Democratic Inputs to AI: Grant Recipient Demo Day at OpenAI
speakers
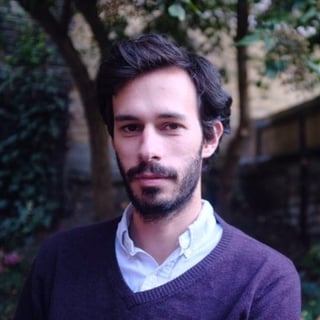
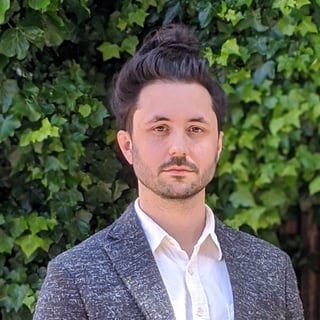
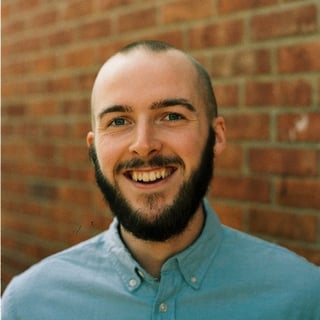

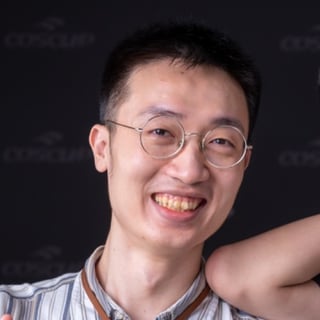
vTaiwan, g0v organization, Youth Internet Governance Forum, Taiwan Network and Information Center
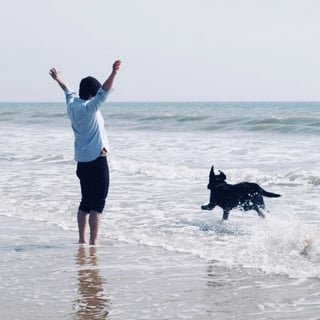
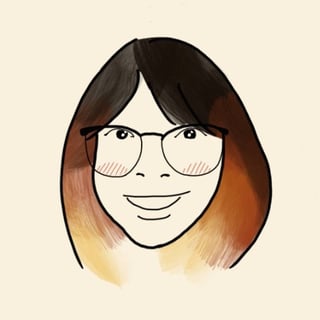
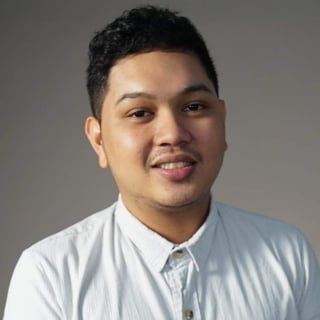
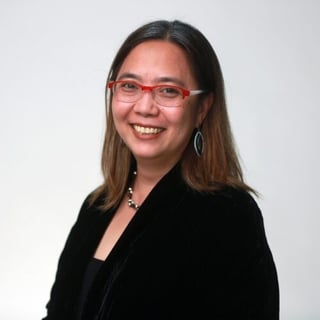
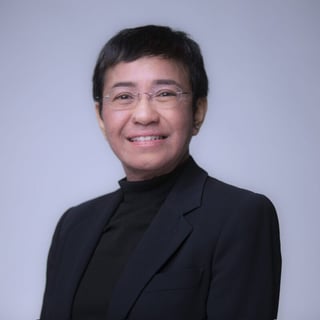
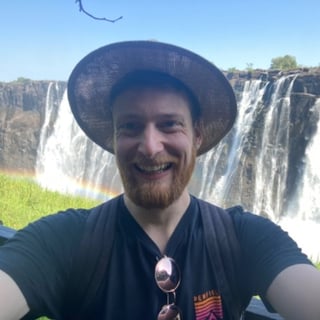
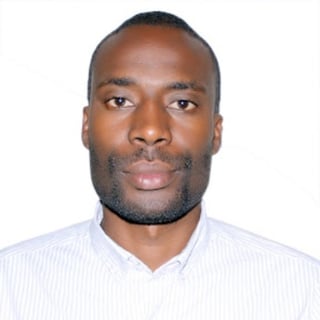
6 years software engineering +4 AI, data, ML engineering and democratic consensus building
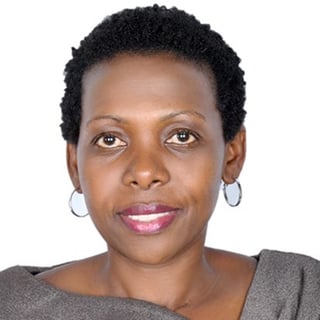

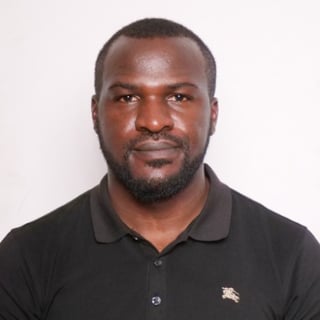
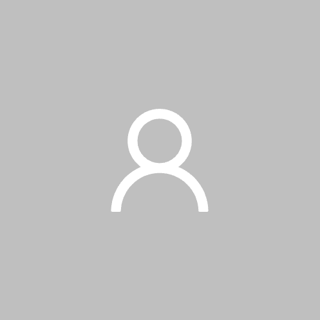
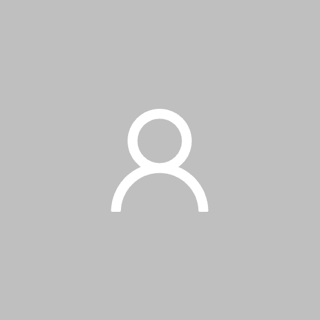
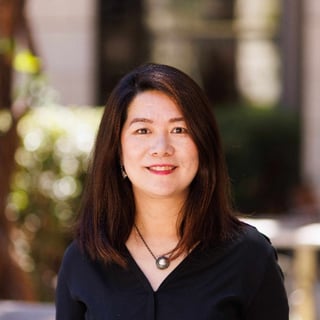

