AI Art From the Uncanny Valley to Prompting: Gains and Losses

Dr. Ahmed Elgammal is a professor at the Department of Computer Science and an Executive Council Faculty at the Center for Cognitive Science at Rutgers University. He is the founder and director of the Art and Artificial Intelligence Laboratory at Rutgers. Dr. Elgammal has published over 200 peer-reviewed papers, book chapters, and books in the fields of computer vision, machine learning and digital humanities. Dr. Elgammal is also the founder of Playform AI, a platform for making AI accessible for artists. Dr. Elgammal research on knowledge discovery in art history, AI-art generation, and AI-based at authentication received global media attention, including several reports on the Washington Post, New York Times, CNN, NBC, CBS News, Science News, and many others. In 2016, a TV segment about his research, produced for PBS, won an Emmy award. In 2017, he developed AICAN, an autonomous AI artist and collaborative creative partner, which was acclaimed in an Artsy editorial as “the biggest artistic achievement of the year.” AICAN art has been exhibited in several art venues in Los Angeles, Frankfurt, San Francisco, New York City and the National Museum of China. In 2021, he led the AI team that completed Beethoven’s 10th symphony, which received worldwide media coverage. He received M.Sc. and Ph.D. degrees in computer science from the University of Maryland, College Park, in 2000 and 2002, respectively.
About the Talk: The use of AI in art making is as old as AI itself. The ways artists integrated AI in their creative process have been evolving with the advances of AI models and their capabilities. What is the value artists find when using AI as part of their process? What is the role of an artist and what is the role of AI in the process? How is that changing now with generative AI being dominated by text prompting as the way of interface? What have we gained and what have we lost with the progress of generative AI taking its course out of the uncanny valley to utility with the introduction of large language models as part of image generation. In this talk I will present my viewpoint about answering these questions as well as feedback from talking to many artists about this issue and trying to understand the ways they have integrated AI in their process.
So, let's commence with our program for the evening. I'm Natalie Cohn, OpenAI Forum Community Manager, and I'd like to open our talks by reminding us all of OpenAI's mission. OpenAI's mission is to ensure that artificial general intelligence, by which we mean highly autonomous systems that outperform humans at most economically valuable work, benefits all of humanity.
Today's talk, AI Art, From the Uncanny Valley to Prompting, Gains and Losses, is presented by our honored guest, Dr. Ahmed Elgamal. Dr. Elgamal is a professor at the Department of Computer Science and an Executive Council faculty at the Center for Cognitive Science at Rutgers University. He is the founder and director of the Art and Artificial Intelligence Laboratory at Rutgers. Dr. Elgamal has published over 200 peer-reviewed papers, book chapters, and books in the field of computer vision, machine learning, and digital humanities. Dr. Elgamal is also the founder of Playform AI, a platform for making AI accessible for artists. Dr. Elgamal's research on knowledge discovery in art history, AI art generation, and AI-based authentication received global media attention, including several reports in the Washington Post, New York Times, CNN, Science News, and many more. And in 2016, a TV segment about his research, produced for PBS, won an Emmy Award. Then in 2017, he developed AIKAN, an autonomous AI artist and collaborative creative partner, which was acclaimed in an Artsy editorial as the biggest artistic achievement of the year. AIKAN has been exhibited in several art venues in Los Angeles, Frankfurt, San Francisco, New York City, and the National Museum of China. In 2021, he led the AI team that completed Beethoven's 10th symphony, which received worldwide media coverage. He received an MSc and PhD degrees in computer science from the University of Maryland, College Park in 2002 and 2002, respectively. Ahmed, it is such a pleasure to have you with us tonight, and we're all here to hear from you. Enough of me speaking. I'm going to hand the mic over to you now.
Thank you for the introduction. And let me share my screen and start talking today. All right. All right. So the title of my talk today is AI Art from the Uncanny Valley to Prompting Gains and Losses. We're trying to basically build into what happened in AI coming to the art domain and creative domain in the last 10 years, where we are now, and what gains and what losses we have made.
