Enabling a Data Driven Workforce
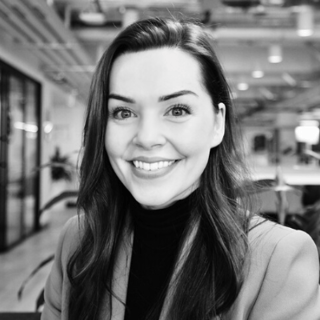
Lois is a Customer Success Manager at OpenAI, specializing in user education and AI adoption. With over 10 years of experience in SaaS, she has extensive experience in developing and delivering engaging content, from large-scale webinars to stage presentations, aimed at enhancing user understanding and adoption of new technologies. Lois works closely with customers to ensure ChatGPT is integrated into daily activities and effectively utilized in the workplace. Lois is known for her storytelling approach, making complex technology relatable and accessible to all audiences.
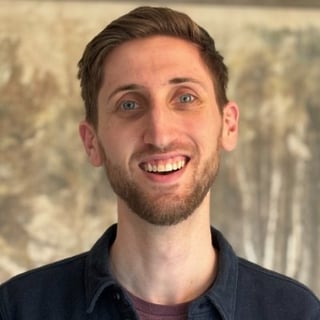
Aaron Wilkowitz is a Sales Engineer at OpenAI focused on the US Federal Government and other Public Sector accounts.
The webinar, part of the ongoing ChatGPT Enterprise Learning Lab series, featured Ben Kinsella, a member of OpenAI’s Human Data Team, alongside Lois Newman, Customer Success Manager, and Aaron Wilkowitz, Solutions Engineer. They explored how ChatGPT Enterprise can empower organizations by streamlining data analysis, enhancing productivity, and fostering a data-driven culture.
Key Takeaways:
- Data Security & Privacy: Lois highlighted the robust data privacy and compliance measures of ChatGPT Enterprise, emphasizing that user data is not used to train models and is fully controlled by the organization.
- Integration with Data Infrastructure: The session outlined how ChatGPT Enterprise can seamlessly integrate with existing tech stacks, providing employees with easy access to powerful AI tools.
- Demos and Practical Applications: Aaron demonstrated how ChatGPT Enterprise helps teams prepare, analyze, and visualize data, showcasing examples from anomaly detection to complex forecasting.
AI-Powered Data Analysis:
- Enhanced Accessibility: ChatGPT Enterprise makes it easier for non-technical employees to run analyses, freeing data scientists to focus on more complex tasks.
- End-to-End Demos: The session included live demos showing how users can prepare data, generate visual insights, and integrate results directly with tools like Jira and Outlook using GPT Actions.
Q&A Highlights: Elan Weiner, Solutions Engineer, joined for a live Q&A, answering questions about integrating ChatGPT into organizational workflows and data security concerns.
Hey everyone, welcome to tonight's OpenAI Forum event. Many of you know me, my name is Ben Kinsella, a member of the human data team, and also your OpenAI Forum community ambassador.
So before we get started, I want to let you know that of course this event is being recorded. So we're going to publish this after the event, we try our best to do it as soon as possible.
So if you have to bounce and leave a little early, although we will be very sad, but rest assured you can find this and all the other content in our content library. So we always record these events.
If this is your first event, welcome. I see a few names, maybe I'll call out one of the smartest grad students at Columbia, I know, Jui Chen. And if it's not your first event, I see a few familiar faces, Kevin, Justin. Welcome back.
As you all know, we always like to start our talks by reminding us all of OpenAI's mission, which is to ensure that artificial general intelligence, by which we mean highly autonomous systems that outperform humans and most economically valuable work, benefits all of humanity.
I'm going to be your host today for this really exciting event, which by the way, is our fourth session in our ongoing series of chat GPT enterprise learning lab series. So if you haven't seen the others, which I'll point to later, you can find those in the content library under the OpenAI, one of the content there for the learning resources.
So before we begin, I want to call out two very important things about this event. The first is you probably know this event that you're seeing is pre-recorded. Lois and her colleagues recorded this earlier. So this is a streamed event, but rest assured.
The second thing Lois and her colleagues are actually behind the scenes right now, probably commenting in the Q&A session or in the comments. And so after the event plays, we're all going to navigate to the Q&A link and we all, each of us gets face-to-face time with Lois and her colleague, Elon.
So think about what questions you want to ask, drop them in the Q&A tab. My amazing colleague, Caitlin is going to collect those questions. And at the end, we're going to have a phenomenal conversation and all things chat GPT enterprise and data analysis.
