Advancing Diagnostic Medicine: The Role of AI in Future Healthcare
speakers
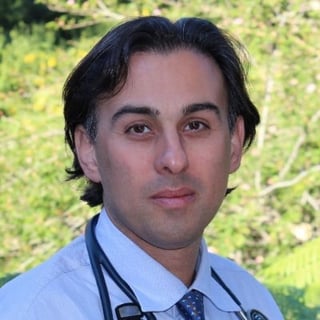
Dr. Shahram Yazdani is a Full Professor of Pediatrics at UCLA, where he serves as Associate Vice Chair of Clinical Affairs at the School of Medicine, and Medical Director of the Children’s Health Center. His research interests include computer-assisted diagnosis, with a focus on modeling diseases and their symptoms, as well as studying the impact of aviation pollution on childhood health. His current work aims to expand access to high-quality diagnoses for individuals in remote or underserved areas, while also reducing the cognitive load on healthcare providers.
Recognitions
- Super Doctors® Southern California, 2010-2025
- Neerhoot UCLA Department of Pediatrics Award for Excellence and Contribution to medical education 2010, 2013, 2024
- Letter of appreciation, Senator Ted Lieu for presenting the data regarding the effect of Jet propulsion pollution in children, 2011
- Letter of appreciation, Congresswoman Jane Harman for presenting the data regarding the clinical health effect of SM airport on children, 2010
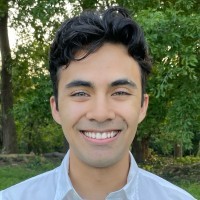
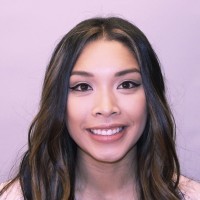
Mericien Venzon, MD/PhD, is a physician-scientist passionate about immunology and advancing treatment strategies by applying ecological and evolutionary principles. Her work bridges clinical insight and scientific innovation, with interests spanning pediatrics, allergy, Filipinx health disparities, and immune system dynamics.
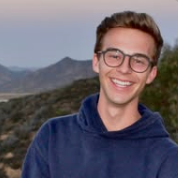
SUMMARY
This presentation showcased how Dr. Shahram Yazdani and his interdisciplinary team at UCLA are using AI and OpenAI’s language models to enhance diagnostic precision and address systemic inequities in healthcare. By leveraging text embeddings and semantic vectorization, their projects aim to reduce diagnostic errors, predict hospitalizations, and personalize care—especially for children with complex medical needs in underserved communities.
TRANSCRIPT
In this session, we'll explore how AI, including OpenAI's GPT-4, is transforming diagnostic care, especially in areas where it's most needed, such as rare and complex diseases and among underserved communities.
This is not a technical deep dive. Instead, we'll take you on a journey onto how Dr. Yazdani's team and lab are using AI to enhance diagnostic accuracy, lighten the workload for health care providers, and promote equitable health care outcomes, among others.
Dr. Shahram Yazdani is a professor of pediatrics at UCLA, where he serves as associate vice chair of clinical affairs at the School of Medicine and medical director of the Children's Health Center. His research interests include computer-assisted diagnosis with a focus on modeling diseases and their symptoms, as well as studying the impact of aviation pollution on childhood health. His current work aims to expand access to high-quality diagnosis for individuals in remote or underserved areas, while also reducing the cognitive load on health care providers.
I want to also emphasize that Dr. Yazdani isn't just a brilliant mind in his field. He's a compassionate leader and has been a true pleasure to collaborate with him producing this event. We will also be visited by his amazing residents that Dr. Yazdani says are actually the ring leaders of these projects we're about to present and surface the most innovative ideas in his lab.
We'll be visited by Dr. Mericien Venson, MD, PhD, one of the only physician scientists in the residency fast-tracked in the Allergy and Immunology Fellowship. We'll also be visited by Sebastian Salazar, who has a master's in biomedical engineering from Johns Hopkins and is a medical student at UCLA Medical School. Finally, we'll also be visited by Tyler Kristensen, MD. He comes from a mathematical background, and his team is very, very grateful for the addition of that skill set in their lab.
Thank you all so much for being here. And without further ado, I'd like to invite Dr. Shahram Yazdani to the stage.
Thank you, Natalie, for that kind introduction. Hope everybody can see my screen. So what we're going to talk a little bit about today is about how we can leverage large language models for disease classification and really what are some of the true applications of these things.
Along with some of the people who were mentioned here, I should mention some of the brilliant people I've had a privilege of working with and continue working with, Dr. Ron Claude Henry from USC, Mr. Isaac Henry, Mr. Avery Byrne, who are data scientists, mathematicians, and just purely brilliant, and Christopher Giza, who's a colleague and a neurologist here at UCLA.
I should point out I have no conflict of interest. All research is done at UCLA and through philanthropy by individuals, namely Kazani families who've donated over years and supported us.
Let's get to it. Why is diagnosis such a critical starting point, and why is it so important? I want you to imagine that if you have a wonderful treatment or a cure, but it's really applied to the wrong disease, it turns out to become very ineffective. So as much as we look for treatments, we really need to start the healing process by making the right diagnosis.
