AI Literacy: The Importance of Science Communicator & Policy Research Roles
speakers
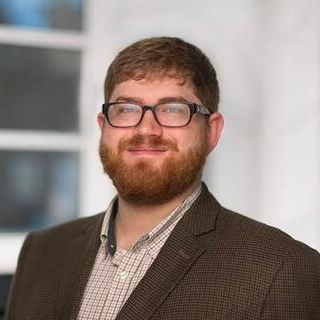
Miles Brundage is a researcher and research manager, passionate about the responsible governance of artificial intelligence. In 2018, he joined OpenAI, where he began as a Research Scientist and recently became Head of Policy Research. Before joining OpenAI, he was a Research Fellow at the University of Oxford's Future of Humanity Institute. He is currently an Affiliate of the Centre for the Governance of AI and a member of the AI Task Force at the Center for a New American Security. From 2018 through 2022, he served as a member of Axon's AI and Policing Technology Ethics Board. He completed a PhD in Human and Social Dimensions of Science and Technology from Arizona State University in 2019. Prior to graduate school, he worked at the Advanced Research Projects Agency - Energy (ARPA-E). His academic research has been supported by the National Science Foundation, the Bipartisan Policy Center, and the Future of Life Institute.
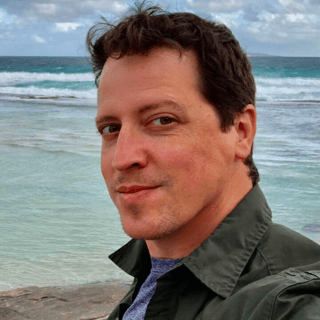
Andrew Mayne is a Wall Street Journal best selling novelist and creator. He was the star of A&E television's Don't Trust Andrew Mayne and swam with great white sharks using an underwater stealth suit he designed for the Shark Week special Andrew Mayne: Ghost Diver. He currently works as the Science Communicator for OpenAI.
SUMMARY
The Importance of Science Communicator & Policy Research Roles with Miles Brundage, Head of Policy Research at OpenAI and Andrew Mayne, Science Communicator at OpenAI
TRANSCRIPT
I'm Natalie Cone, OpenAI Forum Community Manager, and I want to start our talk today by reminding us all of OpenAI's mission. OpenAI's mission is to ensure that artificial general intelligence, AGI, by which we mean highly autonomous systems that outperform humans at most economically valuable work, benefits all of humanity.
Today's talk, AI Literacy and the Importance of Science Communicator and Policy Research Roles, will be delivered by Myles Brundage and Andrew Mayne.
Myles Brundage is a researcher and research manager passionate about the responsible governance of artificial intelligence. In 2018, he joined OpenAI where he began as a research scientist and recently became head of policy research. Before joining OpenAI, he was research fellow at the University of Oxford's Future of Humanity Institute. He is currently an affiliate of the Center for Governance of AI and a member of the AI Task Force at the Center for New American Security. From 2018 through 2022, he served as a member of Axon's AI and Policing Technology Ethics Board. He completed a PhD in Human and Social Dimensions of Science and Technology from Arizona State University in 2019. Prior to graduate school, Myles worked on the Advanced Research Projects Agency and his academic research has been supported by the National Science Foundation, the Bipartisan Policy Center and the Future of Life Institute. Welcome, Myles. I think that policy research is some of the most important work related to the second piece of our mission, ensuring that OpenAI's technology benefits all of humanity. And I'm so grateful that you joined us here today. Thank you for the kind introduction.
Next up, Andrew Mayne is a Wall Street Journal bestselling novelist and creator. He was the star of A&E Television's Don't Trust Andrew Mayne and swam with great white sharks using an underwater stealth suit he designed for the Shark Week special, Andrew Mayne, Ghost Diver. He currently works as a science communicator for OpenAI. Andrew, what an honor to have you here this evening. Thank you. I am so excited to have you here and I am going to be taking my notes from you. I know that you have been presenting and educating folks for a long time, so I was really excited to have you here today. I'm actually going to be taking notes on on the way that you present yourself because I know that you are an absolute seasoned professional. Thank you. No pressure at all, Natalie. No pressure at all.
So now, everybody, I'm going to hand the mic over to Myles. And just as a reminder, we'll hold all of our questions to the end of both presentations. And Myles, take it away.
Great. Thank you so much. Let me just pull up these slides. Hopefully that looks good. Yeah. So thank you so much for inviting me to speak. The topic for my brief presentation will be AI literacy and AI impact. So what do these things mean and where does my team and kind of OpenAI fit into all of this?
So I'll start with my role at OpenAI, which, as Natalie said, is leading a team called Policy Research. I'll give one definition, a little bit of a sense of how I think about AI literacy, though there are many different ways of slicing and dicing it. And Andrew will be giving his perspective as well. I'll give some reasons why AI literacy is important from my perspective as a policy researcher. And then I'll give a quick overview of a couple of things that OpenAI is doing in this space.
So what does it mean to do policy research? Essentially, that means understanding the impact of OpenAI's technology on the world and identifying good ways to shape those impacts. And AI literacy has come up time and again as one of the key variables influencing those impacts. And so this is just one example of where those connections come up, which I'll revisit later in the lower right is the recent paper that my team and some external colleagues put out on the labor market impacts of AI, which might be significantly affected by how people actually understand and adopt those tools.
So what is AI literacy? Well, there isn't really a standard definition that I'm aware of, at least. But one common one in a seminal paper is a set of competencies that enables individuals to critically evaluate AI technologies, communicate and collaborate effectively with AI and use AI as a tool online, at home and in the workplace. So the idea is somewhat analogous to literacy and numeracy. And you might see my cat in the background, if you can still see my face. And they're basically the ability to use AI and do so safely and responsibly.
There are a couple of reasons why this is not trivial. One is that the capabilities of AI systems are improving. So this is an example from the GPT-4 blog post. So you can see in the green is the performance of GPT-4 on various benchmarks listed at the bottom. And the blue is GPT-3.5. So as you can see, the green is often much larger than the blue. And the blue wasn't actually that old of a technology. It was just a year or two earlier. So the theme here is that on human relevant benchmarks, such as passing certain tests that students are required to take, there is significant progress happening in our lifetimes, which can make it challenging to give an up-to-date sense of where things are and where they're going.
