ChatGPT Enterprise 102: An Intermediate Guide to Your AI Work Assistant
speaker
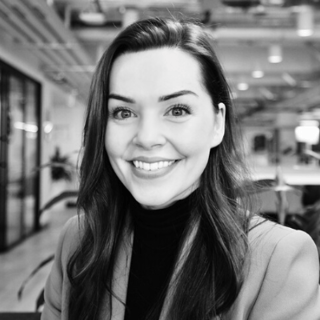
Lois Newman is focused on Customer Education at OpenAI, specializing in user education and AI adoption. With over 10 years of experience in SaaS, she has extensive experience in developing and delivering engaging content, from large-scale webinars to stage presentations, aimed at enhancing user understanding and adoption of new technologies. Lois works closely with customers to ensure ChatGPT is integrated into daily activities and effectively utilized in the workplace. Lois is known for her storytelling approach, making complex technology relatable and accessible to all audiences.
SUMMARY
At the event, Ben introduced Lois Newman from OpenAI, who provided insights into using ChatGPT Enterprise effectively. Lois discussed the recent launch of new AI models and emphasized the importance of prompt engineering to improve interactions with AI. She introduced the 'OK, Better, Best' framework, which helps users optimize their prompts for more effective outcomes. Additionally, Lois explored the concept of GPTs (Generative Pre-trained Transformers) and demonstrated building a custom GPT to automate tasks, illustrating the practical applications of AI in workflow automation. The event concluded with Lois addressing how these technologies could enhance productivity and strategic decision-making across various business domains.
TRANSCRIPT
Welcome, I am so excited to host this event where you get to hear from Lois Newman from OpenAI. If you've been here before, whether it's your second or third time, I'm so excited that you are part of this community. Now if this is your first time, which I know I saw many names on the list, for example, I saw Dean Boudreau, Ian Baker, Bart Kolandowski. Welcome to the forum. This is definitely where the magic happens.
Many of you know me. My name is Ben, and I am the OpenAI forum ambassador. I'm also part of the human data team here at OpenAI. Many of you also know Natalie Cone. I would call her the forum maestro and total mastermind behind all forum initiatives. If you didn't know, she also has a son who is a freshman in high school, and he has a really important football game today. So I'll be stepping in, and I'll be hosting this event with Lois, and I'm so excited, and also as Natalie is able to be with her son. So we always like to start our talks by reminding us all of OpenAI's mission, OpenAI's mission, which is to ensure that artificial general intelligence, by which we mean highly autonomous systems that outperform humans and most economically valuable work, benefits all of humanity. You may know that the forum will be having a series of unfolding events throughout the rest of the year. The forum team is definitely hard at work. We are listening to your all's needs and requests. Our sessions will become more advanced over time, and we're tailoring those needs to the things that we're hearing.
Tonight, it's a very special occasion because we get to learn from Lois Newman. Lois is a customer success manager at OpenAI, and she specializes in user education and AI adoption. Lois has over 10 years experience in SaaS. She has tons of experience developing, delivering, engaging content. I've seemed her on large scale webinars to stage presentations, and really her whole focus is on enhancing user understanding and adopting of new technologies. So Lois works closely with customers to ensure Chachabity is integrated into not just one activity or two, but we're utilizing these across the entire workplace. I've only met Lois a few times, but I'm already blown away by her storytelling approach. She makes complex technology relatable and accessible to all of audiences. And I will also flag that this is the 102 version of her talk, so you're going to find the 101 version in the forum. We have an amazing feature where we've created playlists, so you will find that if you want to go ahead and check those out.
So I would love to welcome Lois to stage. Welcome, Lois.
Hi, Ben. It's an absolute honor to be here. I'm really excited to take you through some of the material I've got. As Ben mentioned, I'm on the customer success team primarily focused on ChatGPT Enterprise. So I have built the webinar program here, and I train thousands of users every month. And really, my hope is that we get high quality education out there into the world to kind of help us achieve our mission.
So what I'm going to do is I'm going to jump over and start sharing some slides. One second. Let me share those again. There we go. So as Ben mentioned, this is the 102. Natalie and I caught up this morning and decided that we needed to make a bit of a pivot, because 101 is very foundational. And so I'm actually going to be taking you through some of the material I present every week to users in the webinar series. And today, I'm going to focus on prompt engineering and GPT building. I'm really hoping that you can leave with some tangible tips and tricks. But I always like to say to my audiences, if you find that this material is too foundational, try and come in with a new mindset and think about how maybe you can use some of my talk track, use some of my tips and tricks, repurpose the material, and then push it out to your own communities. So you can either be a user today learning this, or you can be thinking about ways that you yourself can actually shepherd AI into your own communities.