Making the right diagnosis and becoming a skilled diagnostician is not an easy thing. It takes a lot of training, a lot of experience to develop pattern recognition. And just when you think you have the pattern recognition, it actually works against you. It works against you when it comes to identifying outliers, either rare diseases that you've never seen or you've just once read about, common diseases, which are most of the things that come to me.
In fact, for a rare disease to turn out to be a common disease with an uncommon presentation, or just nuanced prevalences of diseases that are really hard to diagnose. And because we're used to, through pattern recognition, to put things into boxes, it's a really hard thing to do to think outside those boxes and say, is this really an uncommon thing or am I just talking myself into it and thinking about something exotic here?
After all that, you need to have adequate time, which has become an increasingly precious commodity. And then you need support. You need humans, other humans, laboratory resources. In academia, we have the privilege of having a lot of people around us where we can just side-curve and ask. We pick from the resident's brain to the expert's brain and say, what do you think? And in fact, we're thinking out loud and trying to figure out what's going on with these people.
On top of all this, unfortunately, there's a decrease in interest in primary care in the United States and many of the Western countries, if not the world. And when you shrink the pool of this primary care applicants, you're actually shrinking the pool of subspecialties. And this is very much palpable in pediatrics, and we're seeing that year over year.
In rural areas, this happens to be a big problem, but also in the developed countries, this continues to be a problem. One of the prime examples and one of the reasons I actually went into this was that worldwide, about 55 million people get affected by rheumatic heart disease. This is a complication of a simple strep pharyngitis, strep throat infection that goes untreated, and about 360,000 lives per year are lost.
In fact, this number's gone up since 2015 based on WHO numbers. And this is for a disease that's easily treatable, and on top of that, it's treatable with penicillin. A large number of people end up getting treated with penicillin for the wrong disease. They have a viral infection, they have a viral pharyngitis to get antibiotics for. But those who truly need it end up not getting it, even though it's available, it's inexpensive, and it's overused. And this is where it really heads home, that having the right diagnosis, whether it's a simple disease or a complicated disease, can make a difference.
On top of all this, cognitive fatigue for physicians is very real. Some of us have the privilege of being in ivory towers, and we have more time, and we have a lot of support and cognitive support, again, from trainees to colleagues. But then that's not always the case. And then misdiagnosis, we all know it leads to suffering. It causes what's called diagnostic odyssey. It takes longer than it should to diagnose someone, and it has individual and societal impact. For example, parasitic infections have been well-known to cause societies to sink into poverty simply because of anemia and lethargy in patients, parents, children, premature death of parents. All these things are simple sufferings that we can be... Today we can prevent those.
Well, why now do we think that we can do this? People have tried this since 1960s with mixed results. Some were really good, but they weren't scalable. But I want you to think about this.
Most of the time when we make a diagnosis, we do this through a patient coming to the office and expressing verbally, but also through body language, as to what their history is, what's been going on. We ask some questions, they give us some answers, and everything's really exchanged through words. Medical terminology is meticulously used in medical literature. We use Latin, we use Greek. If it's a serious disease, we use German. We use French, we use local language to really describe things meticulously, concisely between clinicians and going back and forth in the medical literature.
So it only makes sense that if we could only quantify language, then we could have a chance at computers doing something meaningful.
So what are large language models? And forgive me if I'm oversimplifying this. But basically, these are trained, just like GPT, on a massive amount of text. It could be specialized text or it could be generalized text. And somewhat learn, so to say, on how words relate to each other. When you take that, you quantify that relationship, you come up with what's called vectorization. You're going to hear me say this a lot, where it encapsulates words as vectors, and then we create what's called word embeddings, which have multiple vectors in them.
So if you think of planet Earth being planet words, and on there, all the homes and all the businesses have a GPS coordinate that, in fact, are embeddings. Now you can see the relationship between words, and now you can have an address between these things and know how you go from one to another. This is kind of oversimplification of all this.
When you do this, now you have a chance for computers to process semantic representations into understanding their somewhat of a meaning, and then be able to calculate things and do magical things with mathematics with these things.
So we promised not to go into technicality too much, but in order to not have such a huge leap in logic, I need to kind of present a little bit about the work we've done so far.
What we did, we started with a very simple hypothesis. We said if the word relationships in a language are to be used for diagnostics, we should be able to see that a symptom of a disease is somewhat closer to its own disease than it is to an unrelated disease. In other words, headache, for example, should be closer to migraine than it should be to eczema.
So what did we do? We took five very special diseases, and I'll go over why these diseases are so special. CHARGE syndrome, Cowden, POMS, rheumatic fever, my favorite, and tuberous sclerosis.
We did what's called disease embedding representations. We said you can look at a disease name as a semantic name. You can take the name, and you come up with embeddings for those, and there are programs like OpenAI's Ada for that. Or you can say, look, when I tell somebody I got...
such and such disease, if that person's knowledgeable or a physician or a very skilled nurse, we'll simply say, ah, you mean you have such and such symptoms? So why don't we take all those symptoms, all the organ systems that are affected and things that are so-called symptoms of a disease, put them all into a basket, and in other words, make an alloy out of these metals and create a singular embedding that's an average embedding of the collection of symptoms for each disease. And we call that ensemble mean of this. And that was a technique. And this was a term that was coined by Ron and Dr. Henry. We use OpenAI 80002. There are a lot of specialized ones out there, but we wanted to challenge our hypothesis. We wanted to use a generalized embedding model to say, if it works for this, if we can create a nuanced, very niche, specialized application out of a general embedding, then you can do so much without really having to train all these words into that space. And therefore, it has general application and you can play chemistry with these embeddings that are general and now create specialized applications for these things.