Another reason why this is challenging is that the features of AI-related products are also rapidly changing, not just the underlying neural networks or AI models themselves, but also other non-AI components like we're adding various tools and plugins and such as the ability to browse the Internet, use calculators, et cetera. So it's difficult to say, well, this is, you know, this AI system is not the same as a browser. It's different in X, Y and Z way. But then, you know, the next day we add browsing to it. So it's very much a moving target.
Another reason is that AI is deployed in many contexts, which raise distinct challenges. So these are just a few examples of areas where GPT-4 has been used. And then lastly, the errors in AI that AI systems make can be very subtle and difficult to notice, even if you're an expert in the domain or an expert in AI.
So why does all of this matter? One reason is getting the most out of the technology at a personal level and avoiding essentially shooting yourself in the foot by misusing the technology or using it in a way that it shouldn't be used, or conversely losing out on opportunities to use the technology that might be beneficial to you. So this is just an example of a lawyer who used chat GPT in a way that, you know, did not set him up for success because it, you know, made some errors that he did not realize.
Another at a higher level, so not the individual level, but at the societal level, getting the most out of this economic transformation at a societal level also requires people to be AI literate. And this is just an example of a case where there are people who are transitioning between jobs. And you can imagine that similarly to, you know, how in the 20th century, a lot of people transitioned from agriculture to service work, partially enabled by literacy and numeracy in the traditional sense, not AI literacy, but, you know, literally the ability to read and do math and those so forth helped people move into new professions. A similar situation might be true today.
And the last reason is that it's important to have informed public debate and policy making. And so it can be, you know, we might ultimately make the wrong decisions at various levels with different institutions, including governments, if people don't understand the nature of the technology.
So given all of this, it's important for OpenAI as a leader in this field to put its best foot forward here and try and lead the way on AI literacy. There are a couple of different things that we're doing. And then I'll turn it over to Andrew to talk about some other aspects of this.
So one is disclosing limitations in the product. So this is an example of the interface to chat GPT. And on the right hand side, you can see examples of limitations in the technology, such as generating incorrect information, biases and limited world knowledge. Publishing more detailed analyses, so not everyone is, you know, so everyone who uses the technology will see some disclosures, but not everyone will read these lengthier documents. But that can be helpful for the research community, policymakers, other companies and so forth. So there's both the kind of expert targeted and the and the more general public facing communication that we do.
And then finally, conducting research on AI literacy itself and different ways of improving it. And so one of the things that my team is hoping to do in the coming months is to build more of a scientific understanding of how.
Different interventions and different ways of communicating the nature of AI help, and kind of in order to build useful mental models so that people can have meaningful oversight over the technologies that they're using. And that, in turn, requires, you know, some kind of things to test different ways of communicating that you can then iterate on. And so, hopefully Andrew can tell you more about that and I will turn it over to him.
Thank you, Miles. It's awesome to be here and part of the reason is that I recognize some of the people here and one reason I was able to get into AI was because of the work other people have been doing in science communication in particular. Jeremy Howard, the fast AI course, you know, when I wanted to get into this field and I knew very little about it, things like that were just wonderful. And, you know, thanks to so much of the stuff that's been out there and just people who've been very thoughtful in communicating, it's been great.
As Natalie pointed out, I have a very unconventional background as far as, you know, where I came from originally. Way back in the day, I was involved in critical thinking education. I worked with the Annenberg Foundation. I did a lot of that. The whole TV thing was actually trying to create an educational television series. And one thing led to another and I'm swimming with sharks and doing prank shows. But my heart was always in that and it was great to be able to come here.
I started at OpenAI back in 2020. I was the original prompt engineer when GPT-3 came out. I started playing without that. It was fun. And I also started writing the documentation for it because here's this really cool technology and it's a very fast-moving company and there wasn't always a lot of time to stop and try to break these things and explain it. So, that's where I stepped into that and did that for, you know, both creating prompts and doing that. And then two years ago, I became the science communicator for OpenAI.
And, you know, my job is to basically talk to really smart people like Miles and have them explain things to me and then simplify them to me and then simplify them again. And then I tell other people and then I get credit for it. So, it's really just the benefit of working around incredibly talented people who are very eager to communicate.
So, let me go ahead and share. I'm going to, I have a few slides here, but I'll probably go through some of them a little more quickly than a couple others because Miles has touched on some great points and I'll just call out things I think are worth pointing out.
So, this is my title card. That's super helpful, I guess. But science communicator was a role that was created two years ago by our former head of comm Steve Dowling from Apple and realized that as we move so quickly, as we do this stuff, we really do need to engage and communicate with people and not just wait for the media, wait for educational resources to catch up. We need to be proactive because we care very, very deeply about how these things are used. The people at this company I think are very special and that we really want to make sure that the success of AI means success for everybody. And the lunchroom discussions, the conversations we have are about how do we make this thing a broadly beneficial thing. And so, we're very unconventional in that regard.
So, what does a science communicator do? Part of it is I work on our storytelling and communication. I use text. I use video. I'll use code and whatever examples I can. Also, what I do is I'll do background. I'll go talk to the media. I'll do one-on-ones with reporters. Let's say they want to talk to some of our scientists. They want to understand how something works. I can go through there and give them a briefing. They want to know what a transformer is. They want to understand some of this technology. Then I try to help them understand that because if I can do a good job of communicating to journalists about how this works, then they can do a good job of communicating with their audience. So, that's a super critical part of this is understanding how to communicate with other people who are communicating to other people. We also work with policymakers and decision-makers and we give them background. And this means maybe giving briefings to somebody might be, you know, we have conflicts for other countries that come here and we have them sit down with our scientists and let them talk to them and hear it. We don't really have like, you know, we don't try to put out spokespeople. We try to put real people doing the real research talking to other people, which I think is one of the things that's critical here.
