OpenAI's AI Trainer Community Mixer with Special Appearances from Research Leadership
SUMMARY
Hear from research leadership first hand about the significance of expert trainer contributions to the OpenAI mission.
TRANSCRIPT
I'm Natalie Cone, and I lead the OpenAI Forum Community Program. I like to begin our talks by reminding us of OpenAI's mission, which is to ensure that artificial general intelligence, AGI, by which we mean highly autonomous systems that outperform humans at most economically valuable work, benefits all of humanity.
Tonight we're here to learn about the expert AI training program at OpenAI. The program is a way for members of our community to contribute to OpenAI research in the form of data annotation and model evaluation. An instance of expert model evaluations that this community has contributed to is building an early warning system for LLM-aided biological threat creation, which our team will drop in the chat for you to read later.
Some projects even require experts from the community to make net new questions related to their field that OpenAI will train or evaluate a model with. By net new, I mean questions that we can't find anywhere else and that we expect GPT-4 will not be able to answer. We've built an expert AI trainer program that we hope demonstrates our appreciation for your contributions.
Compensation for the work is more than competitive in the industry, and all trainers who complete at least one successful project milestone get invited here to join the OpenAI Forum. It's also possible that one of our teammates or a Forum member can refer you to join the Forum before you even complete any work with us.
This community is a huge resource which will connect you to each other, to PhDs and expert practitioners from a broad range of domains and disciplines. You also get invited to meet and speak with OpenAI researchers and product staff during virtual and in-person talks, and you get a behind-the-curtains look at how OpenAI executes some of its research. It's truly an awesome opportunity, one that I'm really proud to be a part of.
One last thing before we move on to our guest speakers for the evening. If you've signed up to participate in training, but you haven't been added to a project yet, it's just timing. We want you to know the project just doesn't exist yet. When one services that requires your background and expertise, you will hear from us. You're in the database, we promise. Until then, please enjoy opportunities such as these to get to know OpenAI and the Forum members.
Our agenda tonight will start with talks from Mia Glaze, Head of Human Data, Lillian Wang, Head of Safety Systems, and Evan Mays, a member of the technical staff on the preparedness team. We recorded this talk live two weeks ago when we hosted this same event in person at OpenAI's SF headquarters. We thought that you all deserve to hear why they think your contributions are so very valuable to the future of safe AGI and OpenAI's mission.
After the talks, I'll introduce Spencer Pepe, a technical program manager on Mia's team, and three of our OpenAI Forum expert AI trainers who have worked on several projects over the course of a year.
You'll even have an opportunity to ask them some questions via the Q&A tab on the top right of your screen. Next, we'll move on to our networking event where we'll all be matched with other members present tonight.
Finally, for those left standing at the end of the event, we'll also raffle off some really cool OpenAI Forum totes and a super nice blue ripple water bottle created from recycled ocean waste. You have to be present to win one of the raffle prizes and we'll send them to you in the mail. So please DM Caitlin Maltiey here in the forum with your mailing address if you win.
Okay. Well, welcome, everyone. We're so happy to have you here tonight. Let's move on to hear from some of our leadership and technical staff about the importance of expert AI training community here in the OpenAI Forum.
Friends, I am really, truly honored to introduce Mia Glaese. Mia is the head of human data at OpenAI. The human data team creates custom data solutions, driving groundbreaking research. Their work enhances and evaluates our flagship models and products like ChatGPT, GPT-4, and Sora, and contributes to safety initiatives through collaboration with our preparedness and safety system teams.
Mia is a researcher focused on advancing AI capabilities in a way that inherently aligns with human values and ethical standards. She works on the joint optimization of human data and training algorithms, integrating human judgment to refine AI behaviors. By developing methods that incorporate human feedback into AI training process, she's contributed to making AI systems more effective in real-world applications.
Previously, Mia worked at Google DeepMind on pre-training, evaluations, factuality, and reinforcement learning human feedback for large models. She's authored numerous publications on the ethical and technical challenges of AI, particularly in aligning AI with human values, mitigating harmful outputs in language models, and developing robust multimodal models. Her work also emphasizes understanding and addressing the social and ethical risks posed by AI technologies, contributing to creating safer and more responsible AI systems.
Please help me in welcoming Mia. Welcome. I'm really excited to meet. This is the first time we are hosting an in-person event for expert trainers that work with us. So, I'm really excited for all of you to meet each other and for us to meet you. We're really grateful for all your contributions to our models, especially now that our models are getting better and better and more intelligent. We need to make sure that we have true experts who can supervise them and evaluate them. And so, your contribution is really critical to opening AI's mission.
I hope that you enjoy the evening today. And I don't know, do I introduce the next person? Yes? Thank you so much. And Mia's going to stick around for dessert. So, if you have any questions for her, you can ask them in the wake of these short chats.
Next up is Lilian Weng, Head of Safety Systems. Lilian is the Head of Safety Systems at OpenAI, where she leads a group of engineers and researchers who work the end-to-end safety stack for deployment of our frontier models, ranging from alignment training of model behavior with safety policies to inference time monitoring and mitigations.