So I'm going to be speaking a little bit, I have a spiel, so hang on for a few comments and then I'm going to begin the recording.
So tonight's events, the speakers are going to be diving deep into all things chat GPT, specifically data analysis and AI. By the way, one of my favorite topics. So hopefully you can realize that I'm very jazzed about this.
So let me quickly introduce Lois and Aaron, who are going to present the talk. While Aaron is not going to be with us for the Q&A, Elon, his colleague is going to be joining us for that.
Lois is a customer success manager at OpenAI, and she is a real expert when it comes to making AI accessible. It's very possible, most likely, that you have seen her on other talks in the forum. We have a one-on-one series, a one-on-two, I don't think we call it a one-on-three, but we have something of that nature.
So a few different installments of the chat GPT learning series. And you know, if you've seen these, that she has truly mastered the art of making really complex technology simple, which is not easy. In this recording, she is joined by her colleague, Aaron Wilkiewicz. Aaron is a sales engineer and his work focuses on bringing AI technologies to organizations, specifically public sector and the government. He has so much technical experience as a sales engineer, and he just knows how to articulate how AI can be utilized at the organizational level at different types of organizations.
This presentation is phenomenal. And in the Q&A session, although Aaron can't make it to the Q&A, his colleague Elon will be joining us. Elon is also a solutions engineer with a background in data engineering and business intelligence. So in his past roles that he's been from looker to astronomer, I would say he is the OG at helping clients unlock the power of AI to transform their data process. I know I'm going to learn a lot from this talk in the Q&A. And so Elon, as I mentioned, will not be in the recording, but is here to support some fantastic technical questions that you probably have at the end.
Here's what to expect. First, you probably have questions about data security and privacy. Lois and Aaron want to give you the ins and outs to give you confidence that your data is protected by chat GPT enterprise.
You also may be asking yourselves, how can we integrate chat GPT enterprise into your data stack? So the goal here is for your workflow to integrate seamlessly with your existing tech stack.
The last thing you may want to know is, can you show me some live demos? Absolutely. Lois and her colleague Aaron have put together a fantastic showcase of how to gather, prepare, analyze, and even visualize data to create some stunning insights and to get insights quickly and effectively.
So I am giving you an exhaustive review of what to expect in this. I'm very excited. So without further ado, let's go ahead and dive into this event. I will see you all very soon after the recording. See you soon.
Okay, perfect. I am going to kick off.
Hi, everyone. Welcome to today's webinar, enabling a data driven workforce. My name is Lois and I am one of your hosts today. I'm a customer success manager at OpenAI and I'm very excited to be joined by my colleague Aaron, who is a solutions engineer. I will let Aaron introduce himself formally in a minute.
But today we have partnered on this webinar to design a session that goes deep on chat GPT enterprise's data analysis capabilities. Our goal for today is that you leave with an understanding of how chat GPT enterprise enables every employee to be self-reliant in running their own analysis.
How OpenAI secures your company's data. And of course, some tangible examples on how your teams can use chat GPT enterprise to gather and prepare data, run analyses and create visualizations.
We strongly believe that using AI in this way will help organizations build a data driven culture, informing decision making and freeing up your people to focus on strategy rather than process.
A little bit of housekeeping from me this morning, this session is live. So please bear with us if we run into any challenges with our friend chat GPT. Aaron and I are joined by several of our colleagues who will be moderating and answering your questions from the Q&A feature, which you can find directly at the bottom of your screen.
Finally, as always, we will send you a recording afterwards and you are welcome to share this recording with others at your organization.
I'm going to now cover how chat GPT enterprise can enable your workforce with secure AI. And after that, Aaron is going to walk you through the analytics stack and an end to end demo.
Let's kick off with data analysis.
So data analysis is a capability available in our chat GPT enterprise product. I know we have some folks in the room today who are still learning about chat GPT enterprise, so it's really important that I start with some quick context.
Chat GPT enterprise is a secure version of chat GPT with access to the latest models and capabilities from OpenAI. But, we've built this especially for enterprises. With chat GPT enterprise, you are giving every employee their own super assistant, helping them to work more efficiently and effectively.
We often see in customer impact surveys that employees also feel more creative in their work on top of saving time on everyday tasks. Chat GPT can help with a variety of tasks from synthesizing large amounts of information, writing content generation, creating images and browsing the web. Also great for coding too.
And we know from speaking to many organizations that figuring out an AI strategy is difficult and it does take time. That's why the Open AI account team partners with enterprise customers to help identify their AI strategy and high-value use cases to support workforce enablement.