You know, what are the challenges in AI? As we've talked about, everybody's a stakeholder. Everybody. It doesn't matter where you are. It's going to affect you. And people need to not just have a stake and be able to have a part of the conversation. Part of that means helping them understand what's going on. And that can be challenging given that there's a lot of confusing things. You know, is AI implementing too slowly or too quickly have costs? That's one of the challenges there. You know, AI is moving fast and important concepts appear every day. And that's part of what my role is. You know, a year ago, a diffusion model, what did that even mean? Generative AI? You know, that's a term that's just become very popular very quickly. But these things are all of a sudden out there. And with, you know, Chat GPT, things like these terms is just all of a sudden, you never know what's going to come next. And so part of what I have to do is figure out how do you break down what was high-level research a few months ago to something that might be in everybody's pocket tomorrow. Predicting the future is very hard. You can make all the predictions you want. Reality has other plans. We joke a low-key research preview. That's what we call Chat GPT internally, because that's what we thought. Chat GPT inherently was built on top of this 3.5 model we had. Its capabilities were approximately the same. We didn't realize what was going to be the impact when you made it, one, easier to use using a thing called reinforcement learning of human feedback, and then put it into a chat interface. And, you know, we have the best predictors in the world, I think, when it comes to trying to predict, like, what's going to happen with capabilities. But this is a situation where we didn't realize really how ready people were for something like this, how people were, but also see how unprepared people were for the impact. And that's kind of the give and take. You know, it can be wonderfully beneficial, but it can catch other people off guard. And some people are like, why didn't you tell us? It's like, it's hard, you know, but we really, really tried to do a good job. We spent a lot of time, you know, GPT-4, we spent six months on that, just testing it, trying to figure out what it was going to be, working on how we're going to communicate it, etc. Things move fast.
GPT was an obscure term. Now it's a comedy punchline. We just heard in the recent debates, even though most people don't even know what GPT stands for, it's generative pretransformer. I know that explains everything
Show somebody an incredible tool, but then people will go, what does this mean to me? What does this impact me? And that's a thing that we're constantly dealing with as trying to navigate that world where we look at these things to amplify, but we gotta be very clear about that. They're not replacements.
So how do you measure your success? It's hard. It's hard to know if you've succeeded what you're doing in science communication. In the case of the Dali video, that got used. We watched the media pick that up. We watched clips of there, and I'll show you, we went through different kinds of examples.
How do you explain how a neural network works? How do you understand, help people understand the association between text and images? And I'm not saying we nailed it, but I would say the graphics and things we used kept getting reused over and over and over, which I felt like, okay, that was a win, that the examples we use to show people are being used by other people. And when you repeat something somebody else said, that's a pretty good sign that it's useful.
So another case study using GPT-4 for content moderation, not just GPT-4, we did a video on GPT-4 and all that, but we had this team that built a really cool tool where basically what it would do is it would help content moderators moderate content, but try to make their job easier because moderating content, not a fun job often because you have to deal with the worst of the internet. And the more you can isolate people from the worst of it, or at least allow them to do a little bit of work and have a big impact, the better.
So we built this tool that uses GPT-4, it uses content, and it's sort of a multi-step process, but we wanted to show this to people because this is going to be a widely used method, at least for us and other partners of ours, for how content is going to be moderated. We didn't want it to be this black box to say it just works. So we had to work through and figure out how to do it. It's a technical method, but we wanted to share it because we wanted other people to use the methods and we wanted to show the public some of the ways AI will be impacting them. And we'll link to, I can link out to the video and you can watch it and sort of see, but it's a much higher level technical video than I think somebody would normally be doing. We wanted to do that because we want to get ahead of it. We want to get ahead of it to show how these things have this sort of impact.
So this was an example of a graphic from there. Basically, we had to figure out what it does is basically we have somebody who decides what a policy is for judging content. We have GPD4 make a prediction. We compare to see how accurate its prediction was versus the other one. Then we tweak the policy. We generate a bunch of synthetic examples and we go through and we have to sort of find these helpful visual metaphors and stuff to make it. And out of context, this probably means nothing, but the idea is that we had to spend time to think about how do you communicate it? It's one thing to put into words. It's another thing to put into images. And when you get the right image and graphics and text together, great things happen or it gets very confusing.
So what I've learned so far is ask the people who create and work with the technology, explain it to you. One of the really awesome things we have here at OpenAI is we have a channel called Explain Like I'm Five, ELI5. And what that is is you can go in and ask any question. You can ask a question anonymously if you want, because sometimes people are afraid. In a technical company like this, you get people who come in and they hear all these terms and jargons and they're afraid to ask. They quickly learn. Everybody is trying to deal with the jargons and the terminology, but also we're an extremely open company when it comes to teaching other people and helping people understand.
But we have this channel and that is a great way because we don't want anybody to think that something's too stupid or too whatever. I've used that channel a lot and having the smartest AI experts on the planet explain things to me, I mean, that should have been like one of the benefits when I first came on board, they listed, and that's been great. So that's helpful.
So I would say one of my pieces of advice is whatever group you work with, create an easy channel for people to ask questions. One of the things I do when we onboard people who come in, let's say non-technical roles, maybe they come in in some capacity, they're like HR or finance or whatever. I reach out to them. I tell them like, if you have questions, come to me because I probably have the same questions. And if you're in a meeting or whatever and somebody mentions a term and you're going, what the heck is this? Just ping me. If I don't know, I'll find out for you. Because I just want people to feel not afraid to ask because you can be overwhelmed by all.
How do I work things out? One, I explain things to myself. I break it down so I can explain it different ways and I keep trying to do it. And that's been, I came in from a very different background into AI. And so part of my ability to function here has been the amount of time that I spend reading the paper, breaking it down, breaking it down again, looking things up and going over there. I wish I could tell people like, yeah, I just got it intuitively. I just read it and get it. But that's not the case. And when I talk to researchers in other fields, who I find out like, oh yeah, that's the same thing for them. They have to figure out what's up some domain, which may feel very similar. They have to figure out like, oh yeah, what this is. So just the ability to constantly break things down is so helpful.
One of the things I try to ask, who will be impacted this? What questions will they have? The artist outreach was just a great example. Natalie Summers, who is on my comms team, she did a big effort to just go reach out to thousands of artists and talk to them. What is this stuff gonna mean for you? How are you going to use it? And she takes that, one, helps inform me in my communications role, and goes back to the researchers and helps them understand that. Because it's not just communication being one way. We just communicate to the world. It's going for the world back in. It's trying to understand how people are seeing this. And that's a big part of our job here, is to pay attention and to listen to people. Not just about what they're afraid of or whatever, but how they wanna use things, how they wanna implement what they wanna do.
