Expertise, Artificial Intelligence, and the Work of the Future Presented by David Autor
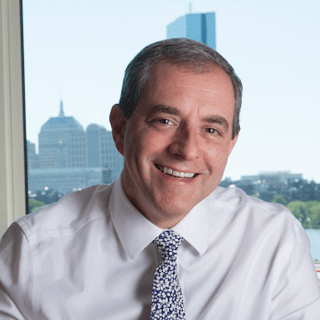
David Autor is Ford Professor in the MIT Department of Economics, codirector of the NBER Labor Studies Program and the MIT Shaping the Future of Work Initiative. His scholarship explores the labormarket impacts of technological change and globalization on job polarization, skill demands, earnings levels and inequality, and electoral outcomes. Autor has received numerous awards for both his scholarship—the National Science Foundation CAREER Award, an Alfred P. Sloan Foundation Fellowship, the Sherwin Rosen Prize for outstanding contributions to the field of Labor Economics, the Andrew Carnegie Fellowship in 2019, the Society for Progress Medal in 2021—and for his teaching, including the MIT MacVicar Faculty Fellowship. In 2020, Autor received the Heinz 25th Special Recognition Award from the Heinz Family Foundation for his work “transforming our understanding of how globalization and technological change are impacting jobs and earning prospects for American workers.” This spring, Autor was selected as one of two researchers across all scientific fields a NOMIS Distinguished Scientist. The Economist magazine labeled Autor in 2019 as “The academic voice of the American worker.” Later that same year, and with equal justification, he was christened “Twerpy MIT Economist” by John Oliver of Last Week Tonight in a segment on automation and employment.
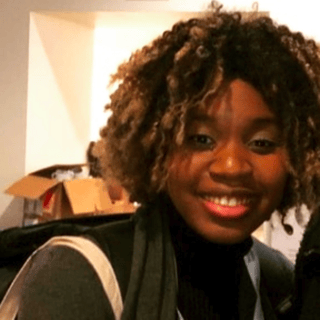
Tyna Eloundou is a researcher at OpenAI, whose most recent work includes leading safety evaluations, economic impact evaluations, and the democratic inputs to AI grant program. Prior to OpenAI, she worked as an associate economist at the Federal Reserve Bank of Chicago and programmer at the RAND Corporation.
About the Talk: Much of the value of labor in industrialized economies derives from the scarcity of expertise rather than from the scarcity of labor per se. In economic parlance, expertise denotes a specific body of knowledge or competency required for accomplishing a particular objective. Human expertise commands a market premium to the degree that it is, first, necessary for accomplishing valuable objectives, and second, scarce, meaning not possessed by most people. Will AI increase the value of expertise by broadening its relevance and applicability? Or will it instead commodify expertise and undermine pay, even if jobs are not lost in net. Autor will present a simple framework for interpreting the relationship between technological change and expertise across three different technological revolutions. He will argue that, due to AI’s malleability and broad applicability, its labor market consequences will depend fundamentally on how firms, governments, NGOs, and universities (among others) invest to develop its capabilities and shape its applications.
I'm Natalie Cone, OpenAI Forum Community Manager, and I want to start by reminding us all of OpenAI's mission. OpenAI's mission is to ensure that artificial intelligence, AGI, by which we mean highly autonomous systems that outperform humans at most economically valuable work, benefits all of humanity.
Today's talk, Expertise, Artificial Intelligence, and the Work of the Future, will be presented by David Autor, followed by questions and discussion, which will include my colleague Taina Ulundu. David is a professor in the MIT Department of Economics, co-director of the N. Barrett Labor Studies Program, and the MIT Shaping the Future Work Initiative. His leadership explores the labor market impacts of technological change and globalization on job polarization, skill demands and inequality, and electoral outcomes.
David Autor has received numerous awards for both his scholarship, the National Science Foundation Career Award, the Sloan Foundation Fellowship, the Sherwin Rosen Prize for Outstanding Contributions to the Field of Labor Economics, the Andrew Carnegie Fellowship in 2019, the Society for Progress in 2021, and for his teaching, including the MIT McVicar Faculty Fellowship. In 2020, Autor received the Heinz 25th Special Recognition Award from the Heinz Family Foundation for his work transforming our understanding of how globalization and technological change are impacting jobs and earning prospects for American workers. This spring, Autor was selected as one of two researchers across all scientific fields at NOMIS, Distinguished Scientist.
The Economist magazine labeled Autor in 2019 as the academic voice of the American worker. Later that same year, with equal justification, he was christened Torpey MIT Economist by John Romer of last week tonight in a segment on automation and employment.
David, how did we land you for this talk? You're sure to be disappointed after that much hype. Great to have you. Thank you so much. This is like a hard act to follow. We are all really excited to have you here tonight, David. It's really an honor.
Thanks. Well, very much. I've obviously been following OpenAI closely, talking with people in your policy group, research group about opportunities, including Taina, who we'll be moderating and we'll be discussing later. So let me just dive in with the talk. And again, I welcome questions and interruptions. I can always shorten as needed. I think it's much more productive to, you know, engage as much as possible. So I'm going to try to share my screen.
Okay, cool. All right, great.
So speaking about expertise, artificial intelligence, and the work of the future. Let me begin with expertise.
So what is the difference between these two jobs, crossing guard and air controller? Both of them essentially are the same job, which is preventing collisions between people and vehicles, vehicles and vehicles. And yet the air traffic controller job pays about four times as much. And the key difference between them is not their social value, but the scarcity of expertise to execute them. To be a guard in almost all US states requires no training or certification of any kind. And to be an air traffic controller, you need to go to an air traffic control college, you need to apprentice and so on. But fundamentally, if we needed to spend $130,000 a year crossing guards to prevent collisions between vehicles and children going to school, we certainly would spend that. So it's really not a question of social value. It's a question of scarcity.
