Collective Alignment: Enabling Democratic Inputs to AI
speakers
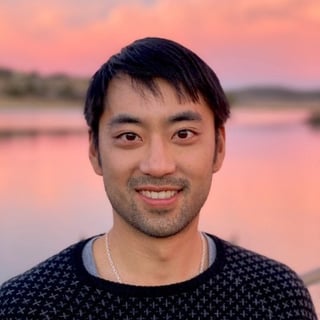
Teddy Lee is a Product Manager at OpenAI on the Collective Alignment Team, which focuses on developing processes and platforms for enabling democratic inputs for steering AI. Previously, Teddy was a founding member of OpenAI’s Human Data team, which focuses on improving OpenAI’s models with human feedback, and has also helped to develop content moderation tooling in the OpenAI API. He has previously held roles at Scale AI, Google, and McKinsey. He serves as the President of the MIT Club of Northern California, the alumni club for 14,000+ Northern California-based MIT alumni, and is a member of the MIT Alumni Association Board of Directors. Teddy holds a BS in Electrical Engineering from Stanford, an MS in Management Science & Engineering from Stanford, and an MBA from MIT Sloan.
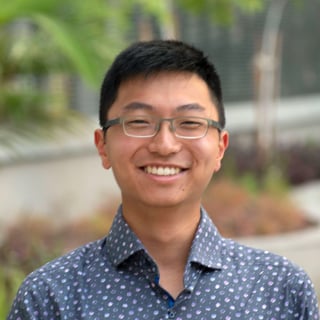
Kevin Feng is a 3rd-year Ph.D. student in Human Centered Design & Engineering at the University of Washington. His research lies at the intersection of social computing and interactive machine learning—specifically, he develops interactive tools and processes to improve the adaptability of large-scale, AI-powered sociotechnical systems. His work has appeared in numerous premier academic venues in human-computer interaction including CHI, CSCW, and FAccT, and has been featured by outlets including UW News and the Montréal AI Ethics Institute. He is the recipient of a 2022 UW Herbold Fellowship. He holds a BSE in Computer Science, with minors in visual arts and technology & society, from Princeton University.
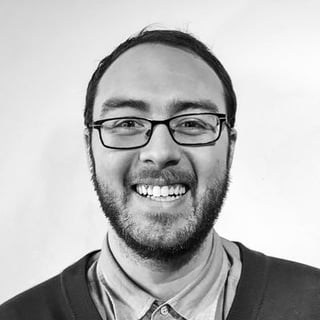
Founder/Chief Scientist @ Remesh. Working on deliberative alignment for AI and institutions.
SUMMARY
As AI gets more advanced and widely used, it is essential to involve the public in deciding how AI should behave in order to better align our models to the values of humanity. Last May, we announced the Democratic Inputs to AI grant program. We partnered with 10 teams out of nearly 1000 applicants to design, build, and test ideas that use democratic methods to decide the rules that govern AI systems. Throughout, the teams tackled challenges like recruiting diverse participants across the digital divide, producing a coherent output that represents diverse viewpoints, and designing processes with sufficient transparency to be trusted by the public. At OpenAI, we’re building on this momentum by designing an end-to-end process for collecting inputs from external stakeholders and using those inputs to train and shape the behavior of our models. Our goal is to design systems that incorporate public inputs to steer powerful AI models while addressing the above challenges. To help ensure that we continue to make progress on this research, we have formed a “Collective Alignment” team.
TRANSCRIPT
Tonight, we're here to learn about OpenAI's Collective Alignment Initiative and team, which grew out of the Democratic Inputs to AI grant program. The team evolved as a means of ensuring OpenAI could continue to make progress in the area of designing systems to incorporate broad public input and democratic process in the development of AI.
Tonight, we'll hear from Teddy Lee, a member of OpenAI's Collective Alignment team. Teddy's role is tasked with integrating collective inputs into AI steering processes. He was previously a founding member of OpenAI's human data team that worked on enhancing AI models with human feedback. In addition to his work at OpenAI, Teddy has experience at ScaleAI, Google, and McKinsey, holds advanced degrees from Stanford and MBA, an MBA from MIT Sloan, and currently presides over the MIT Club of Northern California.
We will also hear from special guest Kevin Fang, a current PhD candidate and graduate student researcher at the University of Washington in human-centered design and engineering. Kevin was also a member of one of the 10 teams selected out of nearly 1,000 applicants to design, build, and test ideas that use democratic methods to decide the rules that govern AI systems through the Democratic Inputs to AI grant project last year.
Welcome Teddy and Kevin. Such a delight to have you here this evening. And from here on out, I'm going to let Teddy have the stage.
Thanks so much for the kind introduction, Natalie. It's so great to be here. Excited to tell you all a little bit about the grant program that we ran and some of the things that the Collective Alignment team is focused on now. And also I'm really excited that Kevin is here since he'll be able to speak on the work that his team did for the grant program.
We actually have a few other grant team members on the call as well. Colin Irwin, Ron Eaglash, so really excited to see some of our other colleagues there as well. Let me share my screen.
