Deciphering the Complexity of Biological Neural Networks
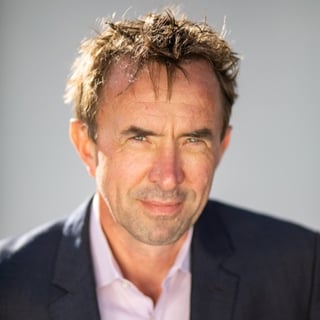
My laboratory at The Scripps Research Institute (TSRI) is dedicated to understanding how neurons in the mammalian brain form their synaptic networks, and how these networks are reorganized during memory encoding. We approach these questions using mouse genetics, deep sequencing, biochemistry, optical imaging, three-dimensional electron microscopy (3D-EM), electrophysiology, and behavioral studies. Additionally, we are developing new methods to access and manipulate specific neuron types in brains of model organisms with small molecules, as well as methods for AI-based analysis of brain structures in 3D-EM volumes. I have been studying neural circuits for over 20 years, as a postdoctoral fellow and as an independent NIH-funded investigator. I have successfully mentored many young scientists, several of whom now hold faculty and postdoc positions at other universities, serve as medical doctors, or work in biotech companies. My group is part of the vibrant and collaborative local neuroscience community, which includes investigators from TSRI, UCSD, and the Salk Institute.
In our conversation, we explored the fundamental principles of organization and function of biological neural networks. Anton Maximov provided an overview of imaging studies that have revealed the remarkable diversity of neurons in the brain and the complexity of their connections. His presentation began with the pioneering work of Santiago Ramón y Cajal and extended to contemporary research that integrates advanced imaging technologies with artificial intelligence. He discussed discoveries from his laboratory at Scripps, unveiling surprising new mechanisms by which neural circuits in the brain are reorganized during memory encoding. His presentation was engaging, with vibrant videos and images to showcase his findings.
So, I'll introduce myself. My name is Artem Trotsky. I'm one of the OpenAI Forum Fellows, and I'd like to start my talk by reminding us all about OpenAI's mission.
So, OpenAI's mission, which is to ensure that artificial general intelligence, AGI, by which we mean highly autonomous systems that outperform humans at most economical, valuable work, to all of humanity. And welcoming you to the AI Forum tonight.
We are really excited to learn from Scripps professor, Dr. Anton Maximoff, who will present and discuss integrating AI and advanced imaging to unravel the mysteries of brain connectivity.
So, before Dr. Anton gives us a very exciting talk, I'd love to give a little bit of a background on the speaker tonight.
So, at Scripps Research Institute, Dr. Anton Maximoff's laboratory is dedicated to unraveling the mysteries of neuronal synaptic network formation and reorganization during memory encoding in the mammalian brain. They approach this by looking at mouse genetics, deep sequencing, biochemistry, optical imaging, 3D electron microscopy, and even behavioral studies.
With over two decades of experience, Dr. Maximoff has studied neuronal circuitry both as a postdoc fellow, as well as an independent NIH-funded researcher. His mentorship has nurtured numerous scientists, many of whom have become faculty or postdoctoral scholars in academia, medical practice, or even biotech.
Dr. Maximoff's group is an integral part and dynamic and collaborative local neuroscience community, and we look forward to hearing more from his talk.
So, I ask that I will now share the screen and pass the baton over to Dr. Maximoff.
All right. Can you hear me okay?
Perfect. Hi, everybody. Thank you, Artem, for this very nice introduction. It was very detailed, and it's really a pleasure to be here. It's a very, you know, exciting time, and I'm happy to be a part of this forum. I would like to say something obvious. I have a strong accent, so if you guys don't understand what I'm about to say, please feel free to stop me, and I'll be answering questions, happy to answer questions during the presentation.
And I would like to start with saying that we live in a very exciting time. So, AI is literally changing the world quite rapidly. It's changing our personal lives in many different ways. Just yesterday, when the coordinators were helping me with setting up the slide deck, I shared a story with them how I, you know, had a lunch with a group of friends, and I happened to sit next to a priest, and it turns out that he is not only passionate about AI, but he's very proficient with ChatGPT, and he is using ChatGPT for speech writing, which was a great surprise to me. This is sort of one example of how, actually, people use these technologies for applications that may not be obvious to others.
Of course, some of us are also anxious about the future. The future is going to be exciting, but it also brings a bit of anxiety. As a scientist whose job is to make predictions and test them experimentally, I wonder what's going to happen to my life and my professional life when AI becomes a reality. But at the same time, I would like to remind you that the human brain remains the most sophisticated and powerful computer that is known to mankind, at least in the present time. It has a remarkable capacity to store information. The estimated capacity is in petabytes. It is also remarkably complex in terms of the numbers of neurons. The counts are in billions, and also numbers of synapses, so they're in trillions. And of course, this number is a very vague estimate. The reality is that we don't know exactly how much information can be stored in the brain, just like we don't know how many synapses and neurons actually lie in the brain. So if someone tells you that they know exact numbers, please don't believe them. We also don't know exactly how information is stored and retrieved by biological neural networks, so there is one principle that appears to be applicable to both biological and artificial systems, and that is long-term storage of information requires some sort of a physical or chemical change in the carrier. This principle applies to our DNAs, this principle applies to computer hard drives, and there is increasing evidence that this principle applies to biological neural networks.