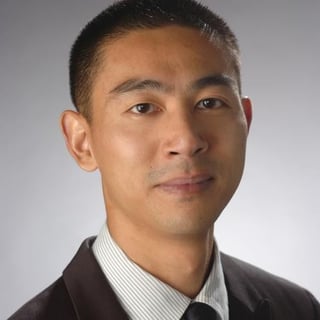

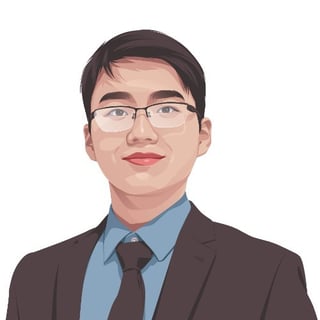
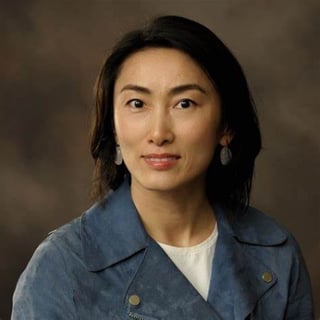
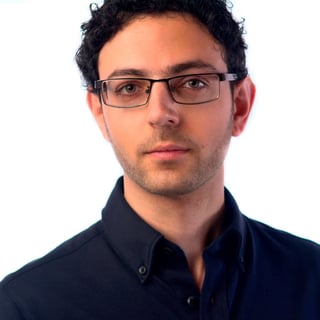
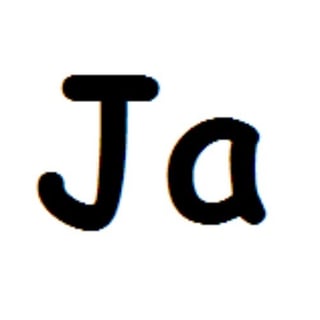
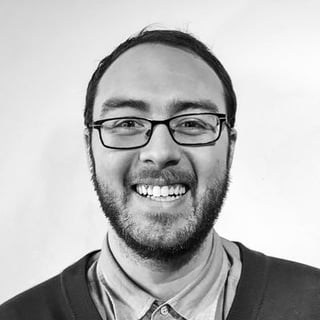
Founder/Chief Scientist @ Remesh. Working on deliberative alignment for AI and institutions.
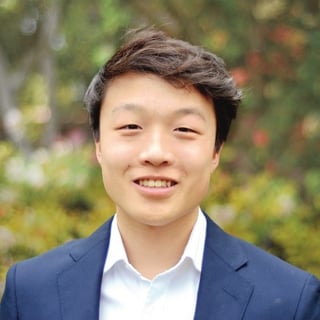
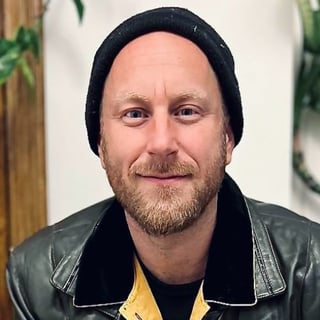
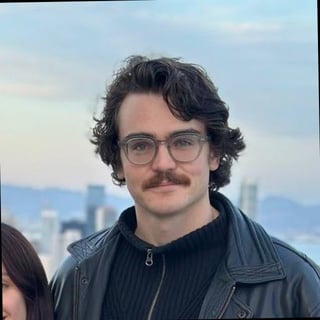
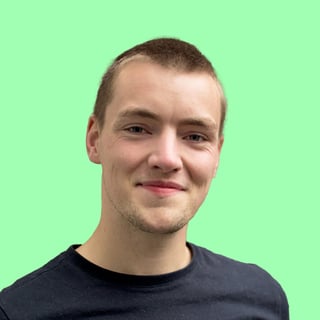
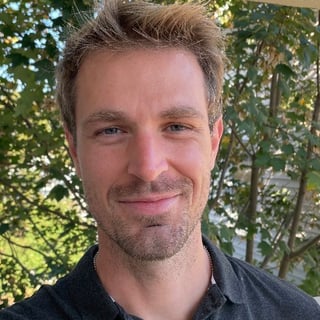
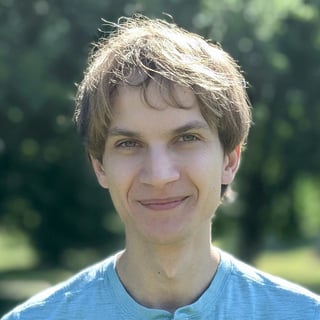
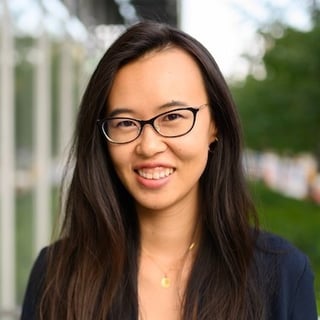
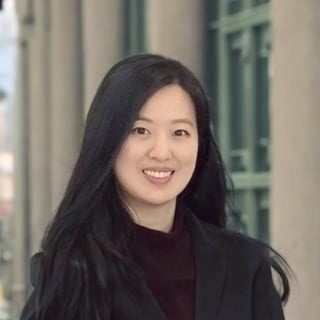
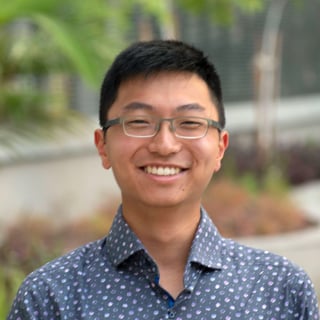
Kevin Feng is a 3rd-year Ph.D. student in Human Centered Design & Engineering at the University of Washington. His research lies at the intersection of social computing and