AI Ethics in Action: UC Berkeley’s Data Science for Social Justice Workshop
speaker
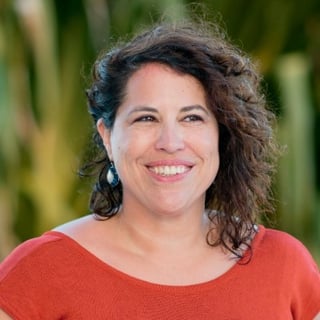
Claudia von Vacano, Ph.D. is the Founding Executive Director and Senior Research Associate of D-Lab and Digital Humanities at Berkeley and is on the boards of the Social Science Matrix and Berkeley Center for New Media. She has worked in policy and educational administration since 2000, and at the UC Office of the President and UC Berkeley since 2008. She received a Master’s degree from Stanford University in Learning, Design, and Technology. Her doctorate is in Policy, Organizations, Measurement, and Evaluation from UC Berkeley. Her expertise is in organizational theory and behavior and in educational and language policy implementation. The Phi Beta Kappa Society, the Andrew W. Mellon Foundation, the Rockefeller Brothers Foundation, and the Thomas J. Watson Foundation, among others, have recognized her scholarly work and service contributions.
SUMMARY
The Data Science for Social Justice Workshop (DSSJ), organized in partnership between UC Berkeley’s Graduate Division and D-Lab, is an 8-week program aiming to provide an introduction to data science for graduate students, grounded in critical approaches of data feminism, data activism, ethics, and critical race theory. Attendees receive training in natural language processing and leverage their skills to conduct discourse analysis on social media data in an interdisciplinary project. This workshop, about to conclude its third year, has trained over 75 graduate students across 20 disciplines. These students form a community of interdisciplinary scholar-activists who uphold a values-driven approach to data science and machine learning.
In this event, Claudia von Vacano, Ph.D., Executive Director of D-Lab, introduces the Data Science for Social Justice Workshop, highlighting its goals, structure, and outcomes. Then, three students who have participated in the workshop – with diverse and rich personal and academic backgrounds – present lightning talks on their experience with DSSJ, highlighting their personal journeys, the projects they worked on, and what they gained from the workshop. The event will conclude with a Q&A and discussion on how workshops like DSSJ present novel opportunities to train a generation of interdisciplinary, diverse data-driven scientists who center values and social justice at the forefront of their work.
TRANSCRIPT
Well, everyone, most of you know me. I'm Natalie Cone, your OpenAI Forum Community Manager, and have been since the initiation of this community a year and a half ago. I like to start our talks by reminding us all of OpenAI's mission. OpenAI's mission is to ensure that artificial general intelligence, AGI, by which we mean highly autonomous systems that outperform humans at most economically valuable work, benefits all of humanity.
Tonight, the OpenAI Forum is hosting some of the founding members of this community, the Data Science for Social Justice Workshop at UC Berkeley. I'm going to let their Executive Director tell you more about the program in a few minutes, but I just wanted to share that having them present for all of us in the forum for all of the events and here tonight to present throughout the past year and a half, just getting to know you all has been a real honor. I have really loved getting to know you all. I love all of the support that you've presented us in the forum, and I hope that we are able to continue to give back to you the rest of this year.
I can't wait to hear the student presentations. But first, let me give you a sneak peek into the rest of the year here in the forum. We're going to establish a regular cadence of presenting OpenAI research once a month in the forum, because we have heard from you all that this is really important. We know that the OpenAI website lists an index of research, and you can read the papers, but it's much cooler to be able to engage with the research scientists themselves and ask questions one-on-one. So we're going to bring that to the table in the forum.
We're also going to be focused on ensuring that our members working, learning, or performing research in higher education for the rest of the year feel that OpenAI technology is accessible. We want to demonstrate how useful it can be to support your research and simple to implement into your daily workflows and analysis. We're focused on sharing positive use cases with our members from higher education to give ideas and successful examples of how OpenAI tools have advanced higher education research and mitigated some of the administrative burdens for deans and administrators.
We're going to offer educational webinars, which is what Claudia and I were just discussing, and we're also going to offer technical support via solutions engineer office hours. I would like to share that we would also love to have your referrals for the forum, because for the rest of the year, as we are focused on higher education, if you have peers that are faculty members, lecturers, graduate students, postdocs, you think would benefit from what I just discussed, please share the referral link with them, and I would be happy to include them in these discussions and these really intimate events that we get to host in the forum.
Last but not least, for all of you who have recently joined the forum, if you're interested in participating in AI training, which many of you here have already done, please make sure that you indicate in your profile field that you want to contribute to OpenAI research, because this is what our new program manager, Wyatt Thompson, uses to identify the members of the forum that want to contribute.
Finally, I want to thank all of the developers in Santiago, Chile, some of which are new members of the forum and here today for warmly hosting me this weekend. I saw some of you trickle into the audience, and I'm really thrilled to have you here. The team and I are going to host weekly office hours starting next Friday, so if you're a new member, please attend and let me know what kind of programming you'd like to see in the forum, and we'll make it happen for you eventually. Some of these things take time, but we're always listening.
