Technical Success Office Hours (December 2024)
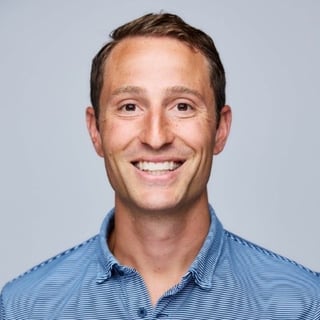
Kevin Alwell is a Solutions Architect at OpenAI, partnering with the company’s largest customers to address their most complex challenges using OpenAI’s technology. He recently joined OpenAI from GitHub, where he spent the last six years implementing developer tools at enterprise scale, including GenAI solutions for Software Engineering. Over the last three years, Kevin has spent much of his time serving Financial Services customers. Kevin is based in the Garden State.
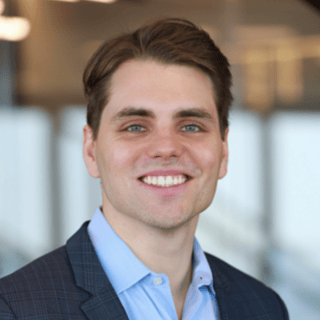
James is a Solutions Architect at OpenAI, partnering with the company’s largest and most strategic customers to address their most complex challenges using OpenAI’s technology. He has implemented LLM-driven solutions in production, pushing the capabilities of OpenAI’s API across telecommunications, automotive, technology, and other sectors. Before joining OpenAI, James worked in Data Science at McKinsey & Co., where he developed deep learning models for client applications. James is based in San Francisco.
The third Technical Success Office Hours, where we tackle technical challenges and share expert insights to support the community. Perfect for those eager to deepen their knowledge and connect with industry experts, this session offers valuable opportunities for growth and collaboration.
Kevin Alwell and James Hills, Solutions Architects at OpenAI, will lead this session as we celebrate the season with cutting-edge AI capabilities designed to enhance your workflows. Dive deep into our latest releases, including exciting year-end surprises that showcase how OpenAI continues to innovate.
I'm Natalie Cone, your OpenAI Forum Community Architect. I like to begin all of our talks by reminding us of OpenAI's mission, which is to ensure that artificial general intelligence benefits all of humanity.
Today, we're going to dive into some of the new features recently released during the 12 days of OpenAI. Our speakers this afternoon are James Hill, a Solutions Architect at OpenAI, partnering with the company's largest and most strategic customers to address their most complex challenges using OpenAI's technology. He's implemented LLM-driven solutions in production, pushing the capabilities of OpenAI's API across telecommunications, automotive technology, and other sectors. James led the development of SWARM, an experimental open-source multi-agent orchestration framework released by OpenAI recently. Before joining OpenAI, James worked in data science at McKinsey and Company, where he developed deep learning models for client applications. James is based in San Francisco.
Kevin Alwell is a Solutions Architect at OpenAI, partnering with the company's largest customers to also address their most complex challenges using OpenAI's technology. He recently joined OpenAI from GitHub, where he spent the last six years implementing developer tools at enterprise scale, including Gen AI solutions for software engineering. Over the last three years, Kevin has spent much of his time serving financial service customers. Kevin is based in the Garden State.
So welcome, Kevin. Welcome, James. Thank you guys so much for being here this afternoon. It's such a pleasure to have you, and I know your time is so precious. So thank you for being here, and I'm going to pass the mic over to you guys.
Great. Thank you so much, Natalie. Great to be here as well. Hi, everybody. Super excited to talk to you all today. So let me just share my screen. Let's go to the slides. All right. So today we're here to talk to you about the 12 days of OpenAI. So hopefully most of you have been following along, but for the past 11 days, we have been releasing something new every single day. And some are big announcements, some are smaller, but really, we are getting into the holiday spirit by releasing a bunch of brand new updates, new models, new features in ChatGPT and in the API that we think everyone really is going to enjoy. So we're going to talk through that in more detail. As Natalie mentioned, I'm James, Kevin will be talking as well. But I can kick stuff off with a broad overview.
So these are the things that we've released in the first 11 days. So I'm not going to spoil tomorrow. You'll have to wait and see. But I would encourage everyone to go onto our YouTube channel. And we live stream all of them 10 a.m. Pacific time. You can watch the recaps of the live streams for a deeper dive than we'll probably get into today. And tomorrow should be exciting as well. So we are going to focus primarily on six of the 11 days so far. But I wanted to give a quick brief overview of the day or the releases that we aren't covering.
Day five was Apple Intelligence. And so for iPhone 16s, you can now use Apple's Apple Intelligence that actually is native integrations with Chachapiti. So think about Siri getting a huge intelligence upgrade. And you can ask specific questions that then route to Chachapiti directly.
So you can use Chachapiti with your phone natively. There's a great video on that on our YouTube channel. Day six was voice and vision. So I'm sure many of you have used advanced voice mode in Chachapiti. But now what you can do is you can share your screen with Chachapiti or you can turn on the camera. And if I had, let's say my computer was broken, there was some error popping up on my screen, I could actually just take my camera, show it, live stream essentially what I'm looking at and say, hey, Chachapiti, what's going on here? And it can see my screen, it can talk to me. So it really is pretty magical. I would encourage you all to try it out.
Day seven was projects. That's just a way to better organize your Chachapiti interface. And so it allows you to create these organization methods if you have these projects where you can have chats that are delegated into their own distinct projects.