I won't go too much into distances. There were five different distance models we used. There are many other out there, but basically we used Euclidean versus Chebyshev. One looks at overall similarity. The other one looks at the differences. At the end, we ended up using the Euclidean model.
Why are these diseases so important? A, they're complex. They're not common diseases. They're not cough and cold. They have a broad spectrum of organ systems affected. They're not just respiratory or just liver or neurologic. They affect different parts of the body. And therefore, they have a very broad spectrum of symptoms that affect different parts. But this last part is very important. They have major and minor criteria. There are not too many diseases out there that have major and minor criteria. What does that mean? When you say major criteria, each have a higher weight assigned to them. So, for example, if it takes two major criteria to make the diagnosis X, it takes three minor criteria to make the diagnosis X, or if you don't have two of the major symptoms available, you have only one, then you need two minor symptoms in addition to the major symptom to create this thing. And we'll see at the end why this is an important thing. It's extremely nuanced, and it's really challenging to any system that wants to claim itself to be sophisticated enough to make diagnoses.
So they're rare and uncommon. We talked about this. And then we're basically trying to stress this thing not only by making it rare, but also they have funky names. CHAR syndrome. CHAR syndrome is an acronym for all the organ systems affected. But it also sounds like you're charging your battery. Poem syndrome. We were worried about poems get confused with poetry and reading poems. And that was an interesting thing. So we wanted to see how do these things really test out.
The research design is a very simplistic way. We took all the symptoms of all the diseases A to E, and then we lined them up, and we said, what is the distance, the embedding distances here? And if symptom A really belongs to disease B, hypothetically, we should see a shorter distance between symptom 1 and disease B than we see between disease A and symptom 1, or symptom 1 and all the other diseases. And this goes both ways. The ensemble mean, the mean of all the symptoms, and then planemic, which is basically embedding of the semantic name.
What did we find? This is, by the way, like a year and a half of work and then a lot of vectorization modeling over four years. So basically what we found was that symptom to epinemic embedding distances works okay. Most of the times it works. A lot of times it overlaps, and some of the times it's completely off. It doesn't work that simply. And I realized AI works in a much more sophisticated way, but we wanted to really titrate it down to the basic bricks and mortar of this thing and see if it works. It doesn't differentiate between major and minor symptoms for sure. Symptoms ensemble mean embedding did much better, as expected, right? You took all the symptoms and you created the average embedding. So there's gotta be some quantum footprint of those symptoms in that embedding. And therefore you should see symptoms being closer to the pertinent disease than to non-pertinent disease. What we saw was no overlap. It was very consistently accurate. And in fact, if you play with this long enough, make it more sophisticated, you can easily create a really good diagnostic tool out of this, as we're going to do in the future. But there's something else also happened. It consistently differentiated between major and minor symptoms for each disease.
I want you to think about this. So ensemble method really showed that potentially if you encode nuanced, specific information into embeddings, now these embeddings have a much bigger meaning, and then you can do much more specific things with them. You can build traceable, deterministic disease models. These don't give you different answers at different times. They give you a specific distance model where you can now deterministically determine what the disease is. And the disease doesn't have to be a disease. Disease can be characteristics, can be an environmental condition, could be an airplane problem. It could be many things. You've now taken a so-called black box and you turn it into a glass box. In this case, a diagnostic tool, but it doesn't have to be that. But it does one other thing. I want you to step back and think about how do we come up with major and minor criteria? A lot of very experienced people get together because they understand diseases, because they have had experience with them, and because sometimes they've actually been patients themselves and dedicated their life to finding out how do you diagnose and treat these people? They get together into international conferences after one or more years, come up with these major or minor criteria, and the rest of us take it for what it is and it becomes part of the medical literature. In other words, they understand disease. Could it be that when we encode enough information into these embeddings or any other vectorization models, you're now, we know computers don't understand, but we now mimic. We parallel understanding. Now, this is one example. There's a lot to be done to prove it right or wrong, but this was kind of a light bulb moment as to what can we do with this and can we take it to the next place?
So I want to hand this over because from here we can extrapolate to the next thing to test it. And this is where the next project comes in. And I'm going to hand this over to Mr. Salazar.
Hello, everyone. So my name is Sebastian. I'm a medical student working with Dr. Yazdani and the rest of the team. And I'm excited to share with you our work so far on leveraging text embeddings to predict hospitalization needs and chronically ill pediatric patients. This project bridges the intersection of AI and healthcare and expands on the work already presented by Dr. Yazdani and aims to enhance care delivery, especially in this vulnerable population.