So our goal of my laboratory is to understand how neural networks are organized. We also want to understand, and this is actually particularly interesting to us, is how these networks are reorganized as we actually learn and remember. And to give you an analogy, we already know that neurons and their synapses in the brain can actually respond and be altered by external environment in many different ways. But many of these changes appear to be transient, as exemplified on this little drawing. So it's easy to draw on the sand, but this information is very unstable because the system has high entropy. At the same time, this Egyptian hieroglyphs have lasted for thousands of years. It's actually harder to engrave them on the rock, but the information is quite stable.
So what we're trying to understand is neural equivalence of these engravings. We want to understand how they're generated. We want to understand what does it actually mean for information storage, and how they progress and how they change. For example, our memories are obviously not nearly as stable as these engravings. They're vulnerable, they can be extinct, they are affected by neurological disorders, stress, and many other factors. For example, how our ability to perceive and store information changes during aging is very poorly understood, and we hope through this research we can also get some insights to that.
So imaging is one of the techniques that we use extensively. Before I tell you a little bit about advancements of contemporary neuroscience, in particular imaging, and also specifically work in my lab, I would like to say that if you attend a number of neuroscience talks, in particular talks that are actually focused on brain wiring, there is a very high chance that you'll hear about the work of this gentleman, Santiago Ramón y Cajal. Cajal is considered the founding father of contemporary neuroscience, and there is a reason for that. His discoveries actually not only impacted the field for the next hundreds of years, but they're still relevant to this day. This is a picture of Cajal in his lab. He used a very simple microscope, in fact there are a few microscopes depicted in this picture. And what he was trying to do, he was trying to actually image or look at human tissues that were stained in a way that brain cells can be labeled sparsely. And just to sort of exemplify what kind of technologies are available to us now, this is a picture of a modern microscope that costs about a million dollars.
As you can imagine, the Cajal's microscope was not equipped with any digital cameras, in fact no cameras were available. So he was not able to digitally record what he observed. And to document his observations, he had to draw by hand. And what helped is that he was actually a very talented artist. This is an example of his drawing, which is actually remarkably detailed. I have to say that one of the reasons why he was able to make this discovery is that the staining procedure of human tissues that he's used worked in a way that cells were stained sparsely. Neural tissues are very densely packed with neurons, and if everything was stained, he wouldn't be able to see these beautiful details. But because of sparseness, he was actually able to see and also draw individual neurons, as shown in this picture.
Now, many of these pictures are featured in museums across the world and some research institutes like MIT. And these pictures are incredibly informative. So for example, these pictures immediately tell us that neurons have very complex projections. They tell us that they also seem to be polarized. And if you look closer, you will actually start noticing that there are small protrusions on every projection. These protrusions are called dendritic spines. So these are actually sites where synapses are formed. And these are the sites where neurons communicate with each other.
Now, before Cajal's work, it was generally believed that the brain is actually a continuous net. Here after making his observations, actually made a very important groundbreaking prediction is that the brain is actually comprised of discrete cells that nonetheless connect with each other via synaptic contact. And this was not the only important prediction. So by itself, this prediction was absolutely transformative. But he also made another prediction. By looking at neurons in different parts of the brain, as shown here, and in fact, you can actually immediately realize what the conclusion was. He started to realize that not only these neurons are very complex, but also that their morphologies are different in different parts of the brain, and even within the same region. And this was also a very important discovery that essentially highlighted the diversity of cells in the brain. So now we know that there are virtually thousands of neurons in the brain.
Speaker 1: Of different neuronal types, the cells actually are remarkably diverse in terms of their morphological features. They release different neurotransmitters. They have different functional roles. And this diversity is largely attributed to the fact that these genes actually, these neurons, express different combinations of genes. So this combinatorial expression of different genes actually define their morphological properties, their neurotransmitter identities, and ultimately their functions.
Speaker 2: So yeah, and this was absolutely instrumental. Now, the bulk of our knowledge about the brain wiring, so which was accumulated over the past 100 years, actually is based on research in multiple model organisms. So the common model organisms that you may actually hear about if you, again, learn about neuroscience, basic neuroscience research, C. elegans is a small worm. It has a very simple nervous system. In fact, we actually know the exact number of neurons in this worm, which is 318. And although the nervous system of this worm is very simple, this model system actually permits us to do a lot of genetic research quite rapidly, because the lifespan of this worm is only two weeks. In fact, this model system actually became very popular among those who study aging, again, because they have a very short lifespan. So you can actually examine the effects of genetic mutations or environmental factors or drugs on multiple generations relatively quickly, as opposed to humans. Something like this would be very difficult to do in a human.
Speaker 3: Then another popular organism is Drosophila mice, which is the organism that we like and use in the lab, and of course, the human. So now, all these organisms have their own advantages and their own disadvantages. We like mice because they're accessible, so we can actually access their nervous system. We can also do genetics. Well, some of you may actually think that mice are quite primitive. And you have a point, but there is also evidence in the literature that mice actually are not only very sophisticated, but in fact, they rule the world, as shown here in this novel by Douglas Adams. I'm not sure if you guys read the novel, but the conclusion there is actually very interesting. So the main character at the end of the story realized that the mice ruled the world. Well, of course, this is a joke. But again, as I mentioned, we really like this model organism because it really allows us to do experiments that are not possible to do in a human for either technical or ethical reasons.