I'll start from my lab, from my academic lab. Basically, I established a lab at Rutgers called Art and AI Lab about 11 years ago now. And my background is computer vision and AI person for the last 30 years. So if you look back 10 years ago, myself and any AI researcher would be very happy if computers would look at an image and tell there's a man, there's a woman, there's a car, there's a cat, there's a dog. So the best we could have done 10 years ago was just object recognition to some degree. That was a great achievement. But when we look at art, obviously art is much more sophisticated than that. There are layers and layers of representations and emotional effects and others that happens when you look at an artwork. So it's not only about objects. However, that intrigued me to really look at how we advance AI by looking at art and what AI can do for art and art history and understanding art history.
So these are some examples of things we have been doing over the years, where we look at artwork and build algorithms to understand the elements of art, the genre, the style, the composition, many, many elements of art and style. And we work on looking at artistic influences over the history, looking at creativity over the history and looking at how art evolved over the history.
I just want to talk about a couple of these before I dig into the topic today, because these are some relevant things to the topic today. So we have been working both in the analysis of art using AI and senses of art using AI, which is a generation which is more relevant to what we're doing today.
But on the analysis side, just a couple of results that are important. Back in 2014, we looked at how can we use the vision to analyze art history and discover influences. And here is some results. And it was very surprising that AI at that time could look at lots of images of art from the Western civilization and really pick up artworks that we never put them side by side and tell us that there is some influence here, there are some similarity, basically. Here is an example that make a big buzz in the news at the time, where in the left here, you can see an art by the French artist Basile and right here, Norman Rockwell. And if you look at them, these are about 80 years apart and different continents. And here I found them very, very similar and give us reason why they are similar. If you look carefully here, there are three men here, there are three men here, there's a chair here, there's a chair here, there's an oven here. There's a big window here, there's a big window here. Even the stairs going up here and there's a tilted frame going almost the same direction here. Even the composition of the scene, the four sides, the four quadrants here has similar four quadrants in the composition here. So it's striking similarity. So that might suggest that Norman Rockwell have been basically influenced by that particular painting in making this very different painting at the Barber Shop here. However, for me as a computer scientist and AI person, the real important part at this point was that AI can really look at art now and tell me something that I didn't know about it before. So no art historian could have ever put these two arts side by side and look at potential influence here.
A year later in 2015, I also made a work that's very, very important to the discussion today, which is, can AI look at art history, for example, and discover what are the most important artworks that changed the history, the most creative artworks, basically, in their time without any knowledge about art, just by looking at images and the date they were created. These are the only two information that we give the AI, the image of the art and what year it was made. And we build a system that's based on network centrality and to infer basically a score for every artwork in a zero-sum game. Some artwork has to score high, some artwork has to score low. And what we found is that basically AI could really highlight very important works of art at the time by itself, just by looking at the images and looking at which one influenced what and which one came ahead of its time. And that was amazing for me. For example, in this figure here, you can see Munch's The Scream scoring very high in the late 19th century. In the first decade of 20th century, you can see Picasso's Ladies of Avignon, which we're going to talk about today a lot, as the highest scoring one in the first decade here. And then you can see that Picasso and Procubism having higher and higher score until you reach basically the total abstract work by Malevich scoring the highest in the whole time, basically. So that's amazing, basically, of art history books or?
Yes. We use basically data collection from WikiArt, about 80,000 images from WikiArt representing art history from 1400 all the way to 2000. So that's basically at that time, that was the biggest available art collection online. Obviously now there are much more available art collection for research purposes. But that was a very good representation, 80,000 images is good enough.
Have you written anything that you could point us to related to this circular effect over the five years where we went from linear to planar, back to linear?
Yes. I mean, we have a paper called The Shape of Art History in the Eyes of the Machine was published in AAAI, 2019. So all this stuff is in there.
Okay. Any other questions, guys?
Oh, there's one. Kelly.
Yeah. Thank you so much for this talk. I'm curious how this research has been received by art historians.
One of my collaborators is an art historian, Professor Maria Mezzoni from College of Charleston. And actually that work has been really, really received. Earlier work like this, it was very controversial. The question we're asking here was controversial a little bit, talking about what are the most creative artwork. However, that relates also to some theory that's coming from art history. There is a book by an author called Kubler from, I think, the 60s that called The Shape of Time. And he mentioned a theory about how art improvisation work in terms of prime object and replica mass to explain basically how art movement, new art movement came around.