So let me explain what I mean. Expertise is what makes labor valuable in a knowledge economy. So expertise means domain specific knowledge or competency that's required to accomplish something, a particular goal. So for your expertise to be economically valuable, first of all, the goal itself must have market value. So it needs to be, you know, not card tricks. And the other is that the expertise has to itself be scarce. Not everyone possesses it. So to quote The Incredibles, if everyone is expert, no one is expert. It matters because non-expert work pays poorly. So think of waiters, cleaners, janitors, security guards, school bus drivers, crossing guards, daycare teachers. These are purely poorly remunerated jobs in almost every economy, although the exact degree differs. And not because these life and death jobs like school bus driver, crossing guard, daycare teacher, but because the supply of people who can do that work is abundant.
So let me talk about demand for expertise across three different eras, the industrial era, the computer era, and now the AI era. And I think it's not hyperbolic to call this a different era, the era that we're in.
So the Industrial Revolution, what it replaced, artisanal expertise. Artisanal expertise meant the skills and knowledge to take a production process from start to finish to make something and do all the steps along the way. So you could be a cobbler or a tailor or a blind man or a millwright. But this was a way of working in which one person or a small team of people with extensive apprenticed knowledge and typically specialized tools would go through the process of making an object from step to step. Mass production, which started in the 19th century, displaced artisanal expertise. And mass production was a different way of accomplishing the same end, which was to break work into very small numbers of incremental steps that then could be divided and subdivided among numerous workers. Not just any workers, actually, I should say just any workers, in fact, that was the key difference, was in the early era of mass production, most of the workers were extremely low paid and untrained. They were often children and unmarried women who worked in fairly brutal conditions with a lot of dangerous machinery and not very forgiving workers to produce things like textiles.
So if you go to the original textiles, for example, in Manchester, England, textile mills, you will see that these machines were incredibly cramped, close together. They couldn't do anything. You needed to change a thread or do a repair. So it was very handy to have children doing the work because they were rapid and dexterous. And occasionally if they lost a finger, it wasn't that big a deal. Maybe it would grow back or anyway, these were indentured children. And so the initial era of mass production was not, was revolutionary for productivity, but it wasn't especially beneficial for workers. And in fact, it displaced artisans, right? It wiped out people who were making things by hand. And what you see there is a dolly generated image of the Luddite uprising. And they were rising up against the power loom, the power frame, because they were concerned, quite correctly, that it displaced the valuable expertise they had to make their labor less valuable.
Now, as industry advanced, especially in the 20th century, it became much more complicated. You're using more complex tools. You were using more valuable inputs. And ultimately, you needed people and customer expectations were changing. And you needed people who could do that well. You needed people who could master tools and follow rules and carry out the steps of industrial production to manage a complex process where the stakes were relatively high to do it well. And so as industry advanced, the conditions went from brutal and low paid to better paid and more skilled and more expert. Also, I don't want to say this is a fully autonomous process. Unionization had a good deal to do with this. But that era of productivity and demand for people to do this type of mass expertise work helped build the middle class in many industrial countries, including the United States.
And when I say mass expertise, what do I mean? Well, on the left of tool and die workers from the Ford River Rouge plant, on the right are students in a typing class at Broken Bow Business College. And I want to say that mass expertise was not simply factory work, but really a lot of modern industrial work essentially was of the form following rules, mastering tools. So that might be in an office, that might be in a phone bank or as a telephone operator, of which there are one point three hundred thousand in the United States. I'll be as a tool and die worker. And and this era of rising of the rising demand for mass expertise also required rising levels of education. It required.
Some degree of literacy and ability to follow instructions.
The US had made big investments at the turn of the late 19th century and early 20th century to send its entire population to high school, or its entire young population, and so it was really well-prepared to capitalize on this change. So this era persisted, I would say, until around the very early 1980s with the advent of the computer revolution.
So here, for your viewing pleasure, is a picture of what I think of as the first industrial computer, which is the Descartes Loom, and this is from 1808. And what made this a computer, distinct from other machines that preceded it, is that it's a symbolic processor. Those brass cards that you see being fed into the machine contain instructions to the machine on what arms to lift, what threads to use, and there's a surviving example of a program of 10,000 brass cards that actually weaves a silk portrait of Descartes himself, the inventor of the Descartes Loom.
By the way, the Descartes Loom was the inspiration for Hermann Holruth, who invented the punch card, the paper punch card, the successor to the brass punch card you see in front of you, which was used to read the 1890 U.S. census, the first machine-read, computer-read census in history, and then Hermann Holruth formed a partnership with Thomas Watson to form IBM.
So what made the computer distinct from prior technology? Well, it's a symbolic processor, meaning it accesses, analyzes, and acts upon information, and gives it the ability to carry out tasks, in particular, tasks that are codifiable, that can be turned into series of steps or instructions that a non-sentient machine that cannot improvise or exercise could carry out faithfully and rapidly and produce acceptable results. And that made computers competitors to workers in these, what I would call, mass expertise tasks, carrying out, effectively following rules and mastering tools.
But computers had a specific advantage relative to workers in this period, is that there was at least a one trillion-fold decline from 1950 to around 2010 in the cost of executing standardized instructions. Workers, the cost of labor did not fall by a trillion-fold, but the cost of carrying out these tasks with machines did, and that created competition. You can see that competition in the change in the structure of employment in the US and many other high-income countries, and what you see is this kind of barbell with hollowing out in the middle and rising employment on either end.
So let me just explain what's in this figure. So these are 10 broad occupational categories that cover all of US employment aside from agriculture, and they're ranked from lowest paid to highest paid. Personal services and cleaning and protective services on the left, on the right are managers and professionals, and on the middle are many of those mass expertise jobs of the mid-20th century, particular production work, operative work, administrative office, and sales work. And many of those jobs involve following well-understood sets of rules and procedures, work that requires literacy and numeracy, is well-suited to be codified into software and executed by machines instead.
And so this had a kind of a bifurcating effect. On the one hand, so it benefited this green group on the top, the people who are technicals, professionals, managers. If you are a person who works with information for a living, the rising availability of knowledge and processing power makes you more productive, allows you to do better legal work, better research, faster calculation, better engineering, etc. College degrees who did a lot of this office and blue-collar work, it essentially pushed them downwards. For those who did not go on for college, many of them found themselves increasingly in food service, cleaning, security, entertainment, recreation, home health aides. And again, there's reliable work, but it's poorly paid. And the reason that it's poorly paid is because it's not expert. It's work that most, that many, many people can do without substantial investments in training or certification.