We just released a post today about education in AI using ChatsGPT. And we've heard a lot of concerns about cheating and other stuff, but we went out and we talked to 20 teachers around the world to find out from them what their concerns are and whatnot. And cheating wasn't a big concern. Actually, teachers told us that it's the same as if a parent was doing a kid's homework. They could tell, they know the student, they know what's going on. They weren't worried about that. There was, one of the big bits was people working in other languages. They said it could help improve their English skills, but on the other side of it was, they would like to have these systems be more native to their own languages. Because one of the advantage of these things is that we take for granted in this world, the fact that we have textbooks in every subject and we have all these materials, educational materials in other parts of the world aren't as abundant, they're not as accessible, and these tools can help with that. But to make them better tools, we need to hear from people, them telling us what the
Any burning questions they'd like to throw out there?
Jai Wei. Hi, I'm Peter, and I'm the receiver of the Democratic grant, and I'm in the scene of regional recursive public, which is held by incorporation of the Chattanooga Housing in the United Kingdom and vTaiwan based in Taiwan. So I'm based in Taipei right now. And I want to echo to what Andrew says, is that reach out to your audience and also find ways to communicate with the audience like that. Because I think just yesterday, the Taiwanese government just released instructions about how to use the generative AI in government agencies. And due to these kind of news release, the PBS in Taiwan, public broadcast station in Taiwan, asked me to, invite me to go on a TV show, which is using the Taiwanese to introduce the AI, which is more challenging because I used to use English and I used to use Mandarin to explain AI issues. But right now I need to use Taiwanese. I can share a video with you guys. If you want, you can just check it out. And it is the first time my family told me that, oh, I really know what you're doing. I really know how you're looking into it, like these kinds of things to happen. But I still have one questions for Miles and Andrew, because in what we are doing right now about the democratic input to AI, we find out that one challenge is that it is very hard to explain the full context toward the stakeholders to about the AI. And we afraid that if we are choosing a certain specific ways to explain to that, maybe we are actually setting up an agent for them and cannot make and kind of like influence their own opinion by the agent setting. So how to avoid these kinds of things when doing these kinds of scientific communication and also to elevate the AI literacy. And other things is that, I wonder how to overcome the challenges that maybe for sometimes, if you want to explain to others, you need to simplify these kinds of things, how to simplify the things, but still remain accuracy when you're explaining AI related issues with your stakeholders you want to reach out. Yeah, this is the question I want to ask. Thanks a lot.
I'll address the last one if Miles wants to take the first one. And I would say that, the kind of my rule of thumb on breaking things down is to say, if I break it down so much, can somebody else make an informed decision with that? Do they have an understanding? One of the metaphors I use to describe AI systems is like prediction machines and say that, it has data, it learns on it, and it tries to predict an outcome on that. So that's helpful to somebody when they see something and the model gets it wrong, instead of them going, oh, it's broken, they go, oh, it figured out of the realm of probabilities. This was one of the answers and the other few times that it got right. And then to help some understand like, okay, if I ask it again, that'll get it better. If I give it a little information, it improves it. So is the instruction, is the explanation useful enough for them to make, help them? Can they make a decision? Can they do something from there and do that? And also not, you also don't wanna make it where it's deceptive. It's very tempting in AI to just tell people like, oh, it's perfect, it's great, whatever, just take it. We don't, you don't want that. You want people to understand and to read the label before they use it, which is very, very, very critical. So I try to break it down to the point that I know that they can then do something useful with it.
Yeah, I'll add on the first point about kind of like making sure people don't over-index on a particular usage. We try to emphasize the generality of the technology. So Andrew mentioned that like prediction machines framing, I think like emphasizing that AI is a general purpose technology using, sometimes analogizing to things like electricity that have a lot of applications instead of just focusing on one particular use case or using lots of examples. Those are some ways to help. I also think like, you know, in addition to the, you know, the videos and explanations and so forth that Andrew and others produce, I think also just encouraging people to get direct experience is like, in some sense, the kind of long run solution there because, you know, we've tried to build the technology in a way that encourages trying different things out. So when you use chat GPT, it'll encourage trying different kinds of applications. So I think, you know, just nudging people in that direction is one of the ways that I think people can, you know, get a sense of the technology as a whole rather than just, you know, one particular application.
Excellent question, Peter. I'm so glad you got to be introduced to them tonight. Does anybody else have a question for Miles and Andrew?
Oh, we have a few. Lex.
Thanks, Natalie. Andrew, you mentioned that I think someone on your team had spoken with artists and I was curious to know if that was published or if there was anything you could share from those, not perhaps to this whole group, but I would be interested in seeing what came of those conversations.
Well, it's partially, it's been an ongoing one. Like we have, some of those artists are now in the community here. And so basically it's been an ongoing sort of thing and sitting down, part of it gets reflected in sort of the way the product is, the way we frame things and whatnot. I don't know that we've done a specific, you know, you know, a specific sort of blog post on, I think we put input in there and some of the blog posts like mentioned some artists or how they're using it. So you can certainly see that. But it's a, it's a not a one-time thing. We just go in and we do a survey and we go, cool, we get it. It's the goal is it's like, like this community exists, an ongoing conversation because first we wanted to just sort of, before we sprung Dolly onto the world to sort of say, hey, let's talk to people who are gonna be impacted about it and see it and then help that understand us how we wanna position this and whatever. And so, yeah, it's basically sort of an ongoing thing. So you can kind of see that in our work.
I will definitely connect you Lex to Natalie Summers, the colleague that Andrew was referring to. And you guys can jam on what that process looked like. And as Andrew mentioned, it is an ongoing process. So we would love to weave you into the fold of all of that, given all of your background in the arts. That would be great. And then Miles, to your point about just getting the experience, something that you've sparked in my mind and that I've heard from community is, enthusiasm to understand how to use AI when applying for resources, like a grant for a public art project or an artist applying for support in some way. And on the grant making side, needing to be very thoughtful about people understanding the tools and what are the right guidelines. And so that's another thing that's on my mind and I appreciated your talk in that regard.