Just helps you track things. And it's a pretty neat way of just organizing Chachapiti, which right now just has a bunch of conversations. And it can be a little bit disorganized, so projects is really great. And then eight was a general availability of Chachapiti search. We also made a bunch of UI improvements. Chachapiti search allows you to search the open web using Chachapiti. It is really quick, it's really accurate, and also you can search Chachapiti conversations now. So if there was something you mentioned maybe a couple months ago and you forget what thread it was in, you can just command F and look through your Chachapiti conversations, which is a really small but nice quality of life update.
And then two days ago we released, or yesterday we released 1-800-CHACHAPITI and Chachapiti in WhatsApp. I think everyone, hopefully after this call, you can just call 1-800-CHACHAPITI. It's really cool. It just allows you to talk to Chachapiti through your phone, and that was a really important step in our broader mission of sharing the benefits of AGI with all of humanity. And so we really are working towards getting our models in the hands of as many people as possible, and distributing in a free way our model capability to as many people as we can in the world.
Cool. So that's the quick overview of the days we're not covering, and now we can jump into the actual deep dives on the other days. So I'm going to start with some of the consumer-facing apps that all people can use, not just developers, or that are most appealing to your general user, and developers as well, but they're not API-specific features. And then Kevin's going to give a deeper dive on the actual API features.
So one of the exciting things we announced is Sora. I'm sure some of you have seen a lot of Sora videos, or potentially even tried it out yourself, but it's now generally available for our plus users and our pro-tier users, and it's really cool. It's a really magical experience. You can create video content from just a text prompt, and I think it's something that everyone should try out. It's really fun, and it's a really cool kind of the future is now type moment when you can just make a video from any idea you have in your head. I'm going to show a demo of what the new interface looks like, but we have Sora.com now where you can actually go in there, you can create a video, you can edit or remix an existing video, and you can also input your own images and have that be a reference point for the video, which is a pretty cool feature. So I'm going to demo all of that, but I want to just go through three of the key updates, and then I'll show you all how they work live.
So another update is Canvas is now generally available, and Canvas is something I'm super excited about. You can think of it as a new interface within Chachapiti for coding and for just generally creating documents.
So one thing is the chat interface of Chachapiti is great, but you don't have this persistence of these assets that you create. They kind of are just buried within the chat, but now with Canvas, you can spin up this Canvas, which is like a side panel that has either your code or some documents or a memo or something you want to draft, and it creates this persistent asset that you can then iterate on, you can edit easily, you can share, and it's just a really nice experience to build things with Chachapiti. So in this example, it's showing how a product manager could mock up some sort of a dashboard, some sort of a front end, and it would create a code-based Canvas where you can have the code live somewhere, you can edit it, you can go back and tweak it, and then you can actually render the HTML on the front end in Chachapiti, which is pretty cool.
The other update is Chachapiti apps. And so Chachapiti working with apps, this is something that we just released this morning, is we had previously announced kind of how you can use Xcode with Chachapiti, but now it's broadly available and there's new apps such as the Notes app, which I'm going to be showing you today, that you can connect to Chachapiti.
And so the way that this works is, for instance, with the Xcode integration, you pair Chachapiti with Xcode, and then you can just have a conversation back and forth with Chachapiti, but it has all of the context of what's in your Xcode. And so in this example, you just say, add the missing planet. You don't have to copy and paste over the code. It can just read whatever's on your Xcode, like IDE, and it can take that in and it can make this adjustment and then give you the code that you need to put back into Xcode. And so it's a really nice seamless integration where the days of having to just copy and paste over context and make sure you had all the files right and bring it into Chachapiti and then bring it out, those are gone.
And we're working towards having this one-click thing where you just pull up Chachapiti and it already knows everything you're working on, and there's a seamless interaction throughout your computer. And so I'm super excited about it. I've been using it for pair coding, having Chachapiti just always know what I'm coding out and what I'm working on, and it's been really cool.
So I know I'm going quickly, we have so much to cover, but I want to just jump to a demo. So I'm going to pull up Sora first. So this is the new Sora.com user interface. And there's a bunch of cool things that you can explore here. But one is this featured page. And so these are just some of the videos created by the community that have been selected to kind of appear on this featured page. And they're usually pretty cool. So you can just take a look. There's some kind of more out of the box ones like this, not sure what's going on here. But then also some like, kind of beautiful natural landscapes, a panda and a guy in a room. I mean, these are all over the place, but it's pretty fun to just check out the featured videos. And you can also look at the prompt that created those videos for inspiration. But let's try making our own video.
So one thing about Sora is, you know, you can just type whatever you want into the description. But there's actually like a lot of guidance around how to make a video look best. And I would say generally what you want is like very descriptive prompts that give a lot of detail, because the modelers typically perform better.
when you give it more granular detail. And so I'm actually going to pull up Chachupiti to do this. And I'm just checking, can everyone see my screen? I realize I haven't been able to see any of you, but I'm hoping that...
Here's a screen, it looks great. Okay, so I am going to ask Chachupiti to write me a prompt for Sora. So I'm going to say, write a text description of a zebra in running through a winter wonderland. So I'm going to let Chachupiti come up with like a much more poetic description of this video.
Okay, this looks pretty cool. So now I'm going to go back to Sora. I'm just going to directly paste that in and I'm going to create the video. So while that's loading, I want to show you all the storyboard feature as well.
Storyboard's really cool where you can actually, if you think about the way that like a video would be made without AI, you might have the storyboard of the different key moments in the scene that you want to create, and you can do that with Sora as well. So I'm going to say here, start with like a drone footage of the Eiffel Tower. And then I want by the end of the scene, the Eiffel Tower takes off like a rocket into space. So that's what I want the kind of general arc of the scene to be.