interactive machine learning—specifically, he develops interactive tools and processes to improve the adaptability of large-scale, AI-powered sociotechnical systems. His work has appeared in numerous premier academic venues in human-computer interaction including CHI, CSCW, and FAccT, and has been featured by outlets including UW News and the Montréal AI Ethics Institute. He is the recipient of a 2022 UW Herbold Fellowship. He holds a BSE in Computer Science, with minors in visual arts and technology & society, from Princeton University.
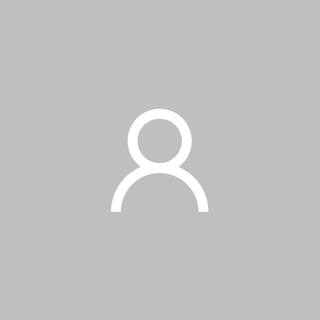
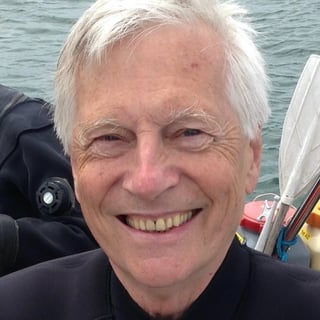
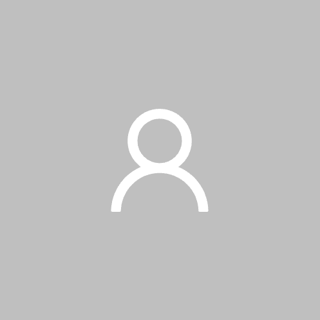
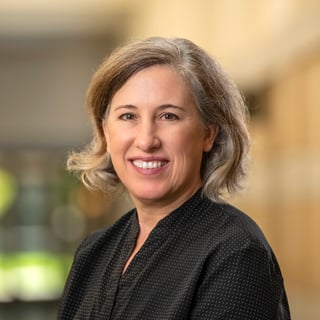

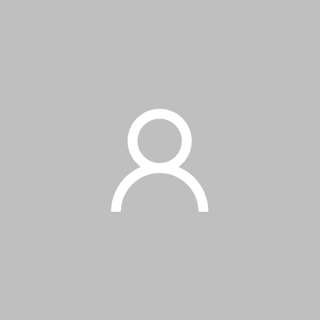
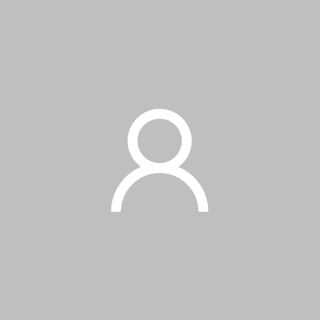

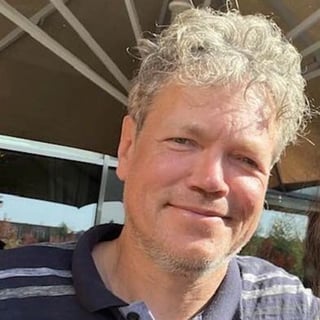
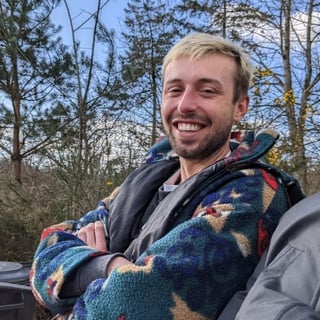
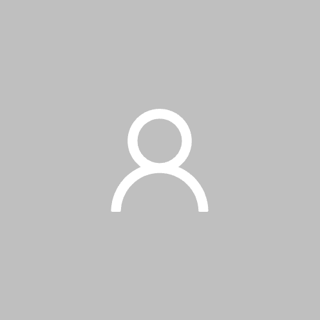
