some skills less often, but we will develop other skills more often. So there's an analogy of chess. So chess is now essentially a solved problem. But people still play chess quite a lot. But the way they practice in chess is quite different now, that they experiment with different moves, and then they ask a chess engine, you know, is this a good move or not? And so for example, the theory of chess theory is flourishing, there are lots of century old maxims about what, you know, what part of the chessboard is good control and so forth that are actually being re-evaluated. Now, with humans asking chess engines various questions, and that is a different way.
of getting intuition about chess rather than sort of the standard sort of just play lots of games and read lots of textbooks and so forth. So yeah, it's, you know, it will be a shift, you know, it's a trade-off, but I think a net positive.
Yeah, I think when, yeah, people ask me also about just like, you know, what, how should they be adapting, right, to AIHFL's coming out. I still think like, largely like there, there's no need to suddenly like abandon studying any particular subject, right. I think really people should be kind of embracing AI and just seeing how it can make them more efficient, like in math specifically, right, like it could help you with a lot of tedious computations, you know, if it's some kind of routine thing that you already know kind of inside out and you can just have the the model kind of carry out the algebraic manipulations or something like that. I still think there's just a lot of alpha and just very deep understanding of a subject.
Yeah, even in machine learning today, right, the people who are affecting the biggest change are the people who just very deeply understand the math or like the, you know, the systems, right, and I think that that will continue to, you know, be a very big lever.
Also just like focusing on abstractions, I think like humans do have a particular aesthetic that's just tied to the core of mathematics. And I think because, you know, other humans are judging that aesthetic, like, you know, models may have a more difficult time kind of emulating that when it comes to, you know, defining the problem and having taste.
Yeah, and of course, you know, math is just like, it's a good skill to have. I think it's like very transferable. It teaches you kind of robust reasoning and and I think people who are mathematicians are just very adaptable in general. So definitely no reason not to kind of invest heavily in math.
It's an interesting point, actually, Mark, when you talk about the aesthetic of maths. We're getting a little abstract, but it is possible that the way that we conceive of maths is somehow tied to the way that we experience reality as humans. And that if you had models doing very sophisticated maths, you might get to a point where it is exceeding the ability of humans to verify or even make sense of it in our context. Do either of you see that as a possible future anytime soon? And if so, how would you react to that?
Well, I mean, actually, it's already the case that mathematicians sometimes produce enormous proofs that no one person understands. We already use a lot of computer assistants. There are some proofs that require, you know, that have like terabyte long proof certificates, because there's a massive SAT solver calculation or some big numerical modeling or something.
And then there's also proofs that are built upon like a tower of hundreds of papers in the literature. And we're taking these previous results as black boxes, and no one person understands everything. So we're already to some extent used to this. And we can cope because we have this language of abstraction, you know, and we can sort of compartmentalize a complex proof. And you just need to understand one piece, and you just trust that either a computer or human understands the other pieces, and it all works out. So this will keep happening with this. So we will have big, complicated arguments where part of it is going to be AI generated, hopefully formally verified too. So, I mean, it's a trend. It's just accelerating a trend that's already been happening. I don't see it as a real phase change.
Yeah, yeah, I think, yeah, a lot of the worries I have are similar, just like you could have some error that, you know, propagates or other people build on top of some result. And you're just kind of building on some faulty mathematics, right, especially if the volume of kind of new computer generated insights increases.
I mean, one thing that we worry about a lot at OpenAI is this more general problem of scalable oversight. And the idea is just kind of like, when a model spends a lot of time, let's say like thinking or, you know, and it comes up with some kind of like, you know, fundamental insight that's, you know, it's thought a lot about to arrive at. How do you know that, you know, the model didn't make a mistake? How do you know it's right? How do you trust it? And, yeah, fundamentally, it's just like a very real problem that, you know, felt fairly theoretical maybe a couple years back. But I think today, you know, models do have that capability to solve very hard problems. And so, you know, how do we vet and trust that the problem came up with the correct answer?
Well, math is the one place where we have a shot because if we have the formal verification, that can also be done in an automated way.
No, indeed. And you would hope that progress there unlocks progress across all the other sciences, ultimately. Right. We can find a way to derive from those mathematical proofs down into physics, chemistry, and so on.
Terry, there are quite a few people in the room today who are working practically in math for students or otherwise. So I have a few very practical questions. Maybe not a phase change using AI or AI-related tools, but there are some cultural elements of math practically that might change. Some of the unique things are math competitions. And I know you're in Bristol not long ago. I mean, back to that theme. Do you see, like, the actual ecosystem of math changing to accommodate LMs? And if so, how?
It will. It will. It's hard to predict exactly how it will. I think there'll be new types of mathematics that are not popular now because they're just technically infeasible. So in particular, experimental mathematics is a very, very small segment. I think it's like 95% theoretical, which is unusual among all the sciences. In sciences, usually there's a balance between experiment and theory. But experiments are hard. You'd have to be really good at programming. And your task has to be sort of simple enough that you can automate it with a regular piece of software, which is within the skill of a mathematician or program. But with AI, you could do much more sophisticated explorations. So traditionally, you might study one differential equation, but you might ask an AI, you know, here's an analysis of this differential equation. Now repeat the same analysis for the next 500 equations on this list. And this is something that you can't really automate right now with traditional tools, because you need the software to do some understanding of what the problem is. So I think the type of mathematics will change. I think there's already a trend to become more collaborative, and that will just accelerate with AI. But I think, at least for the next decade or two, we'll still, you know, be writing papers and refereeing and doing and teaching and so forth. I think it won't be a major change. It's just that we will use more and more AI in our work, just like we're already using more and more computer assistants in our work in other ways.