So let's get this party started and focus on the reason that we're all here tonight. It's a huge honor for me to introduce our guest this evening, Claudia von Vacano.
Dr. Vacano is the founding executive director and senior research associate of D-Lab and Digital Humanities at UC Berkeley, and is on the board of the Social Science Matrix and Berkeley Center for New Media. She's worked in policy and educational administration since 2000, and at the UC office of the president and UC Berkeley since 2008. She received a master's degree from Stanford University in learning, design, and technology. Her doctorate is in policy, organizations, measurement, and evaluation from UC Berkeley. Her expertise is in organizational theory and behavior and in educational and language policy implementation. The Phi Beta Kappa Society, the Andrew Mellon Foundation, the Rockefeller Brothers Foundation, and the Thomas J. Watson Foundation, among others, have recognized her scholarly work and service contributions.
I also really love sharing space with you, Claudia. It's really wonderful to have you here and truly an honor. Thank you for joining us in the forum tonight.
Thank you so much, Natalie. We're so appreciative of this invitation and of being invited into the Open AI Forum community. It's been enriching. Hello, everyone. I am Claudia Natalia Fombacano. At age eight, I came to the United States as a political refugee due to my father's persecution in Bolivia. His work as a journalist and as a political novelist put him at risk and led us to flee the country. This upheaval significantly impacted my education, particularly because I was placed in low academic tracks early on in the United States. These experiences fueled my passion for educational equity, and this is what motivated me to work on the UC Berkeley research and curriculum we will be discussing today.
Before we continue, I want to do a land acknowledgement that comes from the Berkeley Center for New Media. We recognize that UC Berkeley is located in the territory of Huichin, the ancestral and unceded lands of Chochenyo-speaking Ohlone people, specifically the confederated villages of Lisjan. The history of prolific technological development in this region has always depended on this land, and all of our technological infrastructures and activities take place on and in relation to this land. We commit to supporting the sovereignty and ongoing stewardship of this place by Ohlone people through building long-term reciprocity and relationship with tribal leaders and organizations.
For the past five years, I've researched diversity in data science and developed machine learning models aimed at reducing bias and increasing transparency and explainability. I believe diversity is essential in data science because diverse teams bring a range of perspectives, leading to more innovative and equitable solutions. In our Data Science for Social Justice program, we teach students that data science impacts real lives, and diverse teams are crucial for recognizing and mitigating biases.
Despite the importance of diversity, women are still less than 29% of the data science workforce. Black and Latina professionals represent around 7%, respectively. Addressing these disparities is not only a matter of equity, but also a matter of improving the quality of outcomes in the field. The Data Science for Social Justice program is a transformative initiative that combines critical pedagogy with practical data science skills to empower minoritized students. Built on the principles of personalized learning, small cohorts, and mentorship-based education, DSSJ ensures that students, particularly from marginalized backgrounds, thrive in data science.
Participants are trained in Python and natural language processing with little prior experience and engage in discourse analysis projects on Reddit. Alongside technical training, they explore critical theories, fairness, and ethics in data science, forming a community of scholar-activists dedicated to social justice. This unique approach promotes inclusion and fosters the holistic development of future leaders in data science.
Today, we're asking for your support, support from funders and individuals like you. Your support will allow us to sustain this vital program, nurturing a new generation of data scientists committed to creating meaningful change. And I believe we're dropping the URL in the chat where you could give a gift. DSSJ is a workshop aimed to train a cohort of budding scholar-activists in data science methodologies, grounded in a philosophy of social justice. Overall, the course is designed to provide students a highly structured and supportive computational and socio-technical training in data science, while forming a community of dedicated scholar-activists.
to express my deep appreciation to Dr. Elisa Garcia Bedolla and Dr. Denzel Street for championing this program. And from the Graduate Division, I want to acknowledge Kara Genter's tremendous expertise and leadership. From the D-Lab, I want to acknowledge my close collaborator, Prateek Sachdeva, who is always brilliant, patient, and kind. This work grew out of Tom Union's digital hermeneutics course. And Renata Barreto guided the selection of reading, secured speakers, and led the reading discussions. Next, I want to acknowledge our instructional team, Helena Nogatu, Mingyu Yan, Farnam Mohebi, Stephanie Andrews, and Violet Davis. Many of these folks in our instructional team this year came through the program and stayed in the D-Lab orbit, and we're very grateful for their talents and dedication. Most importantly, I want to thank OpenAI for providing credits for their API, which supported our development and the development of this curriculum materials.
Now it's time for me to introduce our first lightning talk. I would like to introduce Amber Galvano. Amber is a PhD student in linguistics at UC Berkeley, and this coming year, we're excited to welcome Amber to the Data Science Fellows Program at the D-Lab. All right. Thank you so much.