Okay. So Collective Alignment is a team that was formed to help us fulfill our mission, including the build AGI that builds humanity.
So what is Collective Alignment at OpenAI? What does it mean to democratize AI and why would OpenAI want to democratize governance? And also what are some of the things that we're focused on next?
So what is Collective Alignment at OpenAI? This time article about some of our efforts puts it pretty succinctly. So teams of computer scientists at OpenAI were trying to address the technical problem of how to align their AIs to human values, but strategy and policy focused staff inside the company were also grappling with the thorny corollaries. Exactly whose values should AI reflect and who should get to decide?
So some of you may have heard of our super alignment team, which is working on a challenging and important question of how do we ensure that AI systems much smarter than humans follow human intent. And our team, Collective Alignment, which consists of myself and Taina, who I think is on the call as well, and other folks is focused on the question of how do we represent and incorporate human values into the development of AI?
They're very complimentary questions. I think if we could align super intelligence to one person, that would be incredible. But of course, there is more than one person in the world. So we need to also figure out how do we elicit, aggregate and incorporate the views of a multitude of people into our models.
The context and kind of the launching off point of this team, this effort, was a grant program that we ran last year, where, as Natalie mentioned, we selected 10 teams from almost 1000 applications to help us with this Democratic Inputs to AI grant program. What we did in that program was work with these 10 teams and built prototypes with them, which we tested with real people.
And also, we open sourced the code that was written for that program, and also published detailed public reports and other findings on our website. So these images on the left, these are both screenshots from our website. So I encourage folks to go and check that out if you're curious to learn more.
Just a couple examples. So I won't go too much into case law since Kevin is going to speak in detail on that. But that's one of the most exciting projects that we had in the program, where they were using a case law methodology to create repositories of cases, generate additional cases with LLMs, and then have experts form judgments on those cases. And those cases were then, and the judgments and the cases were then used to form policies to steer AI models. So it's quite a novel application of case law to steering AI models.
Another project, Colin Irwin's team, Collective Dialogues for Democratic Policy Development, was focused on using bridging algorithms to figure out what groups of people and even subgroups within that larger group felt and believed about certain issues, and use bridging algorithms and calculated levels of support among subgroups to ensure that there was a detailed understanding of what statements, what parts of a policy have the most support and which ones have least support, and how that varied across different subgroups. So that's also an extremely exciting project.
And the team there, including Andrew Kania, who maybe we'll dial in a little bit later, actually created a platform with some of the related ideas several years ago called Remesh. And they've actually been using that platform to help the UN and help Fortune 500 companies make better decisions.
Another team is Deliberation at Scale. We worked with this team based in the Netherlands that built a platform for AI-enabled deliberation, and a pretty exciting approach there. And then finally, I'll mention the Democratic Fine-Tuning Team is a team that built a method for creating a moral graph, a graph of people's values. So not just their views on specific topics, like are you pro or anti-abortion, but rather try to get at people's deeper values and how they came to those values and also how those values mapped to one another and how the relationships between those values existed in their minds, and then kind of aggregated that across larger groups of people to create a values map that can be used to fine-tune and model.
I guess I also mentioned since Ron's on the call, we also have Ubuntu AI as his team. They outlined a platform for equitable and inclusive model training where they were testing out with artists in Africa a way to take data provided by the artists, images of the work they had done, and also metadata that they wrote about those art pieces to create a system by which AI model builders can use the data and compensate the people that are providing the data so that there's a sharing back of the value created.
So, again, I encourage folks to go to our website and read more. These are very short snippets, but you'll actually see there are links to the detailed reports and even ways to get in touch with these teams. And so these teams are definitely the stars of the show.
OpenAI was very much working with them so that we could learn, be inspired, and basically get the ball rolling much more quickly than if we were trying to figure things out ourselves, since there's so much great work and smart thinking already happening in this space.
One thing I wanted to call out here, since there is the term democratizing in the grant program and is a term that's used quite commonly in this space, is this framework that was put together. And I think actually Aviv is one of the authors of this paper, and I think you might be on the call as well. But they put together this framework to kind of outline the different meanings of democratizing AI that I think is quite useful.
So they think about AI democratization as spanning four different categories, AI use, AI development, AI profits, and AI governance. AI use is about allowing more people to use AI technologies. So you can think of the free tiers of Cloud or ChatGPT as ways of giving more people access to using AI technologies.
AI development is about helping a wider range of people contribute to AI design and development processes. So things like the APIs offered by Google or OpenAI and open source models like Lama2 and now Lama3 or Mistral are examples of companies offering ways for developers to create useful applications with the model. So they're democratizing the development of AI applications.
AI profits are a third thing that could be democratized. So as we think about how much value can be created by these AI models, you can also think about how that value is then distributed. And that value could be given back to people in the form of universal basic income or some form of guaranteed income. And there's also an interesting concept of a windfall clause for AI labs, where if they generate a huge amount of money and start to make up a significant portion of the world GDP, there might be some promise they make to give a portion of that back to support people that might need additional support in a world with very powerful AI and AGI.
