Red Teaming AI Systems
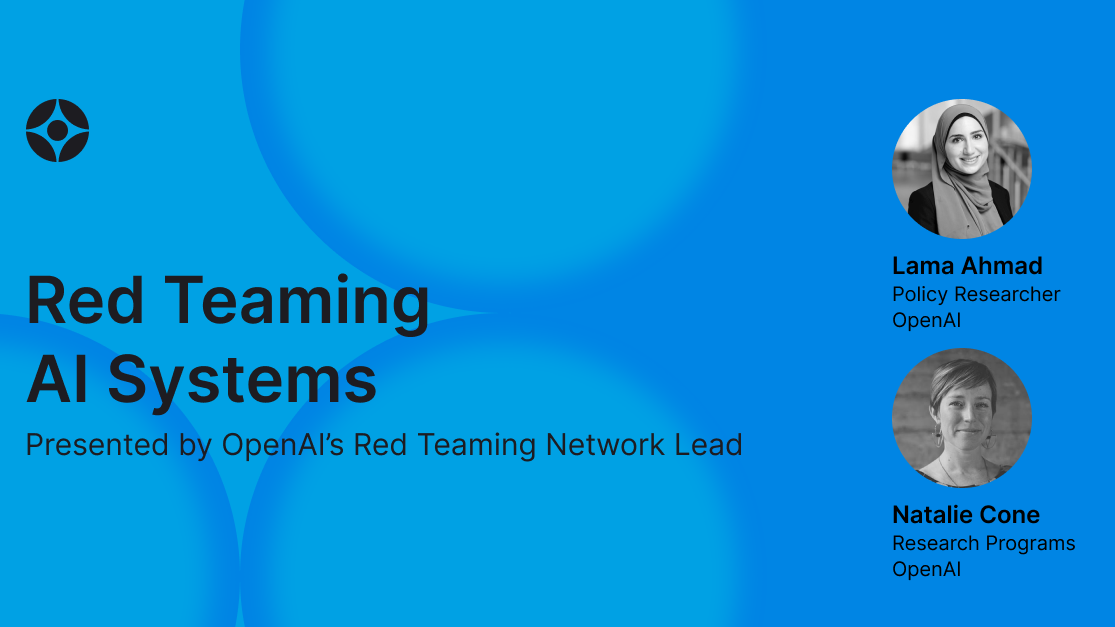
Lama Ahmad discusses the organization’s efforts in red teaming AI systems.

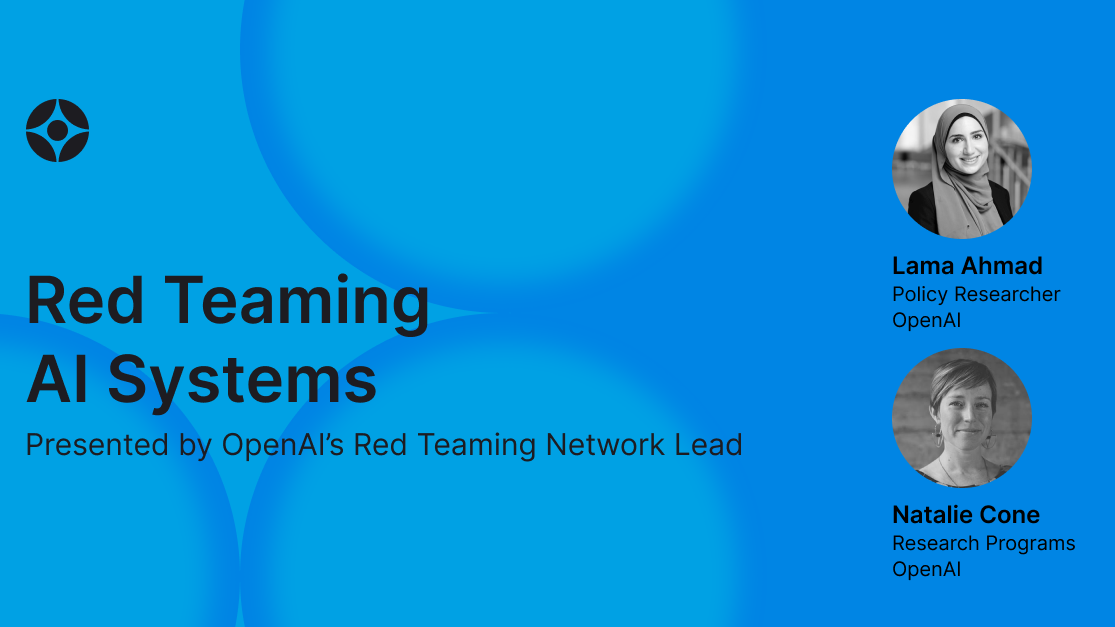
Summary
In a recent talk at OpenAI, Lama Ahmad discussed the organization’s efforts in red teaming AI systems, a critical process for identifying risks and vulnerabilities in models to improve their safety. Red teaming, derived from cybersecurity practices, is used to probe AI systems for harmful outputs and infrastructural threats, both under adversarial and normal use. Ahmad emphasized that red teaming is a continuous, collaborative process that involves internal teams, external experts, and automated systems to assess risks at different stages of AI development. With the increasing accessibility of AI tools like DALL-E 3 and ChatGPT, red teaming has become more crucial in identifying potential risks across various domains.
During the session, Ahmad shared examples of red teaming insights, such as the discovery of “visual synonyms,” which users can exploit to bypass content restrictions. She highlighted the importance of automated methods in red teaming but stressed that human evaluations remain essential for identifying nuanced risks. In response to audience questions, Ahmad discussed the role of red teaming in tackling misinformation and bias, particularly around sensitive topics like elections. She concluded by emphasizing the need for continuous improvement in red teaming methods as AI systems grow in complexity, with a focus on combining human expertise and automation to create safer, more reliable AI.
Key Takeaways
The talk, led by Lama Ahmad, focused on red teaming systems at OpenAI. Natalie Cone, OpenAI's Community Manager, began the session by introducing Lama and sharing a potential project opportunity related to cybersecurity. She emphasized OpenAI's mission to ensure that artificial general intelligence (AGI) benefits humanity. The main topic was the concept of red teaming AI systems, which is an important safety practice.
Key Points from Lama Ahmad's Presentation
