Thinking Machines & AI Economics: How Reasoning AI Is Rewriting the Future of Work, Science, and Strategy
speakers
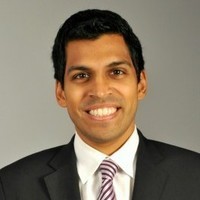
Aaron “Ronnie” Chatterji, Ph.D., is OpenAI’s first Chief Economist. He is also the Mark Burgess & Lisa Benson-Burgess Distinguished Professor at Duke University, working at the intersection of academia, policy, and business. He served in the Biden Administration as White House CHIPS coordinator and Acting Deputy Director of the National Economic Council, shaping industrial policy, manufacturing, and supply chains. Before that, he was Chief Economist at the Department of Commerce and a Senior Economist at the White House Council of Economic Advisers. He is on leave as a Research Associate at the National Bureau of Economic Research and previously taught at Harvard Business School. Earlier in his career, he worked at Goldman Sachs and was a term member of the Council on Foreign Relations. Chatterji holds a Ph.D. from UC Berkeley and a B.A. in Economics from Cornell University.
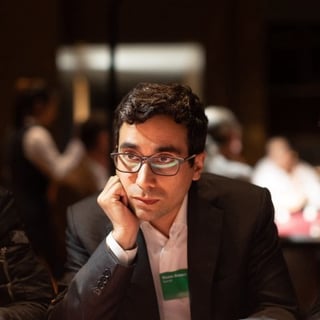
Noam Brown is a leading researcher in multi-agent reasoning at OpenAI, known for co-creating the first superhuman AIs for no-limit poker and the first human-level AI for the strategy game Diplomacy. He holds a Ph.D. in Computer Science from Carnegie Mellon University and previously worked as a Senior Research Assistant at the Federal Reserve Board, where he researched algorithmic trading in the foreign exchange market.
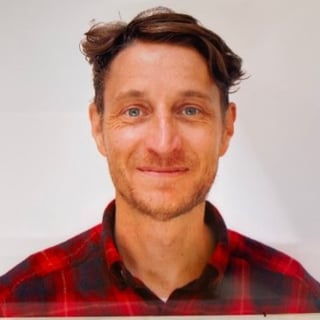
Tom Cunningham is an economist and data scientist with expertise in strategy, antitrust, advertiser behavior, auction design, and network effects, including experimentation, modeling, and tipping points. He is currently a Data Scientist at OpenAI and has previously held roles at Twitter as a Senior Staff Data Scientist and at Facebook as both an Economist in Core Data Science and a Data Scientist. His academic background includes a Ph.D. in Economics from the London School of Economics, and he has also contributed to research and teaching as an Assistant Professor at Stockholm University and a Visiting Associate at Caltech.
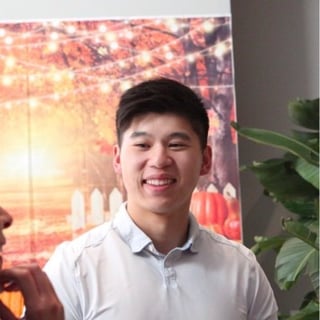
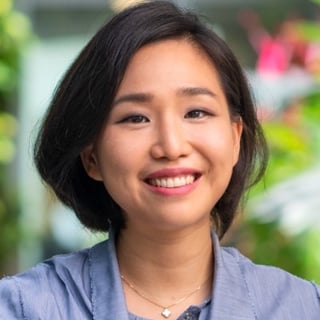
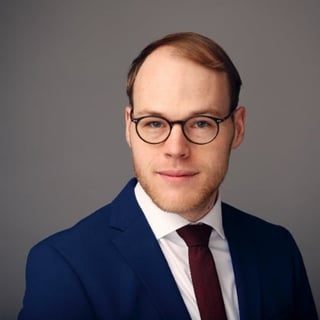
Oliver Giesecke is a research fellow at the Hoover Institution at Stanford University. Giesecke works on topics related to asset pricing and public finance. His recent work examines the finances of state and local governments across the United States, including the capital structure of state governments, the book and market equity position of city governments, and the status quo and trend of public pension obligations. For his work on city governments’ finances, he was awarded the NASDAQ OMX Award for the best paper on asset pricing. His work on pension obligations was instrumental to shaping state legislation.
In addition, Giesecke has conducted a large-scale survey that elicits the retirement plan preferences of public-sector employees across the United States. He is the author of the Stanford Municipal Finance dashboard, which provides, for the first time, credit spreads and fiscal fundamentals for many state and local governments in the United States. The dashboard has received national media coverage in the Bond Buyer.