Let's take a quick look at the agenda today. I'm going to start off with a very exciting update. We launched a new series of models last week, so I want to tell you about those models today. I'm then going to pivot into prompt engineering, specifically focused at the 4.0 model. So how we actually communicate with 4.0 to get the best output. I'm going to introduce you to one of my favorite frameworks. I really like the OK, Better, Best framework, because it helps users to understand where they're going wrong and how to improve their prompts over time. And then, very exciting, I'm going to introduce you to GPTs, and I'm going to do a live demo at the end of the session, which is a full build of a GPT to help you be more productive and to automate some of your workflows.
Okay, so I'm going to kick off and start off with just outlining the 01 preview, which is a research preview we launched last week. So it's always good to kind of take a look at where we've been and where we're going.
When ChatGPT launched in 2021, that was with the GPT-3 era model. And a lot of you today are probably familiar with the GPT-4.0 series, and that's kind of where we're at today. However, last week we did make a big announcement and we have launched a new series of models. And I guess that kind of sits in the GPT-Next category. We do feel that because these models are now able to reason through complex tasks, that we've moved into a new paradigm with some of these models and is maybe an insight into where AI is going and where some of these models will take us in the future. And so with that, I do want to introduce you to OpenAI-01, and so a new series of models. And this series work very differently to the GPT-4.0 model, which is our flagship multimodal model. So instead of responding right away, the 01 series has actually been trained to think deeply for longer, and often that results in better answers. So these models can reason through complex tasks and solve harder problems in domains like science, coding, strategy, logistics and mathematics. We've also found that these reasoning models are better at understanding how to be most helpful with less context and actually fewer prompts, even though it's able to provide a more comprehensive output. We've also trained 01 to produce like a chain of thought before producing a response, and that can be really helpful for users to actually follow along with the model and to understand how it actually came to that answer. It's also able to verify its work. So it's very, very different and it's a much newer experience than when you're navigating and working with 4.0. I'm going to just move along to a quick example on this slide deck here.
We have got GPT-4.0 on the left and OpenAI-01 on the right. And what you can see is that the biggest difference is the response speed. So the 01 series marks a change from predictive responses to logical problem solving with the ability to reason. And through this training, the 01 series has learned to really refine that thinking process. It's able to try different strategies and actually recognize when it makes mistakes. And you can see there as well, there's a really that process of summary and that is the chain of thought. And both of these models right now in this very quick demo have been given the same task. And so the model is trying to tackle a conversion rate optimization question. 4.0's answer isn't bad. It's actually very quick. It gives an output straight away. But what you can see is that the 01 preview example gives a more robust prioritization framework and more accurately calculates the stack ranking of priorities. So just a snapshot at how they're different.
So the first two models in this new series are 01 preview and 01 mini. I just want to touch on the difference between these two because there is a difference. The key difference is that 01 mini is a smaller model. It's not as knowledgeable about world facts. In chat GPT, 01 mini is far better at writing and debugging complex code. What we found is that 01 preview is actually much better at tackling complex problems across domains. And it does have more of that real world context as well. One thing I will call out is that both of these models right now are, it's only processing text. So it is not multimodal in the same way that 4.0 is. With 4.0, you can upload lots of files. It's able to analyze images, PDFs, work with Excel files. Right now, the 01 preview model is not able to do that.
And so I really want you all to think about this release as it is a preview. It's for testing. It doesn't have full functionality and capabilities. And so we are recommending that you test and experiment. But for most of your use cases, you really stay focused on preview. Really stay focused on GPT 4.0. I want to call out a workflow where you might decide to use both models. At the moment, you're going to have to switch between the models. So you're actually going to have to select which 01 model you want to use. And then you're going to have to move back to GPT 4.0. But what's really great is that you can actually use these models together to work on an entire workflow. And what I've done for you here is I've really spelt out a workflow and where each of those models would be appropriate. For example, let's say I am in the research phase of creating a product. 01 is really well designed to help me with some market research and to actually survey and create surveys for a target audience. It's really, really good at that. But once I've kind of done my research and extracted that information from 01, because it's not multimodal and I can't upload an Excel file, I would need to switch back to GPT 4.0. And that is where I would do my analysis. That is where I would upload my survey data and work with 4.0 to extract insights. Then potentially we move towards some strategy. So we have an understanding of our survey results. We're now looking to understand how we actually bring that product to market. And again, we would move back to 01 because 01 is very, very good with strategy. And you can see there the final two sections of the workflow. We would move back again into 4.0 to actually execute on the task and create that content. And that final piece of content might be something like a blog announcing the new product. Eventually, these will feel more connected and the experience is going to change over time, so just bear with us. It's going to improve. But I wanted to give you a workflow so that you can really understand the context in how these models can work together.