We've worked with hundreds of enterprises since we made chat GPT enterprise about a year ago. Since we are talking about data today, it is very important that I cover our security, data privacy and compliance practices.
From day one, chat GPT enterprise infrastructure was built to meet security and compliance industry standards. With chat GPT enterprise, you own and control your data. Three really important messages from me today.
One, we never train our models on your data. You have complete ownership over your inputs and outputs. And finally, you control how long the data is retained, providing you with full autonomy over your information.
Secondly, you decide who has access to your data. We support you managing access permissions effectively and securely within your organization.
Lastly, chat GPT enterprise has been audited internally by compliance and security teams and externally by third party auditors. In addition, chat GPT enterprise helps support customers compliance programs with applicable data privacy regulations.
These measures truly underscore our commitment to maintaining the highest standards of security and privacy, ensuring that your enterprise data is always protected.
Before we dive into today's demo and take a look at data analysis, I want to start with why we actually built this functionality. We hear from business leaders that building a data driven organization is table stakes. But in reality, this is very challenging.
First, working with business data is often complex. Data analysis can be time-consuming and the average employee needs support from data professionals. Analysts and data scientists are some of the busiest people in an organization.
So they can't help everyone. Secondly, even for data professionals, the majority of their time is spent on the process of data analysis. These are some of the most valuable people in your organization spending time on data hygiene tasks.
And finally, for employees who do get access to data and manage to get it into a spreadsheet, there's a lot of noise and they have to comb through this before getting to the answers they need to inform their work. Informing strategy and work with data is ideal. But obviously the process of getting there is cumbersome, time-consuming and inaccessible for many employees.
But GPT Enterprise removes some of the bottlenecks I just mentioned. It enables everyone to run an end to end analysis alongside their own AI thought partner. It analyzes data, surfaces takeaways and creates charts. This helps the average employee to be more self-reliant, which in turn frees up your data team to focus on more complex problems.
In addition to saving time on those hygiene tasks, like normalizing data sets and generating Python code. By combining its reasoning skills with analysis capabilities, Chat GPT can proactively pick up insights, suggest questions to go deeper and answer specific questions about a data set.
To help support this kind of collaboration, our research and product...
Post-trained the model on specific data analysis use cases and built a more intuitive UI that helps users to iterate on outputs and ask follow-up questions in the context of their data set. This really enables teams with Chat GPT Enterprise and will help to unblock data-driven decision-making, but also make the process more efficient.
At a high level, Chat GPT Enterprise can be used for numerous data analysis use cases across industries and departments. For marketing and sales, it can be really great for campaign analysis and lead scoring. For finance and accounting teams, they can use Chat GPT Enterprise for more complex analyses where spreadsheets could be limited. By explaining what is needed in plain English, Chat GPT can write Python code, which is much more efficient in processing CSV files. For analytics and operations teams, it can help with internal business reviews, forecasting, and predictive analysis.
I'm now going to hand over to Aaron, who will take you through some practical examples of how you and your teams can use Chat GPT Enterprise for data analysis.
Aaron, over to you.
Thank you, Lois. I appreciate it. Hey, everybody. My name is Aaron Wilkiewicz. I'm a solutions engineer at OpenAI, and I've spent the last 10 years focused on how to help customers really get the most out of their data analysis and data science workflows. Today, I want to focus on how Chat GPT Enterprise can be a part of that solution.
Before we dive into where Chat GPT Enterprise can help, I want to take a little bit of a step back and provide an oversimplified version of the typical data analytics stack that someone in your organization might see. First and foremost is gathering the inputs and data required for data analysis or data science. Typically, that involves bringing a number of different data warehouses and third-party SAS and API applications together to bring that all in a centralized place.
Once that data has been centralized, there's typically a process of data preparation. That can be around data hygiene, removing and detecting anomalies, and overall, just getting the data in a state that's ready for this particular analysis or data science exercise that's required.
Third is the really fun part. This is where data scientists and data analysts can ask and answer all the questions that they're interested in and can start to get to the kind of insights that actually drive value for their organizations.
The third and perhaps most importantly is making those insights actionable. It's delivering those outputs to different parts of your organization so that individuals can actually take action based on the insights that have been seen.
In terms of where Chat GPT Enterprise can help, there's really two key features that we're going to go over in more detail today. GPT actions and data analysis.
GPT actions enable users to be able to connect to third-party applications to get more out of their Chat GPT experience. Chat GPT uses OpenAI's powerful large language model capabilities to take natural language questions from users and convert that into the API input that's required for a third-party API call, send that input to the API, execute the API, and then return back the results. That includes OAuth authentication or API key authentication. So it's done in a secure way but still allows for Chat GPT to connect to other systems. That's critical for inputs in terms of getting data warehouses and information from those systems into Chat GPT and critical for outputs, taking action in those systems and connecting to things like JIRA or Outlook or anything else that you would want to send your insights to.