Before beginning his current academic career, Giesecke worked for Germany’s Federal Agency for Financial Market Stabilization and as a senior quantitative finance consultant. He received a PhD in finance and economics from Columbia University, a master’s in economics from the Graduate Institute in Geneva, Switzerland, and a BA from Frankfurt University, Germany.
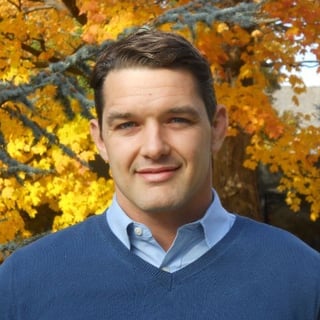
Christopher Neilson is a Yale professor, economist, and entrepreneur specializing in education markets, whose research, policy work and startups have helped millions access education and improved school choice systems in multiple countries.
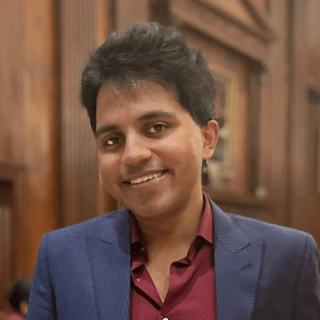
Hemanth Asirvatham is a member of the Economic Research team at OpenAI, primarily involved in using LLMs as economic research tools and exploring the intersections of AI with labor, quality of life, and tech adoption. He recently graduated from Harvard University with a degree in Economics.
SUMMARY
The evolving landscape of AI is marked by increasing generality, scalability, and advanced reasoning capabilities—trends exemplified by OpenAI’s "O series" models, which demonstrate the potential for AI to "think" before responding. In remarks by Noam Brown, Researcher at OpenAI, the discussion highlighted two key AI paradigms—pre-training and reasoning—and how models improve as they process more data and compute. These technical advances are not only accelerating model performance but also reshaping the strategic and economic dynamics of AI infrastructure.
Complementing this, discussions led by OpenAI’s Chief Economist Ronnie Chatterji and Forum members explored how AI intersects with geopolitics, national security, and economic policy. They examined the balance between democratic and autocratic approaches to AI development, the implications for global alliances, and how AI infrastructure investments influence both economic and military strategy. Together, these conversations underscore the dual trajectory of AI: accelerating technical progress and deepening its role in global policy, infrastructure, and institutional governance.
TRANSCRIPT
Yeah, thank you all. It's great to be here. Yeah, so I do have a previous life as a wannabe economist. I went to the Federal Reserve after college, did it for a couple years, planning to do a PhD in economics, and then decided, actually, I really like building things, and so I decided to do computer science instead, which I think ended up being a good choice. So now it's great to talk with all of you.
So I want to start by talking about what is different about this era of AI. I don't know if any of you have been following AI for a long time, but there have been a lot of cool, impressive results in AI for a long time. So for example, IBM's Deep Blue beating Garry Kasparov in 1997, or IBM's Watson winning at Jeopardy in 2011.
And there's also been a lot of pretty impressive results in certain domains, like the Postal Service has been using optical character recognition to sort mail for a very long time, and facial recognition on Facebook has been around for a very long time. So what is different about things like Chachabiti and the era of AI that we're living in today? And the answer is really about generality.
Like I'm going to show you a lot of impressive results in this talk, but I think the most important thing to understand is that when IBM's Deep Blue beat Garry Kasparov in chess in 1997, they spent two years, or more than two years, working just on getting an AI to play chess better than the world champion. And same thing with Jeopardy. They spent years figuring out how to get this AI to play Jeopardy super well, and it can't do anything else. But what's really special about Chachabiti and the AIs that we see today is the generality, the fact that they can do so many different things without being targeted at those things.
I want to talk about two paradigms. There's the pre-training paradigm and the reasoning paradigm. And I want to start with the pre-training paradigm, which has been around for longer. It's really what was initially powering Chachabiti. It started off, I guess, in 2019 with GPT-2.
And the idea is that you just collect a ton of text, basically like large fractions of the internet, and you train this AI model to predict the next word in a sequence of words. Now, this leads to surprising levels of intelligence. And why is that? Well, I would argue that the reason is because if you take the entire internet and have sequences of words, and then like this, for example, somewhere in that internet is chess games. And if you ask the model to predict the next word in this sequence, well, what does it have to understand in order to predict that next word as well as possible?