Great. So now I've introduced you to 01 Preview. I'm going to come back to focus on GPT 4.0. GPT 4.0 is our flagship model. That is the model that will be in TrackGPT by default.
And GPT-4.0 requires a certain prompt engineering framework, allowing users to communicate with it effectively. So everything from this point onwards is going to be 4.0. If you want to find out more about 0.1, please check out the OpenAI blog.
Okay, prompt engineering. What is it? I honestly don't love the term prompt engineering. It sounds super technical and somewhat exclusive. And I'm here to break down some barriers today.
Prompt engineering is simply good communication with AI models. That's it. And I'm going to walk you through one of my favorite frameworks today. Essentially, the better your input with ChatGPT, the better the output you can expect.
And when I'm working with users on a day-to-day basis, quite often they say to me, Lois, I don't really understand. ChatGPT is not giving me what I want. I'm finding that the responses are very generic. Or even better, sometimes people tell me it's like a glorified Google search.
And so that instantly in my mind, it kind of signals to me that we need to focus more on prompting and really crafting that good communication so that users feel that ChatGPT's output is valuable.
Again, it sounds very scientific. I've labeled this slide anatomy of a prompt. But really, a good prompt when working with GPT 4.0 requires three things. It requires context, role and expectation. So let me tell you a little bit more about that.
The context is really the material that you want ChatGPT to work on. The instructions and the role is really identifying to ChatGPT who you are and how you want ChatGPT to work on that material. And then finally, and this is probably the most important part of the prompt, is your expectation. What do you actually want ChatGPT to produce?
What I find is that when a user comes along and they start to piece that anatomy together and start to build their prompts, they get far more valuable output.
So what I'm going to do now is I am going to kind of do a series of prompts, but using a framework that I like called OK Better Best. And what you're going to see is that I'm going to improve the prompt over time, layering in different parts to the prompt. So let me show you what I mean by that.
Oh, sorry, my mistake. Let me give you a couple of guidelines first.
So very important that when you are writing this prompt, you are expressing your intent clearly and specifically. An analogy I always like to use is when I am communicating with ChatGPT, I always imagine ChatGPT is my colleague and ChatGPT is helping me on a task.
And so the more information I give ChatGPT, the more I can outline the steps of the task, the better the output.
Essentially, if I said to one of my colleagues at OpenAI, go and create a sales report, they'd probably look at me blankly because that type of direction lacks clarity. It lacks expectation. And there's a high chance that my colleague comes back with a report that is not what I'm looking for. And that's exactly the same as ChatGPT.
We need to be really clear in our communication and honestly, treating it like a colleague can be really helpful for prompt structuring.
Sometimes users ask me, should I add more text? Should I keep adding more text? And more text is OK if it is adding clarity and context. You can also add delimiters in your prompts and those delimiters can be used to signal to the model keywords or important pieces of information.
Something I'm going to show you in the next slide is an example of a few-shot prompt.
Few-shot prompting. Again, it sounds more technical than it is, but few-shot is where you simply add in examples for ChatGPT to reference to guide the model to a more specific output.
And then just over on the right hand side there, this is something I actually stumbled across only a couple of months ago. You can ask GPT 4.0 to actually slow down, not rush to conclusion. And you can also ask to specify steps and chain of thought as well.
I was going through this slide deck today and I actually thought this is really interesting because this is exactly the direction we've got in with the O1 series. And the O1 series is actually slowing down, providing its chain of thought to allow it to do more complex tasks.
So an example of few-shot prompting would look something like this. So your prompt would be analyze the sentiment of the following operational feedback using the examples provided as a reference. You've got the positive sentiment example closely followed by the negative sentiment example.
And really what you're doing here is you're guiding ChatGPT. You're providing an example and you're getting closer to a more specific output. OK, so we are at the point where I'm going to share this framework that I love. Sorry, I kind of skipped ahead of myself and moved on a little bit too quickly. But let's jump into that now.