Yeah, I would just say definitely like, I think Andrew has also been talking to some teachers who've found applications like that kind of grant writing. And I mean, in the kind of medical domain as well, there are lots of applications that people come up with that you wouldn't necessarily naively expect and you just get from like, interacted with it. So I mean, people might think like medicine, AIO, it's like diagnosis, but actually like things like writing a note more empathetically is something that has come up a lot. And it's just kind of hard to anticipate those things or come up with them without just kind of building a mental model of what you can do with it and then going about your daily life and saying, oh, this is where it could help.
And speaking of, I'm sorry, go ahead, Andrew.
Yeah, I just had to add on to that too. It's like that to Miles point, like, yeah, very often, when I'm sitting here trying to think of examples, I'll have probably a model, somebody that looks a lot like myself in that world and that's not the rest of the world. We did these surveys and one of the things that was really interesting was a school in Palestine was able to get four more grants this year than they'd expected. And part of the reason was, because they are using chat to help them write slightly more professional grants. And one of the things is, is that we often overlook in the grant writing world is people who can hire really good grant writers get a lot of grants and they don't always go where you want to because really under-resourced people in organizations don't have often the ability to write, to bring in top tier grant writers. And that was the thing that like, oh, this is a really cool kind of equalizing technology. Now, if somebody sits down to do this, this is kind of really cool.
Exactly. You just touched on what I wanted to share, Lex, is that we actually have the head of development for Larkin Street Youth, a nonprofit in San Francisco here, Gail Roberts. And one thing that we've observed over and over again in our conversations in the forum is exactly that. Like how can nonprofits leverage chat GPT and other AI products?
Colin:
- So I'm with the team working on democratic inputs to AI. And we're getting some really interesting results here. We're looking at medical policies at the moment and public acceptance for medical policies. And we found already that there's a correlation between that and people's predisposed attitudes towards AI. If they're kind of positive about AI, then they're more inclined to be accepting the policies that we've developed for medical issues and vice versa. So I guess the bottom line here is that to get the whole thing working and to get policies accepted, there's going to have to be a general acceptance of the sort of proactive approach to positive attitudes towards AI. And I'm just wondering, well, you don't want to go into a hard sell on it because that could be counterproductive. But clearly, public attitudes are going to affect all the way through to detailed stuff about AI. So maybe positive stories could be very, very helpful. Certainly, they help. But I want to double down to what Miles said, is getting people to use it is really, really critical, and both to see what it's great at and to see the limitations. And when you can get people to use it and understand the context of it, whatever, once you get over the weirdness, it's good. And I think that that's a thing. It's like, we just put this post up today showing how teachers are using it in education because we want people to see there's a lot of positive things out there. And we also have the warnings and things like this that help you with that too. But if you give people the tools to sort of use it and experiment with it and limit the downside, I think it's great. And I think the stories like this are exciting and they're empowering. The grant writing stuff is such a great news case because there's going to be a person in between there, the chat GPT in the world, taking a look at the grant, deciding they should send it or not or whatnot. But yeah, I think that the goal is to encourage people to try it, but give them the information to know what's going on. Thank you so much, Colin.
Natalie:
- Thank you so much, Colin. And Colin, are you going to be in town September 28th and 29th with the other grantees?
Colin:
- Absolutely, yes. And I'm looking forward to the sail in the Bay.
Natalie:
- Yes, there's a sail in the Bay. And there are extra spots for OpenAI and forum members. So Miles, Andrew, anybody here that might be interested in joining us for a couple of hours outing and meeting Colin in person, just DM me and we can make some space for you so you can meet Colin in person. Thank you so much for your question, Colin. I'll be there too. I'll be flying in from Austin to hang out with you guys.
Greg:
- OK, Greg, thank you so much for waiting. Your hand's been up for a while.
Greg:
- All right, can you hear me OK?
Natalie:
- Yes.
Greg:
- OK, great. Very nice to meet you, Miles. And nice to see you again, Natalie. Just briefly about what we do, and then I have a question on how to approach this problem. So we are a life science generative AI company. We've been around for about six years. We've had an early partnership with OpenAI. And so our customers are global pharma companies, medical diagnostic companies. Think of what we do is this automated researcher that goes through both public and private data and answers questions for those who need the analysis. And it takes a process that usually would take about six weeks and turns into a five minute process as it scans all the data. It's already in production. We're coming out with a paper that talks about how we do this. And so it's very exciting for us. Our co-founder, Lotta Fong, who came from Precision Medicine, she started this because she was so frustrated. She had access to all the data, but she couldn't get any insights out of it. So we're very excited because it also is transparent. It shows you all of the analysis, all the citations, all the deliberation, and all the explanation of how it generated these answers. The challenge I see, and this may sound like a negative, but it's actually something I'm concerned about, is in doing research the old fashioned way, the manual way, you learn things. There's something that goes on in the brain as you're going through this. It's a painful, arduous process. I still think our process is better. But a downside is there's probably a lot of thinking that goes along the way in doing the research that now is at your fingertips. How can we then transfer this? Now that we've done sort of a lot of the manual work, how can we transfer this and make sure that people are absorbing the research that is done?
Miles:
- Yeah, it's a great question. I don't have a good answer, but I'll just note, I'll say a little bit about how I think about the issue. I think it's not just a matter of medicine or research, but more generally, the question is, how does AI affect skill development and skill retention? And is there a risk of it changing, or risk or opportunity of it changing kind of the pathway into different professions? And what is considered a requisite skill for that profession? I think we'll probably see some of those effects earliest in cases where there's been the fastest adoption. So for example, like GitHub Copilot and software engineering, what is considered essential knowledge and what you're able to do on a per person basis has changed a lot over the past few years. I don't know of an easy solution, but hopefully as we kind of incorporate AI into education, we will increase the emphasis and not lose the emphasis on things like co-thinking and understanding that what is going on under the hood, even as we're using these powerful technologies. But yeah, I mean, that's not a very satisfying answer, but I would just say it's definitely a serious one. I see, I use the GPT to code a lot. I like to make little apps and stuff because I'm into memory methods and stuff. And I do get to the point sometimes where it produces it, I just copy paste it and I do the horrible thing you're not supposed to do. I don't even look, I just see if it compiles. And sometimes I feel guilty because, oh, it worked, it's great, like, I don't know what each line did. I know what this, I'm like, oh, but then I thought about it one day, I'm like, you know, in code, you have these things called libraries. And so basically, if you wanted to do stuff with data, you use the pandas library, which is data frames and stuff like this. I have no idea what that's doing. All I know is I give it a CSV, it's not the best case. And all of a sudden, it makes it very pretty and very nice. So should I feel guilty about that? Maybe, but I would say that for the amount of time that I'm saving by not looking at what's going on over there, which is a bad practice, I'll just say that, I am spending doing deep dives and do other stuff, though. I am spending going much, much deeper into topics and stuff and saying, hey, what do I know about this? Cool, well, let me go look that up, make sure it's real.