The second feature where we'll spend the bulk of our time today is around this data analysis capability. We've seen large language models have the ability to probabilistically generate code, whether it be in Python or SQL or really just about any other coding language. What we see with data analysis is not just generating the code but then executing the code inside of Chat GPT's IDE to enable users to be able to actually run data analysis exercises, generate visualizations, and provide the necessary tools to answer their questions.
In today's demo, there's a number of different steps we're going to go through, and we're actually going to go a little bit out of order. We're going to start with a little bit of a crawl, walk, run approach where we're going to start with a CSV of data and start right at that second step of preparing data.
So we'll start with an existing CSV, prepare some of the data, describe it, run some anomaly detections. We're then going to do some relatively simple data analysis exercises and then get to increasingly complex exercises along the way. Then we're going to switch over to delivering those outputs via actions.
And then at the very end, we're going to go back to the beginning and show how some of the data administrative teams and data analysts can actually pull data directly from a SQL data warehouse.
Before we dive into a lot of the fun of this demonstration, I do want to offer three really important and sobering limitations on the ChatGPT Enterprise product.
The very first one is actually not specific to ChatGPT or any large language model, but is true of any data science or data analysis exercise, which is that governance across your data definitions is key.
Let's take a simple example where maybe somebody on your team wants to understand what's the profit margin in your organization last year. Well, even just in that one simple question that you might ask in a large language model, there's so many ways to interpret it. How do you define profit margin? What columns are you using? What's the way that you define sales or cost or profit? Those things could be totally different. Even last year could be trailing 52 weeks. It could be the last calendar year. There's a lot of different ways to think through that question. And so it's really important that your organization is aligned on what a certain definition means and that you can feed that level of metadata and that specificity of metadata to the ChatGPT Enterprise product. Otherwise, you may get to a wrong answer.
The second limitation we want to be explicit about is the existence of hallucinations. This is also not specific to ChatGPT. It occurs in any large language model because fundamentally these LLMs are probabilistically predicting the next token rather than how typical code systems work where you're deterministically determining the outcome.
As a result, any of these large language models have the risk of hallucinations. So even if ChatGPT is using the correct definition of profit margin or whatever other KPI you have, there's still a risk that it writes incorrect Python code. And it's important to have someone from your organization who understands the data and who understands Python to be able to validate the results before you do anything in production.
The third and final limitation is specific to ChatGPT Enterprise, which is that as a SaaS tool, it requires a human in the loop to log into ChatGPT and actually click off prompts and workflows.
So you could use OpenAI's APIs to make a fully programmatic solution for data analytics and data science workflows and use cases. This particular product is not meant for that. It's meant to have a human in the loop that actually kicks something off manually to answer and ask any of their questions. In some ways, this is a good safeguard, though, because of the first two limitations that we called out.
With that in mind, we want to go through the actual data set that we're going to use across this demo.
We know that the audience here involves a lot of different lines of business, a lot of different verticals, and different kinds of companies. And so we've purposely picked a data set that is relatively universal.
For anyone who's been on a flight, you can imagine what a flight transaction data set might look like that has a list of flights, the month and date of their departure, potential delays that they experienced, information on the origin and departure airports, as well as the flight numbers and the carrier of the airline as well.
Our hope is that by making a data set that is universal and specifically not tied to any line of business or vertical, that it will be an experience that everyone can gravitate to.
I encourage you to think about how this kind of problems that we're going to solve or the kinds of questions we're going to ask and answer, how those could be applied to your specific line of business and your specific vertical.
With that, we're going to dive in and start this exercise. Right now, I'm in the chat GPT enterprise workflow. For many users who have used our free, our Plus, our team accounts, the UX is extremely familiar, right, where I have this window to ask and answer all sorts of questions.
The key difference is that this enterprise product comes with a lot of enterprise-grade features around security and also has significantly higher rate limits than those other tiers.
But the UX experience generally is meant to be familiar for anyone who has used chat GPT in the past. As a reminder, everything that you're seeing here might differ slightly from the screen that you might have in your chat GPT instance, either because you do not have an enterprise account yet or because there are specific things in this demo environment that may not exist in yours. But again, for most things, you should see a similar experience.
To get started, I'm going to upload a dataset. So I'm going to upload from my computer this CSV file that has every commercial flight in New York City during the month of January 2013. But you see I also have the ability to connect to Google Drive and Microsoft OneDrive if my administrators have made this available to me.