Previously, Lilian built and led applied research at OpenAI to leverage powerful language models to address real-world applications. In the early days of her OpenAI time, Lillian contributed to OpenAI's robotics team, tackling complex robotic manipulation tasks, such as solving a Rubik's Cube using a single robot hand.
With a wide range of research interests, she shares her insights on diverse topics and deep learning through her blog that is very popular in the machine learning community, which, by the way, guys, we've linked on the event page in the forum, if you'd like to check it out if you're not already a subscriber.
Please help me in welcoming Lilian. Thank you all. I'm very excited to be invited to give a little talk here, and I'm so happy we have a group of people who are really passionate about actually guiding what's the future of the AI models. I would say I see human data and expert feedback as a super-critical thing.
As the model becomes more capable, we really need to have focused collection process to find the weak spot of a model that the general average way of cross-sourcing cannot help, and we need experts' inputs on things like lots of things we internally cannot judge, and we need you guys to tell us why it's not good and how we can make the model better.
So think about when the model becomes super-intelligent, maybe none of us can help the model, but we still have some way to get there. As we're getting there, more and more expertise will be needed. So this is why I feel very excited about human data.
To take a step back about myself and my team, our team, as Natalie just shared, my team owns an end-to-end safety stack. We do consider all kinds of approach that can help make the model safer, make the system safer, how we can improve the iterative deployment of the model and learn things from the production data, try to do better risk assessment based on what we've learned from the practical challenges so that we can feed back into our understanding of the practical problem and reiterate our methods, mitigation, and then push that into the next generation of the model.
So this is a very nice loop as I see why we're doing this deployment and the iterative deployment to tackle the safety issues rather than just talking about it because it will keep out our problem, our selection of the problem and selection of method more grounded.
And to take one step further, to make the connection with all you guys here, I see safety is a very special domain that we need a lot of human, like we need to make sure we align with the human values. Like for example, a lot of topics are kind of soft and a lot of like, you can see a lot of subjectivity in different topic, but in order to figure out what is the general principle, what's the right position to be at, what is the general public opinion about certain topic, how we can range from a more polarized opinion to find a balance of spots to design a model behavior, we need a lot of human data and human feedback in this process.
So we recently, like with post-training team together, like we recently launched this model spec documents and just describing the ideal model behavior, the general rules and defaults. I think that's just a starting point as we're getting deeper, adding more granularity, getting to more sensitive topic, like we need to talk to human to understand the preferences.
And another aspect I see the value of human data is in really expert domains, like for example, think about people ask for medical question. I do that all the times. I have a young baby, I run into a lot of situation I don't know, I'll just ask the model, like, oh, my baby had a fever, what should I do? It's hard to get doctor's contacts, like, 24 hours. So we actually did notice a lot of people ask this type of question. Then how can we be confident the model can be safely used in those domains? Then we need help from our doctors. And other issues, like people ask the model about mental health, I mean, that's a very controversial domain, has a lot of different opinions about what's the best way to interact with the user. But the fact that some people will reach out to the most advanced AI model, ask this type of question means that we need to take this topic very seriously. I mean, it's a hard domain, but we need to find a way to deal with that, meaning that we need to, like, we talk to, like, therapists and ask about their opinions, ask professionals' opinion, but this is not like we can do that correctly, 100% correctly on day one, and we need everybody's involvement and help and collaboration together to make those behaviors better, behavior as a model better. So that's roughly what I want to say, and if you have, like, safety-specific questions, like, I'm happy to chat.
Yeah. Thanks. Thank you so much, Lilian. Last but not least tonight, we have Evan Mays. Evan is the infratech lead for preparedness at OpenAI. The preparedness team is responsible for evaluating and forecasting the risky capabilities of our most capable frontier models. Evan holds a degree in computer science from Johns Hopkins University. He previously founded an AI company researching web agents. Please help me welcome Evan.
I'll hold it. Nice to meet everyone here. Thank you all for coming. Yeah.
So I think a lot of people here actually have, like, helped out preparedness-specific evals, which I'll talk about in a second, but I think we'll start with, like, what is preparedness?
So preparedness, we're another safety team at OpenAI, and we care about catastrophic risks of people using the models, specifically, like, misuse of the models. So there's four categories that we care about, and these are, like, CBRN, which is, like, includes chemical, biological risks, nuclear risks, et cetera, cyber security, model autonomy, which is, like, models doing AI R&D on their own and just kind of, like, living out in the wild, and then what's the last one? Persuasion. This is also a big one, and, you know, specifically, we care about these risks on, like, a catastrophic scale. We work with, like, governments a lot. They're very interested in, like, the research we're doing, et cetera, and our team is, like, we do two kind of we have two big work streams.
One of them is evals, where we're trying to evaluate, like, how good are the models at these risks in these different categories today and really formally understanding that, and we make these things called scorecards, where we rank the models, like, risk level on a scale of, like, low, medium, high, critical for every risk category, and then we, like, present this to the company, and it's really important that, like, we understand exactly what the models can do and what they can't do, and human data is a big part of that, which I'll get into in a second.