And basically this work actually kind of implemented this theory without actually knowing it at the time. I was later approached by art historian to explaining this relation. But that work anyway, called The Shape of Art History, that was really well received because it's really explained to something for art historian that we never really look for. But at the same time, it also, some art historian resources have actually cited something like that. If you look back to Wolverine, early art history research, he mentioned that art history evolved, or style in particular evolved as a rock going downhill. Basically, he tried to say that art evolved in a smooth way, style change in a smooth way, which is exactly what's captured by here, quantified here. So that actually was quite well received for that purpose.
Awesome question, Kelly.
I imagine also Dr. El-Gamal, because art historians, they take the formal visual analysis piece of their skillset so very serious, because it's cherished that this is one of the circumstances where we're kind of moving into a new age of work and skillsets where a computer can actually do the work that historically was reserved for people with very high expertise.
I guess you're right. We could continue to talk about this forever.
Yes. Yeah, I just want to mention this very important point. I mean, definitely art history in the last decade have been focused on social and other contexts of art making. However, informal analysis have been set back. And one of the things that we noticed now was AI coming to the equation is that more interest now is coming back to formal analysis of art history. And there was a book just by MIT Press, like a month ago, that really talk about this, talk about how art history now is coming back to formal analysis because of AI. So that's really a very interesting point.
Let me move forward to the AI generation side of things.
Obviously, with any new technology, artists take notice and artists start using this technology in making art, whether from the tools in cave painting to paint in Renaissance, to cameras in 19th century to digital art. And the trend usually is that with the technology, there are more and more artists coming into the scene. So that's really a trend. So AI is no difference here. So the use of AI in making art is as old as AI itself. So at the left here is Harold Quinn, who have been using AI for making art from the 60s throughout the 90s in a system that he built that's called Aaron. In the right here is Lillian Schwartz. She is actually a computer graphics pioneer who also have been experimenting with AI in making art for a long time. When you look at Harold Quinn, that's very interesting because it's very clear to what we are doing now, although it's very different. So this is one of the artworks by Harold Quinn. So just to give you an idea about the aesthetics. So the kind of AI that he was using at that time was mainly rule-based system. He write himself a lot of rules about composition and color and others, and let AI basically navigate through these rules through basically randomness and try to generate something interesting. And the kind of aesthetics here is very interesting, what he's looking for. So really the kind of aesthetics here is all about this unexpected or surprising result that AI add to the composition. And that's very relevant to the discussion today. So I'll come back to this. So in the last 10 years in particular, there are a lot of advances in AI that are used in art making. Back in 2015, there was a style transfer had been very popular. In 2016 came GANs. And then from that, lots of variety of GANs until we have DAL-E and other system that started using text to generate. And in 2022, it was a whole revolution of text prompting with DAL-E2, diffusion models, and many others. And nowadays we have stepping into beyond just two-dimensional to three-dimension and video and things like NERF and text to video and others. So a lot of progress happened in the last 10 years. And that's what I wanna analyze and look into in this lecture.
So here's earlier GAN. I mean, the very early GAN, Haber, actually, 2014 by Goodfellow. These are kind of images that was generated, very fuzzy, very hard to see anything that was really the silk art back then, almost 10 years ago. When these, for example, images of, typical images of bedrooms that are generated by GANs. And here are the first power. I'm gonna talk about powers used in improving AI image generation over time. The first power was adding convolution networks to the process in DC GANs. And that started giving us a little bit better results. Still very small, 64 by 64 pixel, very small, but you can recognize some figures here. So GANs, when GANs came around, the first question I started asking myself, does these GANs, do these GANs can make art? I have seen at the time some papers came around where they fed AI some images of art and start making images that looks like art. So that's the question.
If you feed these AI models, GANs in particular, images of art, what it will generate? It will basically emulate the art that you give it. But is that art? That's the question.