So a bit of a background, chronic illness and pediatric patients are often unpredictable leading to frequent hospitalizations, which are often for similar or very similar diseases or repeating problems. And such frequent hospitalizations are a strain to both families, patients and healthcare systems. Much of the patient's story lies in what we call unstructured data versus structured data that involves vitals and lab values. And these unstructured data are typically written in free text notes called history of present illness or HPIs. And so our project aims to harness the power of text embeddings and vectorization to analyze these HPIs and identify patterns that signal a higher risk of hospitalization and allow for physicians to be proactive before an urgent hospitalization is needed.
So with that bit of a background, I will let you, or let me go over the details of the project itself.
So like I said, the population includes pediatric patients with complex chronic conditions and a history of prior hospitalizations. Our method was to de-identify the patient's HPIs from their visit notes and obtain text embeddings to be able to calculate the distances to recognize the problematic organ systems that keep popping up in each visit. From our preliminary analysis, we saw that how these embedding distances over time or over visits were able to reveal patterns that correlated with organ systems that were problematic organ systems that can, with further work, be used to predict future admissions. And then to validate this method, we compared what we were able to calculate for the embedding distances to human annotations that were provided by our two resident physicians.
I'll pass it on to Dr. Venson.
Hi, everyone. I'm Dr. Venson. I'm one of the pediatric residents at UCLA working with Dr. Yazdani. So to give more details on how the human coding of patient histories was performed, it was done by two physicians, me and Dr. Kristensen, first independently. And what we really wanted to do is put ourselves in the mindset of actually seeing each patient in the clinic. And let's say we're given this particular history, what issues would we try and address during that visit? And we identified up to three issues of highest priority. Then for each issue, we coded which organ system or systems best were associated with that issue.
For example, cough corresponded to respiratory, constipation corresponded to gastrointestinal, and fever corresponded to constitutional or general symptoms. At the very end of that, we compared our independent human codings, discussed them, and then decided on a consensus of the issues and systems for each patient visit.
So far, we've compared our human and AI annotations and have been really reassured by the fact that we see that we're on the right track. Our AI model overwhelmingly, greater than 90% of the time, is able to identify the same organ systems of highest priority as our human physicians, or Dr. Kristensen and I.
So I'll take the mic to Tyler to say more about the future directions of this project.
Hi, I'm Dr. Tyler Kristensen. I have also been working with the rest of the team on all of this and the human annotations aspect of comparing the AI model that we've been using to what we as physicians would be seeing on the actual practice side. One of the things that's really interesting and important about this is so far, we've been having a very, very good result with what we've been seeing from the human-compared annotations to the computer-compared annotations.
And in that, we're able to see frequent visits where you might have a patient with chronic illnesses who's coming in for repeated increased respiratory secretions or other sorts of things that as physicians, we'd pick up on on the outpatient clinic time, but these are patients who have many different subspecialty cares with neurology, gastroenterology, pulmonology, other primary care doctors, and then go through many of these visits where these different symptoms might come up multiple times, but with different practitioners who might not have that continuity to be able to continue following each of those complaints.
The thing that's really useful in how our AI system so far is being seen and used is that we're able to see through all of those different visits and all the different documentations, the consistency of bringing up the problem systems and the fact that the annotation on the AI system is able to say there is a concern that we're seeing the pulmonology system come up multiple times over and over between different visits.
The way that we're able to then use that as our physician side is to be able to say we should bring this patient in more frequently for increased pulmonology follow-up, coordination of care with their primary care doctor, or otherwise, depending on what system is coming up the most frequently. And that is able to give us the red flags prior to these patients continuing to develop worsening of these symptoms that they've been having, ultimately leading to hospitalization.
We know that for all of these patients who have complex chronic illnesses, these patients at some point in time will be hospitalized, and that is basically a guarantee for all of them. What this project is aiming to do is be able to implement a system that works on the back end of all of our documentations and the rest of our electronic medical record to be able to see the problem areas that are coming up frequently for these patients, address those issues quickly, give red flag notifications to physicians to encourage the families to come in for repeat scheduled visits, to address those issues before it leads to one of the hospitalizations.
And in that standpoint, it would save significant costs to the healthcare system, decrease burden of the hospitalizations and utilization of high, high cost resources in complex medical care for these patients, and be able to long-term really significantly benefit the health and well-being of these children who have the complex medical conditions that we deal with.
And in saying all of that, I want to make sure in the future as a hospitalist, I will be continuing to take care of these patients when they do end up in the hospital, but I'm hopeful that in using these systems, we're able to mitigate and minimize the number of times that these patients do end up needing to see me in the future, which is a very good thing, but also I'll be missing them when I'm in the hospital, but they'll be getting the best care that they can be getting in the outpatient setting to be able to continue receiving all of the care that they need to prevent those long-term hospitalizations.
And in saying all of that, I want to pass it back on to Dr. Venson again to talk a little bit more about her interest in the AI utilization in the future.
Thank you, Tyler. So my future is a little bit different and I plan to go into subspecialty, but I also see a great way to utilize AI for earlier detection for these kids and specifically earlier detection of children at high risk for severe eczema, food allergies, or drug allergic reactions, thus decreasing the occurrences of dangerous reactions like anaphylaxis that put children's lives at risk every day.