OK, so an example of a basic prompt would be something like this. Can you summarize ABC Therapeutics latest report? And I often find that users who are new to ChatGPT, they will engage in a conversation with ChatGPT where they layer in question after question. And that's a very typical user journey. And it's pretty great at getting you to where you want to be.
But it is not going to give you the output you were looking for straight away. And so what we want to do here in this example, if we were to create a better version of this prompt, we would begin to layer in context and add in our role. So who we are and what we're trying to do.
So in the next example, I am saying I'm a clinical trials manager at ABC Therapeutics. Can you summarize the latest report of their ALS drug candidate? Focusing on key results, conclusions and next steps in development. So again, we're really layering in more information.
And very importantly, we've identified who we are and what we're doing. That actually offers ChatGPT more context and, again, will provide a more specific output. In the last version, I am going to layer in my expectation of ChatGPT and be very specific to the output that I am looking for. This is an example.
This is the best example. So this is a really great prompt. Not going to read it line for line here or word for word. We've got that same initial two sentences identifying who we are, kind of where we work and what we're doing. And then we're being very explicit about our expectations.
So here we're saying, please focus on the key results, clinical outcomes and any significant findings. Additionally, include a comprehensive overview of the conclusions drawn from the trial, the implications for future research and the next steps in drug development process, including timelines for upcoming phases or regulatory milestones.
You can see that the difference from the first prompt all the way down to the third prompt is it's pretty vast. There's a big difference. And I can guarantee you that the results you get from ChatGPT with the first prompt are significantly different than the last.
And so my key message is if you want to work well with GPT 4.0 or you're even working with users right now that are struggling, kind of adopt this framework where you're layering in or improving the prompt over time and observe the output that you're getting.
I just want to show you just one more example. So let's do this again. We're going to do this for a data analysis use case.
First example of a prompt. Using this data set from an external trial, can you provide a basic analysis of patient outcomes? Very straightforward. It's it's a question. When we actually layer in the context and who we are, we're adding in additional information for ChatGPT.
So we're saying I'm an analyst at ABC Therapeutics. And then we're adding in more information about the task at hand. So using this data set, can you provide an analysis of patient outcomes? And then we're giving the focus. We're actually asking ChatGPT to call out key metrics about disease progression, treatment response and survival rates.
Let's take a look at the best version of this prompt. So very similar introduction, context role. And then finally, a pretty lengthy expectation. So please apply advanced statistical methods to identify significant correlations or trends. Additionally, highlight any outliers or anomalies in the data. Discuss these insights and discuss these insights to inform clinical strategies and suggest potential implications for developing therapies for neurodegenerative diseases such as ALS or Alzheimer's.
So pretty wordy prompt. Honestly, I even struggled to read that out. But I think you get the gist here. The better the input, the better the output.
Great. So that was kind of an overview, an introduction into prompt engineering and the OK, better, best framework. And the reason why I did this before introducing you to GPTs is because GPTs and creating GPTs requires a good understanding of prompt engineering.
When I get to the building phase of today's session and I actually demo how to write instructions, I often think of the instructions within a GPT as a very lengthy prompt. And again, the more context, the more clarity, the more you outline the instructions, the better the GPT is at completing a task.
OK, so GPTs, what are they? Well, at OpenAI, we believe that the next step forward with GenAI resides in tailored and capable assistance available for every worker.
So I like to think of GPTs as mini experts or assistants that can help me with a specific repeatable task. If you're not familiar with GPTs, GPTs look a little bit like this.
We can see the HR helper, which has been designed to answer a user's question about HR policies. So this is a GPT we actually built at OpenAI. It has been designed for demo purposes, but essentially we loaded this GPT with HR policies and procedures and we started testing it.
And we noticed that users could use the HR helper like a Q&A or like an assistant before they actually submit a ticket to their HR department. And so what this is doing is it's minimizing the amount of tickets that get sent to HR that are kind of pretty basic and Q&A. That's really kind of allowing a user to self-help more quickly.
It's reducing the amount of time that the HR department have to sift through.
with questions and answer those. And so overall that type of GPT is very powerful, especially in a business setting.
And so obviously because I'm focused on chat GPT enterprise, I do work with a lot of businesses and a lot of users. And everyone right now is trying to figure out how can we automate our workflows with GPTs? How can we have many agents within the business potentially doing the grunt work and doing the work that we don't want to do?