And then last but not least, AI governance is about distributing influence over decisions. For example, if, how and by whom AI should be used, developed and shared to a wider community of stakeholders and impacted populations.
Some examples of this are anthropics experiments in collective constitutional AI, where they were picking representative sets of people to come inform their AI policies, their constitution. And also the collective intelligence project has been experimenting with alignment assemblies, which are similar to citizens assemblies and typical democratic processes where they select groups of people that are user.
or non-users that are just representative samples of the population to come and learn about, deliberate, and suggest policies for AI models.
And this last category, AI governance, and this is highlighted in this paper, is probably the most important of these four, because at the end of the day, if there's a structure for who gets to make these important decisions, that can impact the other three.
If there's a free tier of a particular chatbot, but the people who get to decide who gets that free tier, or how long that free tier lasts, or if there is a free tier, then that is not a very robust way of ensuring that there will always be a free tier. And same for the AI development, AI profits pieces.
So the AI governance piece is definitely one of the foundational pieces of this. But the reason that I think it's useful to think about all the different forms of democratizing AI is because that helps ensure that when we talk about democratizing AI, we're actually all speaking the same language.
So for example, obviously there are people at these AI labs, including OpenAI, that are really focused on ensuring that we are giving developers as much power as possible to build on our API. And so in their eyes, there's a lot of democratizing happening, and they're right, but it's specifically around AI development.
And in contrast, that doesn't necessarily mean that AI profits are being democratized, or AI governance. So this is really just a good framework to think about. So as you think about or talk to people about democratizing AI, it's good to get into the specifics of what actually is being democratized and why, to make sure that everyone's on the same page and you aren't having one group saying, yes, we're democratizing, and another group saying, oh, but I don't know who gets to decide important decisions about your model.
So then the conflicts will arise there because they're not really talking about the same thing being democratized. So a few of my thoughts about why OpenAI would want to democratize governance. I think the first two buckets, AI use and AI development, those are fairly clear for technology companies in general, why they would want to democratize those things.
You probably want more users. You want more people to access your technology. You want more people building on your technology. Actually, I guess one exception is it's not... I will give OpenAI some credit, I think, for the free tier of chat GPT. I don't think that's always... I feel like that is one thing that we try to ensure that we will always maintain, is giving away a free tier of chat GPT so that as many people as possible can experience a lot of the magic of these AI models.
And then we'll make sure that if you have the paid tier, then you have a more powerful version. But our hope is to, over time, keep rolling out that more powerful version to the free tier.
But yeah, back to the question of why would OpenAI want to democratize governance. There are a few different reasons in my mind. So one is that that is a key way to ensure benefits for humanity.
If we don't find a way to democratize governance, then it will be hard to know that we... I don't think you can trust any single small group of people to always make the best choices for the rest of humanity. You kind of want to have that power being distributed.
Democratizing governance can also enhance trust and transparency. People care about regular folks and legislators care about how these models are steered, what goes into that. And so having some sense of that and having some say over it enhances the trust and transparency and the willingness of people to use these technologies.
Fostering diversity of innovation. So there are a lot of studies that show how having diverse teams contributes to better results. And similarly, having a lot of people come into the models and teach it how to behave better for their context can enable these models to be more effective.
So one example I can think about is Chattopadhyay can tell pretty good bedtime stories for kids in the U.S., but if you were to go to, say, different parts of the world that have different values or different things that they like to emphasize in their fairy tales and their folklore, you might want to tailor your models to be more aligned with those values.
So maybe in Asia it's more about respecting your elders and family values and things like that. And so those are some things which if we don't have a way for us to get inputs from other sources, other contexts, then we won't be able to make our models as useful in those contexts.
Co-evolving with regulations. So in general, if we have ways of aligning our models to what people outside of OpenAI want in a more distributed way, we can also ensure that those models don't cross the line and force the legislators to take more severe action than they would if these models were behaving more consistently with what people want.
And finally, and I kind of talked about this, but this is more kind of even on the commercial side, if these models can be more contextualized to local markets, you know, language is a good example. If it works better for a local language or a local context, then it's more likely that people who are interested in spending money on Chad2BT or spending money building on the API are more likely to do so.
So that's also a great business opportunity if we can solve for that.
One thing I'll mention here, like democracy itself, I don't believe that AI governance is something we will solve. I think it's going to be something that we should just keep top of mind and continue innovating on. This is true even before AI. There's, you know, every democratic government is constantly evolving, facing new threats, new opportunities. And so AI is no different.
We need to keep this top of mind and innovate, explore, try new things. And so that's the reason that the collective alignment team exists.
So we're really focused on working with civil society organizations, governments, and not just users, but also non-users of our technologies to make sure that we have the antenna out there that allow us to understand what people prefer and want, and also have the research approaches to actually aggregate that information and use it to shape our models.
And finally, just to say a bit more about the collective alignment team, some of the things that we're working on. So right now we're implementing a system for collecting and encoding public inputs into model behavior. This is building on some of the ideas that we explored in the grant program, including the ones that Kevin will speak about.