One other thing you can do is you can add in reference images. So I could add in a video or an image, and that could influence the way that the visual actually works. But I'm not going to do that for this case. I'm just going to have these two instructions and I'm going to create that.
So these are both kind of working in the background and it looks like the zebra video is ready. So let's look at it. So it's going to give me two options. And I think that's pretty good. So I think, you know, there's not a lot of videos out there of zebras in the snow. I don't think there's any real videos that this is based on. So this is all pretty imaginative stuff, but that looks great. And then we'll let the Eiffel Tower video.
Okay, so it seems, yep, it's like a drone shot, zooming in. Seems to be some rockets outside of the Eiffel Tower, but let's look at the other option.
Okay, you know, it's not exactly what I had in mind. I could probably remix this or recut it, but it definitely is a rocket in the Eiffel Tower. So I like it for the first draft, but yeah, anyway, so those are two of the cool features. Other things you can do is you can change the aspect ratio, the quality, the time of the video. There's a ton, I encourage you all to explore.
And so the second thing I wanted to show is Canvas and ChatGPT apps. And so let's show those two together. So what I'm gonna do is I'm gonna pull up the notes app. And so let's say that I had taken this note where I had said, I need to email Kevin saying thank you for participating at the Tech Success Office Hours. I'm gonna tell him happy holidays, and let's throw in a pun about Christmas trees just for this email.
So what I'm gonna do is I'm gonna use the shortcut for the ChatGPT desktop app. There's a shortcut where you can pull it up on your screen. It's really cool. So if you do Option + Space, ChatGPT will now, as you can see, I have the desktop app open, but it would just pop up on my screen, and I'm gonna actually pair it now with Notes. So now it's working with Notes. I don't have to do anything else. It just now automatically sees whatever's on my Notes app. So I'm gonna say, make a Canvas for and write this email, and I'm gonna submit that.
So you can see what it's doing is it's gonna create a Canvas that includes the email, it writes the happy holidays email, and it should also have that pun that I asked for. So let's open ChatGPT and see what the canvas looks like.
Okay. So it's not the best pun. So anyway, this is what Canvas looks like. So it pops up this side menu. There's a couple of features I wanted to highlight. One is you can add emojis. You can add final polish, which edits it. Reading level, you can decide if you wanna keep it as graduate school or kindergarten. I'm gonna keep it at the current level. You can also adjust the length. So let's say I wanna make this longer.
I'm just gonna have it rewrite it, and it's gonna take another stab, but instead make it a longer email. This email, James, my AI is actually gonna summarize it for me anyway, so. Exactly. So then, yeah, we're gonna get to the point where AIs are just talking to each other back and forth, and there's no human involved. So it doesn't matter how long it is, I guess. I think that one thing I can do here is I can go in and do line edits. And so you can think of this as your personal copywriter.
And so I'm gonna say, make this pun better. Let's see how Chachapiti does with that edit. But then what it's gonna go in is it's gonna look at just a specific line and rewrite this.
Okay, I like that pun a lot more. So one thing that's nice about this is instead of rewriting the entire email, you can just specifically take parts of the actual output, and you can tweak them. And then one thing I'm gonna do is suggest general edits.
So it can actually edit itself. Okay, it wants to simplify its own pun. But you can see that it can go through. So I could copy and paste my own email, and have Chachapiti go through and provide edits that I can either apply or I can reject. And then finally, just for fun, let's add some emojis. So you can choose if you wanna add it for specific words. Let's put it as section.
So I want each paragraph to have its own set of emojis because I want Kevin to know how excited I am that he presented at these office hours.
Okay, great. We got some fun emojis. And so it's gonna keep all of the content the same. It's just gonna add in some of these emojis at the end of the paragraphs.
All right. So hopefully what's next is the integration with Gmail or your mail client. So I could just send this automatically. But for now, I could just copy and paste this into email and send it. But that is, that's it. So those are the three main updates for the customer-facing non-developer updates. And now I will pass it to Kevin to talk through some of the API-specific, developer-specific updates.
All right, thanks everyone. Let me stop sharing my screen. Thank you. This may take me a second. Kevin, it is all yours. Thank you. I'm gonna go ahead and share here. All right, where are we? There we are. All right, hey, that was terrific, James. Thank you so much. And thanks everyone for just dropping questions in the Q&A tab and also in the chat. It's been super fun to see you going back and forth. And also for some of the comments around, what's to come and excitement for what is. So I'm here to talk a little bit more about what is and what's new across the platform. On Tuesday, we held a little bit of a mini dev day where we introduced a handful of things, including more capable snapshots. So upgrading the intelligence of our foundational models. We also added new tools for things like model customization and upgrades that improve access, performance and cost efficiency for developers like you and I.
Some of the kind of highlights, if you will, that you're seeing up here was the GA of O1, our reasoning model in the API. And not only is it GA inclusive of an updated snapshot, but it has support for a lot of the functions that you'd expect to see, or a lot of the capabilities rather, such as function calling, developer messages, structured outputs, multi-modality with vision and reasoning tokens. So we're going to look at all this live in a demo in just some time. On the real-time side where speech to speech updates, we delivered some upgrades in the intelligence. So the base snapshot itself has been improved, which is really exciting. It addresses a lot of the feedback that you've provided to us and to date on terms of the model's performance. And we delivered some other really important things that we'll talk about that actually lowers the barrier for entry to building with these tools. We also delivered some capabilities around customization with preference fine tuning and new SDKs, which are always welcome to make our development lives a little bit easier.
