Making AI Work For Everyone
speakers
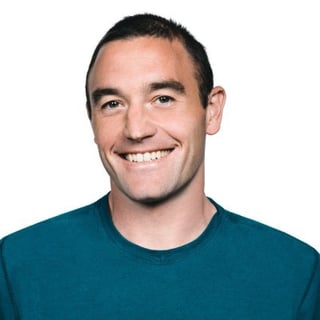
Kevin Weil is the Chief Product Officer at OpenAI, where he leads the development and application of cutting-edge AI research into products and services that empower consumers, developers, and businesses. With a wealth of experience in scaling technology products, Kevin brings a deep understanding of both consumer and enterprise needs in the AI space. Prior to joining OpenAI, he was the Head of Product at Instagram, leading consumer and monetization efforts that contributed to the platform's global expansion and success. Kevin's experience also includes a pivotal role at Twitter, where he served as Senior Vice President of Product. He played a key part in shaping the platform’s core consumer experience and advertising products, while also overseeing development for Vine and Periscope. During his tenure at Twitter, he led the creation of the company’s advertising platform and the development of Fabric, a mobile development suite. Kevin holds a B.A. in Mathematics and Physics from Harvard University, graduating summa cum laude, and an M.S. in Physics from Stanford University. He is also a dedicated advocate for environmental conservation, serving on the board of The Nature Conservancy.
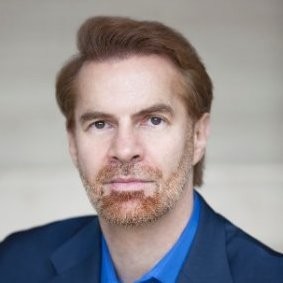
Dr. Erik Brynjolfsson is the Jerry Yang and Akiko Yamazaki Professor and Senior Fellow at the Stanford Institute for Human-Centered AI (HAI), and Director of the Stanford Digital Economy Lab. He is the Ralph Landau Senior Fellow at the Stanford Institute for Economic Policy Research (SIEPR) and holds appointments at the Stanford Graduate School of Business, Stanford Department of Economics, and a Research Associate at the National Bureau of Economic Research (NBER).
One of the most-cited authors on the economics of information, Brynjolfsson was among the first researchers to measure productivity contributions of IT and the complementary role of organizational capital and other intangibles. He has done pioneering research on digital commerce, the Long Tail, bundling and pricing models, intangible assets and the effects of IT on business strategy, productivity and performance.
Brynjolfsson speaks globally and is the author of nine books including, with co-author Andrew McAfee, best-seller The Second Machine Age: Work, Progress and Prosperity in a Time of Brilliant Technologies, and Machine, Platform, Crowd: Harnessing Our Digital Future as well as over 100 academic articles and five patents. He holds Bachelors and Masters degrees from Harvard University in applied mathematics and decision sciences and a Ph.D. from MIT in managerial economics. His papers can be found here. https://www.brynjolfsson.com/research
SUMMARY
Replay: Learn how AI is reshaping the economy—and why its true impact goes beyond traditional metrics.
Earlier this year, the OpenAI Forum hosted a fireside chat featuring Kevin Weil, Chief Product Officer at OpenAI, and Erik Brynjolfsson, Stanford professor and digital economy expert. They explored how AI differs from past technological revolutions, why its benefits often escape traditional economic measurements, and how businesses can integrate AI to augment—not replace—human workers.
TRANSCRIPT
Next up, we have our fireside chat. Thank you so much for being here, Eric and Kevin.
Good to be here.
Awesome. Thanks so much for joining us. I got to say, I'm excited about this because I have a bunch of questions to ask you, and I'm eager to hear your thoughts. So great. We'll just jump right in.
So one, we'll talk about AI relative to other general purpose technologies and the impact that they've had. So if you think about things like electricity, things like computing and their impact on GDP, it took decades for that impact to be felt. You're the expert, not me. I've heard people even wondering today, where is the impact of the Internet on GDP? It's not like we've suddenly gone to five percent. How do you think that plays out with AI?