- Background on Red Teaming:
- Red teaming in AI is derived from cybersecurity, but has evolved in the AI industry. It refers to a structured process of probing AI systems to identify harmful capabilities, outputs, or infrastructural threats. The goal is to identify risks to apply safeguards and communicate those risks.
- Red teaming considers adversarial use of AI, but also normal user behaviors that might lead to undesirable outcomes due to quality or accuracy issues.
- Evolution of Red Teaming at OpenAI:
- Ahmad described the growth of OpenAI's red teaming efforts, mentioning early efforts like the release of DALL-E 3 and later systems like ChatGPT.
- She emphasized the importance of accessibility in AI and how it impacts the risk assessments of AI systems.
- Lessons from Red Teaming:
- Red teaming is a full-stack policy challenge: It starts at the ideation stage of model development and requires collaboration across different domains, involving external and internal teams.
- Diverse perspectives are essential: To understand the potential failure modes of models, experts from various fields must be involved.
- Automated red teaming: Involves using AI models to generate additional test cases, but human involvement remains crucial for nuanced and domain-specific testing.
- Red teaming is used to develop mitigations and improve model safety at different stages of deployment.
- Challenges and Future Directions:
- As AI systems become more complex, the red teaming process will need to evolve, combining human-in-the-loop testing and automated methods to address new risks.
- The role of public input and collaboration across industries was highlighted, with OpenAI focusing on gathering diverse perspectives and applying them to develop safer systems.
- Examples of Red Teaming:
- Ahmad provided examples of vulnerabilities uncovered through red teaming, including visual synonyms (e.g., bypassing content restrictions by using synonymous terms) and potential misuse of features like DALL-E’s inpainting tool, which allows users to edit images.