The other big work stream we have is forecasting. This is more about how do you know when the model will be good at doing these catastrophic, like, bad risks? For example, like, is the model capable of hacking into, like, an iPhone or something? Like, this would be something people want to know. They'd want to know when are models capable of this? How is OpenAI planning to, like, mitigate these risks? How are we planning to, like, deploy models that might have these capabilities? We probably should not deploy models that have those capabilities.
And so those are the big work streams. We work a lot with, like, other teams at OpenAI, and I think I'll talk about, like, two big evals we have.
One of them is a bio eval, which Natalie tells me a lot of people in this room were involved in, where we bring a bunch of biology postdocs, people currently in Ph.D. programs, undergrads, we bring them into a room, we split them up into a control group and experimental group, and we say, all right, here's a problem you should all research, and this problem is something biology related, something related to catastrophic risk, and the control group gets the Internet, so they can do research on the Internet and try to research to figure out this problem. The experimental group gets the Internet plus chat GPT, and we're trying to see, like, what's the uplift there? Can the folks that have access to the Internet and chat GPT do better than the folks with just the Internet?
So a lot of people in this room were helpful with that, and very much appreciative. This was, like, very difficult to get this eval running, where it's, like, you have, like, experts who are very busy with their schedules in a bunch of different regions around the world, et cetera, and very appreciative for that.
Another big eval we have is capture the flags. So if you're familiar with cybersecurity, which you might not be, I'll explain it, essentially capture the flag is a cybersecurity challenge where you're on your computer and you're trying to kind of, like, get access to another system that you don't have permission to. I mean, this is, like, all sandboxed and, like, it's, like, for fun challenge kind of thing. You're trying to get access to the system, and you're trying to, like, find some piece of information on that system, which would be the flag. So if you make it into that system, you get the flag, you come back and you say, all right, here's the flag, the judge can say, wow, that's the correct flag, like, great job.
So we work on, like, basically setting up sandbox environments where we give models access to their own computers, and we also, like, in that environment, there's, like, another computer, like, a web server, maybe, and we tell the model, hey, model, try to the flag in that server. And the model goes and makes that attempt. And if it gets the flag, we grade it. This is how we understand how good is the model at cybersecurity type of things. Or it's at least one of the ways. And human data has been very important for that because getting all of these environments set up such that we have hundreds or thousands of tasks, different CTF challenges, this is very difficult to do on our own. And we would not be able to do it without expert software professionals and cybersecurity professionals from the OpenAI forum community. So I think these are the things I want to talk about. I'll be around for dessert after. Thank you. Thank you, Evan.
OK, that's it for the talks, guys. The reason we brought Mia and Lilian and Evan to the stage wasn't to give you a big lecture. It's because we know that you're all highly educated professionals and that you don't do this work only for the compensation. You do this work because it's intellectually rigorous, because you're curious, and because you're interested in contributing to the future of AI as it intersects with your discipline. So we're hoping that we were able to share a little bit of insights so that you understand a little bit better what you've been contributing to. And that makes the work a bit more meaningful. And we also just wanted you to hear from leadership and from the people that are running the projects how much we actually value your contributions. We're so grateful that you're here. We're going to have dessert now. You have an hour to mingle. And thank you so much for being here in community tonight. So I hope you all enjoyed those talks. And I also truly hope that you're able to make it for one of our in-person events in the near future.
Next up, we're going to be talking live with one of the members of my team and three of our expert AI trainers. This is the time where you're going to want to be in the audience. So if you're in the audience, you're going to want to be able to talk to one of our AI trainers. And we're going to be talking live with one of the members of my team and three of our expert AI trainers. So I want to start contributing to the chat, the Q&A chat. And we're going to call on you at the end of these short talks and introductions and actually ask your questions to the speakers tonight. So this is the live portion of the event.
First up is Spencer Papay. Spencer is a technical program manager on the human data team, principally responsible for the quality of the data that we create for our research stakeholders. He's designed and executed the projects that, among other brought voice capabilities to GPT-4 and continue to improve the performance of chat GPT. He was previously a project, a product manager at Scale AI, and in a past life, a hedge fund investor. Spencer works closely with AI trainers on research projects. And he's also one of my favorite people to work with at OpenAI. So welcome, Spencer. Thank you for coming.
Thank you, Nat. The feeling's mutual.
Oh, thank you. So Spencer, I know a lot of the folks that are here tonight have a lot of questions for you, and I've tried to target just a few. First, how do we determine who is a good fit or qualified for one of these research projects?
It's a really good question. And there's really no one right answer. I'd say, you know, for some of them, it's like really strong technical ability, right? Like very complex coding questions, kind of new, like trying new architectures and like real like coding challenges. But in others, it's really focused on like problem solving for like capture the flag, for example, requires like a level of ingenuity and kind of resilience to very advanced models that you're, you know, you're always trying to be like two steps ahead from something that's really advanced. And so I'd say like in terms of what we look for probably varies, but in terms of how we determine who's a good fit or qualified, there's usually a series of like screening questions. So if we think your profile is a fit or if you know that there's someone else in the forum, like working on a project and you want to pass it, toss your hat in the ring, that's always a good start. But after that, we usually have like some onboarding assessments to make sure that kind of your relevant skill set and your like work product kind of matches whatever research team is looking for.