Well, as it showed up there, I'm optimistic in the longer term. But right now, if you look at the official productivity statistics last quarter, it was 1.2 percent, which is not that impressive. In the 90s, it was more than twice as high. In the early 2000s, it was more than twice as high. I think there are two main things going on there. We have a lot of specific instances that are very impressive. But one thing is that we're not measuring things correctly. The average American spends a little more than half of their waking hours looking at digital screens. Most of you are not right now, but it's about eight and a half hours per day of different size screens. That means when they're voting with their time, they're spending a lot of time consuming digital products. A lot of them have zero price. GDP measures a lot of things, but it doesn't do a good job of measuring things that have zero price. So we're missing a lot of the value that people feel like they're getting. That, of course, is getting more and more. It wasn't digital goods weren't very important 50 or even 20 years ago. So that's part of it, but I don't think that's the main thing.
The main thing, I think, is that these general purpose technologies and earlier someone put up a slide, I forget which one of you did that, about how electricity took a long time before we got the payoffs, the steam engine did, and that is something we sometimes call the productivity J-curve, that initially you have to invest a lot in re-skilling, changing your business processes, figuring out better ways of using the technology. And sometimes all those costs don't translate immediately into benefits, but when they do, you get a real takeoff. And I think we're still haven't gotten all that reorganization. The good news is I think it's happening a lot quicker this time. There's a lot of reasons why there won't be as much need for much of those complementary re-skilling and new business process redesign. So I expect it to be shorter and faster and shallower, but it still is part of the problem.
I mean, you think back to building out the railroads, obviously there's a massive amount of work for the deployment phase of the technology. Ditto electricity, you've got to build a bunch of infrastructure. For internet, I guess you can sort of argue you had to lay a bunch of fiber and the networks were sort of so-so. It's not just the physical, like take electricity. It wasn't, they actually put the machines in the factories and they still didn't get the productivity. So it wasn't the physical installation so much. It was that initially they would take out a steam engine and put in a big electric motor where the steam engine was and nothing much changed and they didn't get much productivity gain. It literally took 30 years for a generation of managers to retire and then they started to realize, wait, we don't have to have this one big electric motor in the middle with pulleys and crankshafts. We can have a separate electric motor for each piece of equipment, lay it out on a single story, have it based on the flow of materials, have an assembly line. That led to a doubling or even tripling of productivity by people like Paul David who measured it. So it was more actually the process, the rescaling, all the intangibles rather than the physical building.
That said, I do agree with the broader points you're making that we may be able to do it a lot faster than having a digital infrastructure already in place and having some of these tools that don't require time training to use chat GPT. You don't need to learn a new arcane coding language or anything. It does, maybe you have to learn a little bit of prompting, I don't know. But it's not nearly the same kind of curve that it was with earlier technologies.
Yeah, I think it'll be interesting too, Jason was talking about the YC companies and the rates of growth, to see if there's more sort of creative destruction in companies that do pick it up faster. Can they make the cycle go faster because they're actually able to punch above their weight class in a way that maybe was harder in previous eras?
Yeah, I hope so. And one implication is that for policymakers, they need to think about ways that we can have that kind of dynamism where new companies can be started. Dynamism has actually gone down a lot in America. There are actually fewer startups, maybe not in this neighborhood, but nationwide. And there's less movement between companies, there's less geographic mobility. All of those metrics are going down. But America has never been successful by sort of like freezing in place the way we're doing things. You've always got to embrace change. In order to have new technologies take off, you need to have new companies or at least new processes within existing companies. That hasn't been happening as fast as it used to, but maybe we can do more. If we want to speed technology payoff, we need to speed up those kinds of changes.
And so you mentioned, we were talking about GDP as sort of the way to measure this. And you said, well, all this stuff is free these days, doesn't contribute to GDP in the traditional ways that you measure it. So you're king of the world for a day. How do you actually measure progress?