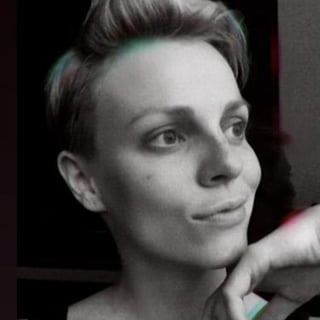
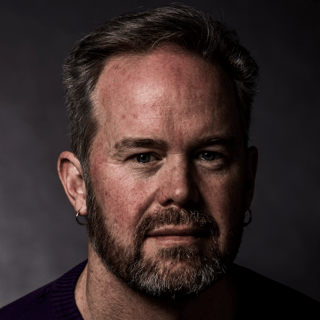
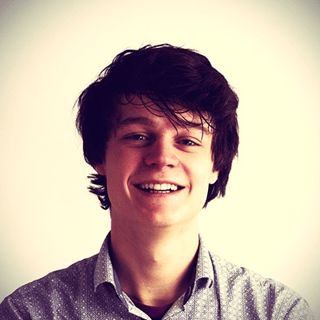
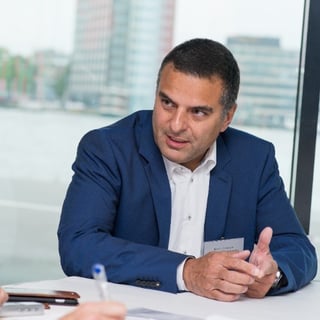
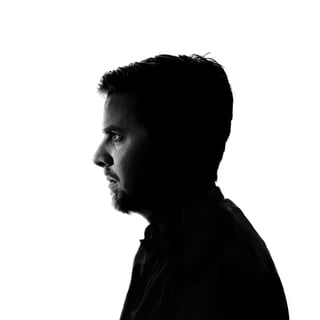
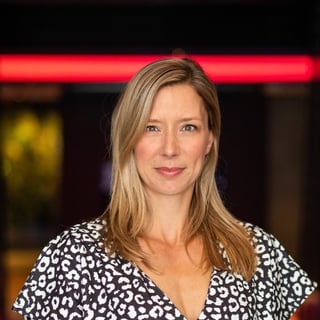
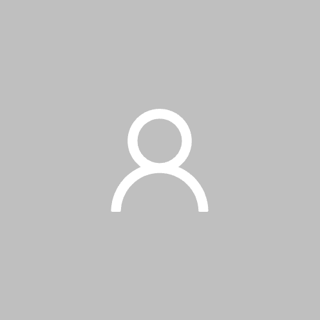
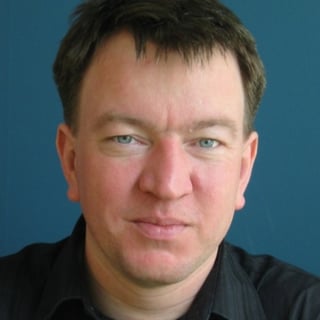
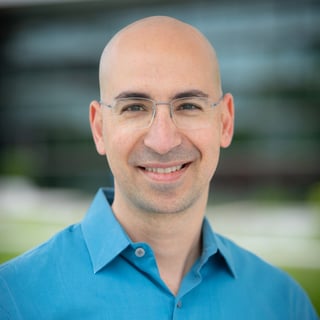
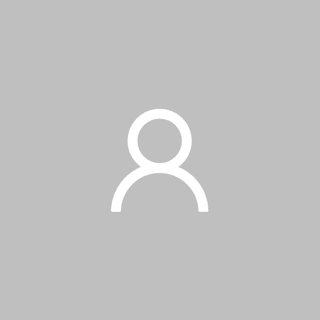
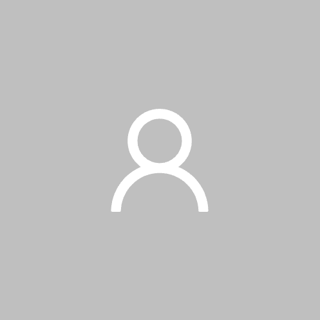
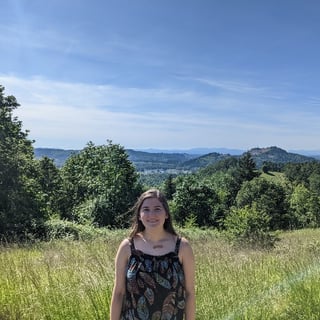
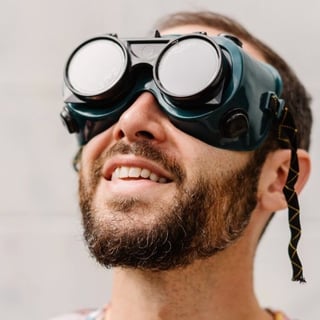
SUMMARY
Watch the demos presented by the recipients of OpenAI’s Democratic Inputs to AI Grant Program https://openai.com/blog/democratic-inputs-to-ai, who shared their ideas and processes with grant advisors, OpenAI team members, and the external AI research community (e.g., members of the Frontier Model Forum https://openai.com/blog/frontier-model-forum).