Oh, it is. It's cool. So I would say that like a lot of things, uh, abstraction, you know, the guy who flies your plane, a little one who your co-pilot, whoever, they have a basic understanding of how the jet engine works, but they don't know a lot about, you know, they know a certain thing about fuel mixtures, but they're not gonna be able to fix it. And that is scary. It is the idea that we're building a world of complexity that might be too complex. And cause when things break, they might really break in that world. But it is sort of the way we sort of built on top of it is, you know, we, we passed the point of, you know, a hundred years ago or so, where somebody could understand basically how to build everything from a cathedral to, you know, um, a wagon. And so knowledge is going to get like that, but you know, as Miles said, these tools can be helpful for us to sort of then break. We have the most patient tutor in the world now, because if I say, you know what, I want to know everything down to where the Silicon was made, who mined it, whatever, that's the other side is now we can get an opportunity to work and dive deep. If we choose to.
Yeah. Andrew, thank you. And thank you, Miles. I think that was my house. I actually asked Chad GPT this very question before the meeting. And, um, and so my thinking is to build in knowledge retention, targeting humans so that if they're interested, they can go ahead and take what they're looking at and really try to help them deeply understand what the analysis they've found done.
Yeah. Thank you. Thank you very much for this. I really appreciate both your feedback on this.
Yeah, of course. And I would just add one thing is that like, you know, I don't have a neat solution for every domain, but there are definitely some cases that we can learn from like, for example, airline safety, where, you know, over reliance on autopilot is like a known issue and people did studies on it. And then they learned that, you know, occasionally handing control back. So people keep their skills fresh is, you know, one of the things you do.
Great. Thank you both. I really appreciate it. Kara, you're up.
Thank you. Thank you so much for your presentation. Um, this is eye opening for me. I was very interested in this conversation. My name is Kara Ganter. I'm director of digital education at the graduate division at UC Berkeley. And, um, you know, as I'm listening to you speak, I just have a few reflections and then a question or some comments, you know, I, in my role, I serve as kind of a science communicator. I'm realizing that now I've always seen my role as a translator. I have a JD, I got involved in technology in law school and came to UC Berkeley in order to launch online programs, use technology and teaching, help faculty modernize their instruction and to meet better meet the needs of, of, of the current generation of students and their launch into the world. And so, um, right now faculty at UC Berkeley and higher education institutions across the country and world, they are in a tailspin and they're looking to people like me who, um, who is, are really the train, the trainers train the faculty on how to use these tools. And then an additional layer beyond that is to train the students who are entering into the economy in which AI and chat GPT and tools are going to be ubiquitous. And they're going to need to need to know how to use these tools to get jobs and survive in the world. So, um, you know, just, you know, Andrew, you were saying that, that you've used methods on getting people's hands on the technology. That's just the best way for them to understand what it is. And then meeting with Berkeley law faculty today on a chat on envisioning a chat GPT workshop and teaching Berkeley law faculty on how to teach students. Um, you know, I said, well, here's what we're going to do. We're going to take your assessments and we're going to sit in front of the, all of you faculty, a hundred, a hundred faculty, Berkeley law faculty, and put in your assessments and see what it says. And then we're going to workshop it together and see, you know, what, what the results. And so demystifying the technology, getting their hands on it, but also providing that, you know, the materials to break down the tools is kind of where, you know, is where I'm struggling because I'm not an expert in chat GPT. Um, how do we find the resources, you know, those of us who are training the trainer, the trainers, like the meta, how do we learn how to accurately describe these technologies that are changing weekly in a way that is accurate, um, and allows them, gives, gives our faculty the power to, to use them in a way that's pedagogically sound and also train the students who are heading out into the world and in an AI or world of AI.
Well, you, you're on a great path. I mean, the challenge is, is that, you know, we're looking for textbooks for something that's not even a year old and it is, and it's a challenge. Amazon's filled with them now by chat GPT. But you know, one of the things you said that I think is really keyed on was workshop together. One of the things that when I talk to companies, I talk to other people that want to say, what do we do? I'm like, one, play with it. As we said to create a channel, create a form where you can share, where you can go back and forth because the process of isolated, I'm going to work on this by myself and find things can be fun. But ultimately, if you have four or five other people doing that, I meet every week with a group of people who experiment and play with this stuff. And they're the most advanced users on the planet, in my opinion of this, because they just share with each other. And you can go in, you can take four or five people who know nothing about them, put in front of chat GPT and say, let's try to do this and let's share what we learned. And you can create a group of experts. And I would say that, and they can filter in, you know, I have channels. I met with people, people bring in like, Hey, I saw this. This is really cool. And I would update. Nobody's an expert on chat GPT right now. There is, there is, there is, it is a, the, the, the, the most knowledgeable person about it next year may not have even touched it right now. So I would say the path you're doing is great and just create a channel for people to continue to share. And just like literally it can be a message channel. It could be a Facebook group. It can be whatever, an email thread and say, Hey, I found something cool. What do you think? And I can plant some seeds, Cara, for you now, Miles and Andrew Cara is putting together a workshop for faculty at UC Berkeley. I think originally we thought it'd be in September, but we've pushed it to October. And so if either of you are interested in joining Cara to participate in that, please let me know. And I will connect you guys. I reached out to Cara and said, Hey, there's two really amazing gentlemen in presenting in this event today that I think could be great. Could be great for your workshop. So thank you so much for your question, Cara. Thank you, Victoria. You're up next. And then Denise. So I, my question, I'm a philosophy graduate student. So this is kind of some of my friends and colleagues are in my thoughts with this question, but have
Excited, or they might be terrified because this is the first time a machine could capture life as good or better than a person. The first time we had a machine capable of doing that. And that would be intimidating.