Speaker 4: Now, of course, research with mice has limitations. And one of the limitations is that they don't talk. I would like to share a story with you to sort of exemplify how something like this actually complicates our life. So when we study memory, the studies of memory in mice actually prompts us to develop all kinds of assays that sort of assess the ability to learn and remember relatively indirectly. And to exemplify this, I will share a story about, it's actually a personal story. So my wife's grandmother passed at the age of 94. She had a great life. She was actually quite healthy until the very end of her life. But unfortunately, in her late 80s, she started to develop memory loss.
Speaker 5: And this condition was progressive. This condition was actually really impairing her ability to have short-term memory and also remember the recent events. So she could actually remember the events that took place in the distal past, but she wouldn't be able to form new memories. And in fact, when my wife and I were already engaged, I had to be reintroduced to her grandmother over and over again because she would remember who I was when we stayed in the conversation. But if I leave the conversation, she would immediately forget who I was. And what this tells us is that our knowledge about memory loss in humans is actually largely based on conversations. So mice don't talk, and this is a limitation of the system.
Speaker 6: And what I would also like to highlight is that our knowledge about the organization of the brain, about the structure of neurons, about the function of neurons, our current knowledge, is based on a variety of approaches. So contemporary neuroscience leverages virtually every branch of biology, ranging from biochemistry, a technique that allows us to isolate proteins and look at the interactions, molecular biology. So we manipulate genes, manipulate genes to understand their function, and also manipulate genes to actually develop tools to access, label, and manipulate neurons in the brain. We learn from structures. We learn from cell biology of neurons. And of course, recently, the advancements in chemistry and computer science, and particularly in AI, have been absolutely instrumental for contemporary neuroscience.
Speaker 7: And of course, as I mentioned already, imaging remains one of the most important and powerful tools. Contemporary imaging techniques allow to image neural circuits in individual synapses at different resolutions, as exemplified here. So what you see on the left is actually an image of the mouse brain, where different types of projection neurons are labeled and imaged. You can appreciate the complexity of connectivity between the cells. And so oftentimes, they project the axons far away from where the somas are located. And the opposite side of the spectrum is individual synapses, as shown on the right side. So these are structures that actually are individual units of information processing. These are structures that allow neurons to communicate via chemical signals. And they're also remarkably complex.
Speaker 8: I was fortunate when I was a postdoc to be a part of the research group led by a famous German neuroscientist, Tom Sudow, who is currently at Stanford, actually. Well, when I was a postdoc in Tom's lab, he was still in Dallas, Texas. And so Tom's work is widely recognized. In fact, he received the Nobel Prize in 2013 for his work on mechanisms of neurotransmitter secretion. And I would like to very briefly tell you about how synapses operate for the purpose of this talk. So what's shown here on this image on the right is actually a structure of individual chemical synapse. And what you see at the top of the image are small circular structures. So the structures are called neurotransmitter vesicles. So these vesicles store neurotransmitters. And they're able of rapidly releasing those neurotransmitters to the extracellular space in response to external stimuli when neurons become excited.
Speaker 9: Upon release, neurotransmitters diffuse. And then they bind to receptors that are located on the opposite side of the synapse. So these receptors are located in the so-called postsynaptic neuron. And this allows neurons to rapidly transmit or convert the chemical signal into electrical signal. Because by binding to receptors, neurotransmitters produce an electrical signal in the downstream cell. So this process is very efficient. So in fact, the so-called exocytosis of neurotransmitter vesicles that leads to secretion of neurotransmitters operates on a scale of hundreds of microseconds, not even milliseconds. And this process is also very precise. And needless to say, this precision is absolutely important for every aspect of neuronal function. So we wouldn't be able to move, we wouldn't be able to learn, we wouldn't be able to actually perceive the external world if synapses were not operating with such precision and speed.
Speaker 10: And the type of imaging that we are particularly excited about, the type of imaging that we extensively use in my lab is called ultrastructural imaging. What I would like to tell you now about is a research in my lab that is focused on understanding how neural circuits are modified during learning. And I would like to say that this work would not be possible without terrific collaborators at the University of California of San Diego. This group of collaborators are led by Dr. Mark Ellisman. So Mark is considered the founding father of contemporary imaging. So his contributions were numerous and widely recognized. And in my lab, the young fellow who actually leads this research is Mark Utsepa, who is actually currently here with us in this audience. And Mark has become very passionate about neural circuits. But he's probably equally, if not more, passionate about AI. And I will tell you a little bit more about his work in a minute.