Let's spend a few minutes talking about O1 together before real time and jumping into some demos. So just a bit of history, like how long has this arc been for these reasoning models? We delivered O1 preview in September this year, including a preview mini.
And it really unlocked a variety of computationally intensive workloads that previous frontier models struggled to achieve. And I think we knew when we delivered this to you and to the rest of the community that it lacks some core features that you might expect. Things like what we just talked about, function calling, structured outputs, multimodality streaming rate, all these things. But what's really virtuous about delivering these in a preview state is that we can collect your feedback on what use cases matter the most to you. And we can then optimize the models, optimize the services to better suit those use cases. And so we are constantly iterating, if you haven't experienced that already, to upgrade the capabilities that we're delivering early and then knock it out of the park when we deliver GA, as I think we've done here. We'll take a look at some evals.
Yes, O1 is GA in the API. And if you don't already have access in the API, we're continuing to roll it out to larger and larger bands of customers over the coming weeks.
And I think that in terms of the core highlights here, you have a more intelligent model measured by standard industry benchmarks and also some internal benchmarks on capability performance. But now you also have the ability to build intuitive agentic workflows with function calling where O1 is increasingly acting like the orchestration engine for agentic workflows. And of course, agentic workloads require some degree of predictability on responses. If you wanna interpret a response from an open AI so that you can do something with it, you'll need structured outputs in order to build your application. And structured outputs is also released as part of this GA.
Now, what's interesting is developer messages. There's a little bit of confusion around this one because developer messages is really just another name for the system prompt. And the reason that the naming shifted was because it actually adheres more to our published model spec. I think we published a model spec sometime in like September or late summertime. And of course, that is the ability to imbue an identity on the model and a sense of responsibility for what the model actually does. And some folks were asking like, how can I guide the model? I have a use case where I'm authoring books or articles. And this is always the first place that we tend to visit this with some meta-prompting in order to shape the.
shape the output of the models, right? That's always the lowest hanging fruit. Now, vision's huge. Vision's huge, not just because you want multi-modality support in your reasoning model, you know, in any kind of latest model, but also because of the quality of the vision in O1. And so, you know, I think the primary use case here is of course extracting insight from those images with high detail and precision. We're gonna take a look at an example in a second here.
And then last but not least, is this new concept of reasoning effort. And so this basically allows you to define how many computational resources you'd like to assign to a given task, encouraging the model to think harder about problems based on their difficulty. And so we'll show you an example of what that looks like in live code in just a minute here. So I wanna share this example with you. I think it's a good example of multi-modality and the difference between vision and some of our, you know, frontier models as they existed prior to O1's GA and where we are today.
And so we had this automotive customer who was looking to release a knowledge assistant both to the folks who are in-house, working on optimizing these systems and keeping them well-documented, but also to the people like my father-in-law, who's a machinist, who needs to actually maintain these systems after they're delivered to the customer. And so in the past, we were able to use 4.0 and, you know, using a six-shot prompt, we were able to get pretty acceptable accuracy in order to deliver this to production. But with O1, we're actually able to zero-shot it and get the correct output. So the extraction quality and precision is extremely high with, you know, with O1.
Going to like, well, the base model, you know, one of the kind of key axes of improvement of these models is obviously the core intelligence itself. And so I wanna give you perspective on where the latest snapshot sits in comparison to O1 preview. Just so that you see it, it's capable of solving more complex tasks. And I think where I would point your attention with notable increases in coding, math, and of course, with multimodality and vision. So there's some notable improvements there. And this benchmark performance is all available in the blog posts we're delivering around shipments. And so this is available to you if you wanna go back and look at it. I'm just being sensitive to time because we have quite a bit to demo here.
On capability evals, I also wanted to call out like, you know, these models are getting more intelligent, but that also means that they're more capable. And so one thing that really struck me when I was looking at the performance of O1 GA is internal function calling. And so I start to think about our customers who are rolling these, you know, rolling out these applications at scale. And you need almost absolute certainty that, you know, if I wanna hand off a call in a customer support scenario or retrieve information for search, I need an extremely high precision on my function calling. And O1 GA, you know, the latest snapshot is able to do that for you, among other things in terms of, you know, the quality of output and quality performance.
You might be asking yourself, I've seen, you know, some folks have asked me like, okay, what are some great use cases for O1 and what's its impact been to date? And something that I like to point at, although this slide's not super exciting, what's exciting is what it represents. If you look at scientific research, and The Economist wrote about this recently, which was basically that these AI models are accelerating scientific research authorship. Okay, and so you have a greater volume of these scientific research papers that are getting submitted for peer review by researchers, you know, using AI as kind of the backbone for their research in order to accelerate it. And their observation was like, we need to find a way to actually incentivize peer review so that we can actually process this stuff and ensure it's of sufficient quality before we incorporate it back in the general corpus of research. And I actually wrote into The Economist and, you know, and shared the idea that it's a very similar problem to what we see in software development, right? Where right out of the gate, AI like the O1 model does with this extraordinary coding performance has not only accelerated code authorship, but, you know, you're trying to stuff a certain amount, a certain volume of research or code into the same size pipe, right? This peer review or code review. And so now what we're seeing increasingly is actually the first pass of research review. And this is what I actually wrote in. And the first pass of code reviews in a pull request workflow are happening by the models themselves. Okay, and so it's accelerating authorship and it's also accelerating review. Okay, which makes this whole flywheel go faster, which means you're gonna have more software that's higher quality, more secure, delivered more quickly. You know, and same thing on the research side, more research that's higher quality, shipped more quickly. And I think that's great news for, you know, for humanity really. I don't know how else to kind of express it.