Hi, everyone. My name is Amber Galvano. Thanks so much for this opportunity to share about myself and my experiences a bit. To understand a bit better where I'm coming from, I grew up in suburban Michigan, where I would say I had a fairly privileged upbringing and educational experiences that took me to the University of Michigan. However, contending with a fraught relationship with my parents, my identity as a queer and non-binary person in higher education, and discovering the many elements of the hidden curriculum as a grad student who didn't directly major in my current field, have all shaped my research interests and priorities in ways that ultimately led me to apply for D-Lab's Data Science for Social Justice workshop.
Over the past three years in graduate school, I've developed a research agenda that asks broadly how the positioning, both ideological and geographical, of marginalized speech communities affects how speech patterns vary and change. My current main focus is in the area of speech and sexuality. And when I first started in linguistics, I learned about the erasure of much of the LGBTQ community, not just in pop culture, but in the sociolinguistics literature too. And I discovered that some of the original work in this area was based in stereotypes and perceptions of queer speakers rather than their actual produced speech.
So for example, the idea that gay men have a very sharp forward S sound, this is a common stereotype. And so I was inspired to design my own study, which seeks to understand how sexuality interacts with gender, race, and other aspects of social identity to influence speech sounds. So can we even say there's an identifiable gay speech when taking a wider range of factors and a wider range of people into account? And to try and answer this, I record interviews with people, and in a nutshell, I measure their vowels and their consonants. And on the slide here, you can see two other types of work that I do. I've worked on Spanish sociophonetics, including language attitudes, and I'm also involved in a couple of language documentation efforts. The project I mentioned, though, on speech and sexuality ties into my motivations for joining the DSSJ workshop, and shaped the project that I worked on during the workshop.
Of course, I wanted to sharpen my Python skills, so that data processing was more efficient, and I had new tools to work with. But I especially wanted to engage more deeply with the sociology and the ethics surrounding my work. I liked the idea of having a space to reflect on my own potential biases in the structuring and interpretation of data, and also to get ideas for a data activism component to the project, possibly including best practices, best practice tips for other researchers, or public-facing materials to help combat linguistic discrimination.
And so on this slide, you can see my struggle to put open-ended survey responses into a format compatible with my largely quantitative study, and then my curiosity about how to best approach open access when considering community and participant preferences. And we actually did some great readings on this latter topic in the workshop, and I ended up writing about it in my final blog post.
In the workshop itself, my group and I analyzed social media data, specifically looking at the subreddit r slash lgbt, to explore how redditors of various identities discuss their own language and speech online. And this was one way to learn more about how at least one subset of this community thinks about language, and what concepts identity labels are treated as important or related. And then this could then help contextualize other types of linguistic work like what I typically do.
As you can see on the slide, it turns out that they're curious about that supposed sharp s on reddit too, among other things. And I enjoyed the collaborative nature of this project, which involves both learning from mentors and students helping each other debug and brainstorm as well. Two types of analyses we used on the reddit data, which I found especially interesting and potentially useful for the future, were sentiment analysis and word embeddings. So for example, we looked at whether posts containing asexual compared to pansexual had a more negative or positive sentiment score. And it turned out pansexual may have the slightly lower overall sentiment. And then also what the most biased words were with respect to gender versus sexuality as target concepts in a word embedding space. And for example, we saw that gender identity words had a stronger collation with healthcare and policy terms.
So I would be curious in the future to determine if something like a sentiment score can be used as a predictor for phonetic variation in the same way that a person's identity label might be. And then perhaps to use similar methods to word embeddings to compare acoustic variables in a multi-dimensional space. One other interesting and amusing task that we tried was to see how chatGPT would describe LGBTQ speech and how it thinks an LGBTQ person would describe LGBTQ speech, which then might attune us to even broader trends in discussion online. And as you may know, LLMs are generally quite relevant to the present and future of linguistics and phonetics especially. And so I would personally like to get more involved in developing models for understudied languages or dialects in order to make the documentation process more efficient, which is much needed in many cases.
The discussions we had during DSSJ about community-centered data ethics will inform how I approach projects like this. Finally, to wrap up, my experience participating in this workshop provided me with first new practical data analysis skills, an understanding of how they work, but also how they can be applied conscientiously. It offered a space to reflect on how my own research could be done both more ethically and more creatively, potentially with this multimodal lens using text data. And finally, it provided an opportunity to connect with and learn from an interdisciplinary group of peers, which I think is very valuable as a PhD student. So that's it for me. Thank you very much.
Thank you so much, Amber. And the next lightning talk is from Davey Sibrian, a PhD student in the Department of Environmental Science Policy and Management. So looking forward to hearing from Davey.
Hi, everyone. Thanks for the opportunity to share my journey and experiences with Data Science for Social Justice Workshop. I sincerely thank Claudia and the D-Lab team for making this possible. So yeah, I'm Davey Sibrian. I'm a PhD student in the Department of Environmental Science Policy and Management. My lived experiences and intersecting identities profoundly influenced my research. These personal histories and cultural connections drive my current academic focus, particularly in examining the social environmental health impacts of digital transformations like cryptocurrencies and AI.