So, and it's interesting to look at this barbell and say, well, what's going on here? Why the things in the middle? And this is what people call Polanyi's paradox, which is that many, many things that we do, that we actually know how we do them. And that has proved a barrier historically to computerizing them because they follow procedures that we don't understand. So we all explain to another person, to a young person, how do you do addition and subtraction? These are formalized procedures, but you couldn't explain to a person, your kid, for example, how to ride a bicycle and give up and get up and give a lecture, nor would it actually be useful to do so. Even if you could do that, it wouldn't actually help them ride a bike, right? The way you help a person ride a bike is you put them on a bicycle, you give them push, and you say, good luck. And that's because that information is learned, that skill is learned tacitly. It's not learned, it's not explicit knowledge.
So whether it's like riding a unicycle or telling a funny story, you know how to do it, but you don't know how you do it. And historically, that was a barrier to getting computers to do it because we couldn't write the procedures.
This era of computerization was really a mixed bag, not just in terms of occupations, but also in terms of wages. So in terms of earnings levels, the U.S. is sort of an extreme example, but we saw this incredible bifurcation of earnings where between essentially from 1980 forward, earnings for those with less than college education fell in real terms. It rose somewhat for people with a college degree and rose a lot more for those with a graduate degree. And that's even more true for women where you see these greater earnings growth.
And this is not fully explained by, but partially explained by this phenomenon of this hollowing out of the distribution of occupations. And, you know, recent work by my colleague, Darona Simoglu, and Paschal R. Strepper, one of our former MIT students, sort of tries to show this very dramatically or try, where essentially, the type of work that was ultimately displaced, saw real earnings declines over the last 40 years. And when I say I do not mean they were individually displaced from those jobs. I mean, they were of the demographic and education groups who were likely to specialize in manufacturing, likely to specialize in work, instead moved into different types of jobs where they earned less.
So let me just pause for a minute to see if there are any questions. I'm about to kind of change gears again, but I welcome any thoughts on this or objections. Everybody, you can just hop on in, unmute yourself if you have a question. Or if you'd like to be orderly, you're also welcome to raise your hand.
I had a question about how you describe the Industrial Revolution. It's a bit different to how I previously thought of it. And I don't know if my way of thinking if it's wrong or if they're orthogonal in some interesting way, or what. But I guess I thought of the Industrial Revolution as being about prior to that, prior to steam engines and so forth, the main thing humans did was inject energy into processes. And, you know, steam engines and so forth allowed that to be replaced with machines. And so the Industrial Revolution was kind of about humans moving from being a thing, you know, mainly injecting energy to mainly injecting kind of cognitive or dexterity. And then so then the way I think of it as AI is like, oh, maybe AI will turn out to then remove the bits where humans inject the cognitive piece and kind of what's left. That's interesting. Is that a reasonable way of thinking about it?
Well, I mean, I think that's complimenting what I'm saying. So it's a case that human muscle power was a much bigger deal in the performance of work prior to the Industrial Revolution. Now, a lot of that had been, you know, was animal power, right? So, now we gave way to animal power for many activities. And then, you know, in the early, well, so but I would say there's really two different parts to this. One is kind of, as you say, you know, industrialization was a different way of producing energy and performing work, but also it's a completely different form of work organization. And, you know, you could have used those machines in theory to, you know,
helping artisanal blacksmiths, right, pound iron more efficiently, or individual bootmakers to use sewing machines instead of, you know, just using their hands and spinning wheels and so on. But so, but so that the so energy was used differently, but it wasn't it wasn't really just the mechanical processes, but the entire way the structure of work was organized and the demands of knowledge of that particular work.
And so, you know, and also what they worked on changed because before the industrial revolution, I think in the UK, 90% of people worked in agriculture, you know, and then nowadays, I think it's like 0.3% or something. Yeah, absolutely. So that's right. So and that's, and I'll get to that in just a minute, actually, and talk about agriculture, because I think it's quite instructive.
All right, that's a great question. Other questions? I'll just, I'll move forward, but please feel free to jump in.
So I, so this, I'm gonna get briefly detour. So it's important, I think, a critical thing to recognize, and which was really implied by the prior question is, when we think about technological change, it's very easy to think about what is automated, that's the most natural frame to take.
But in fact, work isn't merely being eliminated over time, it's also being created. So agriculture is a good example. So at the turn of the century, less employment was in agriculture. At present, it's under 2%. And obviously, that's not because we're eating less. And really, this reflects multiple centuries of improvements in urbanization, irrigation, genetics, and that's led to incredible productivity improvement. So enormous fraction of the population has been able to move out of agriculture because of these technological improvements.
But so you might look at that and think that, well, if that's the case, then hasn't work gotten sort of narrower? Hasn't we haven't we just sort of squeezed ourselves into a smaller subset of the things to do in the world?
But that's really not because there's lots of new work being invented on a common ongoing basis. So how do I how do we know this? Well, for a moment, you can just sort of introspect on this, right? Think of a doctor do, you know, relative to today. So in the good scenario, a doctor 100 years ago would basically, you know, you know, tell you to have some bed rest and give you cold water in the bad scenario.
So, you know, give you a blood letting and things like that they didn't do a lot. At present, medicine is incredibly specialized. And there are hundreds of different medical specialties and literally hundreds of types of, you know, medical doctors. And that specialization reflects a deepening of expertise, right? Mastery of knowledge that didn't previously exist. Isn't there are so many specialties as people have limited capacity to master it all at once.
So you can actually attempt to measure the growth of new work by looking at historical census volumes. And the Census Bureau actually have coding aids that it uses to take the write in occupations that people write on their census forms, and translate them into consistent categories. So this is in the index of industries and occupations.