Yeah, and I think, yeah, just a point on the competitions. I think I can speak more to programming competitions, but I don't know if they would fundamentally change too much. I think, at least most people I know who kind of do that a lot, you know, it's just very fun to do, I think, kind of even beyond kind of, you know, the technical skills that you gain.
Because cheating will become a problem. That's maybe the one trick.
Yeah, yeah, exactly. Yeah. I mean, I think that's also like just a very deep question, right? It's like, even like, how do you interview people when, you know, the models can solve very, very difficult problems? So, yeah, but I do think, you know, contests, a big part of the reason people do it is because it's just fun. And I think, you know, the analogy to chess is a good one.
Yes. So cheating is definitely one element of this. But I guess the less deliberate or trying to break the rules elements is maybe attribution. You know, what happens in a world in which we have potentially large parts of formalization being done by LLMs or even novel ideas emerging from LLMs because of a combinatorial approach? Can you both envision a world in which we are attributing breakthroughs directly to LLMs themselves? And what might that mean?
Yeah, this is going to be a big issue that we have to face. I think it's it's a very
the authorship model that we have of papers where there's like, you know, so in the sciences, we have maybe one lead author and then a whole bunch of secondary authors. And so mathematicians, we don't do that yet. We still like order alphabetically by last name. And we haven't really, we have largely sort of ignored the question of who did what. And we just say, oh, we all contribute equally. I think we're going to have to be more precise about attribution. And papers in the future.
So there's already a trend where, at least in the sciences, where you write a paper, there's some section on author contributions, you know, who did what. And if it's a GitHub, you can look at the GitHub commits. And this also gives you some data. And then maybe there will be some way to automatically, you know, inspect all that data and somehow summarize who did what.
Yeah, so once, you know, half the commits are done by an AI and so forth.
Yeah, there was a question like, do you actually promote the AI to a co-author? Or do you at least put in acknowledgments? We don't have the norms for this yet. We'll have to work it out. There'll be some test cases and some controversies. And we'll eventually work out something that works for everybody. But yeah, I don't have the answers for that one.
Yeah, I do think there's also this related, not exactly the same style of issue of just kind of access. I think if just, you know, models continue to contribute large chunks of proofs, like are the people who have more access to compute or like, you know, are they in an advantageous position when it comes to doing mathematics? Yeah, definitely something to kind of think through. And yeah, I don't quite know how to like follow that train of thought quite yet. But yeah, yes, it's definitely a hard problem.
Yeah, it's going to be interesting to see. I mean, you already get maybe more on the creative side of the world, questions about attribution and ownership. But it'll be interesting as it gets more and more involved in science about intellectual property and how we think about the R&D cycle in such a world. On that kind of topic of applied use of math or science more broadly. For those who are not in themselves mathematicians, we've spoken a lot about the act of math changing and why that's important. If we were, and ignoring the mechanism for how to achieve that, if we were to get to a place where foundational math was being meaningfully accelerated, what would you expect to see happening in the world? What does that unlock for the rest of society?
Well, I think it could increase citizen participation in mathematics. One could imagine, for example, people debate about, for example, is the earth round or flat? It's amazing how this is still debatable. But with an AI, you could actually start constructing models and you can say, okay, suppose the earth is flat, what would the sky look like and so forth? And right now, you need quite a bit of math before you can figure out how much things would change. But you can imagine with these models, it could actually just create a visualizer for you and you can see, oh, this is what this theory of the universe would look like. And so, I mean, I think it would make, it could really connect mathematics to a lot of people who are currently feel excluded from it because of just the sheer technical skill needed to do anything in the subject.
Do you think it is a prerequisite that we get better at doing this kind of maths in order to use AI in other applied scientific applications? Is it a prerequisite for accelerating engineering or physics? And a question for you as well, Mark, whether you see that as a necessary first step?
Well, I mean, so much of the science is already math based. If you don't understand the math, you can't model accurately without the math. And yes, certainly on the back end, I mean, if you want to train the AI and so forth, you need lots of math for that. I mean, it's possible that we could be into a world where you could be, you know, a biologist or whatever, and you could ask an AI to run a statistical study or something, and you don't need to know the fine details of exactly what the parameters are. And if the AI is reliable enough, it could actually, you know, do all the math for you. And so, you know, it could make the math optional to do science in a way that it isn't right now. So it could work both ways.
Yeah, I mean, I think I trust Terry the most on the implications of, you know, like having accelerated math progress and what that means. I think really as a researcher and just speaking on behalf of a lot of the researchers here, I think the most exciting applications of our models are when they're used to accelerate science. I think really like, you know, trying to provide this kind of very general purpose tool that, you know, experts can be, can use in their daily lives to just accelerate their work. Yeah, I think across other sciences, right? Like we've seen, you know, people in material science, people in healthcare kind of already use the reasoning models and have testimonials to the fact of like, hey, you know, this is almost like, you know, some undergrad that I can kind of give tasks to, and they can come with fairly coherent analyses of certain situations or, you know, kind of like Terry said, like a lot of people will be like, hey, you know, here's a scenario, right? Like, can you kind of do some calculations? And like, what would the implications of the scenario look like? And I think people have found it fairly effective in those situations.