I'm going to show you my GPTs in a second. I've built about 10 GPTs and I've probably saved myself around five to 10 hours a week through these GPTs. One of my favorites is my customer call assistant GPT. So I sit on a lot of calls, a lot of focus groups with users. I speak to a lot of businesses.
And so I have an AI bot that sits in my call. It takes transcripts of these calls. I then take that transcript, I enter it into my call assistant and this call assistant takes those notes and creates a formal email for me. And so after every call, this probably cuts out about 20 minutes and I sit on anywhere from kind of five to 10 calls a week. And so just by one individual in the business, me, creating my own GPTs, I've saved a lot of time.
And what we're noticing is as GPTs are being built throughout businesses, we're seeing that kind of huge return on investment where employees are being more productive, reducing that manual overhead and creating kind of these mini experts or assistants.
I'm going to talk now about the structure of GPTs. So very similar to the structure of a prompt, GPTs are powered with three things. Those three things are instructions, knowledge and actions.
The instructions tell the GPT or they tell the model what to do when a user interacts with it. The knowledge is, it can be a document, but it is essentially the context that is you're adding in expertise into the model through the form of a document. And that gives the relevant context, allowing the model to complete the work.
You then have actions, which is the ability to connect a GPT into a third party system or database. And that allows the GPT to kind of push and pull requests so that your GPT can also complete workflows in other systems. I will touch on that a little bit later. I have a JIRA assistant, which I use every day, which is great.
And so actions are a really powerful way, I guess, to kind of turbocharge the GPT. So you might be thinking, why would I actually build a GPT? A lot of users say to me, isn't chat sufficient? Can't I just repeat the task every time in chat?
And the answer to that is yes, you absolutely can. But then you are repeating the same actions, the same uploads, the same prompts, constantly when you're doing a repeatable task. And so GPTs really fit that gap. They allow you to automate that process so that you're not doing the manual process in chat GPT.
Here at OpenAI, we think of GPTs as falling into three categories. You have accelerators, which allow the individual to kind of do the grunt work in half the time at a higher quality. So the GPT I just spoke about, my customer call assistant, that is an accelerator, that really speeds me up. But I haven't really shared that GPT with anyone else. It's really for me and my personal use.
You then have enablers, which actually allow you to accomplish projects or kind of develop a skillset that you didn't have before. Those are GPTs where maybe you share with a couple of individuals, you might be working on a small project together, and that GPT is really helping with that type of work.
And then finally, you have transformers. Those are the bigger GPTs that actually redefine entire work streams. So an example of that would be the HR helper. You have redefined a pretty manual process using AI, and that GPT is now supporting a huge number of people across an entire business.
So, I just want to delve into the HR hero again, or the HR helper. This GPT was configured with instructions and knowledge alone. So all of the HR policies and procedures were actually uploaded into the GPT. So this GPT does not leverage an action. It's not connecting to an external system. It's able to operate using its knowledge alone.
Obviously that, for some businesses and for some users, that doesn't work. They would actually need to configure an action so that the GPT can connect to Google Drive, for example, and search for documents. But this specific GPT was actually pretty straightforward to set up.
The reason why I'm spending so much time talking about the HR helper is because when we delved into the instructions and how it was configured, I think we kind of cracked the code on what is best practice for creating GPT instructions. So if we skip over to the instructions, let's imagine we've just lifted up the hood of the GPT, and this is what you see underneath.
In the next part of this session, I'm actually going to replicate and build a GPT using this format. So best practice for GPTs is to have three sections of your instructions and to break it out in this structure. So you can see there in the GPT, we have a clearly defined objective, which is essentially the problem statement. It's really what is this GPT designed to do? What's it helping you to solve?
You then have a clearly defined section called what you do. And these are really the steps the GPT needs to take to complete the task. And then finally, a very, very important section, which I don't think is discussed enough, is a series of guidelines. And these guidelines kind of add in additional parameters. So you can see there, it says things like accuracy and clarity, privacy, compliance, limitations of knowledge. These are extra things the model can lean on to ensure a better response.
And this is why I paired prompt engineering with GPT building, because I think they are highly aligned. You can see that this is well-structured. We are clearly communicating to our GPT what it needs to do. And I really do think this human colleague analogy translates here. This almost looks like a process guide or a task outline. If we were to send this to a colleague, they would be able to work through the task and execute on the task. And again, that is exactly the mindset we need to adopt when we're building a GPT.
What you can also see here in this example is we have attached knowledge. So we've added in all of those policies and guidelines that we want the model to reference when it answers a user's question.