So for example, here from 1990 is one occupation that you would find in the census file, which is health technicians and technologists. And this is a list of a couple of 100 things that would correspond to that. So for example, an artificial limb fitter, a brainwave technician and extra corporeal circulation specialists, and ocular care technician and surgical bracemaker. These are all new specialties that were recognized by the census in just one decade within this narrow occupation. And I, you know, and if you look at this list, you can see each one of these titles, right, is effectively requires new expertise, right knowledge of limb fitting of brainwave, brainwave measurements on these were these, it's not that these were previously done by machines or by less specialized people, they those that knowledge and expertise did not exist, you know, prior to these decades.
So you can compare across volumes of this document to see what's been added over time. So for example, in 1940, a welding machine operator was first introduced in 1960, the textile chemist, in 1980, drone pilots. In 2000, the Census Bureau recognized AI specialists in 2018. But late, they recognized cybersecurity analysts.
So you might look at this list and think, I see. So new work is essentially the, you know, the kind of introduction of people who know how to build new machines, repair new machines, sell new machines, scrap old machines, etc. But in fact, just as common are new service activities, reflecting changing tastes, changing demographics, rising wealth. So you know, acrobatic dancers, pageant directors, hypnotherapists, sommeliers, drama therapists, these are also new specialized categories.
And so the point I want to make here, and I won't make it at length, because I realize I can see I will run out of time before I run out of stuff to say is that a lot of work that people do at present reflects new work that didn't exist, you know, decades ago.
So this shows you, for example, in 1940, there were about 50 million workers in the United States doing the work that existed in 1940. In 2018, there were about 60 million people doing the work that existed in 1940. And there were another, excuse me, and there were another 90 million doing work that had been created, had been invented since 1940.
So when we think about the evolution of work, it's very, it's very natural to think about what is just being automated. And it's much harder to recognize and even still harder still to forecast what new things will arise in their place.
So I want to emphasize that I'm going to skip this section, we have ways of measuring it and looking at technologies that, you know, automate versus augment different forms of work. But in the interest of time, I'm not going to go through all that, because I want to leave time for discussion. So let me instead, move forward, and that time permits.
So let me instead, sort of walk us forward again. So I've talked about the artisanal era, I've talked about the industrial era, I'm going to talk about the computer era. And so now I'm going to talk about the artificial intelligence era. And I'll characterize it one way, and you may have different ways of characterizing it. And that's, that'll be useful to discuss. So we used to say, you know, previously, computers could only do these routine tasks, right, we only knew how to program to do various things.
And of course, now, you know, well, you know, machines can do autonomous learning, they can do what tasks it would be considered creative if done by people. And of course, they can discover and and, and so this is, again, I'm telling you things you already know, but this is an enormous step forward. And of course, how does it work? How do computers do it? Well, you know, I refer to this as Polanyi's revenge after the same philosopher, Michael Polanyi, which is that prior to AI, we knew more than we could tell. There were things that we knew how to do that we didn't know how to program computers to do.
In the AI world, we now have a world where computers know more than they can tell us either many things that machines are learning, that we don't that we that are that lack of legibility, as you would say, right, that we have. This is actually an interesting irony here, which is that humans are not able to communicate tacit knowledge to computers, and vice versa. And so I really learned by either and yet not not very communicable.
Okay, so what is this change? Right? Well, it means that machines will be entering a domain of activities that was not previously up for automation, right? All of the in addition to all those routine tasks that we've learned, we've gotten so good at automating over the last four decades, there's a whole new domain of activities that can use this different technology. What you might think of as expert work.
So what are the implications of that? Well, I think the first place that most people turn is job displacement, right? So I'm just, you know, recent articles in Washington Post, you know, Chachi, we took their jobs. Now they walk dogs and fix air conditioners. Air conditioner repair, by the way, has a very future. So no one should be worried about that particular example. But in the India Express, they're worried about how this may affect offshoring and whether it will actually increase demand or displace demand of the skilled services that are provided by less wealthy countries to the rich world.
And this is a real legitimate concern, I don't want to say this is a real thing and people will be displaced. But I want to suggest that this is not the most useful way to think about it. So many of you may have heard of this well-known article by Frye and Osborne in 2016 called The Future of Employment, How Susceptible Are Jobs to Automation? And I'm just
altitude stalls and inadequate training on how to respond to such stalls. So this is a case where expertise, the lack of expertise, was a contributing factor to a catastrophic event. And it's important to recognize that in certain high-stakes domains, like aviation or medicine, the consequences of AI eliminating expertise or devaluing expertise could be truly life-threatening. But there's also a positive potential of AI in terms of expertise. And let me give you an example from medicine. So in the field of radiology, AI has been developed to assist radiologists in detecting and diagnosing diseases from medical images. And this has the potential to significantly improve the accuracy and efficiency of diagnosis. For example, studies have shown that AI algorithms can detect certain types of cancers more accurately than human radiologists. So instead of replacing radiologists, AI can augment their expertise and enable them to focus on more complex cases or spend more time with patients. So the key question is not whether AI will replace experts, but rather how it will impact the demand for expertise. Will AI make human skills more valuable or less valuable? And the answer depends on how we integrate AI into our work and how we adapt to the changing landscape. So in summary, AI has the potential to transform work in profound ways, but it does not necessarily mean the elimination of work. The real concern is the impact on the demand for expertise. Will AI make human skills more valuable or will it devalue them? And the answer to that question depends on how we harness AI to augment and enhance our expertise. So let's embrace the promise of AI while also recognizing the potential perils and use it to create a future where human skills are elevated, where work is meaningful, and where expertise continues to be valued. Thank you.
high-altitude manual flying. And so this is an example of the danger of expertise erosion. The actual danger of when humans are not at the frontier of an activity and when it is delegated, one of the possibilities is we actually lose the ability to do this. And this is, in some sense, recognized in any expert field.
So currently, pilots maintain their license. They spend multiple days per year in a flight simulator just to maintain muscle memory. If you're going to get a surgery for some specific medical ailment, you would much rather go to a surgeon who does it often than to one who does it infrequently. Exactly for expertise to be maintained, it needs to be practiced.