Awesome. Thank you so much, Spencer. And if someone isn't a great fit for one particular project, they probably shouldn't lose hope because there could be another one coming down the pipeline that would be a better fit, right?
Totally. I like the way I think about this is that as our models become more advanced, like the reliance and kind of like opportunity for AI training for members of the forum and like expert experts as well is only going to increase, right? Like the level of human involvement that we need in these models, both to ensure safety, evaluation and future training is only going to increase. And we definitely value technical skills and critical thinking abilities, but also expertise in very niche domains, right? Like even like I know the humanities is an area where we really want to start to recruit more folks because we're also going to be training the models in that area.
Totally. I think that was something I wanted to make sure I touched on as well. It was like when you really think about like what, you know, quality looks like on these AI projects, it's not just like about securing an objective, but it's really focused on like having exceptional levels of thoughtfulness that I think is very common in the work that we receive from our trainers. So, you know, in some it's like evaluations that stump our state of the art model. In others, it's like examples of like Pulitzer Prize winning poetry, right? Like both of these are necessary and relevant. And so like the level of skill set is not really defined by domain, but rather around like exceptional ability and thoughtfulness.
Awesome. Thank you so much, Spencer. And how does human data define quality on these projects?
Yeah, so I think basically alignment to research objectives is like how I think about quality and it's like how our team kind of like focuses it on it as well. So like that thoughtfulness or kind of capture the flag to certain objectives or, you know, maybe coming up with a really hard question that, you know, chat GPT can't answer, right? Those are very valuable for us because if we try to use an evaluation set to test our future models, which should in theory continue to get smarter, we want to start from the hardest set of questions possible to see how we improve over time. And so that's like what we look for in quality. But I understand it's like maybe a little daunting to think like how do I like produce quality if I don't really have access to researchers or feedback? And I think that's one of the things that our team candidly does very well is like providing access to researchers and the end user and data users to ensure that we're giving you like the feedback and like opportunity to really like shape your contributions to open AI.
I definitely agree with that. I can speak on behalf of the awesome human data team folks and they set up office hours where you get face-to-face time with Spencer, operators, research scientists. They invite some of the most actually of the project contributors to a Slack channel where you always get this opportunity for rapid feedback. So there should never be any question about what quality looks like. I think sometimes maybe it takes a little bit of time to fine tune what it is we're looking for, but that's what's cool about these opportunities is that these are our collaborators.
A hundred percent. And what traits does OpenAI look for, Spencer, in a great expert trainer?
Yeah, you know, I think like the more I kind of like think of like the profile of a seller, you know, AI trainer is I kind of focus on like two like elements that keep bubbling up. One is like that level of thoughtfulness, right? And like not only having like the right answer or the correct answer, but one that's like robust and meaningful and kind of goes above and beyond. And the second is closely intertwined with that is like understanding the mission of open AI and like how our products work. I think in some of these engagements where you have to interact with a model, like you really have to get a pulse for how it acts, right? Because you're trying to kind of outmaneuver it, outsmart it and figure out elements where you know more than the model in certain domains. And so it's that level of engagement, I'd say, that really, you know, you, our best trainers are ones who kind of like approach this with a level of rigor and seriousness as they do in their other endeavors. So like, aside from like creativity and like accuracy, it's really thoughtfulness and ability to like integrate with our products. I love that. And I think once we hear from some of our expert trainers that are in the forum tonight, we'll also find that they really enjoy the work. They are motivated because they're curious about it. They're really curious about engaging with the model. So that all also makes a lot of sense to me.
And what, from your perspective, Spencer, are some of the benefits of contributing to this work, specifically with open AI?
It's a great question. I'd say, you know, beyond like the compensation, which we offer, I'd say like most of the benefits really come from not only, you know, knowing that you're contributing to open AI's mission, but that you're also kind of shaping the future of safety and evaluation in frontier models that are used by hundreds of millions of people a week around the world and like will only become more significant and prevalent in their use. And beyond kind of like that intrinsic feedback, I'd say like the extrinsic recognition in the form, the access to like the incredible network that you curate, access to all of our researchers, right? I'd say we have a lot of office hours where like we try as a project is concluding to allow trainers to basically like do and ask me anything with some of our researchers, ask about like how the data may be used to the extent that we can share, and also just like questions about their life working at open AI.
Yeah, awesome. I think those are awesome benefits. And we also try to make sure that open AI is represented at all of these events. So for those of you who are new here this evening, you'll notice that all of our virtual events and our in-person events also incorporate many of our teammates at open AI because they really wanna get to know you as well. They find this community program very valuable and they're just as invested as you are and as Spencer in the human data team.