TRANSCRIPT
So I'll keep this brief because the bulk of the credit here goes to Tyna and Teddy for pulling together all the work and I also want to give a brief shout out to Wichex, our co-founder and Miles Breitberger, head of policy research, who championed this when this was just an idea. So, you know, I really can't say enough how impressed we are with the quality of work that's been submitted and as I go through it and review it, I'm just really struck by how much it drives home the point that this is a technology that's not just about productivity and helping people get things done, it's also about how we are going to treat each other and it reveals how we want to be treated and how we relate to each other and, you know, these tools can bring us scale, abundance, and intelligence but none of that really means anything unless we have the wisdom to make sure that it goes well. So thank you for your contribution to that effort and we're really touched by your desire to come together to help us make something special for the world and we'll do our best to make it count.
Thank you.
Hi, everyone. Welcome. I'm really excited to see all of you and meet you in person and not be constrained by Google Meet. I wanted to say a few words just to give you a little bit of context around why this work that you all are doing is important, not just to the world but also specifically to our mission. Here we're grappling with how to bring local context and values into this hyper-scaled technology. In our charter, we expressly make the commitment to build AI that benefits all of humanity, not just AGI, but with humanity's multitude of goals and ambitions, this is a tall order and our small part, my team's small part, and other teams is to measure how well we're doing on that goal and how we can do better. Understanding and reflecting diverse values will have to be an evolving and ongoing process. We're examining how our models align with human values today, are our algorithms inadvertently reflecting biases, are they suitable for all communities, or are they benefiting some communities more than others? How can we ensure that future models can be better aligned with society's evolving values? If we were tasked today with designing systems that reflect democratic will, how would we design them? Where should democratic processes fit into AI development? These are questions that we're grappling with, and we need to seriously question who has the authority and legitimacy to create such a system. It's not an easy question, and so that's why we've tasked all of you with solving it.
Many other teams, including mine, at OpenAI, are devoted to addressing some of these questions. Some of these teams include governance, safety systems, global affairs, trustworthy AI, among others. But we cannot do it alone. The work will become complicated and challenging, probably incremental at first, but it's vital if we're to create technology that serves everyone, from urban areas to remote villages. Part of this process is having both a vision of a positive future and a solid plan to get there, a plan born from relentless effort, experimentation and learning from failures. We are already seeing glimmers of what's possible from all the ideas that you're going to be presenting today. I've had enriching conversations with many of you around important questions like, when does representativeness matter, and how do you reach it for global-scale technology like AI or AGI? How do we draft a constitution or rules of behavior for models that are robust to edge cases and practically useful? How do we draft information packets for deliberation with a public that is highly uncertain about AI? How do we deal with the census? Many of these paths have been previously trod and on the subject of entire PhDs. We're not here to earn PhDs. I think some of you are
For you.
And so here I'm going to show, and this will be done in the form of an interactive tool. And here I'm going to show an example of the first part of this tool, which is making judgments on cases. And so here, as you can see, the participant is shown a case and they're shown several kind of response classes of potential ways that an AI assistant might respond to this case. And the participant can kind of indicate to us the level of desirability of these classes of responses. And they do this by dragging these cases into these classes. And a participant can also indicate indifference, so multiple kind of response strategies can fall into the same class.
Additionally, we want to engage participants to help us perturb cases, to help us generate new cases from the existing seed cases that we have in a way that's guided by the domain experts created dimensions. So here you can see an example of a rewriting of a case. So here we have a case, and we want the participant to help us create a new, synthesize a new case from this case. And what they do is they select kind of an expert created dimension, and they select kind of a level to, and prompt the AI assistant to help generate kind of a case. And they can also freely edit these cases.
And so as a part of this process, we want to prioritize recruiting a diverse population along several key demographic dimensions like political and religious affiliation, socioeconomic status, and ethnicity.
And so finally, the last phase is setting precedents on these cases. So up to this point, I've mostly been touching on generating cases in the case repository. Cases are just half of case law, and we really need judgments on cases to turn them into full-fledged precedents. Now, depending on the nature of the policy, one thing we can see is that through phase two, we already have some judgments. So the kind of easy solution is to use our judgments from phase two. But additionally, depending on the community, they might have kind of harder requirements for the democratic processes involved in making these judgments. And we make this compatible with our process by making this phase open to any additional strategy. So communities might choose to use a deliberative consensus process with a representative sample.
Finally, through these phases, we are able to kind of construct a case repository and decisions around the case repository that engage with a case law-based AI policy. And for the final bits, I'm gonna outline kind of two potential future directions for collaboration. One is the process of turning a case repository into concrete judgments. So here we see kind of an AI-based model which can take a new case, find and retrieve related cases in the case repository that are candidates for potential precedents. And based on these retrieved cases, it can discard cases that don't apply. And finally
Support it had within any of those segments of the population was 70%, so fairly well-balanced support across the population.
All right, so takeaways. Overall, we introduced a data-driven, democratic policy generation process. Specifically, we showed how we can take a scalable, deliberative process and use it to generate high-quality AI policy representing informed public consensus. We enabled this to happen at large scale by using collective dialogues, and we use GTP-powered tools to take a process that might take six months, if you're doing this in kind of a peacekeeping setting, and compress it to two weeks to happen in a place where we have a large surface area policy that needs developed.