So that's the kind of negative scenario, the taxi drivers and the pilots. Let me give you a more positive scenario. So think of the job of nurse practitioner. A nurse practitioner is a registered nurse who also has a master's degree as a nurse practitioner. There are somewhere between 200,000 and 400,000 in the United States. They essentially did not exist 20 years ago or were almost non-existent. And nurse practitioners are able to do diagnosis, prescription, and treatment. In other words, many of the things that previously were exclusively the domain of medical doctors, of people with an MD.
So what enabled this change is a rare case of an expert job being delegated to less expert workers. Not that nurse practitioners are not experts, they are. But they have five fewer years of education and apprenticeship than do medical doctors. Well, a couple of things. One was a recognition, actually started with nurses, that nurses were underused. They had a lot of skills and expertise. They were prevented by scope of practice laws from applying. But in addition, they're enabled by technology. The advent of electronic medical records and of software that prevents, for example, that warns you if you are going to prescribe drugs that are interacting, they potentially have an adverse reaction, allows nurse practitioners to do a broader scope of medical work with guidance and guardrails provided by technology.
In other words, the technology enables a person of not as quite a high level of professional training to do a set of very valuable tasks that previously were limited to an even greater elite. And this notion, and this is not an AI example, of course. This is just a much more pedestrian example. But I think it's a motivating example because it suggests a way that technology can actually enable people to do more expert work and make labor more efficient. Instead of as much of our technology the last four decades has created specialized work with big barriers, technology can also be used to increase the scope of work that people can do.
So let me give a couple of examples of this in the real world. So I think many of you will be familiar with it. I'll go through it quickly. This is the paper recently appeared in Science by my students Shaked, Nguyen, and Whitney Zhang. And stop me if you've heard this one before, but they did an experiment on Prolific where they asked people to do writing tasks. They were actually given two tasks. One was to write an advertisement in a reader report. It was advertising, by the way, for electric bicycle. They were supposed to emphasize green features. The other was to write a report based on reader report and write a short recommendation on whether a fast, fresh Mexican restaurant should open stores in China. And the difference between the treatment and control group is in the initial task, they were both using the same technology. And then the treatment group was offered chat GPT 3 and 1 half to assist them if they like. At this time, many people would not have been using it because this was six months ago.
And so there are three significant findings that I think are really important. First, of course, was a big speed up in the work. So the amount of time on average in the control group fell by about 40% from the first task, whereas it fell just a few percent among the treatment group. So they did.
A second is the average quality of the work improved. So they had great work where other college graduates who did writing for a living to grade the work for creativity, for brevity, for accuracy, and for lack of grammatical errors. And so the average grade rose.
But I think the most important finding is the relationship between use of the technology and the inequality of output. So this lower line in black shows the relationship between people's grade on the first task and the second task in the control group. And in general, people who did pretty poorly on the first task did a little better on the second task. People who did really great on the first task did a little worse on the second task. So this is a mean reversion. But in the control group, what you see is this kind of leveling up. You see this kind of leveling up. So you had improvements. The greatest improvements were the people who were least good initially. It didn't mean that everybody was an equally good writer in the end. But it allowed people who were less good to be at least average. And that's actually valuable because it's always been the case throughout all of human history that people are terrible writers. This has never changed. And I can attest to you as an MIT faculty, it's not changing the time I've been there either. And so this is a kind of interesting case where the technology enables, it closes this expertise gap a little bit.
So another example, and again, I'm sure you're somewhat familiar with this. This is by my colleagues Daniel Lee, and graduate student Lindsay Raymond, and Eric Brynjolfsson, who's at Stanford. And this was using a large language model in calculus. And I'll just bring out to you what I think is salient about this. So the way this chatbot worked, it did not interact with the customer. It instead read what the customer texted. So this is mostly through chats. It read the customer's text and then suggested solutions.
So the visitor says, my name is Alex. I'm super frustrated. I had customers call me all day. They can't access the information on the website. And I need this fixed ASAP. So they're supporting a product that is basically an enterprise sales product for the customers. And then the chatbot suggests a set of answers. For example, it's nice to meet you, Alex. I hope to get this fixed. Set expectations, well, do is first find your codec in the system. We can walk through this, blah, blah.
So here's the relationship. So the measure is called resolve per hour. So the people in blue, they never had access to the software. And you can see their productivity in their first month of service. They do about 1.75 resolve resolution per hour. By the time they're out at 10 months, they're doing about 2 and 1 half call per hour successfully.
David, I just want to jump out there and let you know that we have less than 10 minutes left.
Oh, OK. All right. All right. So we know it's important to you to take some questions from the audience.
Yeah, I apologize. I was thinking we were going till 7 and 15, which are 5, 15 your time. So let me just say, the main thing I want to emphasize is that this tool allowed people not just to get higher performance, but to improve faster. So it didn't mean that people were not people don't start at this work expert. They all start at the same level. But the rate of improvement and the level of final output is much higher. So again, you see this kind of leveling up.
OK. So I'm going to summarize, actually coming to the conclusion. But I'll come to it just a little bit faster. So the potential that I see here is that AI can be developed to complement expert judgment, to enable less expert workers to perform more expert tasks, to essentially provide not guidance and guidance, but guidance and guardrails, what to do and what not to do. But this will not happen of its own accord. This actually requires design. This requires intention to achieve these goals.
So getting quickly to the conclusion. So I said earlier, will AI complement or commodify expertise? Will it make human expertise superfluous or make it more valuable? And the answer is that that's not really the right direction, which is we should be shaping the work of the future, not simply trying to predict what it will be. Because the jobs that we actually get and the technology depends a lot on the investments we make. And just to give you a final example before I conclude, think of, for example, China's use of artificial intelligence. China has the world's most comprehensive automated censorship system, the world's most effective surveillance state. And these are remarkable technological achievements.
payments, they would have been available 20 years ago, and they would also be inconceivable without AI. But this is not because that's what AI does, right? That's because we're trying to invest those AI dollars to build out those capacities. You could similarly use those capacities to use AI to better assist with elder care, as in this example of a Japanese care robot that actually does a lot of the lifting in care facilities a lot where the injuries occur, or to provide instruction to workers doing construction or diagnosis or maintenance or repair, or to make learning more immersive, more accessible, less expensive, and more convenient, right? So these are all possibilities. And as you understand very well, AI is incredibly malleable, and what it does depends on what capacities you to build. And what capacities you build will affect the type of work that people do in the future, whether that work, whether you use it for surveillance, whether you use it to make people to enable expertise.