Before diving into my research, I want to start by acknowledging my ancestors for their sacrifices and express my deep gratitude to my mentors, many of whom are women of color. Their support has been crucial to my survival and success. Growing up as a non-binary first-generation immigrant from El Salvador, I have first-hand experience with intersecting social inequality.
including enduring legacies of colonialism.
My parents, constrained by systemic barriers, only received a few years of elementary education.
I identify as indigenous.
I grew up hearing stories of how my family was displaced from our ancestral lands in Nueva Trinidad during the Civil War.
My grandparents became street vendors to save money for a home.
I was born in their adobe house in Nueva Concepción during the end of the war.
Later, due to social environmental factors, my family was displaced again, and this time it was in Los Angeles, California.
In LA, I assisted my parents with various day laborer duties.
A year and a half or so later, seeking work stability in the meatpacking industry, my parents transplanted the family to Nebraska.
There, I earned multiple undergraduate degrees and a master's in sociology, focusing in environmental health.
My applied thesis project centered on leading an urban farming and food sovereignty public health initiative, emphasizing restorative ecology.
Thanks to my aunt's foresight in petitioning our family, when we arrived to California, I became a U.S. resident after earning my master's degree.
And a couple of years ago, I became a U.S. citizen.
My residency allowed me to pursue work with the government in Washington State, where I began as a farm labor investigator and was soon appointed research project manager for an interdisciplinary occupational health and safety study requested by the legislature.
I wanted to see what kind of impact I could make from within the system using my acquired skills.
I spent a lot of time and energy advocating for various issues.
However, disillusioned by the toxic white supremacist environment, I chose to leave to pursue something more fulfilling.
My experiences with the government solidify what I contemplated before moving to Washington State.
I applied for several PhD programs along the Pacific West Coast and was accepted into most of those programs.
Although I didn't initially know much about Berkeley, a professor from another school encouraged me to apply.
And now I'm a proud member of the PhD program at SBOM.
My PhD research focuses on a concept I coined, cryptonocene, which explores the social environmental health impacts of cryptocurrencies and related technologies like AI and big data mining, particularly in the context of energy transitions and displacement.
El Salvador offers an ideal case study to examine these issues, juxtaposing two global milestones, being the first nation to ban metal mining in 2017 for environmental preservation and becoming the first to embrace Bitcoin as legal tender in 2021 in pursuit of economic development.
My dissertation critically examines projects like the proposed city, Bitcoin City evaluating their potential to foster equitable energy transitions while assessing their ecological and community health impacts.
I analyzed these developments, not only in El Salvador, but also on a global scale, including regions like the Columbia River and the Pacific Northwest.
Recently, I returned from the Columbia River from conducting an informative field site visit, and that deeply enhanced my understanding of these issues.
My recent field visits to El Salvador, the first time since my family's displacement, were pivotal in refining my research.
The proposed Bitcoin City at the base of the Conchao volcano, where the government plans to clear forests for geothermal plants, raises significant concerns.
Conversations with local community members reveal deep fears of land dispossession, a reoccurring issue, and energy transition and resource extraction projects.
My research aims to broaden our understanding of how these developments impact ecosystems and historically marginalized communities, and to advocate for necessary safeguards to prevent further exploitation.
I pursued the Data Science for Social Justice workshop at UC Berkeley to acquire new tools for my research.
The workshop has been a transformative experience.
It significantly enhanced my skills in Python, generative AI, and large language models, and data scraping techniques.
My team applied these newly acquired skills to study the environment subreddit, where we conducted a detailed exploration of global versus local perspectives within quotes and comments.
After discussions and preliminary analysis, we focused on a theme, and we used TIDF scores to determine relevance to local and global context, performed word similarity and bias analysis, and conducted sentiment analysis.
We also explore effective visualization techniques to present our findings.
I plan to use these skills to analyze various social media platforms, and to study the impacts of digital transformations, like cryptocurrency and AI in marginalized communities.
My work is deeply rooted in a legacy of social justice passed down by my grandfather and others.
I delve into these issues and my current research in a forthcoming paper under review.
My research guided by this legacy seeks to establish ethical guidelines for technological use, research management, and energy transition, with the goal of preventing exploitation of marginalized communities, and fostering equitable global sustainable development.
I am eager to collaborate with researchers, policymakers, and activists to build a more just, inclusive technological landscape.
Together, we can better understand the complex interplay between technology, society, and the environment, ensuring that progress benefits all communities, not just a privileged few.
Thank you for listening. I look forward to connecting.
Thank you so much, Davey. Oh my goodness, he always just is very moving. And your dedication to your work and to your communities is just really admirable.
Thank you so much for sharing.
We're so excited to welcome Sol Cheh-Fuzi next, a PhD candidate in the Department of Environmental Engineering.
Hello, everyone. It's a pleasure to be here. And as Claudia said, I am a PhD candidate in the Department of Environmental Engineering here at Berkeley.
I am originally from English or Anglophone Cameroon, and I make that distinction because there is an ongoing crisis between the English and the French regions of the country.
And this combined with many other aspects of domination historically has stagnated many parts of Cameroon and created political and environmental ecosystems, which have forced a lot of families like my own to migrate away.