In terms of the other domains, there's some really interesting use cases I'll just share with you in financial services. One that comes to mind for me is I'm working with a handful of customers who are basically revolutionizing financial advisors where basically the financial advisor will have an awareness of, you know, your financial situation, macroeconomic conditions, the services offered by the financial service provider and any other folks that are in the market with them. And it's gonna provide actionable insight to you on, you know, how you could better manage your finances. So it kind of synthesizes all the information for you and presents it back to the user, not creating the insight itself, but synthesizing it and presenting it back to you. And that's really compelling. You think about a use case where, you know, you have like a credit card, you know, that has call it 28% APR, which I think is actually the average APR for a Visa MasterCard. Don't quote me on that. But so you have this credit card where you have a bunch of debt, but maybe you have some extra money lying around in a low interest savings or checking account. And so it's gonna do an analysis and say, hey, based on your spending patterns, you only need X amount of dollars as a safety, you know, as a safety net. You can take some of that money, apply it to your credit card and pay it off or pay it down. And that'll reduce your cost of carrying that debt over time. And so those are automated insights that are popping up for customers and increasing obviously their financial lives and their engagement with the financial institution. So similarly across insurance, legal, manufacturing, you know, great use cases. I'm not actually gonna dig into them, even though I think there's some really fun and interesting examples and support and using reasoning to argue complex ideas, case law against each other before, you know, ideas against each other before actually putting together your own legal arguments and manufacturing, which we spent some time on. So not gonna dive super deep in those, but just in the interest of time.
And let's shift topics just a little bit into the real-time API updates. We delivered some incredible improvements to the API, making it easier to use, more capable and intelligent. If you've ever built a WebSocket integration with any service, not just, you know, not just real-time circa pre-Tuesday, it's freaking finicky and it's hard to get right. And so what we delivered on Tuesday was this modality of WebRTC, which makes it a heck of a lot easier to build an integration between a client and this real-time service. And I think what's really exciting about this is not only is it a lot more accessible for you and I are the average developer, but it's also more performant because architecturally the way it works is you're not actually streaming. You don't actually, you're eliminating one leg of the request trip where basically the flow goes, your client, in this case, a browser, requests a short-lived token from a web service that you host. That web service goes to OpenAI, grabs a token, vends it back to the client, and the client then uses that token to directly interface with OpenAI, versus in the past where you actually proxied through your server. So you were taking your audio, streaming it to your server, then your server was packing that up and streaming it to OpenAI and back and back, right? Now we're just kind of going direct. So it's more performant and easier, and I can't think of a better, kind of a better world to live in where that continues to be the case.
Last slide, I promise, except for one that just says the word demo on it, is some improvements to the baseline cost and intelligence of the model itself. And so we delivered a new 4.0 snapshot, 4.0 audio snapshot, that's 60% cheaper than the previous one, and mini, we're cutting prices to one-tenth of, it's not yesterday, but pre-Tuesday's audio prices. We made it better at adhering to your guardrails, made it better at function calling and giving you the ability to more granularly control conversation, as well as extending the session length from 15 to 30 minutes, which I think is really great for support use cases or any of the use cases that I've already described. And of course, SDKs, I'm always excited when I see that our lives are being made easier with developer tools.
So I'm gonna go ahead and actually move into demo mode here for you. Cool, okay, so what I wanted to do with you is actually do a super brief implementation of a WebRTC application that uses the browser as a client to connect with the real-time service, just to illustrate how simple the integration can be to get off the ground. And I also wanna show you a coding use case where we're using O1 with significant reasoning capacity to evaluate our code for quality and security implications before it gets merged back into our main branch. So I created this repo, some sample code for you. Feel free to go to the Office Hours branch specifically. I'm actually gonna go ahead and drop this back in the chat here. And just in case you wanted to code along, if not, feel free to just watch. And then later, you can take this up on your own and do your development. And we'll obviously, I think we're gonna publish this recording live anyway. So let's dig into it. One more thing maybe that I'll share as we're rolling is just the documentation so that you have access to that as I'm sharing it. Cool, okay, so you should be looking at my browser, which says real-time API with WebRTC. So this documentation was obviously updated on Tuesday. And what I'm gonna do is I'm actually just gonna skip forward to some code samples that describe how to do the implementation. And again, we're gonna go ahead and do this thing together. I think we have basically like 10 to 15 minutes to get this done. And so we're gonna move pretty fast and hopefully not break things. So here we go. Let's do it together, a live coding. First thing you'll notice is a description of that architecture, highlighting some of the values and connection details, such as where the base service actually lives and which snapshot we're pointing to. Of course, you can look at the snapshot here and you can see the date on it. And that just is a good indication of whether or not you're using the latest. Now, you also have access to this ephemeral key. This lives for, I think, a minute before it needs to be refreshed. So your client and your web service are constantly gonna be going back and forth to do that. Now, the first code snippet you'll find on this page
is client-side JavaScript that actually instantiates the session between your client and OpenAI, as well as reaching out for that credential that our web service is going to vend.
So first things first, I want to make sure that I stand up that front end. What I've gone ahead and done is actually taking this little landing page snapshot, and I pre-prepared a little prompt to just make this a little bit easier for us. And you can just describe what that prompt does in a moment here. I'm going to drop the code in there.