It has to understand chess. It has to understand the strategy of chess. It has to understand how good these players are based on the moves that it's seen so far. It has to understand the geometry of a chessboard and how to move the pieces around. So there's a lot of things that it has to do, a lot of things it has to understand in order to predict this next move. Another example, which Ilya Sutskever used, which I think was really good, is imagine you have a mystery novel on the internet. And the model has seen all the text in the mystery novel, and it gets to the very end. At the very end, the detective says, I know who the killer is. The killer is blank. So in order for the model to be able to predict that next word, it has to understand the entire plot of the book. It has to understand the characters, the motivations, a world model of how an ax can move through the air or something. I don't know. But it has to understand so much about the world in order to predict that next word.
So that's the beauty of this paradigm. And the other thing is that it's very general because it's trained on the entire internet. So it gets a lot of generality in there. What's been really impressive is that we've seen consistently that as you throw more data and more compute and larger models at this pre-training paradigm, it gets consistently better at this task of predicting the next word. And you can actually, there's a very famous paper in the AI space, Scaling Laws for Neural Language Models and then also training compute optimal large language models that show you can very predictably measure how much better the models will get at this task of predicting the next word as you scale up the model size, the amount of training, and the amount of data. And this is really the underpinning that gave OpenAI the confidence to invest a lot of money into scaling up these models.
Now predicting the next word doesn't necessarily mean that it's getting better at things that we actually care about, like coding for example. But what we see empirically is that as you get better at this task of predicting the next word, you get better at doing all the downstream things we care about, like coding, like math, like answering questions. And this is really what has powered the whole GPT paradigm from GPT-1 to GPT-2 onwards. And you can see that the models get better.
So in this example, there's this question, when should I organize the Christmas parties so that everyone can attend, and then it gives a bunch of constraints. If you ask GPT-2 this question, which costs around $5,000, it doesn't get it. It doesn't even answer reasonably. If you ask GPT-3, it gives an answer, but it's wrong. Same thing with GPT-3.5. But if you ask GPT-4, it actually gives a correct answer. And the main difference between GPT-4 and all these prior models is that GPT-4 was just a larger model trained for longer on more data, which makes it more expensive. This is great.
And honestly, when GPT-3 came out and this trend line continued, there were a lot of people in the AI space that thought, OK, this is it. We're done. We have the answer to superintelligence. All we have to do is just keep scaling this up, and we will get arbitrary levels of intelligence. And I actually do think that's true in theory. But the key thing to understand is that this gets very expensive very quickly. So GPT-2 costs, I think, $5,000 to $50,000, depending on how you measure. This website is saying GPT-4 costs around $50 million.
You can imagine that if you want to keep scaling this up by more orders of magnitude, it's going to become very expensive very quickly. And the other thing is that you're not, like, yes, it's getting smarter, but it still has a long way to go. So this is going back to what Ronnie said, that the field is evolving very quickly. And so I think a lot of the criticisms that you might have heard about LLMs and the scaling paradigm might have been true a year ago, but are not true as of September because we now have reasoning models.
And this is the second paradigm of scaling. Now, the idea behind reasoning is that, OK, I've said that pre-training costs have grown rapidly. We've exceeded tens of millions of dollars. Some training runs have cost hundreds of millions of dollars. Yes, you can go further. You can go to billions. You can go to tens of billions. But at some point, the economic returns are just not there. But the key thing to understand is that as these training costs have ballooned, the inference cost, the cost of actually asking the model a question, has remained quite cheap. Now, that is a new dimension for scaling.
What if instead of just scaling the training cost, we scale up the amount of thinking that the model does before responding? And that's the idea behind the O series models, O1. The idea is that if you ask GPT-4 a question, it's going to cost on the order of magnitude a penny. O1, if you ask it a question, it's going to think for a long time. It's going to think for maybe a minute or so before responding. And it's going to cost a dollar, maybe, order of magnitude, plus or minus, for an answer. But that response is going to be a lot better than the one that cost a penny.
And you can see that in this plot to the right here. So this is the AME. This is a competition math. It's the qualifier for the USA Mathematics Olympiad team. And the y-axis is the accuracy, what we call pass at one on this exam. And the x-axis is the amount of compute, the amount of inference compute that the model is spending to answer these questions. On the very far left, it's basically responding instantly. On the very far right, it's taking a few minutes. And you can see that as the model thinks for longer, the score pretty cleanly improves.