My family's experience, my upbringing, and my migration to the States naturally motivates a lot of my work.
A lot of that motivation can be sort of summarized through these sorts of graphics where Sub-Saharan Africa in particular is always shaded in the brightest hues that speak to the region falling short of lacking access to or being inadequate by some supposedly well-defined metric.
I find that many of these metrics are inappropriately contextualized, understandably so due to the lack of diversity in both the people who are defining these metrics as well as the schools of thought that these metrics stem from.
I've had the honor of being exposed to various schools of thought through studying and conducting research at a number of institutions in the Americas, Europe, and Africa, as well as while I was navigating my immigration status, working at an African hair braiding shop, and as a nursing assistant and medication technician with and for some very diverse people.
Through all of these experiences, I came to understand that it was critical for me to circle back to Sub-Saharan Africa as an international development engineer and educator.
It's what I feel my calling is.
And this is partly how I gravitated towards the D-Lab because I realized that focusing more on data science would offer me the geographic flexibility that I think is often overlooked, but is important for someone like me whose immediate family has not lived on the same continent, not to speak of the same country, in decades.
Data science allows me to be based in.
Nairobi, Kenya, where I am at the moment, to complete the segment of my research and to truly establish myself as an international development engineer.
And as someone who's been in college since 2012, I like to joke and tell younger students that I'm technically in the 24th grade. I didn't exactly have the capacity for a full semester data science courses. And that's initially what drew me to the D-Lab.
And I've been here for a while. I've taken their regularly scheduled workshops, their specialty workshops like DSSJ. I've taught as a data science fellow. And I've worked on the curriculum as a senior data science fellow.
And in all my 12 years of higher education, the one thing that was so particularly unique about DSSJ is that they are teaching what is stereotypically considered a very technical set of skills, which arguably can be found through a lot of avenues. But from this critical foundation of justice. And why that was so critical for me as a development engineer is because my work focuses on materials and technologies that are accessible to the most marginalized.
Summarily, I work with biochar, which is similar to charcoal and is defined as burnt organic matter, including agricultural waste and even human feces. So the first chapter of my dissertation focused on using biochar to recover nutrients from human urine.
I love the reactions I'm seeing. It sounds intense, but I have this vision in my mind where we create biochar from human feces and then use it to recover nitrogen from human urine and then apply it to soils. Technically, this is already in this is an ancient practice, so nothing new.
One takeaway from the years spent on this first chapter was the understanding that the technology has a lot of promise in agricultural hubs, like many regions throughout sub-Saharan Africa, because they produce so much feedstocks by way of agricultural waste, like corn cobs or coconut shells and so on. But this technology also requires a separate collection of urine and feces.
And in many places like North America, we have sewerage systems, and we flush our waste with drinking quality water. So there's a unique set of challenges to deal with there, whereas in substantial regions throughout sub-Saharan Africa, they are not connected to sewers.
But technologies like source-separating toilets, like the one we see in this image where this woman is sitting, where urine and feces are collected and thus collected and can be treated separately. And this is part of what we consider the circular bionutrient economy.
So this technology has a lot of promise in regions like sub-Saharan Africa. But most of the research methods and standards on biochar come from and are developed for a specific context in America, China, and Australia.
So in order to realize the potential for biochar in sub-Saharan Africa, my second project focuses on developing a library and models to be able to predict various biochar properties. Generally, quantifying biochar's various characteristics is expensive and inaccessible. And a lot of people end up sending their samples to Germany or the US to be characterized.
But in my second chapter, we use infrared spectroscopy, which is arguably a more accessible technology. And this project I took on solely because of my training through the D-Lab, because I did not have the data science knowledge prior.
Successfully being able to predict a suit of biochar characteristics is then the basis for my third and final dissertation chapter, which is still in development, but focuses on developing regionally appropriate standards and techniques for characterizing biochar across sub-Saharan Africa.
Much of this work thus far has been two years of effort towards bringing together researchers in the region, excuse me, who work on related biochar topics to build a network. We've named this network CBEN, or the Circular Bionutrient Economy Network.
Over the last year, I've conducted interviews of CBEN stakeholders. And this past year, I co-hosted a session on standardization at our CBEN conference. And one of the main goals of this session was to get stakeholders to document thoughts, plans, sentiments that pertain to standardized biochar testing in the region.
So these four images on the right show some of the data that we gathered. And my next step will be to analyze these texts using tools we learned in DSSJ, such as sentiment analysis or topic modeling, which had been talked about a bit prior.
So for instance, one of the relevant sort of topic areas that came from us just sitting down and analyzing some of this documentation that I show here from this session was that a lot of the international biochar standards and organizations were developed with Africa marginally in mind.
And an outcome from this very brief kind of analysis is that next year's conference is being hosted with the International Biochar Initiative, who prior to the formation of this network has had marginal ties with the biochar work being done on the continent.
So this project is still in its exploratory data analysis phase. And the outcomes will help me formulate more explicit research objectives and structure more formal interviews and methods of gathering text data at the upcoming conference we'll be hosting.