So again, we have some client-side JavaScript, and we want to use that to establish a connection to our web service where we get a ephemeral credential, and also to OpenAI. And so what I actually want to do here is I'm prompting O1 to use Vision. So it's going to take its time to consider each element from the UI design, consider how it can be implemented, generate a landing page file that looks just like it, is fully responsive using Bootstrap, leveraging modern UX UI paradigms. I tried to get a little descriptive here just so that I didn't have to iterate on it with you, and that it would be already good enough to illustrate the point, right? When a user clicks get started, instead of getting started, we actually want to establish a WebRTC session. So let's go ahead and and get this design here. Okay, and so we're getting this code back. Is it ready to roll? Okay, cool.
So I'm going to go ahead and copy this, and I'm going to jump over to Visual Studio Code. So you should be seeing my Visual Studio Code page here. I'm just going to go ahead and create a new file, call it landing.html. I'm going to paste this thing in here, and I'm just going to go ahead and validate for a moment some of the things that I know to check for. So okay, we have some styling. We have Bootstrap being pulled in here, which is great. Some styles, powerful web hosting, Blazor is ultra fast, search your domain. How about connect with a smart agent today? Okay, and then we have the search button. Okay, and then we have in our JavaScript, we actually have the code that was provided to us from the documentation. It's going to call my local host, which is great, and it actually has support for cross-origin resource sharing. That's important so that I can connect to my local server and my local client. Awesome.
Okay, so without complicating this, that is our landing page. I'm just going to go ahead and use my finder to open it, and let's see what it actually looks like. Okay, cool. Powerful web hosting. Fair enough. Let me just see if it's okay. Okay, fine. Fair enough on a first turn. Let's go ahead, and maybe I'll click get started. Failed to establish connection. Okay, cool. That's what we expected to see.
Now, I want to take the second snippet of code from the documentation here, which again, it creates that ephemeral token, so you should have something like a Node web service is what this actually relies on, and so I've gone ahead, and I'm just going to go ahead, and actually, I have a second prompt for this one as well. So, I'm going to generate a Node express service. We'll take this, drop it in here, and then I want to take this code snippet.
So, basically, I just want to take the code snippet provided on our public documentation and make sure it supports the cores NPM module so that I could use it for local testing, and that it supports .env because that's not included in the code snippet, but it does try to use it. If you look at this code example where it says process.env, it just assumes that I already have that, and so thought about Node express for a couple of seconds. Cool. So, we got .env. We have cores. We use .env.config to set up our configs, and we use cores. Okay, those are the main things that I need to validate just having done this a handful of times before, and let's see what the web service looks like here.
So, I'm just going to build let's just call it TokenVend.js. We'll go ahead and launch that. Save that. Save that. Cool. Okay. All right. So, what I want to do now is actually run this thing for the first time, and so let's see if it runs on the first try. Node TokenVend.js. I don't think I need Node Fetch, actually, but I'm just making sure I have all my dependencies. You can find Node Fetch. Okay, fine. Fair enough. Cool. Okay. Now, we have the server running on port 3000. Let's go and just make sure that we can actually communicate with it and hit the session endpoint that we've created from our documentation. It's probably tiny for you, but the point of this is just illustrating that, okay, we have a successful connection, and now I want to actually just launch like make a connection from our client.
So, I'm going to go ahead and keep this running, and I'm actually just going to see what happens here if I what happens if I open up my developer tool and I click get started. Okay. So, it looks like it wants to use my microphone. Hello? I'm doing great. How are you? We can't hear, unfortunately, Kevin. Oh, you can't hear it. Okay, great. Well, it's working. It's working fabulously and is charming. So, yeah, feel free to try that one out on your own. I'm sorry you can't hear it. I was warned ahead of time, but I was like, oh, it's going to work. So, let's do this.
Let's go ahead and actually commit this code, and I want to show you the second piece, which is actually contributing this code and using the 01 model to do a PR review on the code quality and security.
So, we're going to go ahead and say git status, git add all, git commit working agent, git push. Actually, I don't know what branch I'm on here. Git branches. Okay. Git push original hours. Cool. Okay. So, this was working. Sorry you couldn't hear the voice, but the voice was there, which is awesome to show how quickly we can actually get it up and running for the first time. So, now we have in our repo office hours push five seconds ago. I'm going to go ahead and open up the PR. Feel free to come here, and since it's a public repo, you can actually comment directly on the PR if you'd like, and I'm going to go ahead and run this, and what should you see in the background? Well, we should see actually in our CI pipeline this quality check kickoff, and so this was just a separate integration that I built out for your convenience, which basically pulls the diff from your pull request. It processes a diff as JSON, sends it to OpenAI, says you're an expert code reviewer focusing on code quality and security, and it uses O1 in order to provide some feedback on the code quality and security of your PR, and it uses a high reasoning effort because I wanted this to be kind of, you know, pretty explicit about where the changes are that we want, you know, that we would want to make. So, this will run on every time we open a pull request against our main branch. If I go to my CI pipelines, I just see that I kicked this off now. It looks like it was successful, which is good news. All the jobs look successful. Let's go back to our pull request and see if we can find it there. I see some folks. Awesome. Juno, you're the best. Thanks for landing here. A bunch of people thumbs upping it. Feel free to do that, and so we got this, yeah, so we got this feedback from the GitHub Action, which is O1 actually analyzing the diff, which it says it returns an ephemeral key in JSON, presumably intentional with a WebRTC flow, and make sure it's done. Yeah. Okay. So, make sure that it's done in a way that is responsible. We have cores, which is great. Error handling, totally non-existent because we didn't productionize this thing. Some environment variables, package versions, et cetera, et cetera.