Speaker 11: The technique that we use is called serial electron microscopy. So this technique allows us to actually take a chunk of brain tissue and then generate a series of images. And these images essentially capture every structure that can be resolved. So to give you an analogy, if you decide to actually understand the organization of a forest that you never knew about, so this technique allows you to recognize every feature, every tree, every leaf, every piece of grass, and so on and so forth. So it's incredibly powerful. And you produce terabytes of data. And this data is incredibly rich in terms of the details. Now, this technique has been conceived about 15 years ago. And when it was conceived, there was actually a lot of excitement. Because people in the field started to realize that it could be actually quite transformative for really understanding the fine scale organization of biological neural networks. But this technique actually has not gotten much traction until recently. And the reason for that is there are precisely two reasons. So one technical reason is that it's actually not easy to collect this data, although now it's becoming more and more popular.
I mean, easy and easier because the instruments have advanced. But the major bottleneck was actually how to make sense out of this data. And to give you an example, until recently, basic neuroscience labs who use this technology would have to employ an army of interns, train them how to use relatively simple annotations using computer software, and would try to essentially annotate these different structures, incredibly complex structures in the human brain, basically manually.
And as you can imagine, this process is very tedious. As you can imagine, this process is also very biased. And it was very slow, essentially.
Well, thankfully, the developments of AI, in particular, algorithms that permit in recognizing different structures has been absolutely instrumental. And I'll show you a movie that sort of exemplifies how powerful these algorithms are.
What you see on the left is a movie that sort of depicts an animation of raw data, image data. And what you see on the right is AI-based reconstruction of different structures in the same exact sample. And the structures include neuronal synapses.
The structures include different building blocks of the synapses. It includes neuronal projections. There are somas, non-neuronal cells. Essentially, everything the brain is comprised of can be visualized by this very powerful technique.
And I would like to say that this sort of type of imaging is also great for training, because we have a lot of data, which means that we can train networks relatively quickly, and relatively quickly get to the level when the precision of these annotations is actually quite accurate.
And just to exemplify how much data we have, so in this little block that you see on the right side, we have about 200,000 synapses. It's still a small fraction of the total number of synapses in the mouse and human brain, but still, it's a pretty good data set.
So now, as I mentioned already, we use this particular combination of ultrastructural imaging with AI-based annotation to answer two questions.
So the first question that we are very interested in is how neurons change their networks as we actually form a memory. And the related question is how these networks are altered as we age.
And for the purpose of this talk, I will very briefly focus on the first part. And I will very briefly highlight studies that are actually still unpublished.
But we are very excited about the studies, because they literally change the way how we think about the structural basis of memory storage.
Now, for the past 40 or 50 years or so, the field has been dominated by the work of Donald Happ. And what you see here is a diagram that depicts the so-called classical Hebbian model.
You don't need to pay attention to all these circles and all these formulas. The only reason why I actually am sharing this diagram with you is because it allows me to make a couple of important points about what we actually know about the basic principles of information coding.
So first of all, I'm sure that many of you know that neurons, all of you know, that neurons actually become activated or they become silenced in the brain as we do certain things. As we move, as we remember, as we become frustrated, and so on and so forth.
So this is a concept that everybody is familiar with. However, not everyone understands that coding of virtually every type of information or every task in the brain is attained in a very sparse manner.
What it means is that only small fractions of neurons are responsible for specific tasks. It could be a memory. It could be a perception. It could be any cognitive or task that requires locomotion and et cetera, et cetera.
So only small fractions of cells become activated or engaged, so to speak. And also, of course, the cells have different physiological properties and also have different functions.
What Donald Hebb proposed is that memory is formed when the sparsely activated cells reinforce their connections through a mechanism that's called Hebbian plasticity. And again, the concept is that there's a few cells that become engaged for memory storage, become more interconnected through these mechanisms. And that's how memory is believed to be formed.
So we started to use ultrastructural imaging to address this idea. And I have to say that we use genetic tools to actually get access and visualize the right cells.
I'm not going to go into details of the genetics because I think that it's going to be a bit boring for this audience. All I will tell you that we actually have ways of finding the right cells, the cells that will actually activate for a specific type of memory, so we can actually image them specifically.
And a type of this experiment is shown here. What you see is a so-called reconstruction of local circuitry. I hope you can appreciate the beauty of neuronal connections.
So what's shown here in different colors are individual synapses with neurotransmitter physicals that I introduced to you. And you see also green or gray colors. And these colors actually depict cells that were either irrelevant to memory coding or cells that were actually activated during memory coding.
So again, so we use genetic tools to specifically recognize them. So the prevailing view when we actually started these experiments was that cells that were actually recruited for memory coding would make more connections. And they would do so in a way that they reinforce the connectivity between themselves.
And this is not what we find. We were actually very surprised to find that, in fact, the cells that were recruited for memory coding in irrelevant neurons were quite similar in terms of the numbers of connections, the bulk numbers of connections, which was very much contrary to the common dogma.
However, what we found was actually very exciting. So if you remember this little image of a synapse that I showed you earlier, you can probably get an idea.
So the synapse actually is formed. So it has two parts. One is presynaptic that secretes the neurotransmitters. One other part is postsynaptic that receives the signal. And it's kind of a one-to-one ratio, right?
So most synapses basically are formed in a way that one connection transmits a signal to another postsynaptic recipient. But it turns out there is an exception from this rule. And this exception is actually exemplified here.
So there are very unusual types of synaptic connections in the brain that do not adhere to this rule. And they're depicted here. So these are connections that are formed by individual presynaptic neurons that secrete neurotransmitters onto multiple recipients. And we call them multisynaptic boutons.