Gathering what will be primarily text data will be easier to accomplish because we've spent the past couple of years developing this network. And as importantly, I find that people use text. They use text as data, but they don't regard text as data.
So there have already been many data-driven decisions, such as deciding to host a conference with the International Biochar Initiative, that have been made from the conversations and conference notes and the natural language processing approaches from a social justice lens allows me to formalize this data in a way that will allow these decisions to be made a lot more efficiently.
In the second chapter, we were working on developing models to characterize biochar. But there are still many steps between having a method that adequately characterizes biochar that is regionally accessible and that translating to actually improving the regional analytical capacity of biochar. And that's the gap that this third chapter aims to address.
This field of international development and working in resource-extracted regions in particular is an atmosphere particularly where best intentions can and often enough do lead to detrimental outcomes. But if my foundation and my approach is justice-based, I feel like I can minimize the room for negative impacts.
And as I'm transitioning from the student role into contributing to the 0.7% of researchers based in Africa, according to the UN, I realize that I'm taking on this massive responsibility. And DSSJ has given me some of the tools, both in terms of teaching me how to be a data scientist from a technical standpoint, but also from an ethical one as well.
So what's as important as having gone through the DSSJ workshop and learned from their approach is that as an educator, as an engineer, as a researcher, I can cite the last few years of DSSJ when I'm developing and discussing my own pedagogies, my methodologies, and my epistemologies.
I have DSSJ to cite as a working framework. It's this idea that you can approach a research or teaching endeavor that is already so loaded with its technicalities and nuances, but start with and spend enough time on the ethics and morality of being done, and this way work towards more just outcomes.
And this is sort of the takeaway from DSSJ that is continuously present in my career since the first offering of the workshops. And I'm looking forward to these next steps in my career. Thank you so much. Thank you so much, Sol. Amazing. I can't wait to see where this all leads, and I'm sure you'll keep us informed along the way. As you all can see.
that the D-Lab at UC Berkeley is a hub for data-intensive social science research and education. It offers resources, training, and support for students, faculty, and researchers to enhance their data science skills, focusing on collaborative interdisciplinary projects.
D-Lab provides workshops and consulting and support, and it does this through mentorship and the development of fellows such as the ones that you have met right now. And we also help people access advanced computational tools and data.
We run the regional FSRDC, the census data, for example, helping bridge the gap between data science and social sciences. And yeah, we really center inclusivity and equity in data science education. So looking forward to some discussion.
I'll hand it back over to Natalie, and thank you so much again for this opportunity.
Thank you, Claudia. And I think all of our presenters are going to join us in the frame now because we have some audience questions for you. Thank you so much for presenting.
Okay, the first question is for Sol. And Sol, this is from one of our AI research scientists at OpenAI, Taina Alundu. She was previously on the policy research team, and now I believe she's working in the safety systems team. Her question is, Sol LeVert, as you mentioned, many of these processes are inherently used in Africa. Do you think AI models can enhance traditional practice, e.g. information collection, aggregation, or diffusion? In other words, is there room for these models to enhance local practice rather than displace it?
Yeah, I think this is sort of what I was getting at when I was saying that people use text as data, but they don't regard it as data. And I think some of the struggles that we have and what I've seen since being here is that there's not enough formalized documentation. So, there's text out there. So, for instance, there's conference proceedings and so on, and even just publications, generally either peer-reviewed or not peer-reviewed. There's a lot of information out there, but I think that the systems are very disconnected, and I think large language models have a way of, you know, if you do some text scraping, of gathering all that information and presenting it in a quantitative method for people to use.
So, I do think there's a lot of potential, and that's the general struggle is there are data out there, but converting that data into a digestible format for the general population to be able to use, I think what is kind of lacking. I hope that gets at the answer.
Thank you so much, Sol.
This question is from me, and it can be answered by any of the students. How did participating in the Data Science for Social Justice workshop transform your approach to research, and what were some of the most valuable skills you acquired? Sol, you mentioned that part of this work you wouldn't have been able to execute or implement had you not participated in the workshop, so I'd love to hear a little bit from all of you what specific tools you gained and how it impacted the work that you've presented.
Amber, would you like to go first?
Sure. I would say the two biggest takeaways that have applied to research are concerning efficiency and then framing of the research. So, I learned some new data wrangling tricks and how to efficiently load in and visualize data and dynamically look at it that I'd like to use in the future.
And I learned about this template for data transparency. It's called Datasheet for Datasets, and I really like that because you sort of have to walk through and explain why your data was created, how it was collected, who it's for, who's going to have access to it. So, I'm trying to incorporate that into my dissertation, which is not necessarily a technical thing, but I think it's really important.
Would you like to popcorn to one of your peers, Amber?
Sure. Let's go with Debi.
It was just an amazing experience. It has been extremely helpful in informing a project that I'll be taking on this upcoming semester. I'll be working with some development engineering master's students to create a live data scraping tool for data transparency. I'll be using that live data scraping tool for different media platforms to create a base layer type of dataset to map out where different kinds of digital transformations are happening, and then I'll be using that with satellite data and remote sensing to do an overlay. So, it's been extremely helpful, and I really appreciate the community and just being in that space. It's been an amazing experience.