So this gives us a new dimension for scaling. And the beautiful thing about this dimension is that it's pretty untapped. Like I said, GPT-4 costs order of magnitude a penny to query. There are a lot of questions that people care about where they'd be willing to pay more than a penny. And now we have a lot of room to scale this further to dollars, tens of dollars, possibly more.
To give you an example of what the model is doing when I say it reasons, how many of you have played New York Times Connections? Has anybody not done it for today's? Because this is today's. So there's going to be spoilers. You have like 30 seconds if you want to look at this and try to solve it really quickly before the model does. Okay.
So the idea behind this puzzle is that there are 16 words and you have to
them into four groups of four based on some shared meaning and you can try to like look at some of these words and see if you see if you spot any connections you can plug this into the model and ask a one you can actually do this right now you have chat GPT plus you can go you can just like log in give it these questions you actually give us give it a screenshot of this and ask you to solve it and this is what it's gonna do it's gonna reason and it's going to reason in natural language in a very human like way it's going to basically what the model does is what we call a chain of thought it's like an internal monologue where the model speaks to itself about how to solve the problem instead of just responding instantly and you can see that it's going to look for some it's going to go through some options so I'm thinking about I'm linking words to form phrases or compound words like frostbite from bites I'm mapping out how words like border edge fringe and skirt share meanings related to boundaries you know it's considering things like French onion and it's gonna just do this for a minute and a half it's gonna get quite long actually and at the end it will say okay I've reasoned about this and the answer is border edge fringe and skirt are all synonyms for a boundary bite clip file paints all go with nails and so on and this is this is actually the correct answer and it does it a lot faster than I can
what does the performance look like on on benchmarks that we care about so far left this is competition math this is the Amy 2024 and gbd4 gets around 13% on this benchmark oh one preview which we released in September gets around 57% oh one which released in December gets around 83% this is going up quickly so I want to go back to the to the thing about like progress is fast we released we had released a one preview in September we released o1 in December and we also announced o3 which we haven't released yet in December and the numbers for o3 I'll get to it a little bit for coding is even higher and the numbers have gone up since that on competition coding which this is code forces gbd4 oh gets 11th percentile this is among you know serious competition coders like human competition coders o1 gets 89th percentile so this is a very impressive result
on PhD level science questions the GP QA benchmark this is multiple-choice questions that basically were you need to be at in domain PhD expert to answer these questions humans get 70% gbd4 oh got 56% which is already frankly super impressive oh one gets 78% and again those numbers have gone up since this was announced in September we announced o1 in in September and then we released o1 preview in September we announced o1 in December we released o1 and we announced o3 on competition coding this is code forces so o1 got 1890 that's 89th percentile so this is elo o3 gets 2700 that places it in the top 0.1% of human professional like competition coders I think it was like the 175th best competition coder in the world we have since announced I guess Sam mentioned this in a talk that we now have a model internally as of like you know a month or two ago that is the top 50th in the world within you know within the top 50 and those numbers look I fully expect that by the end of this year our models will be superhuman at competition coding now that's competition coding and again like there's been a lot of impressive AI results throughout history you know deep blue made an AI that beats Gary Kasparov the world chess champion in 1997 so when I say like we're doing something at a superhuman level that by itself is not novel there's been a lot of results like that throughout AI history but what's special about o3 is that it's not just competition coding it does a lot of things really impressively and in fact there is this benchmark called suite bench verified which has actual real-world coding tasks these are tasks that you know involve require all the things that a human would do it to submit a pull request oh one preview got 41% again this is already state-of-the-art oh one got 49% on this benchmark o3 gets 72% so you know there's a lot of real-world economic impact in this model even though it wasn't trained just on coding just to reiterate what what Ronnie said is that AI is moving very quickly and I want to emphasize this because there's I see a lot of skepticism about AI including from people in the field of AI and I think that a lot of those criticisms are due to the fact that the a that that progress has been so fast and a lot of what they're pointing to as flaws is things that were true six months ago but are no longer true today and or will not necessarily be true three or six months from now to give an example of this there was a keynote talk at a natural language processing conference in 2023 mid-summer 2023 and the speaker in the keynote gave this prompt as an example of something that none of the models could do it's this reasoning task where you say if block C is on top of block A and block B is separately on the table can you tell me how I can make a stack of blocks with block A on top of block B and block B on top of block C but without moving block C and the answer is that it's actually impossible and if you asked any of the existing language models this question they would always hallucinate some kind of answer and say like oh yeah here's how you do it when the correct answer is actually that it's impossible and we see now with a one that it just recognizes right out of the box that this is impossible and in fact even GPT 4.5 now recognizes out of the box that this is impossible so I just would encourage all of you to keep this in mind that when people say like okay well this thing is not possible language models cannot do this that's why it's not going to be a big deal to keep in mind that it first of all it might not be true as of today and even if it is true today it might not necessarily be true in three or even or six months.