So I think we did a really good job of opening up the conversation, Spencer. I wanna remind the audience that this is the right time to be asking your questions. If something came to mind while I was chatting with Spencer, please drop it in the Q&A now and we'll get back to it in just a few minutes. So thank you so much, Spencer. We're now gonna move on to our expert AI trainers in the community. These are trainers that we've been working with for a while, several projects this year at least. And I think they're the epitome of what we're looking for because they're so curious, they're so interested and they really want to have an impact on how the models are gonna be performing and impacting their domains.
First, I'd like to introduce you to Samar Abedrabbo. Samar is a microbiology professor with a profound passion for integrating AI into healthcare research and education. And I can attest to her profound passion. She often inspires me. She's dedicated to enhancing efficiency and advancements in these fields. Motivated by the potential of AI to bolster education and healthcare, particularly influenced by her Syrian background from a war-torn country, she's deeply committed to making these essentials more robust and accessible. Holding a PhD focused on the stomach pathogen responsible for stomach ulcers and cancer, she now teaches microbiology lectures and labs aimed at students aspiring to enter nursing and medical schools, which I also very much respect, Samar. She's enthusiastic about the role of AI in making STEM education both more efficient and engaging. In her daily career, she utilizes chat GPT for teaching infectious diseases and conducting microbiology experiments in the lab. I also want to note that Samar is totally a passionate educator. She's even created slides for me and for the human data team to help the AI trainers that we are working with understand the project better and understand the tooling that we're working with because she's so good at connecting with students.
So Samar, welcome. And now I would just love to hear a little more from you about your background and what brings you to OpenAI as an expert AI trainer.
Thank you so much, Natalie. Hi everyone, I'm so happy to be here. So I'm currently a microbiology professor and I mainly teach students who wanna go to like nursing and medical school. And it's my favorite subject to teach because I love infection. And my passion for AI started when I recognized its potential in education and science and in healthcare, which are my three big passions in life. So just to tell a little about myself, my PhD research was on bacteria that causes stomach inflammation. And so during my PhD, I did a lot of microbial genetics and just manipulation on microbes to make them make people sick so that we can study them better. And so this taught me a lot about science and how pathogens are created. To back up a little, coming from a Syrian background, I've seen firsthand challenges in accessing quality education and healthcare. And there was even a year in middle school where we couldn't attend school in Syria because my parents did not have the resources and we didn't even have healthcare when we came to the US. So I often think of these days with the creation of ChatGPT and OpenAI and how that could have changed someone's life completely, learning how to apply to college. Doing SAT prep classes when people are taking these expensive tutoring classes. So for me, equity and education in science and education is something I deeply care about. And I feel like that's where AI comes to be a revolution in changing this. And every day we're seeing it make huge changes. So one day while I was teaching, I received an email from Natalie inviting me to the OpenAI forum. And I was so excited because I had been using ChatGPT like the moment it was created, I think December or something it launched. And that's when I got on and I started using it. And eventually I became a biology AI trainer and model evaluator, and that's been a great experience. So I use ChatGPT in my everyday life, both as an educator in our labs, I have students use it and I tell them, okay, someone has a sore throat, what do they have? Do they have a cold? Do they have COVID? Do they have strep throat? Do they have cancer? And I've noticed that even though people have a misconception that it can be used for cheating, it's taught students how to become critical thinkers and unconsciously become prompt engineers and tell the model when it's wrong and when it's right. And so that's been a way where I use it. I also always think of how we could have used it with the COVID pandemic, how we could have used it to revolutionize understanding like viral genetics, how the virus spreads, just taking all the data, putting it together. Now to end this, one of the most rewarding aspects of being an AI trainer has been the amazing and diverse people I've got to meet virtually and in person. So there's some of the most incredible people I've met like Natalie and everyone at OpenAI, at the events, people who work there, people who are from amazing companies all around the world, not even the Bay Area. So that's been one thing. The other is, that's been super rewarding is the projects are so fun. They're very challenging, but there are questions that, especially if you love critical thinking, the questions are amazing. So you'll learn more about the training if you become an AI trainer, but it's-
Really, really fun. And I'm really grateful to be here. And I hope that this work definitely helps with the safety of, even though it helps advance education and healthcare, as we can see, there's also the other side of it of creating pathogens, which are microbes that can make you sick. So hopefully people working on that side and all the other realms of it can see the huge potential that OpenAI is making. So thank you for having me here.
Samar, thank you. I think we're actually equally, if not more grateful to have you. So thank you so much for sharing a little bit of your story with our potential and active trainers in the audience tonight.
Next up is Naim Barnett. He's a tooling engineer with a background in engineering science. He recently earned his master's in computer science, specializing in AI from Southern Methodist University. Naim, thank you so much for being here. I've gotten to see you two times in the past month because Naim also joined us all the way from Austin, Texas for the in-person version of this event. So Naim, will you please tell us a little more about yourself and how you ended up and why you continue working with OpenAI training projects?