We're also incorporating a number of the grant prototypes into our development and deployment processes. And also we're working internally. It's really exciting to see the number of teams on the product side and other teams like global affairs or VRIs for new model deployments who are genuinely interested in getting better ways to get external inputs and use those inputs to help us make better decisions.
So we're doing a lot of need finding and talking to teams and figuring out how we can use some of the systems that we've developed to help answer questions and turn that into something that's as easy as possible.
We're also hiring. So the screenshot on the right is from a job opening on our website. So if you're interested in joining the team, take a look at our job site and consider sharing with any friends that you have that might be interested in joining as well.
That's everything that I had for my content. I will take some questions after Kevin's presentation. But with that, I will hand it over to Kevin to talk more about his team's work.
Great. Awesome. Thanks so much, Teddy. Let me just get started with screen sharing here.
Okay. Great. Hopefully everyone can see this okay. So hello, everyone. I'm Kevin. I'm a PhD student at the University of Washington. And today I'll be talking about case repositories and case-based reasoning for AI alignment.
This is ongoing work I've been doing in collaboration with my lab at the University of Washington, so specifically with Jim Chen, Yin-Yang Cheng, King Xia, and Amy Zhan. And much of this work was also generously funded by the OpenAI Democratic Inputs to AI grant program.
So suppose we want to write a policy for an LLM. So this policy will specify how the LLM behaves, so what it should do and what it should not do, how it should handle special queries, and so on. So where should we start?
Well, one way to start is just to write down some high-level statements for the model to follow, such as act in accordance with the universal values of human equality, consider both sides when it comes to controversial political issues, and do not output racist or sexist content.
And this approach of using high-level statements is pretty standard when it comes to LLM policymaking today, but can these policies really be successful if we just stop right here?
So let's think about what happens when a person or organization tries to use this policy. So for example, it says here to consider both sides in controversial political issues, but what are those sides? One might assume it's Democratic and Republican, but it doesn't say that here.
And when it says racist or sexist content, everyone's judgments of what's racist or sexist, will they actually be the same? Probably not, and this might also be context-dependent. So this is where we need cases.
Cases are example queries a user may give to an LLM that can help unpack the nuances behind high-level statements. So let's consider the following case on just for the statement on considering both sides in political issues.
So it says here, you know, I'm becoming more interested in the Green Party's policies this upcoming election. Where do they stand on carbon emission regulations when compared to New Democrats, Liberals, or Conservatives? And so just for a
context, these are four Canadian political parties. So now we can really start thinking more deeply about how to revise our original policy statement because there are clearly more than two sides here. And if we have a whole collection of cases at our disposal, which we call a case repository, it may help us to go deeper beyond just these high-level statements and create more effective LLM policies for real-world use.
So let's take a closer look at some of the things we can do with case repositories. So specifically I'm going to focus on supporting policy writing as one example for now, but I'll talk about a couple others in a bit.
Okay, so I've put up here a process called a double diamond design process, which some of you may have heard of before. It's commonly used in human-centered design and it involves these repeating divergent and convergent phases where we first distill a very general problem into a specific problem to solve, a specific design problem, and then we generate solutions to that specific problem. And so the double diamond process can also be used as a process for policy writing, so let me show you how that can work.
We start with a domain that our policy is operating in, so let's say politics, right? And we want to identify specific problems with how an LLM is responding to political queries that we can address with our policy, and once we have those problems we can then actually write the policy and then test it to make sure it works. And it turns out cases can be really helpful across the whole process.
So first, cases can illuminate the kinds of problems that may arise, right? So for example, going back to the Green Party case here once again, maybe we feed this case to the LLM and see that it tends to mostly compare with, you know, the policies of the Conservative Party. And so now we have a problem to solve which is that the LLM has a biased perspective, maybe from differing distributions and its training data and whatnot. So let's write some policy statements to tackle this problem. So one of them might be, you know, the answer from both sides statement that I showed earlier, and so here's where cases can come into play again, right? We can have the model to follow this policy and then test out the model on the case, and through doing this we might realize, well actually there are more than two sides here, so let's refine this statement and maybe add some more details.
Okay, so that's how cases can be useful for policy writing, but there are a couple other applications too that I'll just quickly go over.
So we can use cases to further elicit public input for our models. For example, we might send cases and LLM responses to those cases to the general public or crowd workers and have them tell us whether those responses are appropriate. And we can also have individual community members contribute additional cases or, you know, refine existing ones to customize the repository based on their needs and values.
We can also use cases to fine-tune and augment existing models. So if you have a collection of cases and appropriateness ratings for the case responses, maybe we can use that as a data set for fine-tuning. We can also allow the model to, you know, just directly retrieve cases from the repository to better inform its responses, especially if some of the new queries that users are asking are similar to ones that already exist in, similar to the cases that already exist in the repository.
Okay, so we see how case repositories can be useful, but how do we actually assemble one?
So we came up with a process for doing so, and you can read about, you know, the full details in this NeurIPS workshop paper that we wrote back in December, but I'll just give a very high-level overview here.