So let me conclude and then leave time for questions. So the challenge that you should be thinking about that we should all be thinking about is not the quantity of jobs. There's no sense in which we will run out of work in the foreseeable future in the next several decades, but their quality. We face weak or negative labor force growth in these populations, and the question is, will we use AI to create more expert work, work that makes human skills more valuable, or we instead use it to commoditize labor, devalue expertise, and displace workers? I think both of those are completely feasible. And we have seen the last four decades actually have been pretty dismal for people without college degrees, and a big part of that, the computer revolution, has in no sense been beneficent for all workers. And so I think of this as an R&D opportunity and also an R&D necessity to use technology to make work more valuable, and that means enabling people to use expertise more effectively rather than making it superfluous. And in its own accord, government, universities, private sector firms can shape the future of the work. And so I find it useful to sort of end by saying, ask not what AI will do to us, but what we want it to do for us. And that's a useful design principle to keep in mind as we go forward. So let me stop sharing there. Thank you so much, David. That was awesome.
I would love to also introduce Taina Alundu, my colleague now, as well. She has prepared some questions for David alongside, collaborating a bit through Taina's time at OpenAI. Taina is a researcher at OpenAI whose most recent work includes safety evaluations and the democratic inputs to AI grant program. Prior to OpenAI, she worked as an associate economist at the Federal Reserve Bank of Chicago and programmer at the Rand Corporation. Welcome, Taina. Thank you so much for joining us and being a voice of expertise in an area where I am not being the co-host tonight.
Thank you, Natalie. Thank you so much, David. It's an honor to be here. In the interest of time, I'm probably going to keep my questions short, maybe ask three questions, and then we can move to audience questions, if that works for you. And if everybody is okay, I'm happy to stay 10 or 15 minutes over so that we can answer audience questions. Is that okay with you, David?
Yes. I thought that's what we were doing. I apologize.
Okay. Awesome. I would have spoken faster or less. All good. So David, you mentioned at the end in your conclusion that you believe that it's feasible to build a future where we don't devalue human expertise. What are some policy levers in your view that might be most useful in this space? And for those of us or of family members who are planning to join the workforce or planning work transitions, what are things that they can do to ensure that they fare well in this world?
Okay. Good. So I think that, so I'm going to make a further distinction between forms of expertise. So if you think about the work that has become much more valuable over the last four decades, it's what we would call professional work, right? So managerial, technical, and specialized professional occupations. What makes professional work distinctive from other types of work is that you're making high stakes decisions about one of the things, right? So how to care for this individual, how to land this plane, how to remodel this kitchen, right? And so essentially, there's a, for professional workers, right? There's a body of expertise that you learn, whether it's law, whether it's medicine, whether it's engineering, whether it's piloting, whether it's contracting services. And you have this formal knowledge, and then there's a bunch of rules and procedures that you follow. Then for any specific case, you essentially have a body of formal knowledge to that specific example. So being a lawyer does not mean taking the bar exam every morning, right? It means applying that body of knowledge to the specific problems that you face. And that's where human expertise really comes in, is that translational expertise. That's where it's so critical. And I think what, I think that the way that AI can be useful here is that it can supplement some of that formal knowledge that people take so long to acquire, while still enabling them to do a lot of this translational work, a lot of the mediation between the formal knowledge and the actual application in the specific setting. And that's, you went into a, let's say you went to see your nurse practitioner and you found me instead. And I said, don't worry, don't worry, I don't know anything about medicine. That's okay. I have this AI right in my office that will tell me what to do. You would run away because of course, that's not going to suffice, right? If anything goes wrong, if anything deviates from plan, right, you need someone who actually knows how to act in that situation.
So I think when I think about what that design principle is, I think I would summarize it as technology to enable a larger group of people to do valuable work, right? If we could enable, for example, coding is a really good example, right? It's quite possible that coding will require less expertise going forward, not more, but not because it doesn't require, but let me say that slightly differently. It requires less specific expertise for languages and more general expertise for architecture, for architecting software, right? And so that I think is a potential model if we could make that work accessible to people without as much, without college degrees, for example, that would be a huge step. So that was part one of your question. Part two is what should people do about this? Let's come back to that just in the interest of time.
All right. I have one more question. One more question is how do you reconcile the finding that there's record employment in OECD countries to the fact that rapidly automated professions experience massive decreases in wages? And how do we know whether we're in a period of rapid transitions? Are there signals and metrics that you would recommend that we keep track of?
That's a great question. So the common experience across many rich countries right now is one of labor scarcity, and it's much more of labor scarcity among people who can do hands-on work in construction, in the trades. And this reflects partly the slow growth of labor force, but also the aging labor force. There's fewer physically able young people to do that type of work. So I think this is, and in the United States, we're seeing a decline in inequality right now as wages rise among young and less educated workers. They're following money everybody else, but they're not rising nearly as fast. What are the indicators? I think that the two things I look at all the time, first of all, are three things. One is employment levels. Do we actually see declines in employment rates? Generally, we're not seeing that. The other is, of course, occupational transitions, which categories are changing quickly. The third is wages. And it's often the case that there's a lot of flux, including among professional jobs, but it's much more adaptable. And so when there is change, they're much more able to reallocate themselves. Like my colleague, John Horton, did a study on Upwork asking what happened when flash programming was eliminated, killed overnight by Steve Jobs. The answer was that
People who did that work moved very rapidly into another form of computer programming. And that was a kind of so, you know, it's not that there's value, but they were able to quickly transition.
I mean, okay, this is a small follow up. But what do you think about quality? That was one of the things you mentioned in your conclusion?
Yeah, so what makes for quality?