So first, usually at that time, when you feed it images of art, it will generate this kind of uncanny look images. And that's where the term uncanny valley came from, that the kind of results you are getting is really deformed figures. So here we train AI on a bunch of classical portraits and this is what it generates. It generates these deformed portraits. So that reminded me of Francis Bacon portraits in the bottom with a very fundamental difference. Francis Bacon has the intention of making the portraits deformed like that for some reason to reflect on the character or personality or maybe other reason.
Very photorealistic, and that was a real change here. However, if you look at StyleGAN, also what it added to the equation is controllability. You really can control many things in the generation. You can really control many aspects of the faces that are generated here.
However, the reason of success of StyleGAN was really that it is really working on faces where all faces are aligned and all faces basically have the same size and the same location. If you try to use StyleGAN in any other domain where this alignment is not there, it will not really give you anything different from a typical GAN. Maybe still high resolution, but it cannot really make any good figures. So that was a limitation.
The next superpower, but before that, back in 2019, if you look at GitHub and search the word GAN, you would find more than 30,000 codes of variation of GAN. So GAN was very popular at that time, so many variations, so many contributions, so many important things happening in the GAN results. Until we started to add text to the generation. Here I'm mentioning DAL-E as one of the pioneering work on adding text to image generation. Obviously, there was other works at the same time and earlier that added text, but nothing as good as what we have seen in DAL-E first version. And that makes a revolution of the potential of what can we do when you add text here. So I call this the power of adding language, because really here everything changes.
When you add language now, before adding language, any image generator will just manipulate pixels without any knowledge of what it's generating. It's just a bunch of pixels with colors. So once you add language, you're now controlling the generation to be having language in control, having language in the driver's seat. Everything, every pixel has to be generated through the language lens. So that's really a major, major thing. And I'm going to talk about this a lot today.
And then the next superpower came in the last year or two, basically, where diffusion models and training these models on billions of images came around with DAL-E2 and stable diffusion and others, and show us the power, the potential power of this. By the way, I totally believe that the power is not actually the diffusion process that's widely used now, because there are other work that the GigaGAN from Adobe that also achieve a similar quality, although it's only a GAN. So it's not the diffusion that's behind this. The main reason behind this is really the use of billions of images, the fact that you created billions and billions of images and captions, and it really generates a very good quality.
So what did we gain and what did we lose in this journey from the early time of GANs until today with DAL-E2 and even DAL-E3 now? What did we gain and what did we lose? So on the GAN side, there are many, many things. Obviously, the first GAN is not described here, which is the fact that millions of users are using ImageGenerator every day. The fact that we have a descriptive UI through text prompt that allow any user, not necessarily an expert, to write a prompt and start generating something. High resolution, high fidelity, definitely we get out of the uncanny valley. We get things that are really clear and clear figures and understand what's happening here. But on the negative side, there are lots of questions. The public relation, deception, the ethical issues, artist identity, losing the uncanny valley is the good thing or not, the limitation of text prompt, the controllability, and many other things that I want to discuss.
So what I'm going to talk about today is backed by two artist surveys, one that we did early in 2019 when we talked to artists about how they use AI, and another one just this year when we talked to artists about text-to-image in particular and how they're using it and what they like and what they don't like about it.
So we definitely went from the uncanny valley to these really great figures. Through having a constructive text control aesthetics. So here, before 2022, that's the uncanny valley basically generation. And once we come after that, really ArtGenerator now becoming a commodity. Now anybody can be using ArtGenerator, not only artists, anybody doing installation, doing content generation, doing design, can start exploring the use of these things. I counted over 100 image generation websites now that are using different APIs available from open AI and other companies to provide this kind of image generator to consumers. So now it's becoming a consumer kind of play.
In terms of public reception, we have seen the early welcoming of AI art, but now in 2022, we start seeing basically some artists community banning the use of AI art on Reddit and others. And that's really a question for me. Why? Why there is this backlash now about AI art? And what is the core reason for that? Mostly people cite copyright issues. But is it the real reason?