Next steps. First is we want to either develop or adopt objective measures of policy quality. So if any of you have any of those, we'd love to talk. We need this because we want to optimize the part of our process that optimizes along the axes of quality. It's hard to optimize for a thing that you can't measure.
Second is we want to tackle more contentious issues. We were fairly pleased at the amount of bridging support that we were able to get so far, but it's unclear if that's because we simply chose a policy issue that just wasn't that contentious across, say, political party lines. And so the next ones we're going to focus on are those which are maximally contentious to see if the process is robust to those types of policies.
Third, and maybe most importantly, is we want to scale to global representativeness. As Taina and Teddy said, the goal here is we don't want to align AI with the US population. We want to align it with humanity overall. And this is actually quite a hard problem, but we figured solve the easy parts first and do it with the US and now figure out how to solve
Yeah, that's a great question. So far, we have been reaching out to different communities to ensure a diverse range of perspectives. In our user base, we have tried to include people from different backgrounds, ideologies, cultures, and income levels. However, we recognize that this is an ongoing effort, and we are constantly working to improve and expand the diversity of our user base. We also welcome anyone who is interested in participating to join our platform and contribute their perspectives.
I want to ask the next question. Thanks.
Yeah, sure. I mean, I think that the grant recipients in general can be binned into three categories. So there's people who work on cases, like the case law group, people that work on representation and participation, and then people that work on aggregation or the actual social choice process. And we're kind of in group three. So we'd be very happy to work with other groups that focus on who should be represented and what cases we should take on.
Jason on the chat asks, how do you know that the process works well across cultures and locales?
Yeah, I mean, so far it's very early. But one of the things we've watched is how people talk with the chat bot. I think this is the hardest part. And also what the degree of convergence is on the, when people say this story is plausible, this person got wiser. Are people just clicking random things? There's also a place where you can say why you think that this person got wiser. So we pay attention to those. And what we're finding is that people are able to articulate their values from a very wide range of backgrounds. And they're able to assess these stories from a very wide range of backgrounds so far. I'll mention when I tried it, it felt very natural to just answer and talk to the chat bot about your own life experiences. I thought that was very nice.
Thank you. We'll move on to Jorm, who will talk about deliberation at scale.
And here we're moving into our group that I'm calling it the AI-enabled deliberation, although obviously a lot of teams are using that as well. First off, it's super inspiring to just be here and listen to all your presentations so far, I guess, and your presentations coming. Yeah, the work that's being done here is really amazing. And what you talked about with the three bins, the generative social choice stuff and the social choice stuff, generally speaking, is really inspiring because I don't have any idea about how to do that kind of stuff. We're deliberation at scale. We are a very large consortium based mostly in the Netherlands. And we are trying to understand how to make deliberation possible at a very large scale, but making sure that it's open-ended, that it's iterative, that it's conversational, and that fundamentally that it's empowering for the participants that are involved. So I'm just going to show you a quick video of what we've built so far. So in our first iteration, we have a web app for deliberation at scale where people can just sign in and where they are matched
Deserve one of the statements as a proportional share. And such that they don't like the statements that we chose, but there is some statement that would unify them, one statement that they could all agree on. This is something that has to be ruled out, and we can never produce such a slate. This is what justified representation says. And actually, Manuel and Ariel were among the first people to point out this connection to selecting representative statements. But this is classical social choice theory. So for that, we don't need any generative AI.
What do we get in addition by using these techniques? Well, this comes down to what I've indicated to you in this thought bubble, this idea of this coalition shows that we messed up if there is some statement that gets them together. But in classical social choice, this is actually a relatively weak condition, because the statements that might get them together all have to be specified up front. And so they're a small set of statements. Everyone votes on them and already indicates whether that unifies them or not. So this is a relatively easy condition to get around. It would be much harder if we were aiming to get justified representation, but with respect to all possible textual statements that might get people together. And this is what we use generative AI for. We use the LLMs in two different manners, in a generative manner and a discriminative manner. The generative manner says that, given a specific coalition, try to find one of these unifying statements and tell me what it is. And then we also use it in a discriminative manner, which, for example, is exactly the same way that Andrew has been using LLMs. We build a process that is based on traditional social choice techniques, but then also uses large language models as a subroutine. We can show that, if these calls to the subroutine actually adhere to our specification, in that case, we would be able to give this guarantee of justified representation in this enormously challenging domain, where all unifying statements have to be considered. And we actually recently put out a preprint on this. And now Manuel is going to talk about what we've been thinking about since then.
All right, so yeah, as Paul mentioned, here we want to extend the notion of these binary approvals to multilevel approvals. And one reason we are doing this is because what we observed experimentally is that, if you just have two levels of approval, then by the way you ask the question, you kind of set a threshold on what does it mean to approve, what does it mean to not approve. So you can think of this this way. So here, these boxes correspond to different levels of approval. And everyone who is inside the box would approve. This would just be for one given statement. And everyone inside the box corresponds to the people that would approve of that statement, if
different processes, and we're interested to see if you could make a kind of infrastructure that would allow kind of cross-collaboration, cross-comparison between different processes happening with different audiences, and kind of really connect up all these places.