So I've given you the framework here and the format. And now what I'm going to do is I'm going to actually do a live demo for you. We're going to build a GPT today in the next 20 minutes of this session.
Let me stop sharing these slides here and let me come over to chat GPT. Great, so we're in chat GPT now. I'm just going to help you navigate to the GPT create button and I'm going to talk a little bit about the GPT store as well.
So this is a chat GPT enterprise interface. This is the area that I work in every day. And to explore the GPT store, I simply go to explore GPTs. Give that a second to load. And the GPT store, very similar to an app store.
This is where you can access third party GPTs that have been published, or you can also access GPTs that have been shared to your workspace. Now, just bear in mind, depending on the subscription that you have and the view that you see, it's going to look very different to mine.
So your admin settings may determine the type of view that you see in the GPT store, but essentially very straightforward. If you know the name of the GPT, you can actually search for it in the search bar. If you're looking for inspiration, I recommend that you kind of scroll along at the top and select a specific topic. For example, writing, productivity, research and analysis.
And you can kind of browse through and take a look at some of these really great GPTs. I have used a lot of GPTs that have been shared with me by colleagues, but I'm a big fan of creating my own. And so that's really what I want to teach you today.
I actually want you to leave with the confidence to go out there and build a GPT to solve something that you're working on right now. To do that, I'm going to go to the create button here, and that is going to bring you into this page here. So let me set the scene.
Something that I do every week is I manage this webinar program. I'm not a formally trained project manager, but I have kind of learned over the years how to coordinate and how to provide the business with updates about the program or the project that I'm working on. So that's the scenario.
Now, what I'm going to do here is I'm actually going to build a project management communicator. So this GPT is going to be fed notes from me or from the team, and it is going to help me create an email that I can share with my colleagues every week.
And so if you think about that process pre-AI, so in a world where chat GPT doesn't exist, I have to go through my emails, I have to go through Slack, I have to reach out to people and find out what their updates are, I maybe have to coordinate a meeting, and then I need to pull together all of those bits of information, and then I need to manually create that email. And so what this GPT is doing is doing that process for me.
It's a very specific repeatable task that I am trying to automate. First thing you'll note is when you get dropped into the create page, you will see that there are two options. There is a create tab, and there is also a configure tab.
Let me tell you what each of these do. If you are not confident to go straight in and start adding in the name and the description and the instructions, then you can lean on the create tab and actually have a conversation with the GPT.
So this GPT builder is designed to engage you in conversation, to actually...
actually tease out key bits of information. And what will happen is when you engage in a conversation on the Create tab, it will automatically configure this Configure tab.
And so you have two options. You can have a conversation with the GPT, or you can actually take control and configure it yourself. Personally, I like the Configure tab. I have a high level of control. And because I understand some of these best practices now, it's just easier for me to go straight in and configure the GPT that I'm looking for.
But again, I would test this week. If you haven't built one before, maybe jump into the Create tab, and then you can actually tweak whatever has been populated in this view.
First of all, we are going to start with a name. So let me grab the name of this GPT. We are going to call this GPT a Project Management Communicator.
And one thing you will note is that anything I add in this left-hand view will automatically populate in the Preview window. I like to think of the Preview window as a bit of an overview of how the GPT is going to look and feel. The Preview window also allows you to test GPT performance before you go ahead and publish it.
I'm now going to add in the description. And the description is what the user sees when they engage in a conversation with a GPT. So please don't underestimate the importance of a really clear and concise description. It's helpful because otherwise a user doesn't necessarily know what a Project Management Communicator actually does. So let's add that in, and you will notice it appears on the right-hand side of the screen.
So the description is, this GPT reviews written project updates and converts them into a formal weekly status email. And it uses a company communication template. So think of this GPT as your Project Management Communicator.
Great. We now are going to add in a series of instructions. This is the most important part of a GPT. And when I'm troubleshooting GPTs or when users come to me and say, it's not working, nine times out of 10, it is the way that they've structured their instructions. And again, back to prompt engineering. Think about this section as you would communicating a task to one of your colleagues.
What we're going to do first in the instructions here is I'm going to add in an overview. If you remember, that was the first part of the HR helper. It's really the GPT statement of intent. So I'm going to expand this view so that you can all see that clearly, and it might be a little bit small on your screen, so I apologize. But what we're saying here is you are a Project Management Communicator. You will receive written updates, convert them into formal emails, and you will use the communications template as a guide. That's our overview.