Absolutely. I'm glad to be here. So I was born and raised in Austin. I went to Trinity University in San Antonio to get my undergrad in computer science or engineering science, sorry. And then I moved up to Dallas to work full-time as a tooling engineer. And that's where I started my master's in computer science, specializing in AI.
Me, I'm sure, along with a lot of other people in the chat and the forum, joined STEM because I just love people. I love helping people, helping the environment. And I think AI has a big contribution moving forward in the future to improve everything, right? And so I've known Natalie for a long time and she's always getting my background in computer science and AI. And so she reached out and thought I'd be a good contributor to work on some of the models and training. And she gave me the opportunity and it's been fantastic so far. Really challenging, but we love a good challenge. So I'm happy to be here and happy to continue contributing.
Thank you so much, Naim. And I've actually known Naim and his family for a long time. As Naim says, I'm from San Antonio, the same hometown. And now we're both in Austin. And I remember, Naim, your dad told me that you were loving the work, but that it was hard. He said it was so challenging and that you were staying up really late outside of your regular job to perform some of the work. It was really hard, but I mean, the challenge is one. Yeah, I love it. That's awesome. We're so happy to have you, Naim. Thank you, glad to be here.
Last but not least is Declan Grabb. He's a psychiatrist interested in human-computer interaction, particularly focused on human-AI interaction. He's a forensic psychiatry fellow at Stanford and also working as an AI fellow in Stanford's Brainstorm Lab. He conducts research on human-AI interaction, paying particular attention to sycophancy and persuasion of vulnerable individuals. He also helps students, faculty, and companies ensure their AI tools are improving mental health and not contributing to harm. His forensic work involves treatment and evaluation at prisons and maximum security mental health facilities. He will be co-leading a course at Stanford in this year's winter quarter on AI and mental health. He gets very excited about the ability of AI to democratize access to mental health care, but he also works hard to make sure it does no harm. He loves to use ChatGPT to generate images of shih tzus doing ludicrous things in order to make spam his partner with said images. They have a shih tzu named Chewy.
Thank you for that, Declan. And he also uses ChatGPT to help code for his projects. Declan, thank you so much for being here. We've also spent a lot of time together. You helped us out with SFHacks, a hackathon where we were there to meet students and recruit them to help us with projects. And it's always a pleasure to incorporate you into not just the work, but also share your story. So would you like to share a little more with the folks that are here tonight and let us know a little bit about why you've been contributing to projects?
Yeah, of course. Thank you so much for the intro. So yes, I love generating pictures of shih tzus, but beyond that, yeah, most of my work is kind of focused on the overlap of mental health and AI. So I'm a psychiatrist and I'm very interested in seeing how technology impacts mental health, both kind of in a positive way and a negative way. It all sort of started when I fine-tuned a model with a friend to sort of streamline mental health intake appointments. And I saw how powerful that sort of technology could be, but you also sort of saw where the errors happened and how, at times, that could be actually kind of pretty impactful in a negative way. So that sort of then motivated me to pursue a lot of original research and mental health risk and AI, which has sort of now led me to Stanford, a forensic psychiatry fellowship, and an AI fellow position in one of the department of psychiatry labs here. So I sort of am two minds, exactly like you said, where I, as a doctor, took an oath to do no harm, and I feel like that sort of extends to technology that has impact at scale. But at the same time, I'm so excited about the ability of these technologies to really democratize and scale access to proprietary information, like proprietary meaning doctors have a lot in their heads, it's hard to access, and it's expensive to go get it. We heard even, I think, Lillian say they're not on call 24-7. So I think it's interesting to see how these technologies can really improve everybody's wellbeing. And I think AI training has just been an amazing way to sort of experience technology impacting at scale. I always think like when I sit with a patient, it's an amazing honor to be able to sort of impact someone's life one-on-one. And it is definitely time well spent, but I often really love to then spend my time on kind of iterative improvements of technology to sort of leverage, I would say, like my domain expertise in mental health to figure out like, how do these tools, like Spencer was saying, that hundreds of millions of people are using, how do we make sure that they are gonna kind of just improve mental health globally? Something that I think is really, really important, obviously, I'm biased. And yeah, and I'll be leading a class on that at Stanford in the winter. So I'm happy to be here, thank you.
Thank you so much, Declan, that was awesome. And I also had a Shih Tzu growing up. I wish I could have used Chat GPT to generate. Yeah. It was this phone. I actually have a photo of, a little photo of, he lived to be 19 years old, by the way. We'll have to chat about that some other time.
Okay, guys, we're gonna move on to the Q&A from the audience. And we're gonna start with the questions that were most upvoted. So first, one of our trainers says, they've only heard about Project Lion. How common are expert human data projects at OpenAI? Like how often are we launching new projects? And I think this question would be a good one for Spencer.
Cool, yeah, happy to take this. I'd say I get, I don't know, maybe four to five requests a week from researchers for contributors of this caliber. So a lot of the time, like I'd say, Project Lion is our largest project leveraging our expert trainers, which is probably why most of you have heard of it. But a lot of our smaller engagements work with between five and 15 trainers, multiple times a week. So definitely more to come. I'd say the velocity is increasing significantly.