And so a couple of different results that we've got so far, and all of these processes are kind of at different stages, so like some are in digital, some are in face-to-face, but for example here's some kind of demographic data about the international cohort we're working with. It's pretty kind of diverse and buried, and as you can see here's just a couple of examples from the kind of agenda-setting question we put out, and so there is actually really quite high consensus in this. It's interesting, and you can see kind of high consensus points around, for example, mixed stakeholders in kind of global AI regulation conversations, and then much more divisive statements go towards the kind of pursuit of superintelligence, or a focus on like existential risk, for example.
And you can kind of see here, you can see points of high consensus, you know, more divisive comments. Big shout out to Colin, who is here today, obviously kind of leading on POLIS.
A bit about Taiwan. For Taiwan's cohort, you can see that Taiwan's POLIS results are just like that. Some people, some results and some POLIS statements actually have more consensus on, but people also have very divisive consensus on maybe like bias and discrimination issues. When chat GPT is being used on the comedian to writing jokes about LGBTQ groups, people have very divisive views on this kind of statement.
And so that based on these statements and results, we actually hold this kind of face-to-face consultation meeting, and you can see that this is a demographic of this consultation meeting, the gender ratio, and also, sorry about that, and gender ratio and the backgrounds. We actually cover a plenty of backgrounds, from aboriginal people, new immigrants, communists, to the public sectors, including the self-man, public sectors, agencies that govern AIs and AI-related issues in Taiwan. Yeah,
Thank you for your feedback and suggestion. We chose to focus on Africa because we believe it is important to represent and uplift African artists and their work. However, we appreciate your idea of exploring cultural artifacts and value systems of ethnic minorities in China through video blogging. It could indeed be a valuable avenue for cross-cultural creation and understanding.
Speaker 1: this minority to other cultures or cross-cultures, yeah. Ultimately, I think we do want to have a model that can be applied to any knowledge domain and in any geographic place, but we wanna make sure it's actually anchored in the folks who are producing the value, right? And not just the people, but also the ecosystem, right? The non-humans who are producing the value. So I had an example up there of the basket from Ethiopia, and I actually used an image of a basket that I watched being created, and she was wrapping this beautiful thread around some grass, but that grass is now under threat by climate change, right? And so one of the things we've been talking about is now adding in the ecological domain into this and trying to get that data onto the system in some way that's beneficial. Thank you so much, it's an amazing project.
Speaker 2: My question is a bit, it's a nasty question, and it's how do you stop bad faith actors just stealing all the data? So, as Sam mentioned the magic word here, which is NGO, so we're not working one-on-one with individual artists. There's no way that an individual artist can just go onto the website and start uploading things. And we realized early on that if we were trying to vet millions of artists, they would just overwhelm us. So we're contacting NGOs, so these are non-governmental organizations, whether it's a small studio of artists, or Nigeria has just started a new institute for African artists. So whether they represent just a small number or a very, very large number, we're working through them, and they're the ones who are gonna make contact with the individual artists, right? So essentially we're outsourcing our vetting process.
Speaker 3: One last question, thank you. Have you thought anything about distribution strategy for this, so as to meaningfully rival Amazon Turk or other expected alternatives, scale-wise? I'm not sure what you mean by distributions.
Speaker 2: On the input side of folks?
Speaker 3: On the input side, yeah. So as far as I've understood, part of the goal here is to kind of create a data-labeling data set that could be used to do something like what people use Amazon Turk for. So on the one hand, you wanna do a process that is grounded, right, and connected in some way to the real world that has a kind of authenticity to it. On the other hand, you wanna do this in as large a scale as possible, if it's actually gonna be beneficial. But those two things have to be in tension with each other. You can't just go off in just one direction. And so I think the challenge of doing it with something like NMT is, well, now I'm not necessarily working through an NGO. I've just got this solicitation out there, and how do I know that that's actually an African artist who's applied, right? Thank you. Thank you. Thank you.
Speaker 4: Okay, so I'd like to invite Tanu Shree and Yang to come on the stage to talk about inclusive AI, how they're thinking about engaging marginalized groups for AI.
Speaker 5: So we're representing our team, Inclusive AI. I would say one of the main motivations for this work is that in the past, we've done work with a large group of underserved populations, people with disabilities, youth, and people from the global south. So really, the motivation is how can we better engage these underserved populations in these sort of democratic decision-making process? Our team is from three universities, and many of us are here, so save your hardware questions for them during lunch.
Speaker 4: Okay, so in this project, we were inspired by the current sort of practices of decision-making in these decentralized autonomous organizations, or DAOs. I would say, specifically, our main contribution is to explore, develop, and evaluate effective decentralized governance mechanisms. So for instance, different kinds of voting mechanisms that can better engage these diverse underserved user populations at scale.