So it's actually interesting fact, a lot of what makes for job quality is itself expertise. So people like jobs better, that actually require a level of specialized knowledge. And, you know, you know, yeah, so what one, why are people so nostalgic, for example, about coal mining, which is, you know, a very, it's actually, it's skilled, this work, that an elite group of people perform. And people are much more attached to that work than they would be to, you know, similar work in grocery stores, or in, you know, janitorial services. So part of what makes work good, actually command of expertise that comes both with intellectual rewards, but also social rewards, it gives you status.
You know, the second, and this is gonna sound very crass, but the other thing that really makes for good work is good pay. If you look at any statistical job satisfaction, the strongest predictor by far is whether people are actually well paid for the work, because they want security.
And then beyond that, and I think this is really is some degree of autonomy, and decision making and control, right. And, you know, another potentially perverse use of AI is to do a lot more monitoring, and in fact, rights, or, you know, or prevent people from, you know, engaging autonomously, or even bargaining. So I think that's a third dimension.
All right. I want to leave some time up for audience questions. But yeah, thank you so much for your presentation.
Thank you, China.
So members of the audience, if you have any questions, please feel free. Connor has a question for you.
Great. Thank you, David. Thank you so much. I'm Connor. I'm at NYU Stern School of Business. I'm the Dean of Students there. And I work with companies on training generative AI. And so I think you're just dead on about how AI sort of has to, you know, meet the challenges. I'm wondering if you've seen I'm wondering if you've seen, kind of going back historically, one of the big challenges that I think pops up, not necessarily that people are worried that there's that there are going to be fewer jobs or something like that, but they're worried that they won't be able to meet the new demand. So what I hear just the thing is, I've been doing this for 20 years is some hotshot coming out of college, who knows it's going to be able to take my job and the job of five of my colleagues. And I'm just wondering, historically, or even if sort of in your breadth of knowledge, how would you sort of think about that in terms of transferability of skills like that? Because again, often people aren't worried that, you know, they're going to lose their job, but more that they just can't jump on the next one, especially because generative AI is natural language and open. And I would say intuitive, but but do you know what I mean? Like, this is you have to learn a skill.
Yeah, no, I think it's a it's a very serious issue. The thing is, people are just not that fast at acquiring expertise. And it's quite it's possible for, you know, labor demand to change a lot faster than people can change their specialized skills. And I so I think there's, you know, it's, it's a it's a it's a quite a legitimate concern, right? And it's not that people will necessarily that will they will be unemployed, but in some sense, they'll be doing less expert work, right? You can always, you know, you can always get a job in, there's a lot of activities that would employ basically anyone who's, you know, sound mind and character, you know, sound mind, and, you know, sound health. But those don't pay well. And, and so I think, and, and, you know, we've seen over the last four decades, you don't have to look to the future for this, right? The decontenting of work done by people with high school degrees, people without college degrees, right? They did more meaningful, more skilled work, when they were working in offices and in factories, than they do in food service, or cleaning, or security. And again, I'm, I'm not saying that's not good, not socially valuable, but it's poorly paid. And it's less gratifying, I think, for the people who do it. And, and that's the concern we should have. And so I think, and it's quite possible to create a lot of displacement, and not even with a lot of social value in the process, right? So it's, you know, I'm very concerned for people who do translation for people who do, you know, graphic illustration, for people who do generic copywriting, I do not think we should just say this problem will take care of itself, I think it can be very displacing. And so the objective should be to, you know, to design work that enables people to use the expertise they have more effectively, right? So in other words, you could say, well, we could, you know, you know, a good example of this would be like, if you do skilled repair, or you're like, you repair aircraft engines, very specialized skill, you could imagine a set of tools, technologies that would enable you to use that skill more broadly, right to do a broader set of repairs to work on a broader set of specific machines, because you're in some sense, you know, you were in some sense, guidance in the guardrails to do that properly. And let me just give you like a super trivial example of this, right? So, you know, let's say I wanted to, you know, replace the, the fuse box in my 130 year old house with a breaker box. And I could go to YouTube, and watch a video. And, and I would surely electrocute myself or set my house on fire in the process of doing that, right? It would not actually be a good idea. But let's say I actually knew a little bit about electrical work, right? I knew how to, you know, wear, you know, insulated gloves, I knew how to use a wire stripper, I knew how to, you know, check, you know, voltage and conductivity, and so on. Well, then it would be complimentary to me, right, then having that same tool would actually enable me to use that expertise more effectively. And that's, I think, the, the goal, the design goal that one should be shooting for is saying, is there a way to enable the skill set to become valuable, because it can be applied more broadly, right? And, and many tools do have this feature, right? It's not, this is not a novel feature of the world, right? Most people are less valuable in the work they do, if they didn't have the tools they use for that work. But it is quite possible in this technology to do many things, right? Some of them true. And I did again, and I said this before, but I'll just say one more time. It doesn't choose its own objectives, right? The way AI is used for surveillance, the way it's used in medicine, right? That's not a characteristic of the technology. That's a characteristic of design choice, people who are building out the technology. And so you have to have this in mind when you're deciding what applications are worthy targets of the technology.
Great question, Connor. Thank you so much for that, David. Raymond has a question. And David, I also just want to share that Raymond shared a podcast with me and is ultimately kind of one of the reasons that led me to invite you to do this talk. So Raymond shared this podcast about your research that argued that AI might actually support the rebuilding of the middle class. So the middle class. So just to give you a little bit of an intro, Raymond.
Yeah, exactly. Thanks, David and Natalie. I wanted to follow up on that topic and see if I would imagine folks in your team at MIT are still researching that area. And is it still the optimistic take that we can rebuild the middle class utilizing AI? Or will we still have the sort of problems that we have with other technologies, such as computing? It took a long time for a computer to be trained on computers to then be able to work in those fields.
Yeah. So, I mean, there's a good... Rebuilding the middle class is sort of... I have to say that that
To the highly educated workers and effectively enable competition between workers without college degrees and people with college degrees because tools enable them to do more valuable work, whether it's in medicine, whether it's in design, whether it's in software development, whether it's in engineering, whether it's in skilled repair.