We now want to add the what you do section, which clearly spells out the task in hand. Let's add that in. And we're going to number these as well. So I always recommend numbering this section. It clearly indicates to the model the steps it needs to take, and I'm going to read these to you in a second.
So the first step. A user will send you a weekly written update from the project team. Confirm that the user has sent all of the updates before you move on to step three.
Step three. You will use the weekly project update comms template as a guide and populate the updates in the same email format identified in your knowledge. Just so you know, I've created an email template that's in a Word document. So that's what that template is referring to. Essentially, I decided that there is a format that I want to send these emails in. And so I'm providing that as additional context to the GPT to make sure that the output is specific to what I'm looking for.
And number four, provide the user with an email, which they can easily copy and paste into Gmail. Okay, so the final step is to now add in guidelines. The guidelines, again, are those additional parameters to make sure that the GPT responses are more clear, they're more accurate, and it also guides the model in the instance that it can't answer the question.
What we're going to do is add in a series of guidelines. Let me add those in. And I'm going to number them again, also important. And so here we are saying, accuracy and clarity. Ensure responses are accurate based on the internal knowledge and communicated clearly to be easily understood.
Number two, limitations in acknowledgement. If a query falls outside of your accessible knowledge base, or requires human judgment, direct the user to contact the project management department and suggest that they contact this email.
And then I'm also adding in an email. We're really giving the model an opportunity that if it doesn't know the answer, it can redirect the user. And that's exactly the same framework that we applied to the HR helper as well. We actually redirect the user to a HR stakeholder.
So what we've done is we've got the overview, we've got a section identifying the steps, and we also have some guidelines. And this really is best practice for creating instructions.
I'm going to close this view, and I'm going to talk a little bit about some of the other features within this view. So let's move on to the conversation starter. Okay, so I like to think of a conversation starter as a pre-loaded prompt. Not only does it help the user to understand what they should be asking, it actually takes the effort out of the user needing to craft the right prompt. So if you're creating a GPT, you're essentially the expert in how this should be used.
Really think about conversation starters as a way to help out the user. So pre-load as many conversation starters as you can. I am going to upload one for this GPT. It's a pretty straightforward GPT. And what I'm saying is, again, using the email format and your knowledge, take these written updates and create that formal email for me.
Okay, we're going to come down now to the knowledge section. So this is where I'm going to upload that template that I want the model to reference so that it creates the email in the exact format that I like. So we're just going to go over to here. I'm just going to add in the comms. And you'll see that that document has now been uploaded.
We're just going to come down to capabilities. So you can toggle on and off capabilities within a GPT. And again, capabilities are a way to turbocharge that GPT so that it can do something specific.
For example, you might create a research GPT and you want it to browse the web so that you can kick off some research in a certain topic or theme. You might create some kind of marketing GPT or brand GPT, and you might want that GPT to generate images using DALI.
So you can see here that you can toggle these on and off depending on the task within the GPT. Now this one here, code interpreter and data analysis, this capability is super important. So this capability needs to be turned on if you're creating a GPT that analyzes data.
So if you're uploading data to the GPT, you're uploading structured data like an Excel file, you will need that turned on so it can actually analyze the data. You will also need to turn this on if you want the GPT to generate downloadable links. So if you want the GPT to actually generate a Word document or generate a link to a CSV file, that code interpreter capability must be turned on.
So I'm going to leave that turned on. Maybe I actually want to create a Word document in the future with the full email. And I'm also going to turn on the DALI image generator because you will need that turned on if you want to create a GPT profile picture.
And to do that, all I need to do is click on the plus sign and select use DALI, and that should pull up a profile picture. And the reason why that's important is because it will help me identify the GPT in my sidebar. And there we have a nice creative picture. I don't know how relevant it is to project management, but I think you get the gist.
Okay, let's scroll down. Last thing I want to show you in this view before I actually publish this GPT and show you how it works is I want to talk about actions. So actions is the area of a GPT where you can connect it to a third-party system.
I think I mentioned that I have a Jira assistant. I have a Jira assistant that allows me to enter in unstructured text. It takes that text and it actually pushes that text into Jira and creates a Jira ticket in a backlog. So you can see how you can do some pretty interesting things with GPTs to speed up or automate that workflow.
I'm going to click into actions here. I'm not going to configure an action today because this GPT doesn't need it. But essentially, you can select the type of action you want. So you can have an API key, make a post request, for example, or you could also have an OAuth authentication type as well.