And I see the great potential of utilizing what we know about high risk factors like family history and previous reactions in order to earlier identify children, even before they start to introduce solid foods into their diet as high-risk children that need more personalized care.
And so with that, I'll pass it back to you, Sebastian.
Hello. And then my future career goals is to continue working with primary care patients, potentially both pediatric and adults. And I see the integration of text embeddings and other AI tools with biological data to help us further develop personalized medicine.
And I believe models could help predict how certain drug interactions with patients' unique protein and genetic makeup can help us optimize personalized medical recommendations.
And with that, I'll pass it back to Dr. Yazdani.
Thank you. Now you see why I'm so excited about working with this group. So I just wanted to wrap this up a little bit. We've had technology come into medicine with a promise of really making life easier and more fun for physicians and better for patients. And some of that was successful. Some of that was not. EMR is one of those things that we were not part of and came into our life. And at best, I would say it's got mixed results.
There are things that it does much better, allows us to do much better. And there are things that actually consumes a lot of time. I do think the future of AI in diagnosis should be really, we should think about how we incorporate this seamlessly into workflow so we don't have more clicking, more looking at the screen rather than looking at the patient.
And I see AI scribes as being one of those that really incorporates diagnostic tools into AI scribing. And it's really exciting. I think it'll basically merge some of the benefits of EMR into the benefits of AI and ambient voice recognition.
I do think there's a lot of work to be done in visual recognition. We do gather a lot of information from visuals, what are looking at a patient's posture. So there are hybrid vectorization models that I think we are interested and we're working on. And it has applications in retinal pathology, which was brought up previously, radiology and oral pathology in the future.
But the next level, I think, may be that now that we have AI, that we can digest and take unstructured data and make it more structured to a point that it's really digestible by a physician.
We can now bring in a lot of sensors that already exist for whether it's diabetics or those who have a predisposition to neurologic disorders, from Parkinson's to other things, to sleep disorders, balanced posture, activity level, vital signs, and compare patients to themselves and look at their natural progression.
I think that's something that those who have the privilege of having a doctor with them all the time have, those of us who monitor ourselves and maybe to the point of hypochondria, they do this a lot to ourselves as physicians. But having that in a better way for patients to come to the doctor, that would be a good thing to have.
And those are just some of the simple ones. I think some of the challenges we're going to see is training physicians in the future with systems that are really intuitive and they're easy to use, and really making AI not as a tool of taking cognition away, but with every case as a reminder of some of the things you should think about.
You're really getting educated. You're keeping up to date by simply seeing a patient. When a patient knows that their physician, by having a cognitive assistant, is always going to stay up to date, is not going to miss anything, and whether they're tired or cranky or sleep deprived or any of those things, there is a little bit of a driver's assist there that'll help them.
We're not taking away from the joy of medicine. I think we're going to add on to it and make it much more interesting and then learn more and more by having reminders of cognitive assistance.
I also think we're going to allow physicians to work, and nurse practitioners, and pharmacists, and NPs, and those who triage patients to work at the top of their license and be able to act as if they have at the tip of their finger a consultant who can help them out and say, no, don't overthink it, or you didn't think about this, maybe you should think about it or ask these questions.
Electronic health record fragmentation is a big problem. I think there was a great paper out of McGill by Dr. Cohen and Tobiano. It talked about how much fragmentation we have, how much we can do work if we really have a Rosetta Stone that binds all these things and really decrease the effort of incorporating this data in a way that physicians can incorporate in the EHR and work, again, with less clicking and more of the face-to-face contact with patients.
So we spend our time counseling patients, holding their hands and letting them know it's going to be okay, we'll be in this together. That's what humans do best, let's dedicate the little time we have with patients towards those things.
I'm going to stop there, I don't think there's much to say. You know, we're all available if you want to contact us. This is our email, it's going to be there. Everyone consented to this. I'm certainly available for any collaboration, I would love to work with other people on how we can shape the future.
Thank you so much, Dr. Yazdani. Thank you to Dr. Tyler Kristensen, Dr. Mericien Venson.
and Dr. Sebastian Salazar. We'll see you all in a few minutes in the live Q&A.
And for anybody in the audience that's a full member of the OpenAI Forum, you can also send them a DM directly from the OpenAI Forum. You go to the people tab and then you can message them directly.
I wanna thank the audience so much for being present for this very special presentation today. If you're a guest in the forum and would like to be a full member of our community, please ensure that your profile is complete and we'll be in touch.
If you're a member of the forum, you can join the link in the notification you see now, which will direct you to a live Q&A with our guests. Keep an eye on your inbox. Tomorrow, we're gonna be sending registration links for all of the June events, including the Future of Work with Karin Kimbrough, the Chief Economist from LinkedIn, and Ronnie Chatterji, Chief Economist from OpenAI. It'll be premiering June 4th as a presentation with a live Q&A at the end. I'll see you all soon, whether in Singapore in real life in two weeks or in the live Q&A in a few seconds.
So Galadriel Bonnell, she's a nurse practitioner and medical writer. She asks, would word embeddings be similar to MeSH terms from a traditional literature search point of view?
Not sure who wants to take that one on, but Mericien, you can keep yourself unmuted and you guys can just jump in to answer that.