I'm then going to enter in my first prompt, and I'm going to ask it to describe what's in this dataset. First of all, chat GPT gives you a little bit of a UI to experience the file directly.
So when I upload a file like this CSV, I can see all the columns around the flight ID, the year, month, day, departure delays, arrival delays, etc.
And we can already see a response from chat GPT that has described what's in the dataset. Well, how is it doing it?
At the very end of this response, you can see this little kind of blue set of characters where it says View Analysis. When I click View Analysis, this is really the core of that data analysis product where you can see that chat GPT has probabilistically generated code.
In this case, it's generated Python code. It has run a few different commands to import a Pandas library, to bring in the CSV we uploaded, to read that CSV, and then to run a command that brings back the first five rows.
But the key here is that chat GPT not only generates the code, it then executes the code inside of this IDE and gets back the response. So chat GPT can read these first five rows in this dataset to begin to understand what's happening in the data. It can then take this response and use this response to inform the user about different information about the metadata to ultimately describe the data.
And you'll notice it describes each of the 29 columns. And in some cases, even when metadata is not explicitly said, it infers what the metadata means. For example, departure delay, it correctly infers, is in minutes.
So again, this is an example where it's really important that definitions are correct. Because if that were incorrect, if it were seconds or hours, it would be important for you as the user to inform chat GPT to make sure that future calculations are accurate.
So we are now beginning sort of the stage two of the four stages we mentioned where we are preparing the data. So now we've uploaded it. We can take a few steps to prepare the data. One of the first steps that can sometimes happen is that maybe there's a particular column that you need to add to this dataset for a certain data analysis or data science question.
So I want to show chat GPT's ability.
to not just investigate the data, but to actually change and alter the data. So, I'm going to go back to this window that has my list of columns here.
I'm going to select the year, month, day, and departure time. And I'm going to bring in a set of instructions. First of all, when I highlight these four columns, you'll notice that on the right-hand side, ChatGPT notices the four columns and understands that I'm now asking a command that is specific to these four columns.
When I paste in the instructions, I'm going to hit enter, and then I'll show you what I did in a second. I basically asked, hey, these four columns would be more helpful merged as a Unix timestamp for departures. In other words, I want to take that year, month, day, et cetera, and turn it into a particular timestamp. And then I want to do the exact same thing for arrival times too.
Can you add two new columns to this dataset and then merge them into the original one? So, what you'll notice is that we run this initial one, and it's looking for, hey, are there missing timestamps in the departure time and arrival time?
So, it's doing a little bit of checks to see what might we need to do. And then the second time, it tries running the actual code necessary to apply the Unix timestamp for arrivals and departures.
And one small note that I'll make here is that it's actually able to understand a relatively nuanced issue where instances where there's a flight directly at midnight or the departure time directly at midnight, for the Unix timestamp, it needs to change it from hour 24 to hour zero.
And it's able to understand error messages that it got and then actually update that correctly. So, it's not just generating the Python code. If it receives an error, it's understanding that error and then rewriting the code necessary to get to the answer you need.
If I scroll over to the far right here, you can see the departure Unix timestamp and the arrival Unix timestamp. This is critical for some of those preparation steps to make sure that you have all the columns necessary for your analysis.
Let's go to one more question that might be common in a data preparation step. And it's all about anomaly checks. Typically, if you're familiar with your data set, you know the kinds of anomaly checks you need to run. But what about a data set like this that you're maybe not as familiar with?
Well, you can ask ChatGPT, what are some anomaly checks that I should run? And then go ahead and just run those checks and summarize the anomalies you find.
So, ChatGPT is able to come up with 10 anomaly checks that it recommends running. And then it actually goes through the step of writing all of this code. And I'm jumping ahead because it wrote so quickly.
But you can see that the code necessary to write number one, number two, number three, et cetera. It's going through all 10 of these anomaly checks and writing the necessary code and writing comments as well. So, it's writing documentation so that others can follow along with it as well.
And then it builds this kind of summary table. And then it actually runs this step. It brings back the results of these anomalies. And then at the end, it can summarize for a user in plain English what's happening with the summary of anomalies.
So, no duplicate rows, but plenty of missing values, a few negative values around departure delays, invalid departure and arrival times, et cetera. So, it's able to find some instances of where that might go wrong.
That was really kind of the second part, right, around data preparation. But now that we've prepared the data, we feel comfortable with the data, let's go to the third and kind of most exciting step, which is around data analysis.