Sol, is there anything you want to add before we move on to the next question?
I will just pop and say, because, Debi, you mentioned development engineering, and I have a designated emphasis in development engineering, and the one thing that I find is, I think engineers, we have this sort of complex where we don't regard non-technical knowledge bases as valid or valuable, and I think that's what DSSJ offered, is that there is this way to combine the technical with the socio-technical, with the ethics, with the morality, and it's necessary because until we do that, we'll continue to recreate a lot of these problems.
So, I think my biggest takeaway is that DSSJ is this example of a working model of a combination between what is stereotypically considered technical and what is rendered non-technical and considered maybe social or whatever, but are both necessary in order to actually move things forward. So, I think that's probably my biggest takeaway.
I love that, Sol, and maybe you and I can collaborate in the future on some community initiatives because bridging that divide is like 70% of my job as well. So, I'd love to hear your insights.
Anne Murphy, Anne is a very tenured member of the community and a leader in the non-profit sector. Anne, do you want to ask your question personally? If you do, just unmute yourself and jump in.
Is it on? Yep.
Okay. There was so much there, by the way, as a proud non-technical person. That was dope and my brain is exploding. So, and congratulations and kudos and thank you for the work that you're doing.
I remember some news stories about when OpenAI and Reddit formed a partnership, or maybe it was that at some point Reddit data made its way into ChatGPT without permission. I'm not sure. I wanted to just learn a little bit about how you got access to the subreddit conversations for your data. Did you work through ChatGPT or did you go in there and grab it?
And I had actually a second question that I started to type it out and I couldn't quite find the words, but let me try to get it out of my mouth, which is when I heard about the Reddit stuff, one of the thoughts that I had was I immediately thought of all of the things that I've posted on Reddit and various subreddits that at the time felt, regardless of the fact that everything on the internet is for everybody, felt kind of intimate. It felt like I was in a safe space. And then I realized this is a perfect example of, well, Anne, actually as a reminder, that's not true. And I thought, gosh, I wonder of all the random things that I've posted on Reddit, what's going to make its way into ChatGPT. So I was wondering if one of you could comment on that topic, ChatGPT plus Reddit and your data science.
Prateek, I was hoping that you can, Prateek and Tom, assemble the data, and it's a really good question, and it ties in with the work that they've been doing also, so he'll chime in.
Hi, can you hear me? So yeah, there's been a, so Reddit provides an API to access its data. You may have heard about a lot of the issues with APIs and how the whole infrastructure about how that is changing. It's very hard for researchers to get access to the API to get Reddit data now, but there are systems still in place to get access to the data. So we had access to a bunch of data that we had before the API kind of shut down. So we were able to still use that this year. Now Reddit works with moderators to make their data available, and there are ways you can get access to that through certain torrents if you want to access Reddit data that way.
So there are still ways to access Reddit data, it's just a little bit harder now. But it almost surely is used in many large language models. It's actually very, it's some of the best data for large language models to use because you want it, the best data for large language models to use is kind of high surprise data. It's a lot of creative ideas, it's got a lot of colloquial language, and so it's very good for quality for large language models.
And so some of the things we talked about are in the course or how then these kinds of models may be then used to then produce data or text that goes on to Reddit. Like that was one of our discussion points. And so you end up in like cyclical scenarios where the data is coming in, but also data is coming out into the models and going in. I hope I answered that, your question.
Thank you, Prateek. Thank you, Anne. Ben Kinsella is a member of the OpenAI Forum community team. Would you like to unmute yourself and ask your question? I can also ask it for him. Ben is curious about the role of AI in your research. How specifically were LLMs leveraged to accelerate enhanced traditional research methods used? And maybe we'll start, Davey, did you use LLMs?
Yeah, that's something that will be used for the data scraping tool I mentioned. And yeah, it's something that I'll be using. I haven't really used it that much. I played with it a little bit, but I don't have a lot of experience with it. Any of the students want to jump in? And if not, I know Prateek can definitely give some insights into the projects that he's been working on in the past year.
Prateek, would you like to specifically describe how you've been leveraging LLM in your work at UCB?
Sure. I can speak to it in this workshop. So one important aspect of this workshop is we combine, it's a quant, it has heavy emphasis on quantitative training, but also qualitative training. We want to encourage our students to leverage both qualitative and quantitative aspects so that they're more interdisciplinary researchers.
And so one of the ways we have our students engage with LLMs in this course is by having them try and prompt different chatbots with prompts from Reddit to see what kind of outputs they can elicit and whether they can distill norms or beliefs encoded into these kinds of large language models.
So it's kind of, we encourage them to take on like a more qualitative interrogation of large language models. And so that's been one of our related research projects that one of my colleagues in the audience, Tom Van Noonen, and I have been working on is trying to distill some of those norms or beliefs that you can extract from large language models that are echoed by large language models, depending on how you prompt it.