Welcome back, thanks so much for doing this. The geopolitics discussion was fantastic. Just a couple of things I wanted to summarize from that discussion where we got great participation and a lot of interesting ideas.
One is just starting with the mission of OpenAI, which is to benefit all of humanity. And as I said in the group, I mean, people take that very seriously around here. So this idea of how a more divided geopolitics, how great power competition, polarization are shaping our current environment and how that's gonna affect our technology is really first order at OpenAI.
So we talked a lot about people's advice for me. I kind of used my group as a bunch of free consulting. I hope that was okay. Then I asked them, what should we do, right? What should we do? And the group had a bunch of different ideas. Some folks really saw the importance, and I think it's shared by a lot of people inside the company, of drawing a line between sort of democratic AI and autocratic AI and sort of the dividing lines that are already being drawn in the world in many different dimensions and kind of embracing sort of the values that come with democratic AI and embodying them in our product. And you're seeing that in some of the work we're doing.
Others cautioned us, though, and I took this to heart about accepting sort of this divided world and cautioning us that if we did so, we might both lose credibility in key markets where we're trying to gain trust, and we actually obviously might lose access to key talent. And so balancing that was a really important theme in the discussion.
There was a really interesting thread about, hey, what does the chief economist really have to do with any of this national security and geopolitics stuff? Isn't there someone else at OpenAI who's supposed to do that? Which is a really interesting question, and I think I made a point which I think I'll make here, which is that I'm not sure that economics as of late has had much as a feel to say about some of these national security issues, but when I was in the administration working in government, I was often paired head-to-head with generals and people representing three-letter agencies. Economics and national security are being blended in a lot of contexts, and I think for economists now who are operating in the world in a company like this from governments, we might not necessarily have the toolkit in all the ways to engage in these discussions, but the problems are coming at us and people are looping us into those discussions.
So we do have a great geopolitical team at OpenAI, close colleagues of ours, but a lot of those questions are fundamentally economic as well. And when you bring someone into the discussion and you have a different language, an economist, right, talking about, let's say, consumer surplus or externalities, and a national security person talking about existential risks, that's a conversation I think economists need to learn how to have, even though economics is probably still catching up. The third theme that arose, which I thought was really interesting, was this notion of, with all the work on foundation models and the idea of deep-seek and the notion that being a fast follower might be good enough, how does that shape the geopolitics? And so in some markets, if you're a fast follower and you have a model that performs at 60% as well as the frontier model and it's a lot cheaper, is that what you're gonna adopt at the country level and what does that mean for OpenAI? On the other hand, do you really want your country to have a model that's at 60% of the capability of the frontier model? Will that allow you to build a competitive industry? I can tell you, in Europe, that's exactly the discussion now, which is one of the reasons where, when we were in Paris, Macron, President of France, he announced a huge infrastructure investment in France funded in part by private capital, but including sovereign wealth funds from the Gulf. And so you're seeing new alliances now, in that case between France and Middle Eastern countries, formed to build AI infrastructure.
These things matter to people inside OpenAI. If you look at our Slack channels, if you hear our town halls, you'll hear people really thinking about this carefully. We have a very international group as well in OpenAI. So this notion of different parts of the world adopting different kinds of technologies and competition between superpowers is unsettling for many.
The last piece, we had someone in our group from the Naval Postgraduate School, right? The military and defense component is really key here to the geopolitics. Militaries are adopting AI at rapid rates. And it's interesting to think about how the structure of militaries, like a pretty decentralized military, actually, the US compared to a more centralized military in China, what that means for the diffusion of those technologies and the development of military capabilities and how we should think that, take that into account as we're developing our models and disseminating them. So great discussion, really interesting, enjoyed it. Hoping to follow up with everyone in my group.
And now I'd like to pass it up to group number one, and we'll go in order as we go down the list. Group one?