Again, if you're less familiar with this data set, the first question you might want to ask is, what should I know about, right? So, I'm going to ask it, what are four interesting data analytics questions that I could ask?
Go ahead and answer these questions and visualize them too. So, it comes up with these four questions. What's the distribution of flight delays, both departure and arrival across different airlines? Which airports experience the most delays? How do flight delays vary across different times of the day? And what are the top destinations from New York City?
And then it goes through the process of going question by question, writing the necessary code.
And this is not just the code necessary to get the answer, it's the code necessary to visualize it too.
So, in this first example, it is bringing in a bar chart and providing x and y axes, creating an x-axis label, a y-axis label, a title, and a legend, and then saying, hey, make sure to show this plot. It's doing the exact same thing with the second visualization, the same thing with the third visualization, in this case, a line plot.
The same thing with the fourth visualization, another bar plot. And then down below, it actually executes that code. And now you can see different visualizations that emerge.
So, you can see the average departure and arrival delays by airline. SkyWest is not looking so good. You can see the average departure and arrival delays by airport here as well.
You can see the average departure and arrival delays by time of day.
And then the top destinations. In each case, it's writing the necessary code to build the visualizations. And of course, if you wanted to change the color scheme, the font, anything else you wanted to change here is absolutely possible.
I do want to show you in just one of these examples that we're also working in ChatGPT with updated visualizations or more configurable visualizations. So, if I go to this line graph with the departure and arrival delays by time of day, you can always go back to our old sort of static visualizations as well.
But you have the ability to configure what each of these look like. So, if I take something like departure delay and I change the color scheme, this might not be, let me try one more.
Well, normally when you change the color scheme, it updates the visualization accordingly. And that's usually the way it works.
And we are constantly increasing the number of configurations that we offer. So, not just different colors, but we're going to be adding in more configurations around labels as well. So, stay tuned as we add in more of those configurations along the way.
Let me ask a few more challenging data analysis questions. One thing we haven't shown yet is our GPT vision capability. So, I'm going to upload a picture of a dashboard. This is not actually a dashboard. This is my own handwriting that I wrote on a whiteboard.
And I'm going to ask ChatGPT to describe this image and then generate a dashboard like this. So, if I open this up, again, you can see this is not a real dashboard. It's just my own handwriting.
On the left-hand side, you can see the number of total flights. In the middle, you've got a pie chart with the number of flights by origin. On the right, you've got the number of flights by route as a bar chart.
So, first, GPT vision is going to do its work and take this image and convert it into text.
So, you can see what it's done. It said, hey, this image is a conceptual sketch for a flight analytics dashboard, and there's three sections. And it correctly identifies what these three sections are and correctly identifies the visualization that goes along with it. So, you've got total number of flights, a pie chart, and a bar chart.
And then, of course, data analysis is able to go through the necessary steps to write the code to put all of this on a single dashboard.
And voila, you now have a dashboard with those three visualizations right in a row. So, again, the power of this is not just running data analysis steps. It's taking folks that are less familiar with being able to build visualizations and enabling them and empowering them to be able to get to the kind of analytics they need.
I next want to show a couple of examples of more complex questions. Everything we've done so far has been pretty basic data analysis questions.
But I want to really make sure that, you know, for teams with a large data science function, that they can appreciate what we can do as well.
So, I'm going to ask a couple of more complex data science questions. One is around k-means clustering.
So, I'm going to ask ChatGPT to basically cluster these flights and the routes across three dimensions.
So, I'm going to ask it to cluster by average delay, average flight time, and the number of flights, and then show that visualization as a three-dimensional visual.
You can see that ChatGPT has no problem doing that. So, it's made three clusters. It can make five or six, but it figured out that three is kind of the optimal number.
There's a yellow cluster that seems to have a higher number of flights and lower average departure delays versus, you know, that blue cluster that's got a lower number of flights, but maybe higher average departure delays. Purple is kind of in the middle there.
And to really see what this is doing, I'm going to, again, click on this view analysis, and you can see that we're now starting to bring in libraries, this sklearn, for example, that are much more focused on data scientist workflows.
But it's able to take my question, convert this into the necessary steps to apply k-means clustering, and then actually build that 3D visualization with an x-axis, y-axis, and z-axis as well.
The next one I want to show you is around forecasting, since that is certainly a common task the data science teams need to complete.
Monte Carlo simulations are one of the more advanced ways of forecasting, where instead of running a single forecast, you actually run hundreds, if not thousands, of forecasts simultaneously.
So, I've asked ChatGPT to prepare for a Monte Carlo simulation to predict next month's flights.