The next slide I show is James Cameron on the set of Avatar Way of the Water, which is a billion-dollar production, state of the art, employing thousands of artists to work on a thing, create an incredibly detailed world. And he is holding the camera. But this camera has no lens because it's a completely virtual camera, and it works inside of a massive array of computers and whatnot.
I think if I was going to try to explain to an artist in 1838 what was going on there, I would be at a loss. And I'm not even talking about the blue people with tails. Just trying to explain what's going on from there is hard. And I think that's the world we're going to get to for artists and people like that. I think for a lot of people, reservations, as they see that there can be a canvas for them, will be excited. But I have to give them room to have opinions and to feel like, hey, tell me why this is worthwhile. Tell me why this is valuable to me, or accept the idea if they decide it's not.
Great question, Victoria.
So, Denise, I know that you just wrote this in the chat, and I don't mean to put you on the spot. But one thing I did want to share with Andrew and Miles is that Denise is the mother of one of our engineers, Rory. And it just reminds me of part of what you said was your role, Andrew, as the storyteller, that if our parents can understand the contemporary technology, then maybe we're doing something right. Denise was a lifelong medical practitioner. She's a retired physician, and she's very interested in evaluating models for us. And she shows up all the time to these events. So welcome, Denise. I just wanted to introduce you.
Okay. Hello, everybody. Thank you for Rory. Yeah. Thank you so much, Rory. And do you want to share a little bit about what you dropped in the chat, Denise?
Yeah. I basically, one of my concerns with all of the implementation of technology, I'm in the generation of physicians who initially practiced without electronic implementation and medical records and all of that. And we're part of that experimental generation where it was just thrust upon us to, you know, get on the train. And the trains left the station, you know, just get on or whatever. And one of the things that I think is happening, any other intervention in medicine historically has to go through a rigorous process of testing. You have plenty of people who have ideas, and some of those ideas turn out to be nonsense.
And some of them turn out to be right in terms of what's best for patients. And so we have a process where if you have an idea that you think something's going to be, it first gets studied in a retrospective way, and then eventually goes to a process of a randomized controlled study before it is implemented on patients and put into practice in a widespread way. Okay. And when electronic medical records came on, that wasn't done. Electronic medical records, somebody had the idea almost like science fiction that this would be great and just said, everybody implement it now. And that has had implications in the practicing of medicine that have real consequences for patients that are not all good. Some of them are good. Some of them are not good because having all this information available to you didn't change the fact that you don't have any time to absorb it and to make those decisions.
So that's my biggest concern is in my playing around with chat GPT, I sometimes find that it'll give me inconsistent answers. Sometimes it will skirt the question. It won't give me something that's particularly useful. Some of that may be on purpose because of blocks that are in there to avoid people using it as a replacement for a medical practitioner. But I just really think it's important that we do some of these kinds of tests to the best of our ability on a small scale before we're doing widespread implementation in medical practice. And I will say to people play with it, that's fine if they're playing with it in a situation that does not involve patient care, but if they're playing with it and then using that in their practice without it having been tested, who knows how that's going to go.
It's a great point. And it's a thing that we saw a lot of really early science and GPT-4 could be very useful in medicine and whatnot and diagnosis. But we're not eager to say, oh, let's go put this out there because of those concerns and these things need to evaluate it, need to be tested, particularly when it comes to the situation. But I would say the other side of it is, is that if people are dying of diseases and things every day, and there are people who are getting bad diagnosis every day, and if AI has the ability to improve those outcomes, we need to be testing this with good reason, but also understand if we just say, oh, let's not do this, or let's take a lot more time. There is a cost to that. There is the other side, there's a cost to it, and it is hard. That's just like when we implemented things like CAT scans, MRIs, those kinds of things. When I first was in practice, those things didn't even exist. We implemented all of those things. But when they first came out, you would see these little blips on an MRI and no one knew what it meant. Then over time, you start to develop that that doesn't need follow-up. You don't have to go operating on somebody for that particular thing. The fact that you can see something retrospectively doesn't mean that when you see it, it'll always turn out to be the problem that you think. That's the same with any kind of diagnostic tool that we're using, lab tests, x-rays, et cetera. We're always analyzing, is it over-diagnosing things or under-diagnosing things, and how does it compare with our current tools that we have? Is it better? Is it worse? I think that's the important part. It's not that we shouldn't be implementing these things. Anybody who's had an electronic medical record doesn't really want to go back, but they want to fix the problems with it. I think that that's true with this tool as well. It can be a wonderful aid. What really matters is what's best for patients, but making sure that that's what we're doing and not just imagining we're doing when maybe we are and maybe we're not. That's what I'm saying.
Completely agree. I completely agree with you. I had to deal with a situation where a group we were working on was super enthusiastic about these medical applications. They wanted to run out and tell everybody. I'm like, we need to get more people to weigh in on this. We need to get more medical experts and people to do this because my fear was we could tell people this was great and then find there were these problems and prematurely bring in a stop to this sort of development before we want to, so 100% agree with you.
My son asked me one time, said that one of the things they're bringing up is how physicians are overwhelmed by documentation requirements and how could AI help with writing your notes. I think one of you mentioned that earlier. How is AI going to get the data to analyze that, to write that note? How is AI without me as a provider entering that data? I'm in the room with the patient. Are those patient encounters going to be recorded by AI and then AI is going to analyze and decide? How is that data getting, because if that data is getting put in by the provider, the data entry is overwhelming for every patient encounter. That's the kind of thing that I'm curious about. What's the vision? What are people thinking when they have those kind of ideas? Do they understand how it might play out on the actual implementation?
This sounds like a great forum conversation.