Well, that's a really hard question because there's aspects of well-being and other, but we are trying to make a dent in one piece of it. So we've introduced a tool called GDP-B. The B stands for measuring the benefits rather than the costs. So, you know, ChatGPT, even the free version or Wikipedia could create a lot of value for a person even if they pay zero. So what we are doing is doing millions of online choice experiments where we ask how much do we have to pay you to stop using this for a month or a week or a different amount of time? And we sum up all those values. Some people you have to pay a lot, some you don't have to pay that much, and you get a demand curve for what the value of that good is. Now you're getting an estimate, this is a room of economists, of consumer surplus, and that is a measure of the welfare contribution. So we are... Actually, on March 14th, we'll be unveiling some new results looking at the consumer surplus from a whole set of digital goods and a whole set of non-digital goods. It's meant to be a representative market basket of what's in the economy. We can put those alongside the GDP numbers and start understanding, you know, not just where we're spending money, but where we're getting value. And my hope, you know, to answer your question is that, you know, we'll be able to do that on, like I say, a quarterly basis and start having a metric that is suitable for the digital economy similar to what GDP was for the previous economy.
So maybe we'll switch gears a little bit. You look at the history of diffusion of technologies. There's some new modality, you know, I'd say in media, and what people do is take what they know from the current form of media and apply it, just like copy it over to the current one. So, you know, there's TV. How do you advertise on TV? The first thing you do is you get some people standing on a stage reading their radio ads, you know, right? And then eventually, to your point about going from steam to electricity, people figure out the ways to actually use the new technology and things change. So maybe one interesting analogy or interesting angle on this with AI is the ways that we benchmark AI today, we have these evals that kind of correspond to the way that we operate today. So, you know, we measure intelligence of our models through things like GPQA, which is a benchmark that basically looks at graduate level reasoning across a whole bunch of range, you know, a whole bunch of different graduate science fields and says, how does the model do as if it were
talented grad student having to pass the equivalent of quals relative to every other grad student. And it turns out, does really well. But there are these human measures of intelligence. That's not necessarily the right way to think about some of these models.
Maybe they're intelligent in totally different ways. I know you've done some thinking about this. I think it's fascinating. This is a really important question, one that I hope people in the room can contribute to, because it's totally understandable and natural that we look at human intelligence as kind of the benchmark.
But if you think more carefully, and kind of a pet peeve I have is the way OpenAI defines AGI, general intelligence. You kind of map it to human intelligence. And with all due respect to my fellow humans, we are not the most general kind of intelligence.
My calculator can do a lot of things that I can't do. Google can search billions of documents. Even chimpanzees have better short-term memory than humans do, and bats can do echolocation. There's all sorts of other kinds of intelligence.
And what I'd like to see is a truly general set of benchmarks, instead of just mapping people onto humans. And it's not just an intellectual debate. It has to do with the direction of technology, because you guys are so good at hitting and saturating those benchmarks when somebody publishes one, that in a sense, the benchmarks are steering technology.
And they're steering it towards matching humans. And economists know that if you make something a close substitute, it tends to drive down the wages, drive down the price of that substitute. But if you make something a complement, something that makes the other, if A is a complement to B, then it makes B more valuable.
And if you can make AI a complement to human, doing different things than humans, it'll make humans more valuable. And the benefit of that is not just that it increases payoff and productivity, either one of them could do that, but it's more likely to create widely shared prosperity.
Now that said, I think it's a lot harder to come up with these broader metrics of intelligence that are truly general. And so I'm not saying that it's an easy problem, just like it's easier for an ad guy to come up with, I guess, a radio ad and just have them read it in the script but eventually, people were creative enough to come up with better ways of thinking about the problem or just like they were with electricity.
And so my challenge to this group is let's think about broader benchmarks that will not only measure intelligence, but will also indirectly help steer intelligence towards ways that we can create broadly shared prosperity. I'm curious to go deeper on this and I don't exactly know where I'm going here, but how do you think about-
Join the club. Yeah, right. How do you think about measuring complementarity in that way? I mean, humans are very industrious and creative and in lots of places where you would want to complement human intelligence, we found different ways to do it already. Like, how do you invent new versions that are measured?
I mean, I think we often think of machines as kind of doing what people do, but actually, if you think with the broader sweep of history, there was a couple of cosmic slides up there earlier, most technologies have mainly been complements. The value of a human, an hour of human labor used to be very low a couple hundred years ago or a hundred years ago or 50 years ago. It's been going up and why is that?
Because we have a lot more tools that make one hour more valuable. A coder is more valuable now than they used to be. A guy working with a steam shovel is more, I don't have steam shovels anymore, whatever you call those things. So, those are things that have mostly complemented us and I think that one thing that's happening now, partly because of the Turing test, this iconic measure, is that AI may be becoming a closer and closer substitute.