No, absolutely. I mean, I suppose where my mind is going is that very rapidly, you hit a world in which a very small number of people could actually validate whether or not the answers you're being given are correct. And perhaps the structure of theorem proving plus an ever more sophisticated LM in math is the only way if you actually get a scalable verification solution to that problem. And so in a way, we always have to have full mathematics at the top and then everything else is derived from it. And given that that's a potential future, and some of the other things we've spoken about, Terry, do you have advice for young mathematicians on where they should be focusing and the kind of questions they should be tackling?
I think, yeah, it's my main advice is that you have to be flexible. I think mathematics is becoming more technology infused and more collaborative. And maybe 50 years ago, you could specialize in one sub-sub-subfield of mathematics and barely even interact with other mathematicians and you could make a living out of that. And that's basically not so feasible now. I think math is part of a much larger ecosystem, which is a healthy thing. And with AI, it unlocks much broader collaborations than previously thought possible. You could collaborate with scientists in a domain in which you really have no expertise, but the AI can help you get up to speed at a basic level and serve as sort of a universal translator between scientists. So, yeah, just be open-minded and also recognize that these tools also have limitations and you can't just sort of blindly use these tools. I mean, you still have to build up your own human skills so that you can supervise the AI. Yeah, it isn't a magic wand.
Yeah, so I don't think even we of OpenAI would be encouraging folks to use it without quite a heavy bit of expertise and oversights.
Maybe a similar question for you, Mark, but slightly barer, which is just based on the trajectory that you're seeing, what skills would you encourage students to be picking up now to be able to make the most of these models over time?
Yeah, yeah. I mean, honestly, like technical fields, we still need technical experts in technical fields to essentially who can kind of synergize with the tools very well. I love the idea, just the general advice to stay flexible, right? And to show kind of like AI research a little bit, I think it'd be very helpful for people in just a variety of fields to at least kind of understand the basics of how neural nets work, how they're trained, what their dynamics are like, and just like what their limitations are, right, as an implication. So I think just the more that people kind of play around with it and just understand how it can accelerate them, I think the more effective they will be. I do think there will be
multiplier on everyone's efficiency, maybe a couple of years down the line, right? And that multiple hopefully will be like, you know, significantly greater than one, but I do think people who effectively leverage AI tools will be by and large more effective than people who are just kind of blind to it.
Yeah, that certainly resonates. I wonder if the key question has become less, will they be useful and more, the speed of their evolution. Some ways, Terry, you've been on the inside watching as these models get better at different moments in time. And I do hear, you know, recently the performance on IMO and silver level, accepting that there was a little bit of sharing going on to make that happen. Have you been surprised at the rate of progress?
Yeah, it's been sort of both exceeding and also probably under my expectations. Yeah, so it seems like in any task which you can generate data of similar tasks. So, you know, for example, the IMO thing, DeepMind generate a lot of synthetic proofs, actually a lot of synthetic failed proofs. That was actually part of the secret. So a lot of tasks which I thought would not have been doable for several years are now done. On the other hand, every time you sort of go beyond the sphere of where there's data, and like you go into a research level problem where there's like only 10 people in the world have really thought hard about this question. And it's still, you know, the AI tools are still not being so useful. So I have this project I'm still running right now where instead of proving one big problem, we're proving like 20 million small mathematical questions. And I thought this was a task in which AI would be ideal for, you know, because they could handle some percentage. But it turned out that, you know, all these questions that this project studied, you know, maybe 99% could be handled by kind of more traditional computational brute force methods. And of this 1%, which was quite hard and quite human intervention, the AI tools that have been tried, they could recover much of the 99% of the fairly easy problems, but they didn't really contribute to the hard core of the really challenging questions. So that could just be the nature of the state of technology today. So yeah, I don't see the, there would have to be quite a few more breakthroughs, I think, before you see them sort of autonomously solving these research level questions.
Yeah, I think to speak to one anecdote in my mind that speaks to this kind of like, you know, impressive and at the same time, you know, room to go kind of angle. I think, you know, we participated in the IOI this year as well with our own models. And I think on one hand, you know, it's like, it did take them a lot of samples per problem. I think like we announced in our blog post, like you need 10,000 samples per problem to extract kind of gold medal level performance from the model, which feels like a lot, but at the same time, it's like just incredible to me that it can do this at all. And, you know, some of these are very like anti-pattern style problems. And so it's like somewhere in there. And I think I'm just really excited by kind of really getting that capability out.