Thank you, Spencer. And somebody asked, how many people are usually working on a project and what kinds of collaboration are team members doing throughout the project? And I think we could give this to Spencer, but I think we can also give it to the AI trainers because you guys have worked on different projects. So while we can't talk about the details, I think we can talk about the environment. So maybe Samar, you can talk about the in-person collection first. So like how many people were around you? What, was there any collaboration on that particular eval?
Sure, sure. So just to give broad information, because a lot of it, we do sign an NDA, but in-person had, the one that I went to, I think had about 30 people in the room. And for that one, there wasn't really collaboration. So everyone got their own device and you got to train the model and this was said I will say some people were allowed to use chat gpt others weren't so but besides that was a really fun part see who got able to use it and who didn't so that was that experience and then there's the virtual one as well and both are very fulfilling in their own ways and also maybe fulfilling for Samar because she had a lot of wonderful critical feedback about the in-person one that we took and will be incorporating into the next one so you also get to teach us at open ai how to be better teachers so thank you for that Samar
Naim i don't know if you were ever able to come to any of the working sessions with one of the projects that you've been working on but if you have can you tell us a little bit about it or um the expert questions challenge like has that been collaborative at all what does the work look like uh you definitely do have opportunities to speak to other experts uh i unfortunately haven't been to any of the synchronous sessions but you can sign up for one every week they also do have office hours to come and talk about any problems that you're having but everyone in the form in the project has been super helpful so there's never an opportunity or a time where i feel as though i don't have help with something or i can't reach out to someone so you'll always have help and different people to bounce ideas off from
Thank you Naim and would that take place in the slack channel then if you're doing it um virtually you can do in the slack channel uh there is a link for the office hours and the synchronous sessions that are outside of the slack channel okay awesome
Declan, I know again some of this is very um we're not allowed to talk about it but is there anything about the environment um through these projects that you've contributed to that you might want to highlight for folks here just to give them a sense of something different that we've already heard from yeah for sure just i mean i think the scale like some some groups are really small some groups are really big and i feel like there's a lot of spirited conversation no matter what you end up doing um you meet a lot of cool people
thank you Declan there's a question here that i'm going to take um because i specifically know the answer to and the question is is there a large overlap with experts of the red of expert trainers from the open ai forum and the red teaming network and i will say there's not a lot of overlap overlap there's a little bit the red teaming network wants to make it very clear that that is a very specialized program focused on a very specialized type of data collection and model evaluation and it's highly sensitive so there's not a lot of overlap but we do collaborate with open ai forum members and red team members when it makes sense but those two types of collaborations are actually quite distinct
what type of technical training do you give to trainers who need help interacting with a system to give it feedback that is a great question so i think here guys we can focus on um interacting with the model system but we can also talk about what kind of training you get to interact with all the other tools that we use like the time keeping tools and even some are like the ipads that we use in one of the evals so i am going to let Naim start on this one
okay uh so there is a session that you can join for the onboarding process so if you do get the opportunity to work on a project there is an onboarding meeting that you'll join and they'll walk you through the steps of how to submit your time card and how to write questions and and do all these uh all these things there's also videos that they've pre-recorded to to give out you can watch at any point so a lot of helpful resources and if you have any questions you can just ask them on the slack channel and they're always really helpful responsive
thank you Naim i can share that in our very first project where we were using experts maybe it was a second or third but it was early up there and Samar was involved and we were talking about onboarding onto green light onboarding onto some time tracking mechanism onboarding onto you know you name it all these different applications and we were just throwing them out left and right in in a way that was not conducive to learning and Samar made a beautiful deck like that that laid it out in a very organized way that we still use today so Samar do you want to speak a little bit about how we have prepared you know the ai trainers for the projects and how to actually execute them or you can talk about the tools but you can also talk about like how to interact with the model during one of the evaluations
sure and to um to say to add to it and i imagine i think about onboarding is really really great you get this onboarding uh you get a lot of emails about what to do you're added to this slack channel which is like direct messaging if you're not in tech so you get access i think that the really nice thing which i love about open ai is as Natalie said they're very open to feedback and i love that because you can just say i don't understand something without anyone making you feel like it's not okay to say that which is amazing so you get you get the project and you get told all the details about it and honestly at the beginning if you're not in tech like i'm not in tech i'm in more science and education it may feel overwhelming but as time goes on everything makes sense that's what i've learned so if you get on a project in the beginning if you're a little confused not about the project but about all the details like how you get paid how you communicate with each other just give it time and feel free to ask questions a lot of us had a lot of questions so ask as many questions as you want and to anyone even other people there it i felt like that's the best advice i can give you is don't feel like you have to know everything so it's okay to ask questions but they do an amazing job training you and answering your questions when you're confused
thank you Samar and i will add that i started working in tech just four years ago and in the first tech company i worked in they're like yeah we added you to a slack account so i'm particularly sensitive to these things that Samar is talking about where now i've been using slack for four years and it just feels like it's ingrained in my dna but it's not the way that other people across donate domains are communicating with each other on a regular basis so we are learning as we go and we're getting better at training and and yeah and people like Samar and Declan and Naim have definitely helped us with that so thanks guys this question is going to be for you Declan and and i think you can approach it in a couple of different ways but the question is how do you balance the automation capabilities of ai with the critical thinking and creativity of human researchers in the projects
yeah i think um like you said yeah you could approach that from a lot of different angles i think i mean the whole reason there probably is like a human data team at ai is because of like the very valuable information that comes from humans sort of interacting with models it's very easy to sort of automate things and i would say that like even in some of the projects i've done outside of open ai in terms of like crafting prompts or um you know generating questions you're sort of introducing a bias if you use a language model to like create sort of information that then you're going to evaluate a language model on which sort of means like the human piece is invaluable right because if you want to truly have kind of untainted data the human piece is is really like um top tier um i will defer the question probably to Spencer maybe who knows it a bit more or anyone else but to me i think like the human piece is is really really fundamental
um for that reason Spencer would you like to add just a tad to that i feel like Declan like absolutely the best
awesome thank you Declan um yeah i'll i will add just a tad then the reason we value this community so much is because we don't believe we can automate the interaction we don't believe that we can just pull inner you know data as it exists from open data resources and continue to make our models safe and and continue to advance and that's why we value this community so much there actually is nothing like having humans in the loop and i personally
don't believe that will ever go away. I think that experts are going to continue to be needed to be in the loop until the end of time with AI. That's just my stance on it.