So group one, I guess, is education, broadly. And we got really into, really, one thing, I think. We started talking about how does students using AI in the classroom, education, the production function, how is this affecting how you do assessment and what needs to happen. So we had a little back and forth there. Some comments about how hard it was to evaluate somebody that could just copy-paste something in the chat GBD and then give it back to you. And then another, a little bit, how curriculum might need to have to change to adapt. And some comments, also, is this similar as to when we have kids multiplying but you could just not let them use a calculator? And then you gotta get really good at using a calculator. So we had some conversation about that.
And I think it did pose a second thing related is how much penetration, how much was being used and what type of people were using these things, both on the professor's side as on the student's side. And so I guess, maybe I'm collecting some different comments. Some of this was like there's heterogeneity in who's using these things and the intensity in which they're using them. So everyone was like, yes, I use chat GBD, but it's not the same to be using it in a structured way to learn or answer questions. So yeah, I think that was basically kind of where we ran out of time. It seemed like it was like five minutes and then they said the bell rang and we were done.
Yeah, I take my notes because we had discussions spanning a lot of topics such as market structure, social safety net, emerging markets, inequality and taxation. But I want to focus on a few more specific things. So the first one was sort of an extension of the existing task measures. So in the paper, GPT says there's a sort of exposure measure for each task, but we were discussing that we could maybe have a sort of multidimensional task measure that includes like first of all, the economic value of a task. So if AI performs a task, well, how much economic value is graded as a result? The second dimension would be the risk dimension. And that includes like, if an AI fails on a specific task, what is sort of the, how costly would an outcome, an extreme outcome be in that context? And the last one is task complexity.
And so here really, this is, I think like was inspired by the conversation that we had prior. There's in each task, there might be some distribution of subtasks and some of them may be at the very end of the tail. And so they might be unlikely to, where an AI might be unlikely to perform well. And so basically these task complexity measure would give us a sense about how likely is an AI to perform well on a task.
The second big topic that we had was more going into sort of the geographics. So we discussed just certain things like, especially if you go to the developing market context, there might be a very different industry composition than in the developing world. And industry composition might be highly suggestive of how well AI is performing in that context. We also discussed the aspect of language that AI mostly developed in the developed world may not perform as well in like languages where there's less training data available.
I think sort of a separate aspect in that context was also like, what are the opportunities in the emerging economies? So take for instance, last mile delivery of healthcare. Everyone that has a cell phone and connectivity can potentially suddenly get healthcare advice. And once again, we have to keep the context in mind because the outside option is basically receiving no healthcare. So maybe the really the threshold that we have to obtain is a lot lower in that context and could really lead to a lot of benefits, but also potentially to like leapfrogging of certain technologies. Thank you.
In group three, we spoke about the economic consequences and implications of AI in enterprises. And so at a high level, we came to the conclusion that AI is fundamentally reshaping the enterprise landscape. But the transformation isn't just about replacing humans or companies, rather it's about a race to technologize. And this is within.
companies, Fortune 500, SMBs, and everything in between. So the way that we discussed it broke down into three main dimensions. First, AI replacing humans. I think the real question is not if, but how AI will augment and or replace human roles down the line. And the second is AI replacing companies. This was an interesting topic that was discussed by one of the members. And rather than AI startups overtaking established firms, it is rather a competition to adopt and integrate AI effectively. You have industries like banking that illustrate this divide. Some have the technical talent to invest in AI, while others rely on legacy systems like mainframes using AI to modernize. Companies must decide to either lead in AI adoption or fall behind. The third dimension is implementing AI from the perspective of a human and or companies. So success depends on those who understand and apply the latest AI advancements. And the impact spans across technical support, operational efficiency, and strategic decision making. And so the big question is, what is the AI journey? And companies must plan their transformation. Where are you today? And where do you need to be in two years? These are the big questions that companies are asking. And the journey isn't just about buying AI tools, buying licenses or receipts, but rather about embedding AI across the value chain.
And so we asked ourselves, what does it mean to be an AI leader today? And it boils down to a couple of things. Number one, empowering teams. Deploying AI-driven co-pilots for engineers or other roles. That co-pilot is just becoming an assistant today. There's a really good analogy. GBT-3 was a tool. GBT-4 was an assistant. And GBT-Next is an agent. And so today, we're deploying co-pilots. We're deploying assistants. But in the future, maybe we'll be deploying agents. Second is enhancing the customer experience. And this can look anything like assistant chatbot to depersonalization. The third is strategic AI implementation, identifying high-impact areas within the organization for AI deployment. And finally is going beyond licensing. AI adoption is not just about purchasing tools, but ensuring, number one, that a significant proportion of employees actively use AI. They use it well. And number two, that AI systems are customized for tailored insights. And so I think, ultimately, the enterprises that win with AI won't just use it. They will build, adapt, and lead with it. The question is, for companies, will they be a leader or a follower?