Speaker 5: So what I mean by these sort of voting schemes, so let me give you two concrete examples which we believe can potentially give more voices to these underserved population. So the first one is the voting method, which is basically what you would use to calculate votes in the voting process. So traditionally, if you have one voting token, that equals to one vote. But this is challenging for underserved populations because oftentimes, they're less resourceful, so it's harder for them to gain a large amount of voting powers. So basically, their voice were silenced by whoever has a larger amount of voting power. So under the scheme of quadratic voting, basically, the number of votes equals to the square root of the voting token that you place. So for example, if someone has four voting tokens, instead of having four votes, this person only gets the square root of four, which is two. So this is one way to give emphasis to the number of voters rather than the size of the voting power. So this is one way to give more voice to the underserved populations.
Speaker 4: Another example is sort of how do you distribute these voting tokens to begin with? So traditionally, you can imagine that every individual in the community gets the same amount of token. So the challenge with vulnerable groups where underserved population is that usually, they're the minorities. So if every person gets one vote, then they're
method for different group of peoples. And that's kind of based on the literatures. And the second one, the ranking choice voting, we use the weighted choice as a proxy. And this is about the electoral vote in normal elections. So these are the two voting methods that you consider as our first treatment condition, as our first phase of experiment. But there could be a lot more. If we do so, that would incrementally increase our treatment condition to 16, which is not possible within this scope of time. That's why we choose to do with the most popular two voting method.
And I just want to quickly add that you can also think about how do you actually implement the different factors, like token distribution. Right now, we're only considering at the get-go. We're going to do 80-20 split. But you can imagine that when we start, everyone gets the same amount. But maybe as they participate in this process, they're going to earn tokens. So then, they're going to have a differential token distribution. So that will be a variant of how do you implement something that's different for everyone.
Is this helpful for a cell and a data structure? OK. Or is it just ways of getting voting power? That's interesting, yes. Incentive mechanism has been widely researched in online community and, again, in the blockchain community as well.
Now, the thing is, are we trying to creating new technologies? Or we are going to explore the existing technologies to see if there is applicability of the blockchain-based application in the AI context? So we are not reinventing the wheel. We are trying to see if this really has a value in AI context. And we'll get to see some of the results when the large-scale study is done. So hoping for the best. Thank you. Thank you again, Tanushree and Yang.
OK, so we actually have a bonus presentation. You might be asking yourselves, how would we even develop these processes? Or how do these teams collaborate? Well, I want to introduce one of our advisors, Aviv Ovadia, to talk a bit about some of the thinking and work that he's done. So to introduce Aviv a bit more, Aviv has been a leader on the impacts on democracy of generative AI, long before it was even called generative AI. And he's focused much of the past few years on supporting companies in implementing democratic processes for their decision making. Aviv recently co-founded the AI and Democracy Foundation, is an affiliate at the Center for Governance of AI, and a research fellow at the New Democracy Foundation. He'll take the next few minutes to talk about the process cards and the process hub. And then I'll say a few more words, and then we'll go to lunch. Welcome, Aviv. Thank you. Thank you. Thank you.
Hello, everyone. I'm going to jump right in. So this program could have been developed as you could have just had teams developing their own democratic processes in complete isolation, and they submit a report to OpenAI at the end, and we're done. But the question is, could we do better? Could we increase the likelihood that the processes developed here could be adopted within an organization, and specifically a frontier AI lab? Could we accelerate a global ecosystem of democratic innovations beyond just OpenAI? Could we help people build upon each other's work?
So in order to support adoption by labs, we need a standard way to describe the processes being developed just as we have with machine learning models. What are the inputs and outputs, the side effects, the evaluation criteria of these processes? Where do they fit within an organization's existing decision-making processes? This is the kind of information they need to know to determine where those processes can actually be used. And that same information is also needed to help ensure people can build on each other's work and use each other's processes.
So I've been developing concept of process cards prior to the grant and introduced them to the program to help address those goals. So we asked the teams for cards for the main processes that they've developed and also the sub-processes. We also had them provide or having them provide run reports to understand what happens with particular pilots of the processes they're creating. And all this is currently managed in a process hub, which enables comparison and exploration across teams and is currently implemented in Notion, which is imperfect but works.
You can see the different parts of that hub here. It's helped us identify points of collaboration, evaluation, and adoption, and even where some processes might be swapped in for others across different teams. My understanding is that many people who are watching externally in OpenAI may have started to get access to the process hub. I want you to keep in mind, these are technical documents, just like model cards. And they weren't meant to be polished by this time a few weeks from now. And so it's going to be a little challenging to navigate if you just jump in. But it is a place to look at some of the details of the processes and how they might interoperate and interconnect.
And for more context on this, there's a public post on process cards and reports, even moving forward process benchmarks, where to think about how we can build this ecosystem going forward. And it's far from complete. There's a lot to do in enabling a vibrant ecosystem of democratic innovation for AI and with AI. And just to tease that as an example, we want to know, what are those decision points? What are those buy-in needs? Where you can actually slot this into processes within a lab or beyond? And so a taxonomy of that is obviously invaluable to put into process cards, and which can be referenced when someone is actually making a decision or trying to figure out what they want to use within their organization.
And finally, I'll flash onto the screen for one second the actual hub. So yeah, this is very briefly. You're in very quick skim. There you go. I don't expect anyone to be able to see very much here, but you can see specific examples. Yeah, and if you want to start, I would just start there, which is just a very high-level overview. Reach out to me for any questions or feedback.
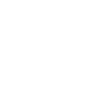