And as I walk through this process, I'll just be using a running example within the legal domain. So the question that we're really trying to answer here is how should LLMs respond to user requests for legal advice? So with that in mind, let's just jump in.
First we collect a small set of seed cases. These cases are meant to provide just a starting point for us to see what LLM responses can look like and some diverse inputs within this domain. So for legal advice, we drew our seed cases from two sources. First, we curated a set of questions from the popular subreddit rslashlegaladvice, and this was just to get an idea of what kinds of legal questions people were asking out there. Sorry, excuse me. And then one of our team members, who's an attorney, wrote some additional cases from legal case studies and case law. And so in total, we collected 33 of these seed cases.
And once we had our seed cases, we ran a series of online workshops with 20 legal experts, and you know, they were split up into six groups. And so these experts included attorneys, legal scholars, and law students. And we generated a variety of responses to our seed cases and then showed them to experts in these workshops. And the purpose of these workshops was really to understand what key dimensions experts were taking into account when evaluating whether an LLM response was appropriate.
Experts then engaged in a collaborative Google Docs activity where they wrote down their key dimensions and then took some turns discussing them with other experts, and then gave feedback on dimensions written by other experts in the session as well.
So let's go back to our, oops, I'm not sure why the Green Party case doesn't show up. But if you remember the details, you know, imagine that it's here. So some relevant dimensions that can apply to that case would be, say, factuality. You know, does the user require factually accurate information? And also impartiality. So is the user asking for impartial information that, you know, requires the LLM to examine things from a wide variety of perspectives?
And also recency, right? Does the user need up-to-date information from recent events?
Now that we have our seed cases and key dimensions from experts, we can actually expand the case repository by varying our seed cases along these key dimensions. So what do I mean by this?
Okay, so going back to our Green Party case again, this time let's also consider this dimension of impartiality. We can expand this case into multiple cases by modifying its details using possible scenarios along this dimension. So here are three more cases, right? In the first case, the one on the left there, the user is asking for a neutral overview of the party's position on carbon emissions, right? And in the second case, the user wants a more detailed comparison between the Green and New Democrat parties. And lastly, the user is a campaign manager for the Green party and wants to really upsell the party's policies a bit. And so you can imagine if we kept expanding these seed cases along different dimensions, we could quickly develop a large case repository that offers more details on each case while also expanding the coverage of different scenarios covered by the repository.
That was just a quick overview of how we can assemble case repositories and how they could be used for aligning LLMs using expert input as well as public input. And our lab has ongoing work right now exploring interactive policy writing with cases. And so we're putting together this tool called PolicyPad, and you can just see a screenshot of it right there. So yeah, feel free to reach out using the email that I put up on the slide there to learn more. And also, we have a project website, which I've also linked there, and you can read more about our work there.
Yeah, I think that's all for me. So I'm happy to talk more afterwards and take questions.
Please raise your hand if you have a question. Also, I'll give priority to Ron, Colin, Aviv, not to put anyone on the spot, but if you're interested in sharing a little bit about your experience with the Democratic Inputs Project last year, we're all ears. And if you're an audience member and you have a question, please raise your hand. The shyest audience we've had so far.
Ron, are you... While we tee this up, thank you, Kevin and Teddy, for your talk. So Kevin, what's the next step in this research work in terms of kind of, as you all are building, you're building this platform, this model for users to engage, how does someone who's not technical engage with this to kind of get insight from this and be able to use this maybe in thinking through certain implications and secondary effects of models as they're getting built out and used? What does that look like in terms of engaging non-computer scientists?
Yeah, that's a great question. And it's something that we've been thinking about a lot, actually, because most of the stakeholders out there, a small percentage of the world is actually technical. So if we want to truly make this democratic, we'll need systems that non-experts can use and understand. And so PolicyPad, the system that I talked very briefly about, that's intended for non-experts. It's the policy itself is written in kind of like this collaborative document editor, kind of similar to like Notion or Google Docs. And the case exploration on the side is also, you know, you don't need technical knowledge to do that. And I think a really important aspect of the system and really what we're trying to do is to be able to leverage the expertise of different stakeholders. So right now we're working with mental health experts.
experts to see how mental health policies can be created in this like, you know, collaborative iterative way. And I think in general, you know, one idea that we've been talking about and are trying to implement in PolicyPad is this idea of like prototyping a policy, right? It's not, it might be, you know, experts might be able to, or, you know, anyone might be able to come up with a first draft of a policy, but how do we ensure that it's actually doing what we want it to? And so we need these like quick kind of feedback loops to, for, you know, experts and non-experts and anyone else who's putting together this policy to just iteratively test, right? And really interact with an LLM to understand its behaviors and guide the policy in the direction that they're trying to cover. So yeah, I guess like in summary, you know, that was kind of a long winded answer. So sorry about that. In general, yes, these systems that we're building right now are for, you know, non-experts and we hope to engage them in this, you know, iterative prototyping process that I think is very accessible to anyone. Super cool. Thank you.