Hello, I'm Hemant. Unlike for other speakers, this microphone is the right height for me. So that's pretty good. So there's a lot about the impact of understanding the economics of AI. And of course, that's extremely important, at least to me. But if we are to think that AI is some transformative economic technology, we should also think it is a transformative technology for the field of economics, too. And it is, at least, it might be the way economics keeps up with all of this. So that's what our group was talking about, was thinking about how AI can be leveraged to do new types of economic research that was not possible before. And we were mostly focusing on how that might be comparatively advantaged relative to other industries. Because a lot of the even simple things, text analysis, basic idea of this thing that can just read a bunch of stuff, comprehend a bunch of text, images, whatever, can be like that MTurk, or can be like that simple role, but at massive scale and very cheap quantities that allow new types of analysis to happen. So we talked about that from a spectrum, from the basics of, let me just classify some labels on my text, how liberal or conservative is this speech, things like that, all the way to, let me use a neural network, a fine-tuned language model as a statistical tool in a regression where it itself learns the complex relationship between high-dimensional text, like a police report, and low-dimensional outcomes, like an arrest or not an arrest. And we talked about in the middle of that, what can we use language models to do to reveal not just complicated relationships between text and outcomes, but also parsimonious relationships between text and outcomes. Can we learn and use language models to distill out, somewhat unsupervised from text, the features that matter the most, whether it's specific characteristics of a person or whether it's specific attitudes or emotions.
The second part of what we talked about was how much can AI agents, how much can AI play the role of a human to understand human preferences, to simulate human interactions, like a financial interaction or a financial market, to simulate polling. We talked about that both from the sense of where the research is, still pretty nascent, in terms of understanding AI preferences, where they match up with humans, how you can prompt them to adopt different personalities and thus adopt a more representative behavioral sample of people. We also talked about it in the reverse, about how we can look into the internet, look into much, much more data about people from social media, from many sources, and understand their preferences and understand their choices about whether they like bananas or oranges more. Much more than that was previously possible, and how we can use that to expose human preferences, just as much as we can ask the AI to represent humans. So that was just a sampling of what my group discussed. Thank you.
We talked about how to characterize the AI capability frontier, which is something that came up in Noam's talk, in Eric's remarks, and Ronnie's remarks, and mine. And a lot of it was discussion about how to make that distinction of tasks that LLMs cannot currently do. And there were some beautiful ideas, these points about evolutionary pressure that makes humans very good at certain tasks, that it's millions of years of slow evolutionary drip, which makes us incredibly efficient. So more about what humans are unusually good at, whereas computers are pretty agnostic across a whole bunch of tasks. A second about the training process, that the long horizon tasks intrinsically are more difficult to train in the way we train LLMs. Third, about fuzzy tasks, which can be interpreted different ways, but in particular, with long form output, where deep research seems to be doing pretty well with fuzzy tasks, but inherently, they're difficult to train, again in part because you often need a human to evaluate the output. And it's just inherently very expensive. Fourth is this question that tasks which are not digitized, where the information is not already digitized, difficult. And fifth, a sense in which, in many cases, Eric had this nice example of customer service, that there's still this long tail of things, which humans, characterizing, I think, unusual cases, that unusual case is a good way of characterizing where the computers fall down, and where humans still are reasonably reliable.
And then two long digressions we had, one is this really interesting question about the degree to which it's possible to influence the direction of capability progress, or whether there is truly just a sort of latent sense of intelligence, and that it just makes sense to work on wherever the highest yield in intelligence leads you. And a second one of whether there's a good argument that there's going to be ceiling in capabilities, that obviously, we're growing like crazy, and there's been many historical arguments for there being a ceiling, and a lot of those have turned out to be wrong, and whether there are good reasons to believe there's going to be a ceiling in the future.
Our group discussed two main questions. The first is, what do we think AI's potential is to improve decision making for consumers, for workers, for organizations? There's a lot of discussion that we had here, and I think one big point that came out is, I think that we've seen what the potential could be, but what does the top line potential look like? What's the upper limit? We know that it can process information. We know that it can potentially help with cognitive limits, whether it's about working memory, or in terms of general memory, like cognitive processing. But can it actually think about and absorb what our preferences are?
might be? Can it help us redefine, expand, redesign what objective functions might be? If we think about what improvement of decisions might look like, another sort of dimension beyond just information and cognition might be about how to improve the objectives that we think about.