Do you want to talk about the... I mean, I believe MeSH terms are actually just word findings.
Yeah, if I understand the question correctly, so like MeSH terms would be like key word phrases that would be sort of like associated with disease processes or diseases. The text embeddings would be essentially the numerical representation of such MeSH terms with depending on how extensive we want to define the MeSH term as well with that definition of that MeSH term because the MeSH term itself can have a text embedding that could be a certain number, but then the definition of that MeSH term will have a different text embedding that could be more representative and capture more detail from an HPI.
You know, one of the advantages of embedding is that because you can actually set up a threshold for distances, it's much more adaptable, and it's not so unforgiving as word matching things at Asteroid. So you can set your threshold, and based on that, you can do a lot more than what we could do before.
All right, thank you so much, team. We don't have many hands raised. I think that we have a little bit of a shy crowd. It's also early afternoon, so I'll just keep going through the questions from the chat, and if anybody feels brave and wants to raise their hand, I encourage you to do so.
So Kareem Gutierrez from Long Run Entertainment Group asks, given that patients may not always provide accurate information about their health concerns, how will you address false data that could skew the model's analysis and lead to an incorrect direction?
That's a good question.
Yeah, this is something that we actually deal with quite frequently on the inpatient and outpatient side. It's always weighing the utility and the history that we take from patients. And one of the things that I think is important to kind of clarify on how this project works right now is the documents that it is reviewing is the documentation provided by the physician who is seeing the patient. So it is their typed out responses at this time. In the future, probably using some sort of audio transcription, as well as some people do use those audio transcription files at this time, but the important aspect of that is that it is taking all of the information that the doctor is subjectively kind of inputting into those notes at this time, and it is important that their history taking still leads to the questions that are important for the kind of overall view of what the patient is coming in for.
And so we will have patients who will say, I have a little bit of a fever, and we will clarify a little bit more. What temperature has that fever been at home? Because a fever may be something that somebody says, I feel warm, but it's not actually a true fever, but when we clarify that a little bit more with the details and the history taking, that's where we're able to actually get the true data that we do put into our notes.
I just wanna add on. I don't know, sorry. Oh, hi, Karime, you're here live. You're welcome to...
Yeah.
Yeah, I was just like a follow-up question about that. So you compare the data about you receiving from the pediatrician, I think, or doctor, and then compare with your actual data, and then you tell if the data that the patient are telling to you is correct?
At the moment, no. So what we do is, and maybe I can talk a little bit more about how they teach us this part of history gathering in medical school. Patients are free to tell us whatever they want, but as physicians and as residents, they have more experience with this, but as physicians, the nuance that we add to the notes, as their physician notes, is to categorize or to elaborate more on whether or not certain aspects of their history don't make sense to us, or if we think there's missing data.
Thank you so much.
No, don't do this.
Oh, sorry, sorry.
Thank you. Thank you, guys. Hardy, Kamin, would you like to unmute yourself and join the stage?
Okay. Welcome, Hardik.
Hi, can you hear me?
Yes, we can hear you.
Oh, sorry. So I tried to put the question in the chat and it automatically got removed for whatever reasons. I don't know, but I'll ask it here. I was curious to know about this study. Does this go beyond just note-taking in the sense that would you capture voice data or facial expressions or some sort of other multi-modal data inputs to kind of do the diagnosis?
I'll answer it. Go ahead.
As of now, we don't. It's true that we do gather a lot of information by just looking at a patient. Mary-Jane, do you want it to talk about?
I mean, right now, as Sebastian had said in the presentation, we just use the history of present illness. So one part of the note that we write during the visit, but there's also a physical exam portion of each visit note. And we've talked about in the future next steps kind of harnessing that information. And I feel like that can capture what you're getting at in terms of a patient's body language, what they look like. Do they look like they're in distress? Do they look like they're breathing comfortably? Like that would be noted in the physical exam. And I think that is a great next step to using AI and tapping into that information.
Thank you so much for your question. I really love that. Susan Gundos, would you like to unmute yourself and join us?
My name is Susan Gundos and I'm a pediatrician in private practice. And this project that you are all designing is for an outpatient clinic in a hospital setting. Is that correct?
Correct.
Okay. It will be wonderful if we can start a new project. Obviously it's a lot of work, but for the outpatient pediatric practice because you see we're being rushed constantly to get through the patients. And I devised handouts on my website as well as on paper with information so they don't have to run off to the emergency room due to fear or what they can do for the temperature or everything. And I would love to start a project working with pediatricians in practice because I feel that patients are being shortchanged. They're being pushed out quickly and there's so much information they need for asthma, etc.
Thank you, Susan. Thank you. I think we all share that sentiment, unfortunately. Part of building into the EHR infrastructure and freeing up the position time is really to do what you just mentioned. And I think that's important to do. Tapping into EHR is not an easy thing to do. And I think a lot of these AI scribers are actually trying to tap into that. I'm hoping that we can piggyback along with them because they do all the work and then we really create the infrastructure to better support and provide customized information for the patients.
Anything in your experiences that you guys think we should add on or?