And maybe in the end of the day, that's something that is going to happen inevitably, but I do think that there's a fair amount of room to, in the meantime, steer it towards becoming more of a complement, something that enhances human value. And there are things we can do in policy, taxes, education, et cetera, that will steer it in that direction. Do you think that there are pieces that are kind of humanity's long-term area of ownership in this human-AI complementary?
Are there things that are forever off? I was very frustrated when Noah marched off the stage at the end, because he was saying, all these things that you think the AI can't do, we're going to be doing them. And I wanted to ask him before, is he still in the room somewhere? No, no, he ran away. Okay, but that's a good question for you guys. What are some things that you think will be difficult for AI to do?
I mean, one that comes to mind for me is just exception processing. You put up Rich Sutton's bitter lesson. By the way, it should be noted, somebody got the Turing Award a few hours ago. Guess who? Rich Sutton. So he's been very thoughtful about that. But one of the things that you need to train the models is lots and lots of examples, lots and lots of data, at least with current technology.
And humans, for now, we have a comparative advantage in improvising, dealing with exceptions. In the call center study that we did, we found that there's this long tail of questions that there wasn't a lot of data for. And the machines weren't very good at answering them. But humans, not that they were great, but they could kind of muddle their way through at some of those questions. So one place we have comparative advantage is that kind of improvisation, dealing with things that weren't expected.
Being a CEO is part of that, is dealing with all the exceptions. Once you figure out what needs to be done, then you explain that to somebody or ask them to follow that. So that would be one. But I'm hopeful that you all can help us think of other ones.
Yeah, I mean, that makes a lot of sense. And when we look at our customers, for example, who are automating things like customer support, what they're actually doing is automating the common cases and then having people still handle the exceptional cases.
Yeah, exactly. There's sort of a Pareto, almost by definition, there's some questions that are more common and some that are less common. It often follows a kind of power law. And I see AI kind of marching down that thing, but there's still a pretty long tail. I don't know how long that tail is in different tasks, but for some, as we're discovering with self-driving cars, there's a really long tail of weird cases that are hard for the AI to handle.
Yeah. So switching gears again, what do you think is the most, if we're talking about uncomfortable or like politically unpopular truths about AI and productivity, what are the things that people kind of know but actually don't want to talk about?
Yeah. It's a good question. One that I've been thinking about with Zoe over here is if you do get AI that's really, really powerful, that can do lots of different things, then Friedrich Hayek's lesson that you want to have dispersed information processing, dispersed data, and that therefore you have dispersed power and decision rights, that's been the norm for basically all of history.
And there's a socialist calculation debate that that sort of decentralized decision-making beat centralized decision-making. But in a future system where there are very powerful systems that are bringing all their data to some place in Bentonville, Arkansas, or someplace like that, and they have all the local knowledge all centralized in making decisions, maybe that will be more efficient if you have enough processing power.
And then you've got a world where the good news is you can have way higher efficiency, you can have way higher productivity, you can have UBI that pays everybody, while on the other side of it is the humans wouldn't have a lot of bargaining power. And that's uncomfortable to me. I mean, I'm hoping that Sam will be generous and give us all a share of this benefit. But we won't have a whole lot of leverage over him or Dario or Donald Trump or whoever it is who allocates the UBI. I think it'll be interesting to see whether.
whether the ultimate model, the optimal model, is one central AI system, or whether, like humans, you actually want multiple smart AI systems that collaborate together, brainstorm, collectively can come up with things.
Can I ask you a question? Yeah, go for it. Because, especially with DeepSeek coming out recently, I mean, it does kind of give me some hope that maybe the gradient is pretty flat, that you could have a pretty small model with many or most of the capabilities of the really big models, and that maybe it won't all, sort of the scaling laws won't drive everything, and that, in fact, relatively small models work. I mean, what are you seeing in terms of the cost and the demand for a model that's a little off the frontier versus one that's right at the frontier?
Are you glad to say that?