I would like to say that although we as a neuroscience community already know a great deal about how synapses are organized, we know a great deal about genes that build its structures, what really distinguishes these particular types of synapses from conventional synapses is entirely unknown.
And the reason why we were excited about these structures is because it turns out that they're absolutely essential for memory storage. We find that they become more abundant in the brain. And also, we find that they become more complex.
And in essence, what this discovery means is that instead of just increasing the bulk numbers of connections, neurons change the circuitry. In fact, the circuitry becomes more diverse through a mechanism that allows them to increase the complexity of interactions between existing synapses, as shown here.
And we think that this discovery is very exciting because it really changes the way how we think about information storage. We are very curious to further investigate the structures. We are very interested in understanding what exactly they're comprised of, because this would hopefully give us a means to genetically access them.
And ultimately, we think that the discovery is ultimately, when we combine them with mathematical modeling, could potentially even be useful for design of artificial neural networks.
Yeah, so with that said, I would like to stop here. And I'm happy to take any questions.
Anton, those images were really beautiful. I'm genuinely curious. Let's have you stop sharing your screen.
Yep. And then we can have a conversation.
But for me, I'm genuinely curious, what does it entail to do imaging like that? I'm sorry, can you repeat the last part?
Yeah, absolutely. To image that detailed, like those types of detailed images, how hard is that? How technical does the equipment need to be? And when you're talking about AI algorithms kind of reconstructing those images, what does that entail in terms of the algorithms doing kind of the reconstruction component? I'd love to learn a little bit more about that.
And then folks, if you have questions, feel free to raise your hands, and I'll cue you guys up as the next in the queue to have conversations. Yeah.
So the first part of your question is relatively easy for me to answer. So the instruments that we use actually are quite sophisticated. They're also quite expensive. So we are fortunate to get access to this imaging technology through our collaborators. But unfortunately, not everyone has access to these technologies.
Yeah, and I have to say, these techniques are relatively new. And the imaging part, actually, is also I mean, there are actually current attempts to improve them even further.
I would like to say that this technique is now being used for, essentially, two types of studies. So a few labs, a few consortia, are actually trying to leverage this technique to understand the wiring of the entire brain. So it's been done, actually, with simpler organisms. Currently, there is an effort to reconstruct what we call reconstruction, or reverse engineering the entire mouse brain. And the hope is that, eventually, this will be possible with a human. So the studies, actually, are very exciting. And they produce a lot of interesting knowledge. But they also have a limitation. And the limitation is that they usually look at one brain. And as we all know, we're all different. So we have all different personalities, different genetics, and et cetera. Our brains also have very different histories. Everyone's history is unique. And everyone's memory is unique.
So the approach that we use is actually quite different. So we don't look at the entire brain. We actually look at the parts of the brain that we already know that they're important for memory storage. And we try to zoom in as much as possible to understand the fine scale organization. So to give you the analogy is that we're not trying to figure out if the keyboard or a hard drive are important for information storage. We already know the answer. It's the hard drive. Now we're trying to zoom in onto this hard drive and really reverse engineer it. So we really want to understand how it's organized at a very fine scale level.
Now, to answer the second part of your question, it's actually both easy and difficult for me. So I'm not an AI expert. And I have to, to my embarrassment, admit that I actually don't really know many details of these algorithms. So if you really want to get these details, you'll have to ask Marco, who's here, or other members of my lab.
Yeah, so I hope this answers your question.
Yeah, absolutely. And I think Marco's in the queue as well. Super, super interesting. Thank you, Anton.
Yeah, yeah. But I would like to actually emphasize that these image recognition algorithms have been absolutely transformative. The pipelines that we have already are very useful. We are constantly trying to make improvements in these pipelines. And what we mean by improvements is not only the accuracy, but also speed, which is important. But ultimately, and this is actually a major limitation of where we are right now. So and the limitation is that we actually have a good system to basically distinguish different features and annotate them. But we hope that eventually, AI will help us to get to the next level when, instead of using a human, and humans can be biased and dogmatic, right, to actually get to the next level. And what I mean by next level is when we decide what is important and what is not. When we decide we're going to focus on this particular features versus other features. So we hope that we'll actually get AI platforms that will allow us to not only formulate hypotheses, but also to look at these patterns and extract patterns in a completely unbiased manner. And what I mean by patterns are patterns that may actually not be very obvious to us.
Super interesting, thank you.
Spencer, you're up next. Please.
Hi, thanks for the presentation, really good. I was, the memory, storage and creating of memories. Do you think it's possible to be aware of a situation if you can't create any memory? I wonder if that's something that's known medically or if you've, I know you spoke about people who only have short term memory. But if you stopped the formation of memories completely, so the brain was doing the processing, but couldn't store it even short term, would that remove, in your opinion, perception, awareness of an environment? Does that rely on memory? Do we need memory in order to be aware of our environment or is that a separate block? It relates to the way AI does not do this. AI has no memory. It's only processes. And I wondered whether there was any medical view on that.