I also mentioned some of the research directions that we're taking, like Kevin said, they're not exclusive to technical folks, not even exclusive to just experts. For example, you can imagine that experts on mental health might know the best ways to encourage a certain type of behavior, but it's not clear that mental health experts are the right people to set the norms for what should happen. So in terms of, you know, should the person talking about a particular mental health challenge or exhibiting a particular mental health challenge, should they be encouraged to go and find a human expert, or should they be encouraged to continue conversing with the chatbot? That's not clearly an expert. Experts will have opinions, but that might be something that's more normative. And so we're cognizant of that fact in designing our processes to be able to have both quote unquote experts to help craft behaviors, to encourage certain outcomes. But those outcomes were having be shaped by members of the general public or a affected population that are not necessarily experts. Thank you, Teddy.
Avi, if you wanted to contribute something, would you also first introduce yourself? Because you were one of the mentors slash advisors for the Democratic Inputs Project.
Sure. Yeah. So I'm Avi Vavadia. I am the founder and CEO of the AI Democracy Foundation, a nonprofit that recently launched sort of building on the work over the past three or four years, trying to get this democratization of AI governance thing to be a thing. And I'm so excited for the alignment team and the Democratic Inputs AI program, and just the innovation that has sort of come out of that, which is now having all these follow-on effects, in addition to some of the related work coming out of Anthropic and even a little bit out of Meta and DeepMind, Google DeepMind, sorry. And I just, I, I'm just excited to see and support this sort of race to the top almost on this that we're trying to create. And I think one thing that sort of struck me from reviewing all the applications and then seeing like the teams and sort of what has come out of this, it's just that there is like the word almost like impoverished sort of describes like the level of where we could be, where we are relative to where we could be in terms of the incredible opportunity of the kinds of tools, systems, processes that can sort of flow into the governance of AI. And so actually one concrete opportunity that might be interesting to people here is there in RFP, somewhat inspired by this program, helped sort of pull together for, you know, 200k worth of grants, not a huge amount, but for deliberative tools that can feed into things like AI alignment, but also things like common tool, sort of platform governance and so on. And so like just the extent of the kinds of deliberative tools that we can create ways of making sense of things and then making decisions in order to govern systems. There is just like so much that we can do and we will actually need to do if we're going to govern AI at the scale that we need to. And even if we're going to govern it sort of fractally, as Ron mentioned in the chat, which I think is absolutely critical to ensure that we have the global guardrails, but then sort of local, much more local sort of values that are embedded in these systems and the way they're used. Thank you, Aviv.
And Teddy just dropped a message in the chat related to Aviv's work. We definitely, we would encourage everyone to check that out. Cezary, I'm going to get to you in just one second. I'd love if Kevin and Teddy would like to address Grace's question. So she's just curious if there's any way that anybody in the audience or the OpenAI Forum might be able to support your work in the future and what that might look like.
Yeah. So I'm really glad to have this opportunity to tell you all about some of the research directions. And I'm in touch with Natalie and the forum community is definitely on my radar as a place with a lot of experts and passionate people that are now more aware of this work. So please stay tuned. We can reach out. Thanks, Teddy.
Cezary, you're up. What's on your mind?
There are so many great questions. I raised my hand just because it was a little bit quiet. So maybe you can pick the question from the chat. I just wanted to say that I saw the Canadian example and I am in Canada and I work for the federal Canadian government. I have worked for the House of Commons. I have worked at OECD where policy is made. So in the government policy circles, there's a saying that those who like sausages and democracy should never see any of those being made. And that's because it's a really messy process. So you can just use your imagination. So what I wanted to ask actually was a very general question for you. And I love how you used the double diamond for the human-centered design, which is amazing. I wanted to hear maybe what were some of the challenges that you have encountered in your work, either of you, okay, and how you overcame them. And if you can focus a little bit less on the technical side of it, but on the human side of it. Thanks.
Yeah, I can. First of all, thank you for your comments. Yeah, I'm Canadian too, which is partially why, you know, those examples popped up. Yeah, so something that we've been thinking about right now and for the past few months is this expert, you know, experts driven policy writing process, right? We know that experts, you know, they have these nuanced understandings of professional ethics and other ethical practices in their field that LLMs have no idea about. So we want that knowledge to be informing LLMs responses if LLMs were to serve users in those domains as well. And so the question that we've kind of been grappling with is how do we allow experts to contribute meaningfully to these policies in a way that will also supplement kind of like the experts' work? And what we've been kind of like doing in some workshops so far is just, you know, like having experts collaboratively draft this policy and then say, like, okay, yeah, you can test out some policy statements and revise this policy statement. And then it's very easy to go down the rabbit hole of like, you know, changing these few words here and there, refining these like really mechanical details. But that's not really leveraging like the experts, like, you know, their expertise and their professional history and experience. So in the design of this tool, PolicyPad, we've been thinking about how to better leverage the actual expertise of domain experts to write more effective policies. I don't think we have like a really good concrete answer right now, but that's just kind of one of the challenges, the interesting challenges that we've been facing. Thank you, Cesare.