Yeah, it's one of the complexities that we're currently dealing with. It is extremely hard to fit a discussion of all of the potential things that you could do for anything that could come up after a visit for a well child check, let's say. When a child is coming in for, they're doing fine, but two weeks down the line, they get a cough, congestion, fever, what do I do? We don't have time always to be able to see every single person at every single visit for every single problem. It's just not feasible. And so being able to find ways that we're able to incorporate data that can talk about, let's say, the things to do in these cases.
and be able to have easy access to those, I know at least from our clinic that we work in, we're able to give handouts to patients at every visit to talk about the basics of what may come up and what to see, and that can be included in wild child checks.
From a utilization of AI perspective, it's also interesting to see how we've had a lot of patients who may end up in the emergency rooms or urgent cares who have actually used AIs to talk a little bit about symptoms, things that have come up, what's common things that I should be thinking about, or what's something that actually could be useful in my situation, or is this something I really need to be seen urgently for?
I just wanted to add what I did in my office is because developmental delay is a big issue that's being ignored, early intervention takes up to three to nine months to get in, and so I produced an interactive neurodevelopmental assessment that the child does in front of me, and I have the parent watch, so instead of doing a ASQ or a PEDS, which I felt the parent may not truly understand or be afraid to admit to a speech delay, I have an interactive assessment, I pick up immediately what the problem is within 15 minutes, and then I'm able to send the patient for whatever services, if they have Medicaid at disadvantage, I have a video and teach them, and I'm trying to put this on a digital platform because it works really great.
I made big strides with second English language learners, especially who are thrown into special education immediately, I find, because their parents don't really know. So I just want to let you know what I'm doing, what I'm working on. Thank you.
Thank you so much, Susan. I have so much love for nurse practitioners too. I'm sure the team here also does. We love your ideas, and please stay in touch, and if we ever have a need for expertise, you know, to collaborate at OpenAI, we would love to have you share your ideas with us. Thank you for being here today.
Thank you so much. I'm gonna pull a couple of questions from the chat team. How well do the embeddings capture diagnostic nuance when symptoms overlap across multiple diseases? Mericien, you were quick to unmute yourself on that one. Do you have ideas? Sorry, did I unmute?
I think Dr. Izzani is the best to answer this question. Yeah, that part of the study. So what we found, and if you look at a paper, we actually have graphic models of this thing. What we found was when you go to the ensemble mean, the overlap almost disappears. So same symptom for different diseases. They seem to do very well. It's very interesting when you do this average or mean of embeddings. It really almost feels like chemistry, and I come from chemistry background, so everything seems like chemistry to me. But I have to say it's almost magic. It seems to really discern itself very nicely and be able to pertain to the pertinent disease very nicely. So it does work. It does work well.
Now if you make the context of these things larger and larger, embeddings get diluted if you want to get a technicality of these things. There are 1,532 vectors, so at some point when you go above those number of vectors, you're bound to dilute yourself and lose some context and meaning. Thank you, Dr. Yazdani. That was a wonderful question.
We'll go back to the audience, the folks that have their hands raised. We have a medical student, an anthropologist, Arinze Okemuo. Arinze, if you'd like to unmute yourself and join us, and please tell me how to pronounce your name correctly. I did my best, but I'd love to hear it from you.
Hi, so my name's Arinze Okemuo. I'm a fourth-year medical student at UCL in London, and basically I found it quite interesting because during the presentation you mentioned like diagnostic and prognostics, essentially dreaming, highlighting major and minor criteria. But as a med student, I find it hard to envision that because the stage I'm at, I don't really have access to the data to be able to process and streamline these processes.
So then the other thing you said was obviously the Scribe AI, and I find it interesting because at the GP practice I am on placement at right now, they've just actually started using a Scribe AI, but then I almost wonder why isn't the adoption higher and how I can almost jump in and maybe accelerate that adoption? Do you guys have any thoughts on that?
I think from my understanding of the healthcare system here versus the rest of the world, each electronic health record system here is like independent from one hospital system to another. So I think that accessing the, or having AI Scribes in one hospital system would be useful for like, for example, here at UCLA for UCLA physicians and for those partner institutions that have access to our electronic health records.
But if I were to go to the East Coast, somewhere like in New York, they may not have the same electronic health record system, and they will, if they do have an AI Scribe, it would definitely be probably a different party that may not have like licensing agreements with each other. So that's kind of like one thing that I envision being a limit in having this kind of be adopted very quickly. And then also in general, like general hesitancy from private offices of like not wanting to share or be held liable for sharing private health information, at least in your second part of your question. I think that's, those are the thoughts that I have. Yeah.
Remember medicine is very much tradition-based. We're not really doing things that differently than the Greeks. We go to the patient, we like to feel like a hero. We save the patient, this and that. So tradition does play a lot. You know, it takes time to build momentum. I think your generation of doctors will be very different than my generation of doctors. I do see some doctors adopting these things, but I want to share very interesting data from UCLA itself.
Of all those who signed up and volunteered to use AI Scribe, 25% never touched it. These were volunteers. They said, I want to do this. And yet they didn't do it. Why? Because they had to go through the training, which is ironic because AI is so adaptable. It's almost scary how much it knows what I'm asking better than when I can put it to words. And yet there was resilience towards that.