This is a fascinating question. You know, I don't think there is actually one straightforward answer. So I would like to start my answer with reminding you that the brain is a parallel device. So the brain is capable of doing multiple things at the same time. And in fact, I really didn't want to go into details of nitty gritty details of basic neuroscience and tell you what we actually think about our recent discoveries. But since you asked, I think that one of the implications of what we find with these unusual structures that actually diversify the output from one cell is that it might actually increase the speed and efficacy of parallel processing. Now, to go back to the question, it is actually not easy to erase all forms of memory without really impairing a human. With that being said, there are actually quite interesting examples of people with both short and long-term memory loss who are nonetheless very capable otherwise. For example, one of the, well, perhaps one of the most famous examples is so-called HM patient. I'm not sure if you've heard about him. So HM is an abbreviation for Henry Molaison. So that was his name. The name was only revealed recently. And what happened to him was that he started developing severe migraines when he was in his early 20s. And after all treatments failed, he agreed to have this experimental surgery. And the surgery involved the removal of the large part of his brain that includes the hippocampus. And this is the part of the brain we studied. And the surgery actually worked, and his migraines stopped. But because the hippocampus was removed, he actually lost the ability to form new memories. And in fact, he was interviewed by many psychologists. He was actually considered one of the most famous neuroscience patients or neurological patients in history. But what is interesting is that in spite of this condition, in spite of his massive memory loss, he still excelled in board games, which kind of implies that you don't necessarily need to be able to store information long term. With that being said, I can tell you that I play chess recreationally, so I'm not a very strong player. But I'm OK. And for chess, short-term memory is actually of critical importance. So you know what I'm trying to say. So here's an example. Especially if you play at relatively high speed, when you have to process quickly, any destruction of a short-term memory is absolutely detrimental to your success. Not to mention other factors. Like, for example, if I play online and I know that my opponent is in Europe, and it's still early morning in Europe, my chances, we could have exactly the same rating, so technically suggesting that our chances are about 50-50. But if it's early morning for them, my chances of winning actually are much higher. And vice versa. So I hope it at least partially answers your question.
Perfect. Thank you very much. Spencer, thank you.
Marco, you're up next. Marco, you're our expert here as well. He's the guy who has actually been doing all this work.
Thanks. Yeah, actually, I wanted to take this opportunity, Anton, to ask you a bigger picture question. So obviously, this is one example where we're using AI to analyze the brain. But in a bigger picture, in terms of biology, going forward, how do you actually think that AI is going to impact how we do science? Or are there specific fields that you think in the immediate future it would actually impact it the most?
Marco, this is a great question. I would like to start with saying that there are so many applications where AI is already making a huge impact. But there are also so many applications in both basic science and also biomedical field where applications where AI could be very instrumental. But it's actually quite surprising for me that nobody is using it. So here's an example. So when my wife was pregnant, I attended several doctor's visits with her. And they were doing ultrasound. The quality of imaging is actually quite nice. I mean, you see all these details. And then they literally take a ruler, and they measure the distances between.
into hemispheres and say, OK, so brain development is on track. But this is actually an incremental example.
So I think what you're asking is actually a bigger picture. And I would argue that we'll see tremendous changes when AGI becomes a reality. Because I think that it will definitely change the way how we do science.
We still have a human who makes predictions. Basic science is also, we have a community. And this community is not completely disorganized. But individual labs actually operate like small businesses, essentially. And individual labs make their own predictions. They decide how they're going to prioritize problems. And this prioritization is not always correct. So it's biased.
And I think that the algorithms will actually make a huge impact by not only increasing the speed of data processing and interpretation, but also by allowing us to concentrate on most important problems and formulating the correct hypothesis. This would be one application.
I mean, to paraphrase this, I'm actually worried about my job. So the professors may not be actually relevant in the future because AGI will be actually taking our roles.
I don't think that's true, Anton. I believe that humans will always also appreciate being advised by other humans. And there's an aspect of being a professor and running a lab and being a PhD advisor that I don't believe that robots can supplant. Right, Marko?
Well, I was a bit exaggerating. I agree. I agree with you, Natalie, yeah, of course.
Marko, thank you. But just very briefly, to my answer to Marko, I mean, even if you think about how we formulate hypothesis. So we basically formulate hypothesis by examining what is known and what is unknown. And this process requires a review of vast amounts of literature. This literature is very diverse.
It's kind of naive to assume that everything that is published in scientific journals is correct. In fact, the reality is that science is not bulletproof. People oftentimes make mistakes. And what it means is that oftentimes people publish the results that are not correct, so wrong results. And how do you actually distinguish between good information and bad information? How we actually pick the right, most relevant parts of information from this massive ocean? So it's still done mostly manual. And I think, again, the AI is going to make a huge impact in this area.
Marko, thank you. Meralda, you're up next.
Hello. First of all, thank you so much for the amazing presentation, Professor. My name is Meralda Zhang, and I'm a current molecular and cell biology and data science major student at UC Berkeley. I'm really intrigued by the AI images. And could you please give me some advice about what type of computer skills I should start honing in order to learn to create those images?
Well, again, I think Marko would be able to answer this question better. You know, you don't necessarily need to have computer skills to actually create these images. The background in computer science really becomes relevant when it comes to really understanding what these images mean.