Yeah, that's right. I think we should definitely follow up on this, Denise. I just want to give us a time check because we are technically at the end of the event. Greg has had his hand up for a long time. Miles wants to chime in. Just before we move on and close out, I just want to say, Denise, thank you so much. I think that it is so valuable to have an intergenerational community. You have so much life experience that you always bring to your stories, and I'm very grateful that you show up every evening. I'm going to take that note that you brought with us today, and I think we should definitely talk about that.
that in a roundtable event. Miles, you raised your hand. Yeah, I just want to follow up on some of the specific points there. I mean, definitely won't be able to do justice to the whole issue of kind of AI and healthcare where there's a lot to unpack there. But one of the questions was about whether we can learn things without deploying and kind of testing in the lab. I think that's one difference between kind of AI and medicine. I mean, there are some intersections between the two. But unlike, say, testing a drug, it's relatively easier to test, for example, what the accuracy rate of a speech recognition system is or the test against a certain medical questionnaire and kind of get some information. Obviously, there's always the question of does this generalize to a real-world setting. And at some point, you're going to run out of the testing that you can do. And then you get into questions around RCTs and kind of safety regulations and so forth. But I think on some of the specific applications, I'm relatively optimistic about testing before deployment. But that leads to another question, which is, do people actually do that testing? I think it's important that we not rush things or require doctors to adopt things that aren't necessarily trustworthy. There have been some stories about nurses, for example, being under pressure to use a certain kind of AI diagnosis system that they didn't trust and so forth. So there are definitely risks, as well as opportunities as came up. I think this is kind of why we need investments in AI literacy. And it's like a big difference to blindly trust a tool that you don't fully understand versus being able to use it as one input among others. Thank you so much, Miles. And we have one member who's had their hand up for a very long time. Greg, you are up and I just want to be mindful of our presenters time because we're now three minutes over. But please, we want to honor your question. I got no place else to go. Okay. All right. So sort of, I think some of the things that were brought up, I think, are really applicable. And I think that the human feedback as part of the process around AI is essential. And I think, you know, I think OpenAI has figured that out really early on, but it actually matters, I think, at every level. And we look at prompt engineering and their output of prompts and looking at that, having humans in a loop is incredibly critical. The way you form your question matters. Saying, for example, I'm an expert in life sciences, and that I have a deep background in a particular area, that is actually essentially getting the right answer out. And then having real SMEs test the output at each step. And so I think, just thinking about best practices going forward, I think it would be great if OpenAI sort of started developing these best practices of how to leverage AI in a responsible way that actually produces the best output. Yeah. So I'd love to hear your thoughts on that. Yeah, I mean, Andrew might want to say something here. My sense is that, you know, right now we have a couple different resources for kind of different audience. There's something called the OpenAI Cookbook, which is specifically for kind of people building applications. We put out, I think, as was mentioned, the resource for educators. And, you know, so there are different things for different audiences. I think we're like constantly improving what, you know, what is the most useful thing. And, Andrew, do you want to kind of add anything to that? Yeah. Yeah, I'd just say, when I started three years ago, PromptEngineer is a very different thing. Because the first language model, the big one, GPT-3, was basically trained on a large corpus of text. And to get it to do a thing like write a blog post, you started off with what looked like a blog post. And it said, okay, this is the rest of what this should look like. You know, and that was, you know, the dark art was basically, where in the world of text has it seen this pattern? How do I get it to follow through and complete it? And then when we introduced our instruct models, our reinforcement learning and human feedback, we then said, well, let's take really good interactions and train the model on those. And it will also generalize to understand what you're asking it to do. So you don't have to go to Hogwarts to learn how the model, you know, get the model to do something really cool. The next step is probably going to be, you don't have to be an expert in proteomics to get it to understand, you know, how to solve this question or do this. And so I do think you're right. And I was like, we do want to create more resources to do this. But I wrote the original prompt examples for GPT-3. And I look back at those now. And I'm like, look at like how much contortion I had to do to get it to do something like give me an airport code. And now it's like, just give me the airport code. Yeah, here you go. And so I would say, I think that we should certainly educate, but I think these things are getting so good so fast that hopefully you won't have to be the expert. But I think experts will then get even better value out of it. Like if you were a really good prompter three years ago, you're an amazing prompter now because you know how to get it to you're like, I can't do Wordle. Yes, it can. You just got to understand tokenization. And so but have it meet people's hopefully the goal. Well, thank you so much, fellas. Brad, thank you for your question. It was also wonderful to meet you last week. It's really awesome that we're actually starting to get to know each other. I feel like I'm being really beginning to know everybody's story that shows up. And that's so cool. That's the point of community. So just in the spirit of letting our speakers go and enjoy the rest of their evening, I think we're going to come to the close of our event. It is always such a pleasure to share this space with you guys. In the pipeline for the next few weeks are several more super awesome events. One of them is in person that I want to share with you. You can register for it now. It's the September 7 in person at OpenAI with our CEO, Sam Altman and the founder and CEO of Helion. It's about the future of energy, specifically as it relates to fusion energy. After that, one of our colleagues, Shabani Santukar is going to come on to the forum and describe her research in how language models are, whose opinions are reflected in language models. And I think that that will be a really fascinating talk for all of us here. And then finally, in October, we will be hosting somebody really very special. Evan, I think this event will speak to you, but we're going to be hosting Terence Tao, the future of math and AI with one of our co-founders, Ilya Suskiver. So I hope to see you guys for any of those. I also asked my colleague to drop our newest blog in the chat and also notify us in the event. We just published a blog today on teaching with AI. One of our colleagues, Pamela Mishkin, worked very hard on it, and I think it's absolutely amazing. So I hope you guys will check that out. And then until next time, guys, I hope you all have a wonderful Thursday evening. I will be back in San Francisco next week. I hope I'll see some of you in person, and I'll follow up on all of the connections that need to be made. Cara, I'm going to introduce you to our colleague, Natalie Summers, and then if you guys have any challenges reaching out to each other or messaging each other in the wake of this chat, please let me know. And then I'll also follow up with Andrew to make sure that I make accessible some of the educational materials that Andrew referenced in his talk. So Miles, Andrew, thank you so much for that awesome educational experience, and we will see you guys next Thursday. Take care, everybody. Have a wonderful night.
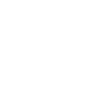