So, this data set is from January 2013. So, I've asked it to predict the cumulative minutes delayed in February 2013, and I want it to take in kind of the average and standard deviation in January, apply that to February with a thousand simulations, put each simulation in gray, and then the mean value in orange.
And you can see it had no problem handling that task as well. You see all the strands in gray with the highest possible values and the lowest possible values of delays, and then that mean value in orange.
So, it's a slightly more complex way to, you know, to analyze something, but it can be critical for data science teams that have those needs.
Again, you can quickly see the analysis that was run. We're calculating the mean and standard deviation. We're running a thousand simulations of these results, and then ChatGPT is able to visualize these thousand simulations effectively.
That was kind of the third section, right, around analyzing the data, forecasting the data, doing all sorts of data analysis and data science capabilities.
I now want to shift into the fourth and final section before we go all the way back to the beginning of using ChatGPT to bring in information from your data warehouse.
So, the fourth and final one is around how do we bring in GPT actions to be able to empower the sharing of these results and the delivering of these insights to others around your organization.
So, to do that, I first just want to show the very easiest thing you can do, right?
So, before we get into custom GPTs and GPT actions, the very easiest thing is to simply just download the results you've gotten, right?
So, I can take, for example, this Monte Carlo simulation and just download the chart, and I will get this as a PNG file, and I can, of course, share this wherever I want. That is the simplest method, pretty foolproof. But even if I wanted a CSV with that underlying information, I could just ask ChatGPT to produce that.
So, I could say, hey, could you just create a CSV of the underlying data and the Monte Carlo simulation? Once again, ChatGPT is going to use Python to turn those Monte Carlo results into a CSV file, and then it's going to give you this link to be able to download the file, and now I can download the CSV directly.
Now, those are great. They're no-code solutions that are very quick, but ultimately, a lot of work happens outside of ChatGPT. We want to be able to connect directly to those third-party systems, and that's really where our GPT actions come in.
Before I show you an example of a GPT action, I want to take a little bit of a step back and say that these GPT actions are a part of something we call custom GPTs.
Custom GPTs allow you to further tailor the experience for users. They don't have to involve GPT actions that connect to third-party APIs. They could simply be something that translates a user's request, or you might attach a document, and it can summarize some of that information.
and answer users' questions. So, they do not have to involve GPT actions. They're just a way to further tailor and refine users' experience. But one of the most popular and common applications of custom GPTs is GPT actions, which can connect to third-party applications.
To show you one, I'm going to show you two examples separately, and then I'll show you running them live directly in this chat. This first one is an example of a GPT action that we built in our demo environment that connects to Microsoft Outlook.
I want to be clear when I show this one in the next action that these are things that we built in our particular demo environment. We do have cookbooks available for other users to deploy them on their environment, but it's not out of the box. You would need to actually build this action yourself. But we've built an action to our Microsoft Outlook system. So, if I ask it to write a haiku, it's going to do that just fine.
And the second one I'm going to show you is around a Jira Assistant, where I'm actually going to create a ticket. So, we have this Jira Assistant GPT action where I can ask it to generate a ticket.
One of the nice things about ChatGPT, though, is that I can call these GPT actions directly inside of a chat window. So, if I call this Microsoft Outlook GPT, I can just start typing an at, and then I'll bring in this Microsoft Outlook GPT, and now when I ask it, hey, could you please summarize the key insights from this conversation and email them to my colleague, this Microsoft GPT is going to go through the steps of understanding what I'm asking. It's going to generate those key insights, and then it's going to take those insights and convert that into the input schema necessary. It's going to confirm that I'm comfortable hitting that graph.microsoft.com API.
You can tell this is a live demo with the error that we hit. Let me quickly show you. I'm going to show you one more time with this one where you can see what I've done. So, in this case, if I said, hey, write a haiku and email it to this email address, it talks to the graph.microsoft.com API call. This is the message that gets sent. So, there's a subject, a body, text that gets sent along the way, and then it says, hey, the haiku has been emailed to this Gmail address. I can test it in my Outlook.
You can see this is the API call that was run, and you can see the action working directly. I'm going to try this one more time. If it doesn't work, I'll jump over to the next action, but let me just try it one more time.
Oh, it's asking about the correct name. And if this one doesn't work, we will jump over to the next one. Here we go. All right.
Awesome. It talked to graph.microsoft.com. One more time. Confirm. Gosh. You can tell this is a live demo. But again, the spirit of this is that it will go directly to graph.microsoft.com, and it will generate the necessary components.