And so the subreddit Am I the Asshole, which is in some sense, like the basis for a lot of the work that we do in DSSJ, it's a subreddit where people pose moral dilemmas, other Redditors respond offering their opinions of the moral dilemmas. So it's very much a discourse community in which their beliefs and their norms are apparent and ready to be analyzed.
We have taken a lot of those dilemmas and asked different types of large language models to directly respond to them and say who is wrong, who's the asshole in this scenario or not, and then try and use that as a way to better interrogate norms that you can extract from large language models. So that's been a very closely related project with Data Science for Social Justice. Thank you so much for sharing Prateek, and hopefully we'll hear from Prateek and Tom later before the year is up and learn a little bit more about that research.
So I think that that's pretty much, let me look through these questions and see some of them we've addressed in other way. This has been addressed, but Fozia, would you like to unmute yourself and ask your question specifically to Claudia and Prateek? Because I think there are multiple different answers.
Yeah, thank you. Thank you so much for the presentation. And I know Claudia, I think you alluded to some of this at the top of your presentation, but I think as Sol was saying earlier, what distinguishes this data science program from any other program is specifically the whole social justice aspect and the foundations of it.
So I'm sure and I'm certain there's very foundational principles that you're looking to, that you sort of like instilled and built into the program. And I'm just curious to know, you know, like, can you maybe share an example of what some of those principles are and what it would have looked like in implementation, if that makes sense?
It's a bit of a vague question, but hopefully, I know, like you mentioned some things around biases and stuff at the top of the presentation.
So I was just curious if there's anything you could share around that. Absolutely.
Yeah, so I think, and also, I'm happy for Prateek to jump in, but I would say that we're at the intersection of infusing social scientific thinking into computation, so computational social sciences, which is different than traditional data science in the sense that we're not just interested in analytics, but we're actually interested in the specific phenomena. And then we want to start with a question, and then address that question in the best methodological way possible.
That means that sometimes the tools haven't really caught up with the constructs or the thinking or the theory. And so we have to kind of bring the tools along. And sometimes, it means that there's not enough data to bear. So we need to really rely on qualitative methods.
So I would say that there's a social scientific approach, which I think is very important in studying human phenomena. And then there is how we approach research, which is very much from a participatory action research type of stance, or cultural humility type of stance, which is going to ask questions about data, first and foremost.
Where did the data come from? What did consent look like? Did it do any harm to the people that we collected data from? Did we have ongoing consent from that population? And then thinking about issues of data return, like if we collected data from a group of people, are we giving something back? How are we enriching those communities?
At the end of the day, how are we promoting justice? How are we ensuring that we're not perpetuating racism and sexism? So those are kind of more concrete frameworks. And we do share a bunch of our publications that can give you much more specificity in terms of examples as well.
Fawzia, thank you for the beautiful question. Claudia, love the answer as well. It was very insightful. Thank you.
Yeah, it was awesome to hear from the students.
been commuting with Claudia and Tom for a while and it was really great to see some of the final outcomes, the outputs from the UC Berkeley's Data Science for Social Justice workshop.
And I hope you guys continue to engage with us here because we have a lot to learn from you. And Claudia, I hope to see you in some of the upcoming educational tutorials because I think that you'll also have a lot to bring to the table there and we can all learn from each other.
But it's the end of our event for the evening. Thank you to all of our presenters. It's an absolute honor to host you. Thank you to the community members who showed up. Thank you to my OpenAI colleagues who showed up.
We would love for the folks who showed up tonight, and this includes Sol and Prateek and Debi and Amber, for you to refer your peers to the forum. I think that the rest of the year's programming has been curated specifically for academics in mind in a lot of ways, especially academics who aren't hyper-technical. So folks working in social sciences but haven't had a lot of experience with large language models, folks working in the humanities and liberal arts as well, I think we'll be able to learn a lot together this year.
And then we'll also be hearing a lot from OpenAI research scientists. So you can ask some of the OpenAI experts as well about how they went about their research. So if you're interested, we've dropped the OpenAI member referral form in the chat a few times, and we privilege your referrals above and beyond any of the other applications because we trust your judgment and we want to keep this community feeling intimate and that the engagements are really inspiring and high quality just like this evening, which is why, thank you for those of you, I responded, I messaged you and asked you to complete your profile and add a profile picture. That's because this is not an anonymous community. We aim for it to feel really personal and we'd like to get to know each other here.
Everyone, thank you so much for showing up tonight. In the future, my colleague Ben, who is an OpenAI forum community manager and working with the research team, he's going to help me host some of these events so that I can show up for my son. He just started high school and he played his first football game tonight. And I'm going to tap some of my colleagues to help me host events so that I can be more present for that stuff. So if you see my colleagues hosting, know that I haven't gone anywhere. They're just supporting me so that I can also be present for my family.
Please show up to office hours. Let me know what's on your mind, what you'd like to see in the forum. I have taken all of my curatorial work from your queues. So thank you so much for being here, guys. I love to be in community with you. It's very meaningful to me and I can't wait to see you again very soon.
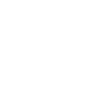