So, for example, there have been some lawsuits against some companies. And here from this Art in America article, I'm quoting one of the lawyers, basically, why they think there is a copyright infringement. They're saying basically the AI training process called diffusion is a suspect because it requires images to be copied and recreated as the model is tested. This alone, the lawyers argue, constitutes an unlicensed use of protected works. Well, obviously, this is still something that's going to be debated for a long time in courts. But is it true? Is the use of lots and lots of images to train the model, and the model is being trained by trying to reconstruct the images, is that violating copyrights? I believe not, because basically we humans do that all the time. We look at art all the time, and through the psychological process of mere exposure, we digest all the artworks all the time. And all that really affects the way we make art. Even artists at school, as part of the practice, they practice copying certain art and certain drawings and things like that. So where is the copyright exactly? Is it in the training, really, or is it in the generation? That's really the question.
But what is more important to me to understand is really the issue of AI and artist identity. So what's artist identity? Basically, the artist identity is very fundamental for art making in general, because every artist has to have their own style, their own way of making art. Other than that, they're not going to be called an artist. This is a very fundamental thing in being an artist. So how the identity of an artist themselves can come through when using the same kind of AI tool? That's really a big question. How can the same AI be used by different artists, and yet their soul and artistic personality can shine through the layer of biases existing in the data used to train the AI? This is not a new question. So actually, this question was also raised in the 19th century when photography came, and it was very fundamental to really accept photography as a medium. And now we have to answer this question again in order to accept AI as a medium.
So here is a case from the French court back in 1861, where a famous photographer at the time, Meyer and Bierson, have quite famous photography of portraits, and some other photographers started to copy the way of making photos. And they went to the court to ask for copyright violation, and the court said, no, basically, there's no art here. Basically, you're holding a camera and pressing a button. Where is art? That was a fundamental question. So it all came down to that one thing. Is the artist's identity there? Can I prove as a photographer that this is my photo that I made, that reflects the way I compose the picture, the way I take this photo, the light, everything? So can I really show that there's something about me making the picture that's very different from anybody else? So here I'm quoting from Aaron Scharf's book, Art and Photography. For photography to be considered an art,
All at the same time generate all the same image and they start claiming that the others is basically copying their work. And here's a big problem, is it really a copyright violation, copyright violation of who? Both of them have used the same system to generate an artwork by tweaking the knobs and generating an image, so how they can claim that's their copyright to start with? And if I, me and you are using the same system and accidentally generate the same image, where is the identity? What can we claim about that?
So the question is, does the use of AI preloaded with content reduces the role of artists to curation and playing around with knobs which although deceivingly allowing artists to mine through an apparently infinite choices fundamentally limit expressing their identity? So this was supposed to be a question but I mean you can also see it as a statement.
So when you replace that now with text prompt, we have the same situation although it's much harder to generate exactly the same thing. But if you plug in, your knobs now is really the text prompt and maybe the other variations and the AI model is really be trained also with lots and lots of images and you generate images.
So here's a situation now, if two artists enter the same exact prompt, it's with very low probability that you're going to have the same exact image. But why are you going to get different images? It's mainly because this system use a random generator as a seed for the generation. So if me and you use the same exact prompt, we are getting two different images mainly because two random numbers have been used in the initiating the process. So it's not because of any action that I did or you did. So here that really put a big question about the artist identity. Where is the artist identity here? Yes, we can do a lot of amazing things with prompting, we can really engineer the prompts to generate amazing things, we have seen amazing things with that. But again, is this the art now? Is the art now just manipulating the words? That's really the question.
Now let's talk about text prompting itself and its limitations. Any questions before I move forward?
I have a question. I'm curious about, do you think that that notion that you just described about the limitations, because you have endless access to all the data that the model's been trained on, there are no more limitations. And lots of times as an artist, the limitations and the confinements are really what the forcing function to great creativity. Do you think that that argument or that statement is going to be more front and center in the near future? Because really, this is one of the first times I've heard that.
I mean, in terms of the limitation, I'm still going to add some of these fundamental limitations that you mentioned. Here, I'm just mentioning one limitation about art's identity. What can we say about art's identity? I'm not taking sides, but I'm just raising a question about art's identity. But definitely, there are some creative limitations that still I want to talk about. So let me jump into that if there is no other questions.