Yeah, it's funny that it always feels a little intellectually unsatisfying when you feel like you almost cheated in a way because you've reconstructed the problem. But then I zoom out and I wonder how much of scientific progress is just lots of that stacked together, and then it creates a paradigm shift that in retrospect seems very clever, but was actually just little things together. To some degree, you know, the joy of programming is exactly that when you redefine a problem such that it can be solved, but it's necessarily first principles working your way through. It does raise the question for me though, which is maybe what we're talking about here is that we're teaching the models to reason in a specific way. And that category of reasoning works well for some type of problem. Do we think that, and maybe starting with you, Mark, and then onto you, Terry, do you envision a world in which one class of models does lots of different types of reasoning simultaneously, or is it more likely to be a world in which you have sort of individual models doing different types of reasoning that comes together? And then for you, Terry, what kinds of reasoning would you need to see to think that you could unlock using AI some of the more challenging, the smaller subset of questions that currently they struggle with?
Yeah, I mean, I do think there's beauty in just having one model that can kind of reason across a bunch of different domains. I think when you try to hook up a lot of complicated systems like you make a lot of design choices, and I think simplicity is really one of the key mantras in AI development. I do think like, yeah, you could set up structures, of course, of AIs that collaborate in a certain way and that's also very exciting, right? Like, could we build out this model of, like, you're a specialist here and you're the PM of this math project and you're the proof writer and you're checking like the 10,000 cases or something like that.
Yeah, I think like that's also a very interesting paradigm to explore.
Right, I mean, I definitely see AI problem solving as a very complimentary way, it's a very data-driven way of solving problems. And as you said, for certain tasks, it's actually much better than humans. A lot of perceived, what we're learning actually is that our perception of difficulty of certain tasks has had to be recalibrated because we just didn't try to use a data-driven approach to solve certain kinds of problems. But some problems are genuinely hard without, I think, I mean, in math, there are even questions that are undecidable, that no amount of data can actually solve certain problems. We can actually prove that they can't be proved. But yeah, so I think, I mean, this is not really the AI strength, but if you want an AI to really compete on solving math problems the way that humans would, they would need to reason in data-scarce environments where there's a new mathematical object that you're studying and you know five or six facts about it, you know a small number of examples. Maybe there's a very vague analogy with some other mathematical object that's already out there. And you have to just extrapolate from a very small amount of data what to do next. And this is something that AIs don't excel at. And maybe, and it's entirely the wrong, I mean, I think trying to force AIs to do that is like it's using the wrong tool to achieve a task. I mean, this is something that humans are actually really good and efficient at. It's all the brute force checking and case analysis and synthesizing, you know, finding the patterns that they're not so good at. So I don't know, it may be a mistake to think of intelligence as a sort of one-dimensional scale and which one's better, AI or humans. I think they really, you should think of it as complementary.
Yeah, I do hope if we're successful in our research program that we'll have very data-efficient reasoners too. So hopefully we can prove you wrong, Terry.
Yeah, okay. I saw Glenn's and Mark's eyes as you were talking. I can see Mitch's to jump in there. Okay, well, I would love to be proven wrong.
Yeah.
We're coming up to the end of our time both. So maybe to end this as a way of tying this all together, if you were both, you know, tomorrow appointed to be vice chancellor of a university, given some meaningful budget, what would you set up to make an effective, in your case, Terry, math department, in your case, Mark, maybe broader science department? And what infrastructure would you be investing in to really take advantage of these new technologies? That's a good question. I can imagine having some centralized computer resource to run local models that you can tune yourself and so forth. It's a little hard. I mean, the technology is changing so fast that an investment in any specific hardware or software now may not be so important in a few years.
Yeah, so certainly some...
a location where you can bring together lots of people from different disciplines and to figure out ways to use these technologies together. I mean, we're already developing, lots of universities have these sort of tech hub type things already. So yeah, but I think it has to be very free form and because the technology is so unpredictable, just, but yeah, we need the different departments to talk to each other and see where the synergies are.
Do you see a room for a sort of concerted effort around math libraries and those kind of building blocks for theorem proving or things like that?
Yeah, so I mean, there's already, yeah, there's this sort of volunteer crowdsourced efforts right now. The federal funding agencies in the US are just beginning to fund a little bit of this. So universities generally have not done this kind of fundamental infrastructure type work. Yeah, that may be a role where actually, I think government will have to play a leading role.
And for you, Mark?
Yeah, I mean, I'll just give a very short answer. I think OpenAI is doing it right. Build a very big computer, let's figure out how to turn the computer into intelligence. It's a pithy answer and one I think Sam would be proud of too, Mark, so that makes a lot of sense.
Well, guys, I just want to say thank you so much both for finding the time to talk to us today. We will be moving from this into a Q&A. So anyone who had more difficult questions for you both will get that chance to fire them away. But Terri in particular, thank you for dialing in. Thank you for giving us the time for this conversation. And with that, I'll pass back to Nathalie. Thank you so much, fellas. I'll see you in the Q&A.
So everyone, if you would like to ask Terrence, James, or Mark your questions live, please join the live notification link that just popped up, or you can go to the agenda tab on the left side of your screen and jump into the Q&A meeting room. I'll see you there in a second and we'll address all of the questions or as many as we have time for that you all dropped in the chat.
See you soon.
Eduardo, let's get the party started with you. Would you like to introduce yourself, Eduardo?