And that's the optimistic right? And I think, again, it's a design goal, right? It's not something that will happen themselves. I gave the example of the nurse practitioner, right? It's easy for me to imagine that 20 years from now, someone would go for a four-year college degree in medicine, right? And then they would obviously need to practice and develop that judgmental, translational expertise, but then would be enabled to be able to do many more procedures, formal specific training, each of them, because they would have a better set of tools, right? And this will be especially valuable actually outside of rich countries, where the scarcity of expertise is even greater for people to do software, to do medicine, to do engineering, right? So if essentially this technology can open doors to skilled work, which is the opposite of what we've done over the last four decades, that would be the good scenario. Thank you.
Okay. I think we have time for one more question. And David Evan Harris has had his hand up for a while. Thank you so much. David, from one David, love this talk. Really appreciated it. Really insightful. Maybe you had more slides and we just didn't get to them, but on the last slide, you made this reference to, you know, choice, industry and government and things that we can do to decide what this future looks like. And I wanted to prod a little bit more about that. I also kind of want to talk about Michael Polanyi and a little less Michael Polanyi. I'm sticking with Michael. You can knock all of this off. Okay. Okay. Well, we can put Carl and Michael in a cage fight later, but I think the question of like, whose responsibility is it to deal with this transition? Because even come 10 years, 20 years, 30 years from now is a good one. It's going to be painful for a lot of people in a lot of vulnerable communities along the way. So like whose responsibility is that? What do we do about it right now? Okay.
Yeah. So I mean, I think so it's very common and easy and people say it's our responsibility to take care of all those people. And I think that's true. And, you know, that's fine, but really the decision making about technology itself goes is much, you know, is not something we think of as under social control. And yet there's no reason to think that the incentives of the private sector are aligned with social incentives for this problem. It's not that, you know, it's not that not, you know, people in technology, AI have malintents, but there's no reason to think that what is being solved for in the private sector is the same as what we would want to worry about.
And there's where I think there's a big role for steering technology. And I think, but how do we do that? So, you know, universities have some role in this, but obviously that's not where most of the money is. Historically, you know, government has played a much larger role in this, right? Many of the sort of foundational technologies that we are all using in some sense, come out of government development programs, come out of the post-World War II era, when the Office of Naval Research was turned into the National Science Foundation, right, turned into this huge government R&D infrastructure. And still, like most of our health technologies, right, are come out of the government. I don't think we don't realize the degree to which the National Institute of Health, much, much, much larger than the National Science Foundation, right, develops a lot of the health things that we use now. So I think there's really a role for intentional design. And I don't know, you know, how we, enough incentive for that, right? So labor unions are really into this, right? They think, I agree, but that's, you know, how much leverage they have is limited. But I think some of this could be done. So it's easier to think of this in terms of specific domain, rather than in very, very broad brushstrokes, right? So you say, look, healthcare is an area where we have, you know, one in five U.S. dollars is spent on healthcare. We have a huge demand for healthcare. We have a huge number of workers in healthcare at all different skill levels, right? And all, like some of the best jobs in the economy and some of the worst jobs in the economy are all in the healthcare sector and a lot of them, by the way, isn't there a way to make healthcare not only, you know, better in terms of the absolute, you know, best care, but also to make it accessible to more people, both more patients, but also more workers. So there are more skilled work that could be done that isn't limited to a small number of extremely highly paid professionals. So I think you have to have, but that's the reason I say, well, why one example? Because then you can say, all right, well, let's build that out, right? Let's think about, you know, like for, you know, one of my colleagues, Julie Schaub, likes to say, you know, the charge nurse on the floor of an ICU is like an air traffic controller without a radar, right? This is an incredibly hard, complicated job that has terrible tools. And why couldn't nursing be elevated, right? With the right set of tools to actually a higher, a better status, better paid profession that more people could do effectively, and we could change the way we care for workers. So I think, and you could say this in education, how do we redesign education to change the role, the division of labor between machines and people to, you know, make it less expensive and to make it, you know, more engaging. So I think it helps, at least for me, to think in terms of specific examples. I don't think there's a general answer.
I will say, you know, I'll say very briefly, you know, people always, you know, you say, well, look, I understand what we do about global warming, right? We reduce carbon dioxide, right? I'm an engineer. You tell me to produce a product that, you know, has less, you know, global warming effect, I just give you something that has less carbon dioxide output or input. Good, right? How do we do that for AI and work, right? What is our carbon dioxide measure? And to me, the design principle is this enables people, someone with less formal skills and expertise to do more valuable work. And if we can actually measure that, that is a goal that you can actually, you know, concretely accomplish or at least attempt to accomplish because you know what you're looking for.
Thank you, David. Great question, David. Well, that was wonderful, David Autor. You are welcome back anytime. That was a really lovely and the audience has been so engaged. We're so grateful that you joined us today. And Taina, thank you so much also for bringing your thoughtful questions. It was really lovely, guys. It was really one of our most fascinating talks so far. I think we all really enjoyed it. Thank you guys very, very much. I really appreciate the engagement. And thank you, Taina, very much for your questions. I really think we're going to need a follow up, David.
So before everyone takes off, just a few announcements. Next week, I will see most of you here in San Francisco, August 24th, 6:00 p.m. at OpenAI. If you've registered, we've sent you all of the address details. Colin, I know you're kind of far away. So we will see you at the end of September for a different outing. September 7th, which is only a few weeks away, we'll be hosting our CEO, Sam, and the CEO of Helion. And we'll be talking about the future of energy, specifically fusion energy, also in person at OpenAI, but we'll record it for folks to see the presentation later. A little bit later in September, I'll be hosting my colleague, Shabani Tuker, and she will be presenting her research, Whose Opinions Do Language Models Reflect? I think we'll all be really interested in that one as well. And last but not least, in October, we'll be hosting virtually a renowned mathematician who really needs no introduction, Terence Tao, founder, Ilya Suskiver. So one of our founders, there are a few of them. I hope that we continue to bring presentations that engage everybody and really resonate with what we're interested in. But the only way to do that is if you know what's on your mind and what you'd like to hear from the community. I hope you guys have a wonderful Thursday evening. It's so lovely to see all of your faces. And I will see you again soon.