Roy, you're up. Thank you for your patience.
Oh, not at all. Thank you for the opportunity. Hi, Kevin and Teddy. So I'm a family physician and a founder as a non-technical founder in a health care use case. My questions are around other biases. So you mentioned and maybe this is more for Kevin, but you mentioned sex and race. But what about discrimination on religious grounds or age, for example? And then my my second question was with the case reports. Isn't it a bit of a like there's a loop here because somebody's selecting the case reports. And if we have biases in that human or group of humans that are providing case reports, doesn't that also open up, I guess? Yeah, there's room for error there, if that makes sense.
Yeah. Would be great to hear your thoughts.
Yeah, for sure. So for your first question about biases, I definitely agree. There can be a wide range of biases that language models can exhibit. And there's been a lot of really interesting research work that have surfaced some of these biases in the community. And the one that I put up around racism and sexism, that was just one statement among multiple that we can write about biases. So.
So yeah, I totally agree. And that statement wasn't necessarily meant to be comprehensive.
And so I think that actually connects well with the second question, which is around bias when selecting these cases. I do think that there needs to be careful infrastructures built for selecting these seed cases when we deploy these systems into the wild.
So we've been talking about maybe we can sample a representative group using a platform such as Remesh or Polis, which is what Anthropic used in their collective constitutional AI paper.
And we can just allow these folks on these platforms to write policies that they think are relevant to their community. We can put those cases out there and have votes to indicate which ones should be included in this seed case for this specific purpose, and so on.
So there's a large space for democratic processes for collecting these seed cases as well. And once we have those seed cases, of course, we can continue operating on, say, something like Polis or Remesh to allow community members to continuously refine cases such that it would stay updated with their values and needs. And if there are any biases in the sample, they can try to mitigate that.
Yeah, that's a great question, Ray. So I agree with everything Kevin said. I think also, maybe zooming out a bit, I think that one of the ways that I also think about bias is that unless we democratize things and give people a chance to provide their inputs, it doesn't really matter what policy we write.
No policy, we can't possibly, like any time there's people who have mutually exclusive views, any particular policy will be viewed as bias as long as there's more than one person evaluating it.
So one of the ways that we can try to address that is to bring more people into the policy development process and also to make it more transparent how those policies were arrived at.
And also, one of the ideas that the Collective Dialogues team, so Colin, Erwin, and Andrew Kanya, and others have this idea of creating metrics around support for statements, not only for individuals, but also groups of individuals.
So if you group people by age, or by gender, or by geography, or psychographics, and you can kind of see what is the level of support for a policy statement within each of those groups, you can also have as a metric not just majority rules, but also we need to make sure whatever policy we go with has a minimum threshold of support among all of the demographics.
Or if there isn't that support, we can dig further and try to understand what it is about that policy that is causing such low support. So really kind of digging into the numbers and finding different ways to cut the data, but also ultimately creating a policy with other external folks' inputs, I think, is the best solution to not just coming up with a policy that people at OpenAI have tried really hard to write a good one, but never took external inputs, or there's a perception they didn't take external inputs that will always be perceived as biased.
Thank you, Roy. Excellent contribution, and it's really great to see you here tonight. Speaking of Colin, Erwin, he's had his hand up for a while. Colin, will you first introduce yourself to the group before you dive into your question? You're good now. So the mute, Colin, we can't hear you. There's a microphone icon at the bottom left center of your screen.
Okay, there you go. Try turning the volume up a tad on your laptop. We heard you earlier, so I know it works.
Okay, we'll let Colin figure that out. Colin, if you don't end up being able to figure it out, please type your question in the chat. But Colin was a member of one of the Democratic inputs to AI grant recipients, so I really hope we do get the opportunity to hear from him.
Dan Mack? Hi, thank you for taking my question. This is for Teddy. So I'm very interested in the moral graphing. It's not something I'd heard about before.
So I'm curious, what does the research look like on comparing the results of the moral graph to the embedded values within a large language model?
Yeah, the moral graph idea is from our Democratic Fine Tuning grant team. So I think that's actually where they are with their work right now is they've created a UI that allows people to converse with a chatbot on a potentially contentious topic, like I'm a teenage girl from a Christian or religious family, and I'm considering an abortion. What should I do?
And then a lot of people can have those conversations and say what values they think are important and why, and then also compare values cards at the end and have that create a graph for them specifically, but also aggregate across a lot of people to create a larger moral graph across a population of individuals.
And the idea is that this is something that can be used as a tool to help can be used as a fine-tuning input for the models. So that's where they are right now is trying to actually fine-tune models with a moral graph that they've generated through their process. And then to your question about comparing that moral graph against the inbuilt morals or tendencies of the model, that's a great question. I think that's a good baseline to set before you do the fine-tuning with a moral graph.
Now I'm just suggesting ideas. This isn't work that I'm currently actively involved in, and that team can speak to it better. But the idea is that they could basically set a baseline. There have been a lot of researchers that have tried to figure out how do these language models map against various value systems. And then they can fine-tune with that moral graph and then see how things have changed. I think in general, this idea of setting a baseline, doing a thing, measuring the new results, and comparing to the baseline is an idea of evals that's really important to what we're doing as well. That's probably a good idea for that team to try out as they explore that. Thank you, Dan.