So what are the limitations of text prompting? Text actually definitely helped improve the quality of generation and help us going out of the uncanny valley. But now, we only can generate through the lens of language, which means that basically, whatever I want to generate, I have to write down this in terms of a prompt. That's really a very big limitation for artists, because first, artists are visual people. Second, language is a higher construct of intelligence than visual perception. So you don't need language to make art. You don't need language at all. Language is a way to communicate, where art is a visual way of communication that doesn't need language. So when you put a language as the main lens where pixel has to be manipulated to generate art, you really limited a lot of what can be done.
For example, how can we write a prompt to generate 20th century art? 20th century art has been fundamentally about non-figurative art. So here are some of the major pieces of art from the 20th century. There are many, many I can put here on the screen. And I'm asking you, how can you write a prompt at that time, at the time when Picasso made that artwork, or when Rothko made that artwork, or when Dali made that artwork, that can generate that artwork? Yes, now we can do that. We can tell the machine, give me an artwork in the style of Dali that has a deformed clock, and it will spread this out or something like that. But because it has seen Dali and has seen what he was trying to make. But imagine Dali himself at that time has to take a prompt and try to generate something like that from what happened before in history. That is almost impossible. It's very hard to explain any of that and many other examples of what's happening in 20th century art in particular using a prompt.
Not only because 20th century art means mainly about non-figurative art like color painting and action painting and abstract expressionism. But many other reasons, even for figurative art in 20th century, it was a study about getting away from the typical formal elements that we have seen before. And big part of that is photography that came around in 19th century and artists tried to find different ways to do that.
Fortunately, we are out of this formalism in the late 20th century and art now is more figurative and that's really make AI coming now into the art scene where it is ready to embrace it. Because now art is totally more figurative from the 90s.
So as I mentioned, we conducted a survey asking artists and creators in general that use text to image in particular, what do you think about that? We have many questions in the survey. I just want to show you some of the results. So 46% of the respondents found such tools very, very useful. 32% of them found them somehow useful but could not integrate them to their workflow. And 22% find them not useful at all. So that's good. So almost half the creators find them useful. And when we dig deeper about what is the main limitation artists find in the process, they mention basically controllability. The fact that really they cannot control the outcome just using text prompts. Because art, as I mentioned, is a visual language, composition is the main thing. So it's very hard to get the AI to do what you want just using text prompts. 50% of the respondents think the results are interesting but not good enough to be used in their practice.
How these tools will affect artists' practice. 90% of artists surveyed think that such tools will affect their practice. 46% found that effective to be positive. And 7% found that effective to be negative. Only 1.4% think that these tools will kill their practice, which is really good news. So most artists don't think it will kill their practice at all. It's a tool they can use. So there are a lot of positive signals here. And the negative things here really can be addressed.
Now I'm going to talk about the difference between making art versus making images. I believe AI art now is really good at making images, but not at making art. And here I want to talk about the story of this painting here, The Ladies Avenue by Picasso. This is an artwork done by Picasso in 1907, early 20th century, more than 100 years ago now. And when Picasso did this, it was very, very shocking, even for his artist close in his circle. He kept it basically in his studio. Not many people have seen it. But that was the beginning of cubism. Here you see how Picasso started flattening the plane and formed the figures, had the influence of African masks. And this was really the very early experiment of cubism that five years later or something like that became a major art
pushing the boundary here. We tried, even unintentionally, just generating things at that region, the negative hadonic, or on the boundary of both the negative hadonic. And that's an interesting region. That's exactly the region where Picasso was playing around when we made that art.
So that's really the source of surprise or arousal that comes from AI art at that time. And that's why the AI was very well received at that time.
Now, with text prompting, these are amazing images. But what is the source of creativity here? For example, if you look at this, the prompt here is an espresso machine that makes coffee from human soul. So the creativity here is really the prompt. The user now is in control of the creativity by writing out a prompt that combine concept that you never thought about together, and that explore what the machine can generate from these prompts.
So basically, the source of surprise is very different. Here, it was the aesthetic of machine failure that was surprising and interesting to artists. Here, it's mainly the user writing interesting prompts, and the creativity is in the hand of the user by writing these prompts, not in the system itself. The system itself has no creativity. The system itself just combine the words correlated with pixels to generate an image.