OK, we're going to just do one more question, guys. We're supposed to go into networking in two minutes, but we'll just take our time with this last question and go into the networking event once we feel like we've sufficiently answered it.
Oh, this is a good one. And Spencer, I'll give this one to you. And maybe we'll have time for two then, because I'm not sure if you have an answer for this.
Are there future projects related to democracy, peace building, AI training? That's a really good question. I'd say most of open AI's intense focus on responsible public government use of AI probably goes through our global affairs team and a lot of our government partnerships team. That said, I did see a question in the Q&A that maybe I'd dovetail in there is just future upcoming projects.
I would say just projects that go deeper in your level of expertise. So if that is peace building and focus, it may not be focused on how we can bring peace through LLMs, but rather perhaps orthogonal contributions to the safety of our model for that. I see a lot of people in the chat who say chat GPT helps them be better doctors. And I think Samar had great examples of the sore throat and really diagnosing patients, potentially, is just bringing that level of expertise to the model to understand where maybe it erred. And it said, oh, your sore throat is something serious when perhaps it was benign.
Trying to get that deep level of expert feedback that only intense practitioners have, I think, will become much more valuable in the future.
Thank you, Spencer. And I just remembered, actually. I guess it didn't immediately hit me as an AI peacekeeping project, but last year, we launched a grant initiative, the Democratic Inputs to AI. And we incorporated 20 different teams that built products or presented a product that could be built that would either incorporate broad public inputs or democratic process into AI. And many of those project contributors were actually expert peace building.
I honestly, forgive me, I don't even know the terminology for it. But Colin, for instance, on one of the projects, if you guys, if you look in the forum, you'll find the demo day for the collective, for the Democratic Inputs to AI projects. And one of them was presented by a man from Oxford University. And he spent his entire life forging peaceful relationships between warring communities. And I think one of his specializations was in the Inuit community.
So these are definitely things that some of our research teams are thinking about. In addition to global affairs team, our policy research team is thinking a lot about this. So if you're interested in that type of work specifically, please DM me in the forum. And it's my job to plug you in to all the things that are happening. And sometimes it just takes a little bit of research.
OK. So we're going to move on to the networking portion of the event. Naim, Declan, Samar, and I are all going to be around for that. At the end of that, we're going to join back into the live stream. And for anybody who's still around, we're going to choose three members of the community that are going to get something really cool in the mail.
One of the Bagu totes, they're black, and they have the OpenAI Forum logo on them. I take mine everywhere. And also a really beautiful blue Ripple water bottle. And if you win, then please DM Caitlin and share with her your mailing address. And you're going to get it in the mail from us.
One last tip as well about the networking. The default for networking is to keep you matched with somebody for 10 minutes. And there's really no way around yet for us to change that yet. But you can manually move on to the next match whenever you want to. So I actually highly suggest that you get the most leverage out of this networking opportunity that you match every three to five minutes.
As soon as you feel like you've completed a thought with each other, you've introduced yourselves, you've answered the prompt, go ahead and move on. Because you can always come back to that person. You can DM them in the community. You can LinkedIn. You can DM them in LinkedIn. And you can sign up for a coffee date with them. But the point of this is so you can meet more members of the community because they're totally awesome.
So now one of the members of our team is going to share a notification with you. You're just going to drill into that notification. And it's going to automatically bring you into the one-on-one networking event. And you'll automatically be paired with somebody else. And then I'll see you back here in about 25 minutes.
Spencer, thank you so much for joining us today. Everybody else, I'll see you in just a bit.
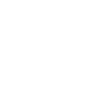