Again, when we start to look at the broader implications of these models, you know, not only is it accelerating things like scientific research, code authorship, but it's also having downstream impacts, and so it's actually like broadening the pipe and increasing that flywheel, so we're getting more software, more secure, higher quality, and the same goes for, you know, so many other places. So, that's what we have to share with you today. I think we landed pretty much on time. We'll take any thoughts, comments, questions.
Yeah, that was perfect, guys. Thank you so much. The timing was amazing, and the presentation was absolutely stellar. Kevin, I'm sorry. The audio didn't work. We're going to find out a way. We're going to fix that for next time. There are workarounds. We'll get it working. So, we actually have a lot of questions in the chat. I want to say thanks so much, James. You've gotten through so many of them. I'm going to start by asking some of the questions from the chat that haven't been addressed, but if you also want to ask your question in person, like you want to be spotlight, spotlight the way we are right now and ask the fellows your question, raise your hand, and I'll call on you, and we'll get you queued up to ask your question live. But until then, we will start with some of our unanswered questions in the chat, which might be hard to find, because James did a really amazing job getting through a lot of these. There was definitely one that I was interested. Okay.
So, this is from Nate Walker, and he's at the AI Ethics Lab. At the AI Ethics Lab, we're publishing an AI Human Rights Index. How might we use chat GPT to translate eight decades of human rights law into structured code? And James, I know you answered this, but I thought it's such a cool question that we could bring it up for the whole group.
Yeah, it's a really cool question. I think that that's the kind of thing, like trying to refactor something like a large corpus of either like law text or really any type of unstructured text into something that is like clear rules or code, and that's like strongly formatted, is a great use case for AI in general. I think that what I would recommend and what I recommend in the chat is you really want to use our smartest models for a task like this. This is a task where reasoning really comes into play. And so, one example that I like to reference is customer service is something that a lot of opening eyes customers are interested in building, something like automated customer service. And a big part of that process is taking these policy documents of here's what we do in different scenarios. And so, these are the policy documents.
that customer service agents will reference when they're dealing with an issue. So let's say I have a policy, if I'm a t-shirt company, I have a policy that if someone wants to return a t-shirt, I follow these instructions. And we want to take that, maybe it's written out in a bunch of paragraphs, and I want to translate that to something very structured and a set of rules that the LLM should follow. To do that, the best way that we found is to feed different chunks of that into O1, our reasoning model, and allow it to then create that structure, and then to go back and have O1 review itself and actually grade itself and say, here's actually, you might have missed this piece, this doesn't make a ton of sense, can you edit this language? And so really that self-reflection is really important as well. So what I would say is try to chunk up your eight decades into reasonable sections, feed it through O1, get the structured output, and then feed it again through O1 and say, here's what O1 made, here's the original document, any tweaks or updates you need or you would make to this. And that's just the general flow that I would suggest.
Awesome, James. Thank you for the details. And for everyone here, just so you know, at the end of the event, if you go to your messages tab, you'll see the entire thread of Q&A and answers so you can go back and observe them in async.
Andy, you're up.
Thank you so much. This has been awesome, you guys, I really appreciate it. And the changes are incredible, I'm still absorbing it. But my main question was because there's been those big changes to the WebRTC, are there any like quick shoot from the hip tips you guys have for people that want to implement the real time voice API into their applications? Anything they just need to know about like nice to know is that I know there's documentation, but anything that's outside of that?
I think that one of the sticky issues that I kept bumping into just when I'm doing my initial development, and I called it out, and you saw it in the code was on cross origin resource sharing. So like, as you're standing up a local environment, it's really nice to be able to kind of eloquently handle that. That was something that popped up along the way.
Yeah, I can jump into one thing that I found is a nice to know is prompt like, it's not a one to one kind of like, plug and play conversion from the text based models to voice. The one thing is prompting is often a little bit different. The models are based on 4.0. And there's now the 4.0 mini version that's available. But that's like the underlying engine behind it. But they've been post trained slightly differently. So what that means is you just sometimes need to prompt a little bit differently for the voice model, as opposed to the text model, also, because you might want it to behave a little bit differently. And you might want it to be less like, like, maybe the outcome, the output would be shorter. Because even though it's okay to read three paragraphs of text, it's not okay to listen to a voice model, say three paragraphs of text. I would say that's my biggest, like, like, caveat is just like, make sure you're you're reprompting and testing that.
And Andy, where are you joining us from?
I'm from Orange County, California.
Awesome. Welcome. We've never seen you before. Is this your first event?
No, I guess I've just been behind the scenes. More secret.
Okay, cool. Well, so good to meet you. Thanks for joining us today.
Thank you. We're going to take a question now from the chat, fellas. And this is from Louis. He's a student. And I believe you pronounce C-U-N-Y, is it CUNY, Queens College? Kevin, is that correct? CUNY?
You're in New Jersey. I mean, you know, anything across the river, we don't understand. So...
James, am I saying that correctly? CUNY?
Okay. So, Louis asks, are there any applications using OpenAI's ecosystem? Your team would be excited to see students implement in hackathons. Super excited to host Hack CUNY in 2025. I love a good hackathon, you know? So yeah, what would I like to see?
I think one area that's really been interesting to me, especially as I was just building this demo for this session here, was what O1 can do in the SDLC. And one of the things that I was really curious about that I don't know if anyone's done any benchmarking on is whether or not... To what degree O1 deviates from benchmark SAS tools, so security testing tools, so that you can actually get an understanding, like a really deep understanding of whether you can just use O1 as your application security testing as an individual or a small team. So that's one thing I'd love to see, is deeper integration in the SDLC and more creative use cases there. Because I know it can do a lot. I've watched it totally transform the space over the past couple years. And with the new models and capabilities, we need folks to think about new ways that you can accelerate the experience and make code better.