So both of them, the system has no intention of making anything creative. Here, it's just machine failure. Here, it's just follow the prompt, and the creativity is in the prompt, not in the machine.
In both cases, the system are designed and built by construction to follow the training distribution, looking for typicality. So in a sense, basically, the system is designed to really go counter, to be counter-creative, to go closer to the zero in this curve here. If you just generate from image net, without having any interesting prompts, you're gonna get very boring images, basically, something in here.
So I totally claim that at this point, AI art has a counter-creative bias, and creativity is all about how human using it in a creative way. And there's a big way to move forward, to make alignments between this AI system and what can we do.
So now I'm going to an older survey that we did back in 2020, before this text prompting at all. When we look at why AI artists using AI, or how artists are using AI in particular, what are the value?
So here's some of the conclusion of this work. I'll try to basically use this to conclude my talk. Artists understand AI as a major impetus to their own creative processes, in allowing them to generate lots of images very quickly, and suggesting new paths of manipulation and disruption of data to create images. For them, it's a vital step in leading them to seeing their own artwork differently.
And the main values that artists found in using AI and making art is, number one, creative inspiration, that give them new ideas that they never thought about. And number two is creative volume, the fact that it really can create things to them very, very fast and very efficient. So it's like having an artist collaborator with you that can help you create things fast.
But when you look at systems today and compare it to Aaron from 60s, 70s and 80s, and by Harold Cohen, there is something common between them. So there are actually several things that are common between them, which is, again, creativity is not in the system. Creativity is in really, the main source of creativity here is randomness. The fact that the serendipity search that surprised us with something that was not expected, but nothing by construction in the system that we can create it.
Last note, I wanna talk about, I know that I have stuff here, I just wanna have this question. How to deal with stagnation in art if everybody starts using AI art tools in making art?
So now if we, all these art tools have been trained on lots and lots of images that the human can create it, and now they're generating amazing work of art or images where user have very creative ideas how to use them. However, what that makes in terms of the future of art, if many and more and more user have been start using that, and many artists have been using that, many designers, many illustrators start using that, that what that tell us about the future of human creativity itself, are we gonna go to a bit of stagnation where basically we keep recycling ideas? That's really a big question because this system, as I mentioned, is not pushing the boundary, human was pushing the boundary historically in art making, but these machines are at this point, just try to generate things following the same distribution.
So we are not pushing the boundary. So some work on alignment has to be done here to really align AI systems with the human values in the creative domain.
So I just stop by just saying that AI is getting better in generating images, but is it coming to be less useful in making art? I'll keep that as a question.
Thank you very much.
That was amazing. So much to think about, and we talk about alignment so much, it's top of mind at OpenAI, Dr. El-Gamal. So I hope we can continue that conversation, but we are a little over time, but if anybody has any questions, I would love to hear from you. Also, if you think of questions later, this chat is gonna live forever in the group channel, and you can add to it, and I can connect the question with Dr. El-Gamal and get an answer for you later.
Thank you, Dr. El-Gamal. That was really awesome. I think this was one of selfishly my favorite talks that we've hosted in the forum.
So we're gonna take a short break next month, guys, and we're gonna pause the events to do some strategic planning for 2024. But December 7th, we'll be hosting Karen Kimbrough, the chief economist at LinkedIn, and she will be presenting the second future of the workforce in Preparing the Workforce for Generative AI, Insights and Implications series. So you can join us for that.
And once again, Ahmed, thank you so much for joining us. That was an awesome talk. I will definitely get this talk translated into a long-form article so we can have it forever in the forum. And we'll have this recording posted in about a week if anyone wants to share it with other members that weren't here.
And I hope you guys have a wonderful evening. And if any questions surface in the wake of this event, feel free to share them with me in email, and I'll connect with Dr. El-Gamal to get the answers for you. And you can also reach out to Dr. El-Gamal yourself and DM him in the forum if you have any questions that you just felt too shy to ask while you were here or came to you later.
So I hope everybody has a wonderful evening. I will see you guys all soon. And thank you again so much.
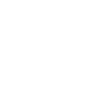