So the more we make these things intuitive, the better we make it in terms of workflow. And the more this next generation grows up going through residency and realizing I shouldn't spend my time memorizing things. I should spend my time kind of deciphering things, learning the human skills, bedside manners, rather than memorizing a bunch of facts and spitting them out, which some days I'm better at it, some days I'm not. Then that culture will change, will build momentum. And people say, oh yeah, how did they practice before all this? They had to click, click, click.
Just like we look back and say, people still put things on paper. I understand a lot of people do and people like it. But as somebody who has horrible handwriting, I look back and I say, I can't believe they forced me to do this. So now I think that's going to, it's going to be very different. I don't even want to predict how many years from now, but it's not going to be that long. Future comes faster than you think.
Well, Arinze, it sounds like your compass is pointed in the direction. And so perhaps keep in touch with the doctors here presenting. I would love to, yeah. You're going to eventually need to do a residency somewhere. You guys have access to each other now. And Arinze, you do have access in the forum as well, like across disciplines. And we're all really discussing how to lower the barrier to entry because in all of the legacy practitioner spaces, I think this is a challenge for all of us, but I'm really grateful that you're a part of the community and I hope you keep in touch with the doctors that are here today. And we can be here to uplift you and help you be a pioneer in the space like the team here today. So good to hear from you.
I think we have time for one more question. Let's, how about Lizzie Shu? Lizzie, if you're here, you can unmute yourself. Your hand's been up for a long time and I'm so sorry I couldn't get to everybody. If we didn't get to your question today, please add it to the chat now and I'll make sure that everybody here has access to it when they have time. Hopefully they can address your questions.
Hi, Lizzie. Welcome.
Hi, Natalie. Thanks, Caitlin, again. Professor Shahram, I have a question for you. So I've just finished my graduate training at a graduate degree from Harvard and I finished my medical training at Stanford in neuroscience in a very basic science research. So I see that you have a lot of clinical experiences, which is amazing. My question is, how do you think AI can help bridging the gaps or help facilitating in a better way between the clinical side of healthcare and the basic science side of healthcare? Because before, I think we're talking about the clinical trials or the healthcare with patients. But if we step backwards, how do you think that AI can help in this space? Because I haven't seen a lot yet in my limited view.
Thank you.
That's an amazing question. Lizzie, maybe you should be the pioneer in all this because I think you have the background in a lot of these things.
You know, everybody has probably their own ideas, but if I hear you correctly, we're really talking about translational work. And translational work where you can take really knowledge and translate it to bedside, it takes a tremendous amount of knowledge and experience. And one of the things I'm finding out is that AI seems to kind of bring it all to you rather than you spend so much time, especially with the newer models, searching for things, putting them into context, putting them into words, now publishing it, testing it. What we're seeing is that what things that used to take weeks to do, I do it in an afternoon. It's great because it gets so much and I can break it, I can test it, I can re-hypothesize. So I think translational research is probably prime for bringing those together. I am hoping that, especially for things like allergy immunology, which are so close to the bedside and so intertwined into bedside, do that. Drug repurposing, another area that I think is absolutely prime for taking what we know instead of just inventing yet another molecule, think about all the things we can do with those and then based on that, create those. And I think that titrating of knowledge and bringing it to the scientists or the clinician, it really means you have an army of people on your side which are tireless, they never complain, and they're not that expensive. It really takes a lot of clever minds like yourself to really think about how to do this, but I think it's doable.
We do need to bring people together, and I think one of these forums, that's the whole purpose of these forums. I would love to hear more of your ideas, and I'm not short on ideas, and I want to hear some of the younger people, what are some of their thoughts? I'm working on it, the next startup after graduation in May, sorry, Natalie, every time I call it's in the restaurant in San Francisco.
Don't be sorry, it's so good to see you, and please keep in touch. Shahram, Sebastian, Tyler, Mericien, I feel so inspired. Thank you so much.
Shahram, we're here because of you, you came to one of our events, and then you told me about your amazing residence, and you have this beautiful idea. You guys are absolutely innovators and pioneers in this space, thank you for uplifting the voices of your residents, they definitely belong here, and there's a place for you guys. Thank you so much for showing up, and just illuminating your work for us, because you are pioneers in this space, and there aren't a lot of public-facing opportunities for us to hear what you're working on, and these use cases, so please keep in touch. You have very intimate access to Caitlin and I now, the OpenAI Forum, we want to continue to lift up your stories, and continue to support your work, the work you're doing is so important.
And honestly, it re-inspires me, and gives me more faith in our healthcare system, because it can be a healthy, it can be a scary system to wonder if the people behind the scenes doing the research, and the physicians, you know, how much you guys care about the patients, and to hear from you today just re-instills my faith that there is a lot of love there. So thank you so much, Dr. Yalzani, for bringing your lab here today, you guys are amazing, this was really beautiful. Our community is your community, don't hesitate to reach out, I'm gonna make sure that you know how to access the chat, because we did not get to get to all the questions, I know you're all incredibly busy, but if you have time, maybe you can address them later. And please don't be a stranger, it was truly, truly an honor to uplift your work today. Thank you so much.
Thank you for having us.
Thank you.
Thank you, Natalie, and the rest of OpenAI.