Yeah, Eduardo Sontag, I'm a mathematician by training, working now, and also doing AI about 50 years ago, literally, 52 actually. But my question for Terri, so 40 years ago, 35 or 40 years ago, I officially asked the American Math Society through Felix Browder, who was a colleague of mine at Rutgers at the time, to propose a big scale mathematics project similar to the physicists were having their supercollider at the time. And I said, let's computerize, let's form a database of basic mathematical theorems in some sort of unified language so that people would be able to refer to those things and find them easily. I was laughed out of the room. I was like, this guy's crazy, you know, crackpot. But obviously, now we're in a situation where this can begin to happen.
So my question to you, and I posed this in the question was, for me, the most frustrating thing when doing mathematical research is you're trying to prove a little lemma and you know that 100 people must have proved this, whether in algebraic geometry or, you know, community algebra, group theory, you know, PDEs, whatever. And it's so hard to find the answer, right? You end up proving it yourself. So my question to you is, do you see in the relatively near future, meaning not 20 years from now, but maybe three, four, five years from now, a capability for being able to, you know, through some kind of learning, right? And could be some sort of, you know, attention-based type thing where you recognize patterns by what is embedded and what's related to what, that would be able to really do this, right? And you know exactly what I'm talking about, right? Semantic search for math would be fantastic.
Oh and actually already does a little bit of this. I did some experiments. Like if you have a theorem that, if you have a result that you heard of, you think you know the name of, or you think you know roughly what it is, but you don't know the name. So you can't just type in a search engine. You can't describe it in informal terms to an LLM. And it can often actually say, oh, you're thinking of this particular theorem. For a more obscure result, which is buried in 20 papers on the archive somewhere, we don't have that capability right now. That is a great problem. I pose it to a lot of people who I talk to in machine learning. Is there some way to extract out the essence of a mathematical result and search for it? Right now, the best way to do it is crowdsourcing. You go to a question and answer site, like a Math Overflow.
Right, that works, yes, right.
Yeah. But I'd like a little more systematic. That's a bit of the outline now, basically.
Right, okay, thank you. Thank you, Eduardo. So good to see you.
So Lizzie, you're gonna be up next, but before we jump to your question, we're gonna let our technical producer find you and unmute you. And we're gonna give a question to Terrence and Mark from Niloy Sengupta, Chief Privacy Officer at Robinhood. Niloy asks Terrence, what's your gut feeling about hard constraints, if any, that these models currently have and will continue to have when it comes to solving previously unsolved mathematical problems?
Oh, hard constraints are remarkably few. I mean, there are a few questions that are just genuinely undecidable. And then there are ones which we know imply other questions that are hard. And we know that they're kind of immune to a lot of standard techniques. But there's always surprises. I mean, in human mathematics, every year, there's a problem which people thought was impossible, and some human came up with an ingenious new idea. So that's the beauty of math. We don't actually know what's hard. So yeah, there's very few hard constraints, I'd say.
Mark, anything from you?
Yeah, no, I largely agree with that perspective. I think hard is a very strong word. And I mean, there's certainly, I think, aspects of mathematics which are difficult for the models today. Like, just like asking the right questions, asking the right questions, having anesthetics for what abstractions to build or something like that. And yeah, I think they're much better in this kind of ask a question and try to solve it setting.
Thanks, Mark.
Lizzie, welcome to the forum. Would you like to introduce yourself?
Yes, so I am currently a medical student at Stanford on neuroscience, which is the real neural network, if you don't mind me calling. But I'm trying to apply using the LLM or the AI model that I'm still learning to apply for AI drug discovery. But I don't have questions for that because there's too many questions regarding that issue. My question is, I run into a technical issue that I live in San Francisco, and I wanted to go to the San Francisco Opera this weekend, which is the past weekend. And I type in into the chat GPT and ask them, when is Carmen on show? That was the Carmen schedule. And then chat GPT told me on Saturday, I can go. So I went there, there was no show. It was only Sunday, 2 p.m. So with this technical difficulty, then how can I trust or use the system in a more, how do you say, cautious way when doing AI drug discovery that I don't know the answer to that I cannot check? And then it will have longer impact. I'm sorry to bring this issue.
Oh, no, no, of course. It's a very fair question. And I think I'm probably the person who should answer. I think, actually, I would encourage you to try to use the models with search today. I think there's existing ways you can have the models kind of just browse and ground the model responses in our output sources. So if you use search today, it will cite particular websites or particular sources which reflect ground truth. I think future versions of this will be extremely precise. They'll tell you the locales within these websites where you can find the answer and find the reference for yourself.
Yeah, and I do think kind of future models, they will be very grounded in this way.
to exactly kind of trace where's this kind of, you know, ground truth nugget where it got a particular piece of information. But I would today encourage you to try the same query with search-enabled. I did use the O1 that I pasted something. So yes, O1 is not a search-enabled model. I will.
Okay. Okay, then can you explain what is the search?
Yeah, there is an icon. If you could kind of, you go to Chattopadhyay 4.0. I know it's very confusing today. We will unify kind of and make everything much more simple. But there's a globe icon. And it essentially enables the model to search the internet for results.
Mark, you've got a very promising career in customer support.