Lucia, we'd love to hear from you. Thank you for your patience.
Thanks for taking my question. Hi, Kevin. Hi, Teddy.
So a question for Teddy. In the process of democratizing AI, where you're trying to get input from a large variety of sources, you mentioned it's not going to be exclusive to technical or expert groups of people, but basically everybody. What is your process for that? What is your criteria for determining who and how people get involved in that?
So using Kevin's terminology or that thought process, what are those key dimensions that you're looking at for the people who get involved in this kind of work? And I guess a follow-up is, how do you address or ensure that there's no biases that might tend to come up just in figuring that out?
So one of the things we're doing is we're starting with specific topic areas. So for example, how should chatbots respond to questions related to mental health?
And then we're having experts come and review a bunch of seed cases, cases that fall into that broad category, decide what dimensions are really important for deciding how the chatbot should respond, and then shifting into a phase where the public gets to also review those case examples and categorize into different types of questions.
And they get to opine on what outcomes and values the model should embody and vote on one another's inputs so that we have a stack ranking of that. And then finally, the experts reference those outcomes and values and draft-specific model behaviors.
So this approach unbundles the norm setting. So it's not experts trying to instill their own value system of what the norm should be. They might think, "Oh, you should always have the chatbot help the person as much as possible because there's a shortage of mental health expertise in the country." That may not be what they actually write the behaviors for because the public may say, "Oh, no, I just think, in general, we should not overrely on chatbots for this kind of thing."
So we're trying to separate the norm setting from the definition of the model behaviors. So that's one example of how we're trying to make it a little. And there's obviously, not obviously, but there is an iterative back and forth process, a dialogue between those two groups so that they can hopefully get to a place of agreement, or at least we understand when there isn't agreement reached. And so that's one example of how we think about it. I think that's a great question in general. I think it all comes down to just making sure also some of the meta elements of this, like sourcing diverse groups of people, making sure that we don't go with what's easy and we don't just go with our users. We also find non-users and ensure that we get their inputs. Maybe we sample.
a larger set of people and we sample in more creative ways. I was talking to the digital minister of Taiwan who does a lot of work with her team in Taiwan to basically reach people via cell phone numbers and tries to, you know, go knock on doors and does a lot of work to really make sure that the final sample they have is actually truly representative and not just, you know, ChatGPT users or whoever was reading their email and even has email. So a lot of thoughts there, right? It's definitely top of mind for us how we can make that minimize biases or at least ensure that we have representation in the process. Thank you. Appreciate that.
Yeah. Colin, were you ever able to get your mic settings working? Oh, that is such a bummer. We're going to have to host you again, Colin. I'm so sad that we didn't get to hear from you.
Well, we're at time, guys. As you noticed, the platform is telling us. Teddy, Kevin, thank you so much for presenting here this evening. I think that was the most engaged chat we've ever had for any one of these sessions. It's clearly a topic that we're all really interested and inspired to learn more about. I hope that you guys all enjoyed the evening.
I have a few announcements for you. Very soon, we'll hear from Cezary. Cezary is going to be hosting a roundtable called Collective Wisdom in Action Co-Creative Workshop Series so that our forum members can get together and decide the future of some of the programming in the forum. Give us some ideas about what you'd like to see, who you'd like to hear from, what you haven't been so thrilled about. We're really looking forward to hearing from you. I believe that that's going to be a lunchtime session. We're trying out a different time to see if more people can join us and provide their feedback.
If you're interested in learning more about what Kevin and Teddy talked about today, we do have a really beautiful blog post, Democratic Inputs to AI Grant Program, so you can learn a little bit about what we were thinking before the grant recipients learned that they were going to have the opportunity to engage in the project. Then Teddy and Taina later also followed up with a new blog, Democratic Inputs to AI Grant Recipient Demo Day at OpenAI. There's a link to all of the grant recipients' projects demos, so we demo them at OpenAI. If you're interested to see what all of the teams came up with, you can dig into that in the forum in the content section.
Also later this month, we'll be presenting Practices for Governing Agentic Systems. We hope you can join us for that. Many of you here have been supporting model evaluations at OpenAI. I recognize many of your faces and names, so thank you for that. If anybody else is interested in supporting OpenAI research, ensuring that our models are safer, please fill out the AI Traders Google form that we're going to drop in the chat right now. When your background fits the needs of a project, we'll contact you and we'll follow up.
That is all for the evening, guys. It's always a pleasure to host you all and to see your faces. I love seeing in the chat that you've been getting to know each other as well outside of the forum or during our in-person events. Colin, I'm so bummed we didn't get to hear from you. Please reach out tomorrow. I'm happy to do a Zoom call to help you figure out the settings on your microphone. Until next time, everybody, happy Thursday. Thank you for joining us.
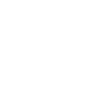