And then the second thing that we talked about was really frictions in that process. So if we know that there's a lot of potential for AI to improve decisions, we also know that there is a lot of frictions. So if we think about tasks versus decisions, what are the unique frictions that come about when we think about decisions? It might be about, you know, whether or not we feel comfortable fully delegating. It might be about trust. It might be about understanding and explaining why we arrive at a particular decision. It might, of course, be about the same types of adoption frictions that we've seen with lots of other technologies that have come so far.
And then I think the last thing that we talked about in terms of the potential to improve decisions is really thinking about, you know, what do we mean by an improvement? So there's the part about objectives, of course, but there's also this question about short-term versus long-term metrics. You know, I think earlier we've had this discussion around how do we actually measure what the impacts look like? And whether it's for an organization or for a consumer, for a worker, how do we actually think about what the long-term effects might be if this really is not actually in line with the short-term effects that we're seeing?
And then the second big question that our group was thinking about was, you know, as AI becomes more agentic, what are the possibilities and what are the institutions that we need to realize some of these possibilities? I think there's probably too much to discuss here.
A couple of things that came out of our conversation, you know, one is really thinking about, you know, the agentic possibilities, or the possibilities coming from agentic AI, there might be three broad categories that we think about. You know, the first category is in terms of incentives. How do we design incentives for agents? We've, you know, we've been studying, you know, agency theory for humans, but what does that look like for agents? How do we think about what their incentives might be in terms of interacting with humans? So if, you know, humans are producing content or have preferences, what are the incentives to actually share those preferences or that content? And then what's the incentive of the agent to actually incorporate some of those preferences and content?
I think the second area is really around management. You know, a lot of us are coming from business schools. How do we think about what the manager of the future looks like? Is this going to be about information processing at some level? Is this going to be about management by exception? Is it something else? Are there a set of new skills that we need to think about in terms of what a manager looks like? And what are the key tasks that they need to do to support some of those decisions?
And then I think a last part is really about organizational redesign. So this sort of connects to, of course, the frictions when we think about AI and decision-making, but as we think about an agentic approach to AI, that raises a whole new set of questions around what is the organization of the future going to look like? How do we think about designing decision process flows, deciding on decision rights, what the key sort of functions of an organization actually look like? How do we even think about what the key pieces are to organizational redesign? I think thinking about those three key parts are going to be important to think about the possibilities.
And then on the institutional front, I think there's mostly just a lot of concerns. You know, we have, I think one of the big challenges that we talked about was how can we learn from history? You know, whether it's the internet, about how do we actually regulate some of these advancements that are coming along? How do we think about the institutions that we need in order to be able to realize some of the possibilities that comes from an agentic approach? And I think a key challenge is that it may be the case that we're not able to get multilateral coordination among private parties that are developing some of these technologies, especially because a lot of the key folks who are behind the regulatory efforts, the policy efforts, may not even be able to understand what the technology is. You know, we're still debating social media and how that works, let alone the pace of technological change that we're seeing.
So if we can't get multilateral coordination, what are the institutions that we need? What are some of the key questions that come up? Like how do we think about, you know, if we're delegating to a whole economy of agents, how do we even verify whether or not that is a delegated agent? You know, what are the new questions that come up that will mean that we need to design new institutions to address some of these questions?
Did we get all the teams? Okay, awesome. So I asked my team what I should say to wrap up, and they gave me the same advice, actually, I got when I had to give a speech at the end of my big fat Indian wedding. It was like a multi-day affair, which is, people seemed like they had a good time. They're probably tired. Keep it short, okay? So I will do that. I actually have the same three things for my wedding, which is dinner, swag, and gratitude, okay? So after this, we get to eat dinner, so you can go right outside. There'll also be some swag. It doesn't have my face on it like the wedding, but it'll be some open-air stuff. And the gratitude, I want to thank Natalie and Caitlin one more time, as well as my econ research team who's done a fantastic job. It's really, really, really awesome.
As you exit today, you're done with dinner. I hope you'll think of this as not sort of the end of a one-off event, but it's really our intention to try to kind of create the beginning of a journey that we're gonna be on together. So follow me or anybody on the econ research team, Natalie or Caitlin, about how to get more involved. Thank you so much for a great day, and enjoy dinner, okay?