And this is where you would enter in that API schema and you would kind of test to make sure that it's doing the right thing or it's making the right call. We do have a whole webinar on custom actions. And so if you're interested in that, please let Natalie know. That's one that I partnered with an SE on, one of our technical team members, and that takes you through the full configuration because that alone is about 40 minutes in itself.
So coming back, we're really at the stage now where our GPT is working, and I'm feeling pretty confident. If I wanted to test it here in this window, I could. But for the sake of today's demo, I am going to go ahead and just publish this GPT and I'm going to show you how it works.
So we come up to create and create means publish. So when I click on that, what you're going to notice is by default, all GPTs are private to you when you create one, unless you specify otherwise.
So you can see that right now, this is just being shared to me and my workspace. But I could actually...
actually publish it across the OpenAI demo account. I could also consider publishing it to the GPT store as well. I can also share it directly with one or two other people. So all I'd need to do is type in their email address here. For now, I'm going to publish this GPT to my workspace. So let's see what happens. We're going to click on View GPT, and that's going to take us to this view here.
And what we're going to do is a live test. So let's give this a go. I'm going to add in a series of notes, a series of notes that I've collected from my project team that outline some updates about the project that we're currently working on. And really what I'm hoping is that I get this lovely structured email that I can send on to my colleagues. Let me just break out these notes for you. And again, I apologize if they're a little bit small on your screen.
So we have some rough notes from myself here, and let me expand this view so you can see these. So rough notes from me. We have a Slack update here from one of our colleagues with the task ID and some information about what's been done. We have an email update from another team member, again, outlining some things to do with a specific tool that we're focused on. And we also have a Slack message as well from Louis, just talking about a delay in vendor selection and some changes to some of the project dates.
Okay, let's minimize this and let's send it across to the GPT and let's see what we get back. Okay, perfect. Okay, what it has done is it has referenced that template that I uploaded in its knowledge, and it has completely replicated that template and created this formal email for me. So now all I need to do every week is I pull in all of this unstructured information, I feed it into this project management GPT, and I get this email in return.
Now, of course, as always, human in the loop, I always encourage every user to check the output every time. It's really a first pass, it might need some tweaks, and I need to make sure that the dates and the task description are correct. But I have done, I've made this GPT probably 100 times at this point, and every time using those instructions and those best practices, I get a working GPT almost every time.
Perfect, so that really comes to the end of my demo. I've gone through the O1 preview series, I've spoken about prompt engineering and also introduced you to GPTs as well. So I just wanna thank you so much for your time. I really hope that you took some tangible tips and tricks, and I'm really looking forward to answering some of your questions live in a second.
Great, well, thank you so much, Lois. It's probably no surprise that I am definitely a power user of TachiBT, but everything that you listed, even the taxonomy of custom GPTs was incredibly helpful. And you may or may not have seen the chat online blowing up about any questions, and so I'm really excited to dig into those.
So I'm gonna make a few closing remarks, and then we're gonna go to live Q&A. So we have a lot of events coming up, and you may have heard before yesterday many times that we have office hours. So tomorrow we have office hours at noon. There's no need to register to join the round tables in the forum. You just log in, you jump in. If you have questions, suggestions, or you just wanna say hello, you're more than welcome to come and just meet the team.
Next week we will be having two events. We have another technical success office hours on Wednesday. So these are very much catered to technical questions. I actually know that many of you have specific questions, and so I wanted to flag that anything you need, additional resources, questions for yourself, or maybe for your team, feel free to reach out to myself or my colleague, Natalie Cohn.
We try to do our best to make sure that we go and do our research and homework to get you what you need, and also to be able to support your work. So now that, oh, I'm sorry, one more event. So we will also have a virtual event, a virtual networking event that I will also be hosting. So if you've been to these virtual networking events, they're very much of 10 minute sessions. They're a lot of fun. We get to meet everyone in the community, and I hope you all can make it.
Now, I know many of you came here for specific questions and to go into the Q&A. And so to get to the Q&A session, there's gonna be, you'll find that link that's hopefully somewhere on your left side that's where you'll be seeing it. You can also access that in the agenda. So you're gonna click that live Q&A meeting room link, and we're all gonna go in there, and that allows us to engage and talk with Lois, and she can answer your questions, and then it becomes a conversation.
So I will see you all in that room. And I wanna thank you all again for joining us and Lois for your time. So I'll see you soon.
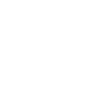