I'm going to try one more action here. This is our Jira Assistant, and what I'm going to ask the Jira Assistant to do is actually build or file a bug that summarizes the anomalies that we discovered earlier. So, I'm now calling the Jira Assistant, and this one should take that summary and send that bug along to Jira. I'm going to hit confirm. Hopefully, we get a better result this time.
So, it's talking to api.atlassian.com. Here we go. And it's bringing in the necessary fields with a project, a summary with information, the customer name, the customer ID, the issue type. And it said, hey, the bug has been successfully filed. Here are the details that we want to include. And you can also view this directly in this ticketing system. And if I refresh my screen, here we go.
Awesome. So, you can see BT1084. So, this is something that I generated in Chat GPT Enterprise, and you can see directly in our Jira system that now I've got all of the anomalies detected here.
And we're off to the races where we've actually done something directly in Chat GPT Enterprise that actually impacted another application. So, this is critical for kind of communicating those insights to your organization. And in this case, it might be helping folks on kind of the data cleanliness team or the data management team to be able to better understand what might be wrong with their data set.
So, now that we've done the delivering the outputs, I want to go all the way back to the beginning. When we started our demo, we started just with a CSV file. But we don't actually have to do that. We could start instead with pinging SQL directly and pinging a data warehouse to bring back the information that we need. So, I want to start all the way back at the gathering input stage and talk about how Chat GPT can help there as well.
Before I do that, I want to show Chat GPT's ability to just write SQL generally. You've seen Chat GPT write in Python, and our data analysis tool requires Python. But Chat GPT has the ability to generate code in just about any major coding language. So, if, for example, I say, hey, I have two tables, a flight table and an airport table. Can you write me a SQL statement to list all the rows from one of New York City's three major airports in January 2013? And it will write some pretty good generic SQL.
We're not sure if the schema is perfectly right. We're not sure if there might be other things that are necessary. But it's sort of a general SQL statement. It does a pretty decent job. And just to show you how versatile it is, if I say, hey, can you now write that same SQL statement in Excel, R, Stata, JavaScript, it has no problem writing the steps necessary in Excel, the steps necessary in R, the steps necessary in Stata, and finally in JavaScript. So, it truly can write in just about any language. We've seen Python. We've seen SQL. We've now seen these other four languages, too. So, anywhere where you need to gather your inputs, you can use Chat GPT as that starting point to help write some of that code.
The final thing I want to show you here before we wrap up and conclude this demonstration is just an example of being able to run an action to do this directly. So, if I go back here to my... So, this is an action that we created that queries BigQuery and Google Cloud directly. So, the dataset that has a lot of this Flights data lives inside of BigQuery, which is inside of Google Cloud. We built an action that can query these directly.
So, if I go here and I ask it, hey, could you list all of the rows from one of New York City's three major airports and make sure to include this other information, this custom GPT that has a GPT action associated with it is going to go through a few steps in a row.
The first is that it's going to fetch the schema information necessary from this dataset. So, it doesn't actually know what the schema is, but the very first query it's going to run is to hit the metadata of this table to be able to bring back the relevant fields and columns. Once it's done that and it's actually brought back that information, it can now structure the query correctly. So, it's building the query that it needs to and it's writing all of this and this is the correct query. And you can see, it's adding in that limit the first time to make sure that it works. Once it works, it actually goes directly to BigQuery to run this particular query.
And once it runs that query, it's then able to hit a Google Cloud function that can bring back the necessary CSV file. So, once I hit confirm, I'm just confirming that I'm okay bringing back that CSV. We just talked to that Cloud function inside of GCP with this information and now it's going to bring back a CSV with all these relevant results.
And here I have the query results from that query and these query results match exactly what we started our demonstration with. So, I could have started with this action or, of course, I could have just uploaded the CSV directly. We're going to jump over and conclude this webinar.
I want to share just a couple more slides. This first one is really just a point about what's going to change and improve in the future. Everything we saw today and we went through today is really impressive and would have been incredibly impressive a year ago, five years ago. But if we kind of continue that curve along, continue that trajectory, the models are only going to get more and more impressive over time.
And we're excited by what kind of data science and data analytics capabilities are possible in the coming years.
Great. Thank you, Aaron. I just want to say a few words to close out. So, we really hope that you took some tangible ways to implement some of these capabilities and we gave you a great overview of ChatGBT Enterprise for data analysis. If you have any more questions, please reach out directly to your OpenAI account team.
And once we close this webinar, you will be shown a short survey. It would be great if you could spend a minute just filling that out to tell us how we did so that we can improve these sessions over time. Huge thank you to everyone for joining for your time this morning. We hope that you enjoyed today's demo.