Yeah, yeah. Sam said, I think, a few weeks ago, if you look at the cost for a particular level of intelligence, suitably defined, it's coming down by a factor of 10 every year at the rate that we're going. And, you know, you think about Moore's Law, that was twice the number of transistors on a chip every 18 months. This is 10x a decrease in cost every year. So it's a much, much steeper exponential, which is pretty amazing. So we're, obviously, the models are getting smarter at a rapid clip, so they're getting smarter and they're getting cheaper.
But if you hold intelligence constant, they're getting dramatically cheaper. So that means you can squeeze more and more into small models, and I think that's gonna be a powerful trend that will continue.
And I think it doesn't mean that you don't want tons and tons of compute, because people are going to want the smartest models possible, especially as these models start doing more for us in the real world. You're not gonna wanna use the thing that's like 80% as good, because it's gonna actually be doing things that matter. And so you're gonna want the best model possible. So you've got both of those trends happening.
I think that's really interesting, and to some extent, it's an empirical question how that's gonna shake out and what the ecosystem will look like, how many people will want that very frontier model. I imagine the Department of Defense or somebody doing frontier scientific research or whatever, getting that last bit, or someone gave the example of the solar panels, that you want the really best model. But if you're running your grocery and you need to organize the shelves better, maybe you don't need hyper Einstein.
I mean, we see that internally. We use a variety of models of different sizes internally to do different things. Sometimes you have a very simple question that you just need to have the model go over again and again and again, and you want it to be cheap and fast, and other times you need the model to have a sufficient amount of generality and reasoning, and you're okay with it taking a little longer, but you want better performance. So it is across the board, and you wanna use the right model for the right job. So we just have a few more minutes. Maybe we open it up to questions.
You brought up the idea of the alternative between the high X system of where, which your markets and preferences make the choices, versus where we have one system that understands all those preferences. But I'd say the biggest argument against the latter is that you need some panopticon to know what we're all gonna want, and the moment you make a choice or whatever, that our preferences are going to shift. So how much do you buy into that?
Well, so one of the things that kind of made me a little worried was I read about this experiment at Netflix where they asked people to pick their favorite movie and watch it that night, and then they asked some other people, hey, we're gonna pick a movie for you. And then the next day they asked each group how much they liked the movie they watched. Which group do you think was more happy with the movie?
So I hate to say it, but maybe we're not all such special snowflakes, and maybe the AI will know what we should be doing, and when we're texting our kids and choosing what dinner or whatever, we'll increasingly, like I do with my GPS, say, you know, why don't you tell me what I want?
I'd say a follow-up on that was, one of the things I find there's a high preference for, too, in situations like that is what did your friends watch, right? And it can go either way, but sometimes our preferences are dictated by our peer group, which is a harder thing to necessarily influence.
Can I offer an opposing viewpoint to yours? I hope so. Which is, a lot of this is based on data. So in the Netflix case, Netflix has all the data about the movies that you watch and everybody else watches, and so it's sort of a closed system. No public model that OpenAI builds or anybody else builds will have access to all of the data that's relevant to solve the totality of problems that we need to solve in the economy.
And so you're going to end up with a whole bunch of different models, because there's a whole bunch of different data silos. The vast majority of the world's data is private. It's locked up behind company firewalls and government firewalls and stuff like that. And so I think that inherently means that we're going to have lots of different models interacting no matter what. And I think that's a good thing, personally.
I'm in AI research, and as I talk to people about AI, I find that there is a big hesitance to adopt it. Like, do you go to the doctor and there's an AI model that tells you about a disease and how to treat it? How much can I trust it even if there's an inherent bias in humans? And what is the inherent bias like in adopting it towards economic growth?