And I have to say that this work that I only very briefly shared with everybody today is a group effort, right? So we are not just solely relying on AI, because we're also using advanced microscopic techniques. And we're also combining it with molecular genetic techniques, which I didn't tell you much about today because I didn't want to complicate my presentation. But these molecular genetic tools actually have critical importance because they allow us to really visualize right cells in the right structures, right?
So we are not just looking at the scene trying to find sort of a needle in a stack over here, right? So we actually have reference points. With that being said, I would actually predict that when these algorithms become even better, these molecular tools will become less relevant because the algorithms will actually be able to replace them, right? So they will be able to actually recognize features much better, not only based on the physical sort of characteristics, but based also on parameters that may not be obvious to us, parameters that can actually tell us about sort of when neurons were activated, and so on and so forth.
But again, so, you know, let me just paraphrase my answer. If I was starting today as a graduate student, I would definitely consider doing something in the area that involves AI, not necessarily becoming an engineer, a software engineer, but I think that, you know, I like to tell people in my lab and my colleagues that if you're not doing anything that is related to AI, if you're not using chat GPT, quite frankly, then you're a beginner, yeah. Okay.
Yeah, and I'm sure that there are many members of this forum who can actually give you much better guidance in terms of how to actually excel on this path.
Right, thank you very much. I'll just mention one thing really quick, Anton and Meralda, we're actually launching a student forum in a week or two. So a subgroup of this forum, this forum is composed mostly of pretty expert level participants, but we know that we also wanna offer a space specifically for students where you can get professional development, types of information. We're gonna do our best to help up and coming scholars and practitioners across domains, figure out how to break into AI or how to leverage AI in their particular domain. So if you're interested in that, just stay tuned, that's coming in the next couple of weeks.
Okay, thank you very much. I would like to say that I'm actually quite humbled to talk about AI in this particular group, because you guys are at the forefront, yeah.
Thank you, Meralda, Puri, you're up next.
Yeah, thank you for the amazing presentation. I think you're muted.
I think you're muted, too. Can you hear me now?
Yeah, we can hear you. Yes.
So thank you for the amazing presentation. So I'm curious about how learning happens in the human brain. So I know you talked a little bit about Hebbian learning. So if you look at like an artificial neural network, if you want to train it to classify cats and dogs, you have to show like a bunch of dog pictures and then a bunch of cat pictures for the network to identify the distinctions.
But humans from a very young age are able to identify the distinction between a cat and a dog without seeing thousands of images of cats and dogs. So I'm just wondering what kind of biological processes give this kind of ability for humans?
You know, this is a fascinating question. It's not very easy to answer it in just a couple of sentences. You know, I think that there are actually similarities in terms of how artificial networks and biological networks process information. And in fact, as far as I know, that early developers of AI actually borrowed from basic neuroscience and particle studies in the visual cortex were actually helpful for this design.
I don't know if there are actually equivalence of structures that I described to you today that are equivalence in convolutional or in other types of artificial neural networks. But the example that you're bringing up to our attention was actually very interesting. I think that although it is not possible to actually precisely answer your question for one simple reason, in spite of the fact that we've accumulated tremendous knowledge about how we learn and remember, we still don't completely understand how it works really.
Right, so we have a lot of information, but we don't have one unifying model. And I would like to sort of quote Niels Bohr, a famous physicist who once said that, when a physicist understands the problem, when they don't understand the problem, they write a whole bunch of formulas. When the problem is solved, it boils down to one equation. And there are many examples in biology where this equation has been defined.
For example, when you think about how neurons secrete neurotransmitters, it's also a complicated process, right? So we have a very, very clear understanding, right? So we know the major players, we know how they interact with each other and et cetera. When it comes to learning, it's still an area of open investigation. And there are many theories. In fact, even if you ask different people in the field how memory is stored, you might actually get conflicting answers. There are theories that memory is stored as a genetic mark. There are theories that memories are stored in synapses. There are theories that memories are stored even in extracellular matrix, believe it or not. But this idea was actually conceived by former Roger Chen, who also is a Nobel laureate. I said it was complete nonsense. But recent evidence suggests that actually it could be relevant to memory storage.
I think that one of the reasons for the difference that you outlined is that we have innate behaviors. And these are behaviors that artificial neural networks do not possess, as far as I understand. And what I mean by innate behaviors are behaviors that essentially do not require any training. And there are many examples like that. Like, for example, children start copying the gestures of their parents without actually learning them, right? And even image recognition, I think that it could actually at least partially be attributed to innate behaviors. So I hope it makes sense for you.
Yeah, it does. Thank you. Thank you.
Anton, hopefully you're OK with going a little bit over maybe five minutes or so.
Yeah, yeah, definitely. And we have a lot of questions queued up. And so people are really interested.
Yeah, absolutely. I can stay as long as you guys want.
Anna, you're up next. Thank you so much for coming to speak with us today. I'm a recent psychology graduate from UCLA. So this is really, really interesting to see the way AI can kind of be incorporated in memory. And something I always found really interesting was the study about dementia and Alzheimer's. And I was just wondering if you could speak a little bit about how you see AI imaging in these models kind of influencing that field.