Thank you, Kevin. Yeah, to add to that, two applications I actually think that come to mind that are fun to hack with. I think Cursor is an obvious one we talk about, but it's the AI-first IDE. And so you can code and just talk to the models and have it code with you really well. The other one that I'd be curious to test out or have you all test out is TLDraw. And I'll post it here. I haven't spent a lot of time with it. I've just seen it a lot. And I know a lot of people love it. But you can pretty much just draw things and then have AI mock that up as a front end. It's a pretty cool, interactive way to work with our models. So I would love to see people try to make things using that. Mock it up as a front end and then take it one step further and automatically write code from it. You know, like carry it all the way through to deployment.
That's awesome. Yeah. And I don't know if you guys can answer this one, but we do have a teacher in the thread right now. Actually, several educators. One specifically through working with kids in K through 12. And there are some limitations to the free chat GPT tier. And one of those is just like the amount of times that you can chat with the bot over a few hours. I'm curious if you guys have any hacks for the students to work around that. And then I'll also try and find some other answers for him. But since you guys work with the technology every day, I thought you might have a hack for the kids.
Let's see. You jailbreak our controls, James?
I think that, yeah, so we're trying constantly to give more models to the free tier and also increase the rate limits. I think that it's hard given how many users we have to, like, jack up those rate limits. What I would say, though, is trying to use, I mean, I would say try to, if you sign up for the API, there's a certain number of, I think, credits you get initially upon signing up. I'm not sure. But I would say that, yeah, generally just be like bundle as much as you can into one query. And then maybe you have like one group plus account that you then share because that has much higher limits. There's ways. That's probably the easiest hack you could do.
I think that's really great. I'm sorry, Kevin. Go ahead. But that's great advice.
Yeah. I was just curious. I mean, we must have communities that serve, you know, like in broader education, especially in underserved communities. I'm definitely going to look into that.
Yeah.
Great. Okay. Next question we have time for from Peter Gostev, head of AI at Moonpig. Has anyone had success in taking thousands of conversations and extracting rules or guidance, for example, from customer service processes? Sounds similar to what James is suggesting.
Yeah, yes, that's a really common use case, actually, is like what we call transcript analysis. And it can be on really any kind of data. One common one is, you know, you take all of the days worth of calls that your customer service agents had and you take all of the transcripts. And usually what the pipeline looks like is you first summarize the conversation and you use our models to maybe like 4.0 or 4.0 mini to say, hey, take this conversation and like pull out the most salient points, the key topics. Then you might take a model like 0.1 or like a bigger, smarter model and feed those summaries in and say, like, take out like the key actions that happened or like the key behaviors that that occurred. And one thing you can do is if you know a certain set is like good examples of transcripts where it may be your best, best people, best agents working on it. It's been vetted. It's like, you know, that's good. You can then use 0.1 to say, create like a rule book or a playbook of like, here's how we should interact in these cases. And like, here are the the like the key components of a good customer service agent. And then if you have conversations that maybe went poorly or the customer like gave it a thumbs down or whatever it may be, you can then say here are things that we want to avoid. Here's like type of interactions that are bad. And you can kind of feed that through 0.1 and get get those those kind of instructions.
Awesome. Thank you so much, James. Guys, we have one more minute. So I'm going to ask one more question because there's another really good one in here. So Michael Spindle is an education, an educator and founder at Align, and he's a special education teacher and a non-technical founder leveraging real time to support populations with social communication disorders. How is the 4.0 mini release different from its other 4.0 peer? I guess because he's thinking about which one to use for his application. Kevin, you want to take that?
Yeah, I'm happy to. Yeah, I think the 4.0 mini release is just we deliver a smaller model, which means that it's more performant in terms of its speed, lower latency, lower cost, which should have a positive implication. You may see some degradation on the benchmarks in terms of intelligence because it's a smaller model. But if it's sufficient for your use case, then I would just say go for it, give it a test, run it against your evals. And, you know, if it works, great. If not, then you can always just use the baseline model. People love 4.0 mini, even though it's a smaller model. It's really good at a lot of things. So it's worth a try.
Awesome. James, Kevin, thank you guys so much. That was super rad. I think this might be the only place on the planet so far where the outside world has been able to have a live session in relationship to all of the new feature releases from 4.0.
OpenAIs or 12 days of OpenAIs. So thanks, guys. It's been terrific. Thank you. Thank you for being here. We'll see you guys again next year, so don't go too far, James and Kevin.
And for the rest of the community, this is our last live event. But for the first time ever, the OpenAI Forum published one of our talks on YouTube because it seemed like a really big deal and we wanted to share it with the world. So our talk, The Future of Math with O1 Reasoning, it featured the world's most renowned mathematician, Terence Tao, and our senior vice president of research, Mark Chen, is now posted on our YouTube. So if you wanted to share that with the outside world, we've never been able to do that before in the forum, please feel free to do so.
And that's a wrap for 2024. Thank you so much to Kevin and James for being here. Thank you to the community for showing up all year long. We hope we've really supported you guys this year. We'd love to hear your feedback. We're going to continue to support you in adopting these tools and making them more accessible for technical and non-technical members alike.
And I hope you guys all have a really lovely holiday. I hope everybody gets a little bit of time off, and we will see you in a couple of weeks. Thank you, and thanks for bringing us together, Natalie. Terrific job. My pleasure.