Lizzie, thank you so much for your question. So good to meet you. See you soon. So next on deck will be Daniel McNelia for a live question. And while we queue him up, I'm going to ask a question from Ahmed Al-Gamal, founder at Playform AI and professor at Rutgers University. And I just want to share with the community that Dr. Al-Gamal is like one of the pioneers of AI art. And he has a really beautiful presentation that we have archived in the forum for anyone who hasn't met him or wasn't with us for that presentation. His question is, what do you think is needed to go from where we are now, where AI can solve math Olympiad kind of problems, to the point where AI can solve PhD level math problems? I think Mark or Terry, either of you can take this one.
Right. I think it depends on whether it's with human assistance or without human assistance. I think if it's human supervised, it can certainly help. It can already do a lot of more menial tasks in a math project. I think it's, as I said before, it's missing a lot of the strategic planning, what to do when there's no data to tell you what to do. And I'm not sure how to get past that, other than to have human supervised, human experts so far.
Yeah, I think at a meta level, just kind of zooming out, I do think like if you looked at kind of how self-driving cars have evolved, right? Like when do you get to the point where you can kind of like trust the car to take you from point A to point B without supervision? And I think really the underlying progression wasn't magic. I think it's just more and more reliability over time. And you start with, it's like 90% accurate in making decisions, then 99%, then 99.9%. And of course, there are no guarantees that the cars make that they're always going to succeed or not going to make errors. But I do think kind of just the amount of direction and supervision will probably shrink over time. You can trust the model to do kind of more self-contained tasks that require more just kind of longer trajectories of thought on its own, and it will become more and more reliable at that.
Just to jump in on that, I think one area where this starts to get really fascinating is for things like physics and maths, where some of the answers at least are axiomatic and you can go first principle down, you can see how long the training cycles and improved reasoning models get you to these answers. But then I think about applications of biology, where there's a huge amount of redundancy, and there's some combination of probabilistic plus first principle plus contextually determined. And you wonder whether that that requires a different approach, whether that's also going to be amenable to this generalizable first principle approach. And as we explore those kinds of things, I think you start to get quite interesting insights about what needs to be true to solve these interlocking, interdependent problem sets versus kind of classical top-down problem sets. Thank you, James. Daniel, so good to see you. Yeah, great to see you too. Welcome. I think last we spoke a couple of years ago, you were wrapping up your PhD. So would you like to let us know where you are now? Introduce yourself to the community.
Yeah. Hi, everyone. I'm Danny. I did my bachelor's in math at UC Berkeley. And then up until about six months ago, I was a PhD student in like AI for science at University of Wisconsin. And now I'm actually in law school, working on like AI and law related topics. So kind of done a bunch of different things. But yeah, my question for Professor Tao was, I know like historically, sort of the math theory has developed first and then researchers in other fields like physics, especially or chemistry or other domains will take that theory and apply it to their problems. Now with AI being such a big thing, do you see any feedback going the other way? Like I know in physics, people are using machine learning a lot for simulating like computational solutions of PDEs and stuff that you can't solve using traditional methods. Do you see like mathematicians gaining any new insights into theory from those other fields, especially because we can just generate a lot more data?
Yeah, no, I mean, mathematics has always been a two-way street. I mean, there have been discoveries by physicists that mathematicians didn't have an explanation for. And then they had to develop theories of mathematics. You know, Dirac invented something called the Dirac delta function, which wasn't a function according to orthodox mathematics. And we had to enlarge our notion of what a function is. It's always gone two ways. So I can imagine a very practical science-driven application, maybe powered by AI, discovering some new phenomenon that just cries out for explanation. And it will be discovered empirically. And then mathematicians will be motivated to find theoretical explanations. So it's always been a two-way street between theoretical and applied sciences.
Awesome. Thanks. Nice to see you, Daniel. Okay, let's please queue up Ashish Bhatia. And while we do that, take a question from the chat. So, Terrence, I think this one is for you. And I hope I can pronounce all this accurately. Can universal approximation theorem be dethroned? A recent paper on Kolmogorov-Arnold networks has gained a lot of hype. What are your thoughts?
Okay, I don't know this particular result. I mean, universal approximation theorem tells you that any operation can in principle be modeled by a neural network. But it is just a pure existence theorem. It doesn't tell you how to find this neural network. It may be too impractical to actually use. But it does tell you that there is no theoretical obstruction to having neural nets solve very complicated problems. I suppose to say perceptrons, which do not have a universal approximation property.
Yeah, in general, the whole theory of machine learning is really lagging decades behind practice. We do have a few sort of bedrock theoretical results like the universal approximation theorem, but we don't have a good explanation of why neural nets work as good as they do for some tasks and why they're terrible for other tasks. So, yeah, there's certainly a lot of theoretical work that has to be done.
Thank you, Terry. Ashish, welcome to the forum. Would you like to introduce yourself?
Thank you, Natalie. My name is Ashish Bhatia. I work at Microsoft as product manager and I build no-code platform for AI. So, my question is I actually want to describe a workflow that I use to write things to do stuff at my work, which is I use O1 for deep thinking of stuff, any topic that I'm kind of working on. And then I use 4.0 to do research. And then I finally, and these are different tabs on my browser, and I finally use 4.0 with canvas to kind of put it together, all of that, right? So, this is kind of human curated workflow. I'm trying to figure out if there would be an easier way to do this in the future.
Very good.