Oh, I think the impact is going to be huge. As I mentioned very briefly, we actually are looking at normal aging. We are not doing these experiments with humans yet. And the reason is because, again, so we think that what we do in mice is not only informative, but we can also do these experiments much quicker, relatively speaking. When it comes to Alzheimer's, just for sort of to give a very brief background to the rest of the forum members, obviously, everybody knows that this is actually a very big problem. So the problem affects millions of people just in the United States alone. It has a huge socioeconomic burden. And there is no solution. In fact, there is no drug that actually cures the disease. We know that ultimately, this condition is attributed to loss of neurons. But we don't know exactly. We don't know much about the nature of early events, right? So early events of disease progression where neurons are still there when there is no clear signs of synaptic degeneration. I think it's actually reasonable to predict that these early events actually coincide with structural changes in neural circuits. But what these changes are, we don't know. And currently, the field is kind of looking at the tip of the iceberg, right? So for example, when we look at structural changes in the brain that are associated with normal aging, we actually see in very old brains changes that actually resemble a very mild form of Alzheimer's disease, right? So every once in a while, cells will be lost. Every once in a while, you will see structures that are degenerating. And we think that these are actually not the most interesting changes. So what we are trying to understand are changes that are not immediately obvious to us. And I think that this is actually the changes that AI is going to be absolutely instrumental for not only detecting, but also interpreting. So I actually predict that the impact of AI is going to be, in particular, algorithms that actually are tailored for image recognition, I think is going to be tremendously useful. Not just for Alzheimer's, but also other types of neurological disorders. Because ultimately, yeah, autism is another example, right? So autism is actually a relatively mild abnormality. So these people who actually suffer even from relatively serious forms of autism are still functional, right? So which means that the changes in neuronal wiring are relatively subtle. But what the nature of these changes are is still not clear. I mean, there are genes that have been associated with autism. But what exactly they do to neural circuits and how is not clear. And again, just from imaging perspective, I think AI is going to be very helpful to really finding not only the basic mechanisms of disease progression, but ultimately for developing cures.
I never thought about it in the lens of autism and other types of disabilities like that. So yeah, that was really interesting. Thank you.
Yeah, yeah. I would like to actually add that there is one limitation of this particular technology. So we can actually use it in the human. It's possible. The only problem is that, obviously, there are many ethical restrictions. And if you decide to actually image human tissue, we can only do it post-mortem, which means that we don't really control the timing of experiments. Needless to say, we cannot just use any genetic manipulations of the brain tissue normally and routinely do this demise. But still, even this analysis of post-mortem human tissues could be, well, biopsies is another exception. But it's not easy to get access to them. And there is a lot of variability in tissue quality. But nonetheless, even analysis of post-mortem tissues could be very, very useful, especially if we scale them up. So again, one of the biggest reasons why there is so much excitement about this development is because we can now do these experiments much quicker. We can actually make sense out of image data much quicker, which means that in the near future, we'll be able to expand the sample size. And we can expand the sample size by, for example, when it comes to post-mortem analysis of human tissues, we can actually track the history of disease. We can compare relatively, ideally, we'll be able to compare relatively large populations. And again, so I think it's going to be absolutely instrumental for understanding the biology of normal biology or biological networks, and also understanding how they're affected by diseases like Alzheimer's and other types of neurodegeneration.
Anna, thank you. Thank you so much. I know we're about to wrap up. So we have this really cool feature in this platform, guys. For a post-event, you can have a recap on all your messages. And we can have more interaction with folks as we kind of message on following up on the discussion tier and such. But maybe as we kind of look at wrapping up, Marco, I would love to have, after the event, for you to kind of chime in on the messaging board as we ask, what is it? How does this AI thing work? Everyone's kind of really interested. And there's pretty images. And then you slice and dice them. And then you create all these things. And so we might even throw up a session or something later on where we can kind of just understand more on how that works. And for folks maybe in the student forum who'd be interested in learning how that works and kind of what skills would be required for that to get better situated with kind of doing that type of biology, that'd be super interesting.
And Artem, just to help everybody figure it out, so this chat, at the end of the event, if you go to your DMs, since there are so many new community members here, if you go to your direct messages in the messaging tab, this thread with all of our questions and our chat will still live there. So if you drop your question in there, we can reach out to Anton or Marco. And perhaps they'll be generous enough to respond to your questions async after the event.
We would be very happy to answer any questions. Post-factum by all means, feel free to reach out. Again, so I would encourage you to actually work with Marco if you're interested in all the details of algorithms. And I would be happy to answer any other questions.
And before we wrap up, one thing I wanted to quickly announce that's relevant for everyone here is we're interested in working with undergrads, recent grads, and doctoral graduates who can help us evaluate frontier machine learning models. And we'll drop a link in the chat of an upcoming research study that we're working on that you all as participants can either amplify into your networks or even participate as well. We're really excited to have you join. It is a paid opportunity as well. So you will be paid for your time. And you will help us evaluate frontier machine learning models as well. So again, thank you so much, everyone, for taking the time and coming. And Anton, I really do appreciate your talk. And the images were really cool. I was looking at them. How detailed has science become that we can see so much detail in something so small that you can only see under a microscope, which is really beautiful?
Thank you. Happy to be here.
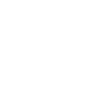