Q&A Session
- Questions from the audience addressed concerns about red teaming in different industries (e.g., life sciences and healthcare) and challenges in balancing mitigation measures without over-sanitizing model outputs.
- Lama emphasized that red teaming is a measurement tool, not a definitive solution, and highlighted the importance of finding the right balance between safety and utility.
- Other topics included misinformation in elections, automation in red teaming, and contextual red teaming (how cultural and geopolitical contexts should influence model behavior).
Conclusion
Lama concluded by reinforcing the importance of iterative deployment and cross-industry collaboration in red teaming efforts, stating that while automated evaluations are useful, human involvement is still essential in identifying novel risks. She also emphasized OpenAI’s commitment to improving model safety through continuous testing and diverse stakeholder involvement.
The session wrapped up with Natalie Cone providing information about upcoming events and how participants could get involved in future OpenAI projects.
Extended Summary
In a recent talk at OpenAI, Lama Ahmad shared insights into OpenAI’s Red Teaming efforts, which play a critical role in ensuring the safety and reliability of AI systems. Hosted by Natalie Cone, OpenAI Forum’s Community Manager, the session opened with an opportunity for audience members to participate in cybersecurity initiatives at OpenAI. The primary focus of the event was red teaming AI systems—a process for identifying risks and vulnerabilities in models to improve their robustness.
Red teaming, as Ahmad explained, is derived from cybersecurity practices, but has evolved to fit the AI industry’s needs. At its core, it’s a structured process for probing AI systems to identify harmful outputs, infrastructural threats, and other risks that could emerge during normal or adversarial use. Red teaming not only tests systems under potential misuse, but also evaluates normal user interactions to identify unintentional failures or undesirable outcomes, such as inaccurate outputs. Ahmad, who leads OpenAI’s external assessments of AI system impacts, emphasized that these efforts are vital to building safer, more reliable systems.
Ahmad provided a detailed history of how OpenAI’s red teaming efforts have grown in tandem with its product development. She described how, during her tenure at OpenAI, the launch of systems like DALL-E 3 and ChatGPT greatly expanded the accessibility of AI tools to the public, making red teaming more important than ever. The accessibility of these tools, she noted, increases their impact across various domains, both positively and negatively, making it critical to assess the risks AI might pose to different groups of users.
Ahmad outlined several key lessons learned from red teaming at OpenAI. First, red teaming is a “full stack policy challenge,” requiring coordination across different teams and expertise areas. It is not a one-time process, but must be continually integrated into the AI development lifecycle. Additionally, diverse perspectives are essential for understanding potential failure modes. Ahmad noted that OpenAI relies on internal teams, external experts, and automated systems to probe for risks. Automated red teaming, where models are used to generate test cases, is increasingly useful, but human experts remain crucial for understanding nuanced risks that automated methods might miss.
Ahmad also highlighted specific examples from red teaming, such as the discovery of visual synonyms, where users can bypass content restrictions by using alternative terms. She pointed out how features like DALL-E’s inpainting tool, which allows users to edit parts of images, pose unique challenges that require both qualitative and quantitative risk assessments. Red teaming’s findings often lead to model-level mitigations, system-level safeguards like keyword blocklists, or even policy development to ensure safe and ethical use of AI systems.
During the Q&A session, attendees raised questions about the challenges of red teaming in industries like life sciences and healthcare, where sensitive topics could lead to overly cautious models. Ahmad emphasized that red teaming is a measurement tool meant to track risks over time and is not designed to provide definitive solutions. Other audience members inquired about the risks of misinformation in AI systems, especially around elections. Ahmad assured participants that OpenAI is actively working to address these concerns, with red teaming efforts focused on areas like misinformation and bias.
In conclusion, Ahmad stressed that as AI systems become more complex, red teaming will continue to evolve, combining human evaluations with automated testing to scale risk assessments. OpenAI’s iterative deployment model, she said, allows the company to learn from real-world use cases, ensuring that its systems are continuously improved. Although automated evaluations are valuable, human involvement remains crucial for addressing novel risks and building safer, more reliable AI systems.
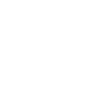