It's a good question. I should mention, last time I went to my doctor, I was a little impressed because she had an AI transcribing the whole conversation. And she really said she'd like to be using it for a few months. And it's filtered out the chit-chat and just kind of gave a sort of a medical thing. She sent me a copy of it afterwards. So she was adopting it, and maybe at some point I'll be talking more to the AI directly. But I think there is a real question there about trust and reliability. And another doctor story that's not as encouraging, there was an article in the Journal of Medical Association a few months ago that Eric Horvitz and others published where they had, if I'm remembering this correctly, they had three treatments, the human-only, the AI-only, and the doctor plus the AI working together. And you'd think, you'd hope that the human plus doctor plus AI would do better. It turned out it did worse than the AI alone. And I think a big part of it is these current systems are not very interpretable. And so the doctor didn't know when to overrule or to agree with it. They ended up actually doing it the wrong times. And I think this is, if we want to have successful systems at work where humans and machines are working together, they have to be able to trust and know, because the AI's not gonna be perfect, the human's not perfect, and it's gotta know when to rely on it. If the AI system just says, hey, cut off the patient's left leg, and the doctor's like, well, why? He says, well, do it, it's 90% likely to be correct. I don't know how many doctors would feel comfortable doing that, but if it could explain all the reasoning and then the doctor could trust it, or the end user, then I think we'd do better.
So this seems, I'm sure you guys are working on this, is more explainable AI, because not just for its own sake and for verification, but it'll make it easier to have human and machine systems that create more value than either of them could separately. Cool, I think we have time for one more.
Hey, I'm curious to hear a little bit more about what you touched on at the very beginning of your conversation, which is something you've worked on, Eric, in terms of your research, on the complementarities that we need to essentially realize some of the productivity gains from a lot of these technologies. And you said that in your view, you think that with AI, the complementarities that we need, maybe this transition is going to be faster than what we have seen before. If you had to sort of think about what are the complementarities that you think are different in this transition versus the previous technological transitions, I'm curious to hear what that would be. And Kevin, as you've seen a lot of companies that you've worked with work with OpenAI's API, what are the complementarities that you're seeing anecdotally or that you're thinking of designing for going forward?
So I was talking to a former OpenAI employee, Leopold Aschenbrenner, and he's, as you know, quite excited about the possibilities of AI. And I was telling him, you know, there's going to be all these compromises. No, no, no. AI is just going to be able to figure them all out and solve them all. I don't think, I don't quite go that far. But I do think that there's an argument that AI will be able to identify more of these bottlenecks. There'll still be some bottlenecks, but it may be, and we saw how quickly chat GPT was adopted, that it's just, there's less frictions and it can do things a little faster. So that might shorten the time a little bit. But I think that the biggest upside from the technology is in identifying new things that we haven't done before. And sadly, I'm not good enough to know what those are yet. But history suggests that a lot of people, like I thought the radio ad example you gave was very apt, that, you know, I and everyone else is kind of stuck in seeing what the technology can do right now. And my natural instinct is to look at what humans are doing and replace it. But there are going to be people who identify some really new kinds of science, new ways of working together that unlock a lot more value. It just has completely changed the production possibilities frontier, and we haven't explored most of that yet. So I'm going to sort of give a little bit of an unsatisfying answer and say that those complementarities that are to be discovered are the ones that are to be discovered. And that is actually exactly why we are so focused on offering an API, like we're not just building chat GPT and our other products and saying, okay, well, those are working, so let's do that. We know that no matter how, you know, how successful we are, what works, what doesn't, we will only be able to build a tiny, the tiniest fraction of all of the possibilities with AI. And so with our API, what we're trying to do is basically get AI out to the world as cheaply as possible. Every time that we get gain, you know, we figure out how to make it more efficient to serve a particular model, or we're able to get the same level of intelligence at a lower cost. We give all those costs back to developers because everything we see is that, you pass some threshold and some other problem that people have that wasn't economically feasible when the AI was this expensive becomes feasible when it's this expensive. And so every time we drop the price and offer more intelligence, people can solve more problems. And we're excited to make that possible. And like it's, that's why we believe in the API.
Yeah. Let me just add to that. I think that is so smart. I'm so glad you're doing that, because it's a great answer to your question, that, you know, some people derisively call these things wrappers, where you take the core technology and you put something off on top of it to make it easier for a user. Actually, I think that's where a ton of the value is going to be coming, going forward, is somebody who figures out, let's customize this for a particular vertical. We need to understand this business's needs or this customer's needs. And that's where most of the value is. And so previous software, it was the same thing that, you know, there was 90% of the investment went into figuring out how to use the technology, not the core technology itself. So by you making that available, then there's going to be a flowering of entrepreneurs in all these different verticals that figure out the answer to your question. And that's ultimately where trillions of dollars of value will come from.
Eric, thank you so much for joining us.
My pleasure.