Very good question. I alluded to this a little bit in a previous answer, but there's so many models, and part of the reason that it's confusing today is O1 was always kind of meant as a research preview, and you know, we just wanted to showcase kind of more advanced reasoning capabilities to the world. We will kind of make it a lot less messy. I think you want to integrate everything together, make it very, very seamless, and I think that'll provide a much better experience for you. So yeah, again, it's hard to promise a date on this, but I think your workflow will become a lot simpler. Thank you.
Okay, can we please queue up? Juanita, Apu, and Juanita, you're gonna have to tell me how to pronounce your name. Please forgive me if I was just inaccurate there. And then we'll take a question from the chat, and this is from Michael Skiba, a software engineer at Insurotics.
Given the capacity for collaboration among humans, could the diversity in having multiple models reasoning together elicit greater creativity in proofs that a single model would fail to reach? And maybe you can kick this one off, Mark.
Yeah, I mean, I think that's a very reasonable hypothesis. I think like anytime you have kind of multiple agents in a system where, you know, maybe like the agents have different incentives or kind of create some kind of environmental dynamics between them, you can get very kind of interesting behavior, right? And I think that certainly should be the case for our AI agents as well.
Yeah, and I think like one particular instantiation of this could be like maybe they just end up specializing in the ways that maybe Terry has described in the past, right? Like one kind of becomes a product manager, and one kind of becomes more of an executor. So you could kind of imagine them kind of developing specific roles. So yeah, I mean, is it guaranteed that this kind of specialization will outperform like a single, you know, very powerful thinker on its own? I think it's still a little bit unclear, but it's certainly very interesting to explore. Yeah, I don't know, Terry, if you want to add something.
No, I think we should try all kinds of things. I think it's good to have a very diverse set of approaches to solve these problems. I think problems where there's a really well-defined metric that you can optimize will work. There, having a team of competing AIs trying to optimize this benchmark will actually probably do better than a very vaguely defined task where having too many voices may actually make things just harder to manage.
Juanita, welcome. And if you unmute yourself, could you first please tell us how to pronounce your name so that in the future I say it correctly?
Oh, hello. How are you? Wonderful. Can you hear me?
We can hear you loud and clear.
Oh, okay. I'm hearing the music right now. I can't hear the audio feedback for some reason.
Maybe you have two tabs open. I'm reading your lips. Refresh the tab. I think you have two tabs open, but yeah, I would refresh the tab. So, Juanita, how do we pronounce your name? Actually, okay, let's go to a question from the tab and we'll get back to Juanita while he figures out his answer.
And we'll get back to Juanita while he figures out his audio. Okay. So, this is from Ankit Kashyik, Executive MBA at Wharton and Google AI.
AI explainability still remains an area of research requiring additional investment. Where can mathematical theory help in formal characterization of AI from a system standpoint?
Yeah, I think this is an area where actually theory is very, very behind where it should be. I mean, we have some difficulty results that show that, yeah, at least current models. I mean, it actually is provably hard to actually unpack, given a model, exactly what was the route taken to get there. The current architectures are not designed at all to do this kind of tracing. I mean, it should be possible, but I mean, there'd be a trade-off. I mean, it will come at a huge hit in performance and training and so forth. So, I mean, there's a reason why we don't do it right now. People are beginning to do sort of post-statistical analysis on a model. Like, you can take a new one and maybe like turn part of it off or swap part of it with something else. And you can start sort of seeing what parts of the network were the most critical in arriving at an answer. But yeah, it doesn't really have, it's still kind of empirical. We don't really have a good robust theory on this.
I largely agree with that. I think interpretability today is a very empirical science. And there's like a lot of mechanistic interpretability techniques that are effective at, you know, like identifying like sub-networks or parts of a network that are responsible for certain things. But yeah, it is hard unless you kind of like architecturally bake into the model in a certain way, like to just be like, oh, you know, this was why a model did a certain thing. Thanks, fellas. Let's bring Aditya Raj to the stage, please. And while we queue him up, here's a question from Belinda Mo, a master's student at Stanford. What are the neural network architectures and data set formats that you find most promising for theorem proving, especially related to Lean's MathLib?
I don't know if there's any specific architecture that is better or worse. I think we actually have to do empirical studies, actually. I mean, I think we need to create data sets of thousands of theorems and just test different architectures and see what happens. We don't have a theoretical prediction of what's going to work right now.
Yeah, very much so. I think in some sense there's been a convergence of architectures on the language modeling side. I think like oftentimes there are variants of transformers. I think today they're kind of people exploring what the next generation of transformers might look like. So things like potentially state-space models. But I think the jury's still out on which is specifically a very good theorem prover. One thing that's been surprising, I think, in AI is that domain-specific knowledge, like AIs that try to incorporate domain-specific knowledge often don't outperform general purpose. The bitter lesson, right? Yeah. So it's still puzzling to me why that's true, actually.
Yeah. I mean, in some sense, like, the architectures that most leverage the underlying hardware, like, maybe it's a statement that, you know, that just is like a bigger gain than like any kind of specific engineering that you could do.
We are having, Juanita is having a technical issue, so I'm going to take another question from the chat. And this is from Shirin Shaf, Director at Visual Academics.
Any advice to researchers from other fields who want to collaborate with AI and mathematical problems despite not being trained mathematicians? What are the common pitfalls and how to avoid them?
I think there isn't enough of a body of successful examples to tell what the common pitfalls are. So the projects that seem to be working that will be well suited for this paradigm in the future are really large collaborative projects run out of GitHub, where a task can be broken up into lots and lots of little pieces, some of which may require math expertise, some may require expertise in smaller science, some may require a facility using AI, but you don't have to be an expert in everything. So that's just beginning to happen. I'm running a pilot project right now to try to see if that kind of thing is possible. But it's certainly not so many of them that you can just sort of sign up for one right away. I mean, it's maybe like three or four of these things right now.
floating around. I think you would have to connect with a human, with experts. You need to find the right collaborators, you know, an expert mathematician, an expert in AI and so forth. And I think you need a lot of serendipity. I think right now you can't just start a project by yourself just because there's so few models to figure out what to actually do. But you know, I mean, we need to experiment and try lots of things and see what sticks. Thanks, fellas.
And last but not least, can we please queue up Jordan? Jordan's a longtime forum member, been with us from the beginning. He has a unique perspective. He comes from a background in Google, but is also a marketing professional. So I'm excited to hear from him.
You're making this brown man blush. Thank you very much, Natalie. And you've done a great job this year. Amazing forum, great guests. Everything's fantastic. Caitlin, too. Mark, thank you for presenting. Thank you, Terrence. Also, thank you, James. Just wanted to ask you, Mark, what are some awesome use cases for O1 that you're seeing people not talk about that you think should get more love?
Thanks. Yeah, really good question, too. So I think there is this kind of misconception that, you know, reasoning is only kind of in math and coding. And, you know, a lot of the use cases that we've seen have heavily showcased reasoning across, you know, diverse domains, right. And I think like, like in linguistics, right, actually, like O1 can really unpack and, you know, help with, you know, like, understanding like linguistics, or even like linguistic puzzles. And, like, kind of like, you know, breaking like ciphers, you know, like getting discerning patterns from data. So I do think like, I would challenge you to kind of look at use cases outside of just pure math and coding, even though it's certainly excels at that. But kind of see reasoning as something that's like, very general and broad based. And, yeah, I mean, I think like, another example is, you know, James and I have kind of worked on partnerships with, you know, like material science organizations or other external organizations, and they found kind of reasoning to be extremely effective there as well.
Thank you, Mark. Anything else from Terrence or James? Okay. James, did you have something to say?
I was really just going to echo Mark and say that there is a tendency sometimes to think that unless the models can answer every scientific question perfectly, there's no utility, you either need 100% or nothing. And that's a sort of binary question. But often, being able to accelerate smaller parts to Terry's point about math more broadly, is itself a huge compounding gain. And very often the impact of science isn't just the theoretical work, or the experimental work, it's the business to commercialize or bring that stuff into the real world. And we see really quite transformational gains across each of those things, but particularly in that last, that last bucket that I hope will ultimately result in better and more science coming to the world. I've heard that big drug companies, part of their biggest gain from using AI tools is actually accelerating their regulatory paperwork.
Awesome. Thank you so much, fellas. What a beautiful talk to end 2024 with. And we'll send all of this to you via email, the recording will be published in the forum by early next week. And as James said, this really is just the beginning of our deeper dive into how our new reasoning models can accelerate math and, and science. And so we can't wait to host you again in 2025. Thank you so much, Dr. Tao. Thank you so much, Mark. James, what a beautiful facilitation. I definitely couldn't have handled that with so much grace. I'm so glad that you came and, and participated as the facilitator for this talk. And Terry, thank you so much. It's been I was just thinking, it's been just a little over the years, a year since the last time you joined us. So thank you for all of the grace and flexibility as we were planning this event. And I hope that we can make this a ritual, a yearly ritual to have you back.
Yeah, it was a pleasure. Oh, thank you.
Okay, fellas. Well, that was our last expert talk for 2024. But we are hosting one last technical office hours December 19. For the community members here who are new, our technical office hours are an opportunity for you to meet for one hour with a software engineer, a solutions architect or a solution engineer and get your technical challenges potentially solved, unblock you, give you some ideas that are related to your use case. I think it's a really beautiful opportunity to connect one on one with our technical team at OpenAI.
And finally, as all the new members are here hearing this for the first time, we want you to know that this is your community. And you now have the agency to refer peers, people in your network, we prioritize referrals from the community. So we're going to drop that referral application in the chat. And we would love to also incorporate some of your community members. Pretty soon in the next few weeks, definitely by the first few weeks of January, we're going to be launching geographic chapters and interest groups. That means that you'll be able to self organize, you can find people in your area of the world, connect with them, host coffee chats. And I hope this makes it easier for you guys to continue conversations outside of the walls of the forum.
And this event isn't over, please, if you want to meet each other one on one, we're launching another notification, you can go into the virtual networking time, it'll be matched one on one with other members of the community. The default is 10 minutes, but feel free to cut it short after a few minutes so that you can meet more people with the allotted time.
And that's all we have for the evening. I'm so so very pleased to end 2024. On this note, what a beautiful event, what amazing people in our community. I feel so grateful that this is my job. And I really love hosting all of you. Happy Tuesday, everybody, and we hope to see you soon. Good night, everybody.